- 1 Nestlé Institute of Health Sciences, Lausanne, Switzerland
- 2 Faculty of Science, Aarhus University, Denmark
- 3 Nestlé Research Center, Lausanne, Switzerland
Comprehensive investigation of nutritional health effects at the molecular level requires the understanding of the interplay between three genomes, the food, the gut microbial, and the human host genome. Food genomes are researched for discovery and exploitation of macro- and micronutrients as well as specific bioactives, with those genes coding for bioactive proteins and peptides being of central interest. The human gut microbiota encompasses a complex ecosystem in the intestine with profound impact on host metabolism. It is being studied at genomic and, more recently, also at proteomic and metabonomic level. Humans are being characterized at the level of genetic pre-disposition and inter-individual variability in terms of (i) response to nutritional interventions and direction of health trajectories; (ii) epigenetic, metabolic programming at certain life stages with health consequences later in life and even for subsequent generations; and (iii) acute genomic expression as a holistic response to diet, monitored at gene transcript, protein and metabolite level. Modern nutrition science explores health-related aspects of bioactive food components, thereby promoting health, preventing, or delaying the onset of disease, optimizing performance and assessing benefits and risks in individuals and subpopulations. Personalized nutrition means adapting food to individual needs, depending on the human host’s life stage, -style, and -situation. Traditionally, nutrigenomics and nutri(epi)genetics are seen as the key sciences to understand human variability in preferences and requirements for diet as well as responses to nutrition. This article puts the three nutrition and health-relevant genomes into perspective, namely the food, the gut microbial and the human host’s genome, and calls for an “extended nutrigenomics” approach in order to build the future tools for personalized nutrition, health maintenance, and disease prevention. We discuss examples of these genomes, proteomes, transcriptomes, and metabolomes under the definition of genomics as the overarching term covering essentially all Omics rather than the sole study of DNA and RNA.
Introduction: Nutrition, Genomics, and Health
Food, drinks, and water are the physical matter we administer to our body, apart from the inhaled air and medical drugs. Nutrition represents therefore the strongest life-long environmental impact on human health. Contemporary nutrition research focuses on promoting health, preventing, or delaying the onset of disease, optimizing performance, and assessing benefits and risks (Kussmann et al., 2006). These directions require comprehensive strategies because: (a) food components interact with our body not only at system, but also at organ, cell, and molecular level (Kussmann and Daniel, 2008); and (b) nutritional improvement of one health aspect should be accompanied by preservation of health in all other respects (Kussmann and Fay, 2008).
Personalized nutrition means in practice adapting food to individual needs. Those food products that address already requirements or preferences of specific consumer groups are typically based on empirical research rather than molecular nutrition. Nutrigenomics and nutrigenetics build the scientific framework for understanding human genomic/genetic contributions to such preferences, requirements, and responses to diet and may develop into future means for consumers to assess their health and nutritional status (Kussmann and Daniel, 2008).
This paper attempts to embrace the complexity of nutrigenomics research, which ultimately requires a metagenomic approach, including the understanding of the interplay between three genomes: the food, the host and – being located at the interface – the gut microbial genome. We discuss examples of these genomes, proteomes, transcriptomes, and metabolomes under the definition of genomics as the overarching term covering essentially all Omics rather than the sole study of DNA and RNA. The scope of the article is depicted in Figure 1 that shows how for example the plant genome affects the human genome either through direct impact of its bioactives (incl. proteins and peptides), or indirectly via the gut microbial metabolism providing nutrients on which specific gut bacteria can feed.
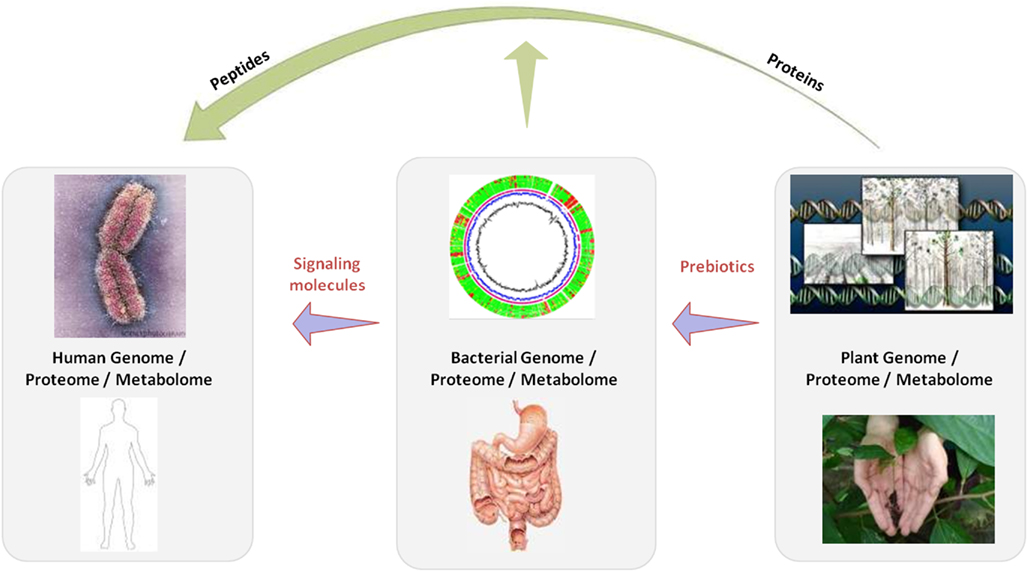
Figure 1. Extended nutrigenomics for nutrition and health: the plant genome for example affects the human genome either through direct impact of its bioactives (incl. proteins and peptides), or indirectly via the gut microbial metabolism providing nutrients on which specific gut bacteria can feed.
Food Genomes Encode for Bioactive Proteins and Peptides
Besides carbohydrates and lipids, proteins represent one of the three classes of macronutrients and are key actors in every cellular process. Enzymes are the proteins that catalyze virtually every metabolic reaction, for example the digestive proteins pepsin and (chymo)trypsin. Proteins also exhibit structural and mechanical functions: the cytoskeleton maintains cell shape; actin and myosin enable body motion. Moreover, proteins participate in cell signaling and immune response.
Food proteins are differently composed due to their origin, i.e., animal or plant source. Hence, a balanced diet contains proteins from different and complementary sources (e.g., meat, vegetables, cereals, grains, legumes) to ensure a balanced amino acid supply. The nutritional quality of proteins is assessed at the level of amino acid composition, protein digestibility, and absorptive ability. The body needs quite large amounts of protein in order to function efficiently, also because proteins are continuously synthesized and degraded, a phenomenon termed “protein turnover.”
Food proteomes are well characterized covering a broad range of animal and plant protein sources (Fong et al., 2008; Gao et al., 2009; Sakata et al., 2009). Beyond macronutrients, proteins, and peptides are increasingly appreciated because of their variety of bioactive components that exert functions as growth factors, anti-hypertensive agents, antimicrobials, modifiers of food intake, or immune regulators. Figure 2 summarizes some biological functions and activities of bioactive peptides released from various plant protein sources either by enzymatic digestion or through processing/fermentation: again, the example of the plant world is chosen with soy, rice, cereals, and sunflower as sources; through enzymatic digestion or fermentation, bioactive peptides can be released either in vitro (by processing) or in situ in vivo (upon digestion) that can exert various beneficial effects ranging from protection against excessive oxidative stress and even cancer; via cardiovascular to immune benefits.
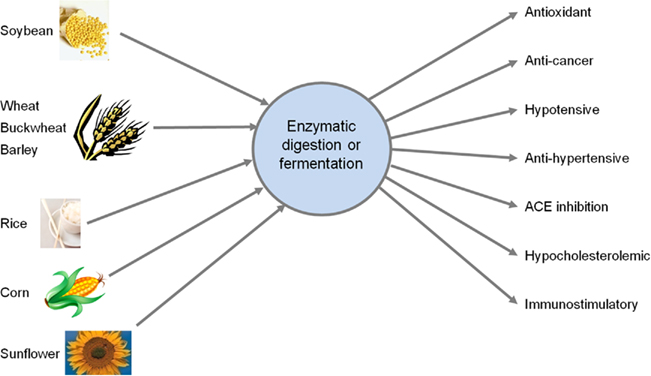
Figure 2. Functions of plant-derived bioactive peptides released by enzymatic digestion or fermentation: again, the example of the plant world is chosen with soy, rice, cereals, and sunflower as sources. Through enzymatic digestion or fermentation, bioactive peptides can be released either in vitro (by processing) or in situ in vivo (upon digestion) that can exert various beneficial effects ranging from protection against excessive oxidative stress and even cancer; via cardiovascular to immune benefits.
Biologically active motifs in polypeptide chains are fragments that are inactive as long as they “reside” in their precursor sequences, but upon release by proteolytic enzymes, they may interact with receptors and exert bioactivity (Schlimme and Meisel, 1995; Meisel and Bockelmann, 1999). Bioactive peptides may be released during the digestion by the host or microbial enzymes (Grigorov and van Bladeren, 2007). They can also be generated during food processing (industrial processing) or ripening (natural processing). In order to accurately address questions of bioavailability and bioefficacy, both systemically (i.e., in blood) and locally (e.g., in the stomach and gut), bioactive peptides and proteins should be identified and quantified all across from the food matrix to the target tissues in the body. Bioactive peptides and proteins have been discussed by Moller et al. (2008) and a database of bioactive peptides has been established for classification and potential bioactivity determination of food proteins (Minkiewicz et al., 2008).
Bioactive Peptides from Milk and Dairy
Human breast milk is the nutrient source that has co-evolved with humans to ideally support neonatal healthy growth and development and to favor the maturation and maintenance of a balanced immune system (Schack-Nielsen and Michaelsen, 2007). Milk bioactives derive from its proteins and peptides (Severin and Xia, 2005), lipids (German and Dillard, 2006), and oligosaccharides (Ninonuevo et al., 2006) and these are present in various mammalian species. The protein content of human milk can be split into caseins and whey with a 50:50 weight/weight ratio, while bovine milk consists of 80% caseins and 20% whey proteins (Severin and Xia, 2005). Caseins function as iron carriers and precursors of bioactive peptides, and whey proteins exhibit critical functions in immune modulation and defense (Madureira et al., 2007). Our research group has recently published a review on Omics approaches to unravel the protein/peptide, lipid, and carbohydrate content of human and animal milk (Casado et al., 2009). We intentionally focus here on potential and proven health-beneficial bioactive milk peptides and proteins. This said, dairy and other food proteins represent also a source of potential or proven food allergens and can therefore confer risks to susceptible consumers. We have discussed this in other reviews with a different scope (Kussmann et al., 2005, 2006, 2010).
Human milk mainly is composed of caseins, α-lactalbumin, lactoferrin, albumin, and various immunoglobulins and these predominant proteins account for >99% of the milk protein mass. The glycoprotein lactoferrin, an abundant mammalian iron-binding milk whey protein (Lampreave et al., 1990), profoundly influences the host defense system: it has been demonstrated to prevent microbial growth, by direct interaction between the protein and the Gram-negative bacterial membrane (Farnaud and Evans, 2003); in addition, lactoferrin and peptides derived from the full-length protein, have also been shown to influence cytokine production in cell cultures, which mimic immune and inflammatory actions of the body (Crouch et al., 1992). Human colostrum (“first milk”) has the highest concentration, followed by human milk, then cow milk (150 mg/L; Sanchez et al., 1992).
However, the remaining <1% of the human milk protein complement encompasses a complex mixture of bioactive proteins and peptides, which is far from being completely characterized and leveraged.
Human breast milk is the gold standard for neonate and infant nutrition. Secretory immunoglobulins, lysozyme, interferon, and growth factors, are known as immunological “assets” of breast milk. Breast milk partly promotes the inhibition of bacterial pathogens and favors the growth of a protective colonic microbiota (Levy, 1998). Apart from delivering basic nutrition to the newborn, milk also protects the neonate and the mammary gland against infection: breast-fed newborns experience normally a lower incidence of gastro-intestinal infections and inflammatory, respiratory, and allergic disorders. These benefits have been associated with diverse protective factors in breast milk. One such specific biological activity in mother’s milk was reported as the action of soluble CD14 (sCD14; Labeta et al., 2000; Vidal et al., 2001): the study revealed a key role for sCD14 during bacterial colonization of the gut and proposed sCD14 to be implicated in modulating local innate and adaptive immune responses, thereby controlling homeostasis in the neonatal intestine. Another related study unraveled an interaction between soluble Toll-like receptor 2 (sTLR2) and sCD14 in plasma and milk, suggesting a novel innate immune mechanism that may regulate microbially induced TLR triggering (LeBouder et al., 2003).
The potential roles of food-derived peptides in reducing cardiovascular disease risk have been reviewed by Erdmann et al. (2008) with respect to their beneficial properties for blood pressure, oxidative stress, homeostasis, appetite, and lipid metabolism. The beneficial effects of lactotripeptides on hypertension have been known for a long time: the tripeptides VPP and IPP can inhibit the angiotensin-converting enzyme (ACE) in vitro and are produced by fermentation of milk by Lactobacillus helveticus and Saccharomyces cerevisiae; this fermented milk was then fed to rats and resulted in lowered blood pressure (Masuda et al., 1996). Since that time, several randomized controlled human trials have showed an anti-hypertensive effect of VPP and IPP peptides derived from milk: in Finnish and Japanese subjects with (mild) hypertension, systolic blood pressure was found reduced by ca. 5 mmHg during 4–12 weeks of IPP + VPP supplementation (Geleijnse and Engberink, 2010). The same suggested effect of these lactotripeptides on blood pressure has more recently been (re-)examined in another six double-blind, placebo-controlled trials enrolling a total of 780 subjects with either high to normal blood pressure or untreated hypertension (UK and NL); intervention periods lasted 4–8 weeks, and IPP + VPP intake ranged from 2 to 10 mg/day; in contrast to the earlier trials cited above, little evidence for an anti-hypertensive effect of IPP + VPP and no ACE inhibition was found in these latter studies (Geleijnse and Engberink, 2010). Hence, while more recent data do not support a role for lactotripeptides in blood pressure regulation, one cannot exclude a beneficial effect in hypertensive subjects from specific populations such as the Finnish and Japanese (Geleijnse and Engberink, 2010).
The bioactivities of peptides “hidden” in major milk proteins are latent until released and activated, e.g., by means of food-technological treatment or during gastro-intestinal digestion: bioactive peptides can be produced in vivo following intake of milk proteins; and the proteolytic machinery of bacteria used in fermentation (e.g., yogurt, cheese) can contribute to the release of bioactive peptides or precursors thereof (Meisel, 2004). Autelitano et al. (2006) coined the term “cryptome” to allude to the “dormant” subset of peptides residing in the proteome; they promote the concept that these cryptic peptides, or “crypteins,” with their various bioactivities can be liberated from the parent protein via proteolytic cleavage. Proteomics is the obvious platform to study those “cryptomes.”
Bioactive Peptides from Soy
There is a current trend toward finding alternative sources of high-quality nutritious proteins either for reasons of economy (plants are cheaper than meat); ecology and sustainability (producing vegetable proteins is less resource demanding than for meat proteins); or consumer preference (vegans or vegetarians). Hence, the genomes of plant substitutes for meat protein including soy, wheat, or legumes are increasingly explored for their general nutritional value and content of specific bioactives.
Soybean is a geographically well established source of complete food protein that supplies high amounts of all essential amino acids. Its reported health benefits are mainly attributed to its isoflavones, saponins, proteins, and peptides (Erickson, 2005). The bioactive compounds are typically not the soy proteins as such but also the phytochemicals (Fang et al., 2004), and synergistic effects between both have been suggested. Lunasin, Bowman-Birk inhibitor, lectin, and beta-conglycinin are some of those biologically active soy proteins and peptides (Park et al., 2005): lunasin is a novel soy bioactive that can stop cancer cell division in a skin cancer mouse model and inhibit core histone acetylation in mammalian cells (de Lumen, 2005). Proteomic and metabolomic responses to soy, e.g., isoflavones, polyphenolics, and carotenoids were reviewed by Astle et al. (2007).
New approaches to more specifically separate and characterize bioactive peptides in soy are emerging: Beermann et al. (2009) investigated the anti-oxidative capacity of proteolytically released peptides from soybean protein isolates. These findings may open new nutritional applications because molecules with free-radical scavenging capabilities may prevent and treat oxidative stress-related diseases.
Bioactive Peptide Discovery – From Classical Screening to Reverse-Genome Engineering
There are in silico and in vitro methods to discover and identify (new or known) bioactive peptides in food sources. The classical in vitro approach starts with a functional fraction or extract, potentially containing bioactives. These peptide mixtures are typically tested in vitro, e.g., for their receptor-binding properties. Once an interesting fraction or extract has been identified, it undergoes further fractionation and subsequent proteomic and peptidomic analysis in order to narrow down and ultimately identify the active principle(s). The typically mass spectrometry-based proteomic and peptidomic pipeline must be adapted to the complexity and heterogeneity of full-length native bioactive peptides. The latter differ from the typically tryptic peptides that are more similar in size and in C-terminal terminal amino acids and are generated for protein identification in the biomarker business. We have reviewed proteomic and peptidomic tools for the discovery and characterization of bioactives and how these techniques differ from the classical protein biomarker discovery (Panchaud et al., 2008; Kussmann et al., 2010).
We and others increasingly follow another, complementary and bioinformatics-driven strategy to discover and leverage bioactive peptides (Figure 3): the approach has been coined “reverse-genome engineering” and enables in silico discovery and prediction of bioactive peptides (Grigorov and van Bladeren, 2007). First, the public scientific domain can be searched for to date known bioactive peptides and their (suggested) functions. These sequences can then be mapped onto suitable food genomes in the plant or animal world in order to unravel, where these peptides may be residing in parent protein sequences (Figure 3). Three sequence mapping techniques can be deployed for this purpose: local similarity, pattern, and motif/profile searches with the latter two offering greater sensitivity and specificity at the cost of slower speed. Local similarity searches weight the amino acids in the to-be-searched sequence equally, whereas the pattern search for example weights the amino acid differently according to their position along the sequence. In a further in silico analysis, either human digestive or food processing-related conditions can be mimicked to reveal the potential proteolytic release of the bioactive peptides from their parent sequences (Figure 3). The result of this bioinformatic, sequence-based approach is a set of known bioactive peptide sequences found in and potentially released from food genomes, which can then be followed up in vitro and in vivo. Evidently, this top-down approach can significantly reduce the number of bioactivity tests to be performed and help filter the nutritionally relevant and feasible candidates.
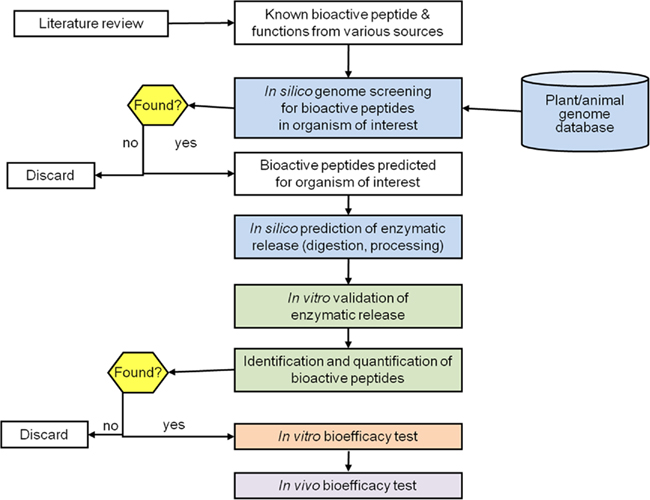
Figure 3. Workflow of bioinformatics-driven discovery of bioactive peptides. Blue: bioinformatics; green: peptidomics; orange: in vitro; purple: in vivo.
Ideally, one would like to expand from this literature knowledge-dependent approach (based on peptides with reported or suggested health benefits) to peptide sequences in general, but the prediction of bioactivity based on amino acid sequence alone is not (yet) feasible.
Gut Microbial Genomes
Humans and other mammals are colonized by a complex, and dynamic community of microorganisms. Adult humans are in terms of cell numbers more prokaryotic than eukaryotic with 90% of our cells estimated to be microbial, and only 10% human (Savage, 1977). The impact of these indigenous microbial consortia on human physiology is likely to be most important in the intestine, because this organ harbors by far most of these bacteria. The microbial densities in the proximal and middle small intestine are relatively low but increase sharply in the distal small intestine (∼108 bacteria/mL of luminal contents) and colon (1011–1012/g; Savage, 1977). The microbiota in the adult human body encompasses a huge biomass of >100,000 billion bacteria spread over >400 different species, which exhibit intense metabolic activity, predominantly in the colon, and play an important physiological role in the host (Bourlioux et al., 2003). Figure 4 shows where food compounds (macro- and micronutrients) are digested and absorbed in the gastro-intestinal tract (GIT) before they may reach various other body tissues. In the GIT, food stuffs interact with and are partly metabolized by an enormous quantity and diversity of bacteria residing in the stomach and, in particular, in the gut.
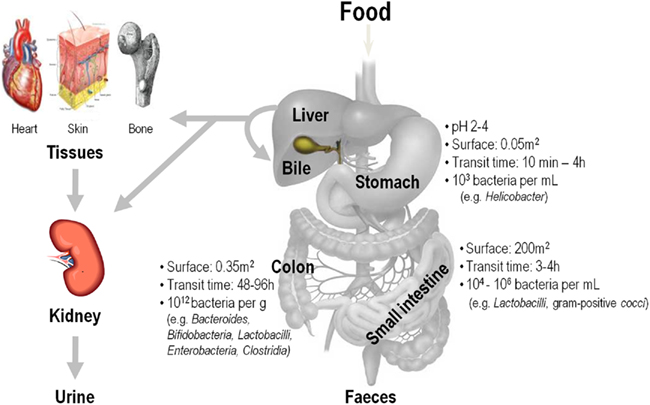
Figure 4. Food compounds (macro- and micronutrients) are digested and absorbed the gastro-intestinal tract (GIT) before they may reach various other body tissues. In the GIT, food stuffs interact with and are partly metabolized by an enormous quantity and diversity of bacteria residing in the stomach and, in particular, in the gut.
The gut microbes can be interpreted as a collective metabolically active “organ” affecting the host’s energy metabolism and immunity (Macpherson and Harris, 2004; Turnbaugh et al., 2006). This concept has been championed by the groups of Gordon (Hooper et al., 2002; Backhed et al., 2005) and Nicholson (Nicholson et al., 2005) and further pursued by ourselves (Rabot et al., 2010). The microbiota can degrade a variety of dietary substances that are otherwise non-digestible and therefore inaccessible to the host (Savage, 1986); one such example is the harvest of energy from carbohydrates (Hooper et al., 2002). Colonization of the gut by commensal bacteria has also been demonstrated to influence the host’s intestinal physiology by modulation of genes involved in nutrient absorption, gastro-intestinal and mucosal immune function and xenobiotic metabolism (Hooper and Gordon, 2001; Hooper et al., 2001). One of the central functions of the colonic microbiota is its capability to resist colonization by any external new strain of bacteria (Bourlioux et al., 2003).
It is an enormous challenge to simulate the complex bacterial–mucosal immune interaction deploying in vivo models. In one such attempt, Nicholls et al. (2003) elucidated metabolic events concomitant to acclimatization of germ-free (GF) rats to standard laboratory conditions. In order to unravel gut microbial effects under physiologically relevant conditions, animals with an a priori sterile GIT and then mono-colonized with probiotics are now used as a suitable model, especially the gnotobiotic mouse (Falk et al., 1998; Hooper and Gordon, 2001; Hooper et al., 2001), but also GF piglets (Danielsen et al., 2007). The latter model was recruited to investigate how bacterial colonization affects the porcine intestinal proteome (Danielsen et al., 2007): small-intestinal protein expression patterns in gnotobiotic pigs maintained GF or mono-associated with either Lactobacillus fermentum or non-pathogenic Escherichia coli revealed that bacterial colonization differentially affected proteolysis, epithelial proliferation and lipid metabolism, which confirms studies of other GF animal models.
Metabolomics is well suited and increasingly deployed to capture the metabolic interactions between the host metabolism and the microorganisms of, e.g., the colonic microbiota (Nicholson and Wilson, 2003). For example, metabolite profiling suggested a contribution of gut microbiota to the fatty-liver phenotype in insulin-resistant mice (Dumas et al., 2006): the relationship between gut microbiota and host metabolism with regard to diet-induced impaired glucose homeostasis and non-alcoholic fatty-liver disease (NAFLD) in a mouse strain susceptible to these traits was investigated by plasma and urine metabotyping; the genetic pre-disposition of the mouse strain to impaired glucose homeostasis and NAFLD was correlated with disturbed choline metabolism, with some of the choline metabolites being co-processed by symbiotic gut microbiota and mammalian enzyme systems.
Metabolomics also facilitated a correlation between the diversity of the human metabolic phenotype and diet and blood pressure, respectively (Holmes et al., 2008). Our research center has compared GF mice colonized by a human baby flora (HBF) or a normal flora to conventional mice at the level of microbiology; metabolic profiling by 1H NMR of liver, plasma, urine, and ileal flushes; and by targeted analysis of bile acids and short-chain fatty acids in the cecum (Martin et al., 2007). An association of specific metabotypes with the resident microbiome became apparent and revealed that the HBF has a remarkably simple microbiome/metabolome correlation network with a direct impact on the host’s ability to metabolize lipids. Overall, this study pointed out that the microbiome globally modulates absorption, storage, and the energy harvest from the diet.
Along the same lines, our group investigated the probiotic modulation of gut microbe–host interactions in a mouse model with a humanized microbiome (Martin et al., 2008): metabolic effects of exposure to either Lactobacillus paracasei or Lactobacillus rhamnosus were measured in HBF mice and generated a systems level view of the host response to probiotic intervention. Probiotic exposure led to microbiome modification and resulted in altered hepatic lipid metabolism accompanied by lowered plasma lipoprotein levels and apparent stimulated glycolysis.
In another microbiota study related to digestive disorders, our laboratory used a Trichinella spiralis-induced model of post-infective IBS to investigate the effects of probiotics on gut dysfunction at metabolome level (Martin et al., 2006). The plasma metabolic profile of T. spiralis-infected mice showed elevated energy metabolism and fat mobilization as well as a disruption of amino acid metabolism due to increased protein breakdown, which were related to the intestinal hypercontractility. Increased concentrations of taurine, creatine, and glycerophosphorylcholine in the jejunal muscles were associated with muscular hypertrophy and disrupted jejunal functions. L. paracasei treatment normalized the muscular activity and the disturbed energy metabolism. Lastly, altered levels of plasma metabolites (glutamine, lysine, methionine) possibly related to modulated immunological responses were also observed upon probiotic treatment.
The intestinal microbiota has also been investigated in the context of the host’s energy metabolism. However, whether different gut microbial populations are a cause or a consequence of for example deranged energy metabolism like in obese and diabetic subjects remains to be proven. Recent studies showed that GF mice are resistant to obesity when consuming a high-fat (HF), high-carbohydrate diet, but it remains elusive which mechanisms are involved in the anti-obesity phenotype and whether GF mice develop insulin resistance and dyslipidemia with HF feeding. To shed more light on this, our research laboratories compared the metabolic consequences of HF feeding on GF and conventional mice (Rabot et al., 2010): GF mice consumed fewer calories, excreted more fecal lipids, and weighed significantly less than conventional mice; GF/HF animals were both more insulin sensitive and glucose tolerant, showed reduced fasting and non-fasting insulinemia, and had reduced plasma TNF-α and total serum amyloid A levels. Reduced hypercholesterolemia, a moderate accretion of hepatic cholesterol, and an increase in fecal cholesterol excretion suggested an altered cholesterol metabolism in GF/HF mice. Our results demonstrated that lower calorie input and increased lipid excretion contributed to the obesity-resistant phenotype of GF/HF mice and revealed that insulin sensitivity and cholesterol metabolism are metabolic targets influenced by the gut microbiota. Moreover and most importantly, data on the link between the microbiota and obesity are also available in humans: Turnbaugh et al. (2006) characterized an obesity-associated gut microbiome with increased capacity for energy harvest (Ley et al., 2006): two groups of beneficial bacteria are dominant in the human gut, the Bacteroidetes and the Firmicutes. Ley et al. (2006) showed a relatively decreased proportion of Bacteroidetes in obese people compared to lean people and revealed that this proportion increases with weight loss on two types of low-calorie diet.
Our molecular understanding of how members of the intestinal microbiota degrade complex polysaccharides derives from studies of Bacteroides thetaiotaomicron, an abundant component of the normal human and mouse gut. Colonization of GF mice with B. thetaiotaomicron (Btheta) has shown how this anaerobe modifies intestinal cellular differentiation and gene expression to the mutual benefit of both the host and the microbe (Bry et al., 1996; Falk et al., 1998; Hooper et al., 2002). The Btheta proteome encompasses specific functions for polysaccharide acquisition and hydrolysis and a system that senses the environment (Sonnenburg et al., 2005). Compared to the gut commensal and probiotic Btheta, Bifidobacterium longum, a minor member but a commonly used probiotic, has a more restricted glycan-degradation machinery but a larger repertoire of transporters (Hooper et al., 2002) suggesting that B. longum may directly benefit from Btheta’s “upstream” polysaccharide degradation (Walker et al., 2006).
The gnotobiotic mouse model serves as a controlled situation that enables the study of how a resident gut bacterium and a probiotic species mutually adapt their substrate utilization. The model furthermore shows the many dimensions and the specificity of the relationship between the host, a generic component of its microbiota, and intentionally consumed microbes such as probiotics (Sonnenburg et al., 2006). The pioneering studies by Gordon et al. demonstrate that gut ecology is extremely complex and requires an ecosystem-level metagenomics approach to understand the health impact of the intestinal microbiota and probiotics (Falk et al., 1998; Xu and Gordon, 2003). Logically, nutrigenomics has therefore been extended via metagenomics toward metaproteomics collecting information from all three proteomes: host, food, and microbes. While techniques such as microarrays and high-throughput sequencing (Huyghe et al., 2008; Hamady and Knight, 2009) have delivered comprehensive data on the intestinal bacterial population structure (Turnbaugh et al., 2009), more recently the question has changed from “who is there?” to “who is doing what?,” i.e., from a population census (Turnbaugh and Gordon, 2009) to an activity profiling, the latter being facilitated by metaproteomic analyses (VerBerkmoes et al., 2009). The latter approach adds the proteomics-typical additional challenge of any (meta)proteome being much more complex than any (meta)genome.
The field of gut microbial ecology has provided a few first examples of trans-genomic interaction studies investigating cross-talk between intestinal microbiota and the human host via the host/guest metabolism. These studies do not necessarily take a whole-genome approach but nevertheless embark in principle on the interaction of the three “Omes,” namely the intestinal metabolome, the host’s genome and the microbiome. Biagi et al. (2011) for example delivered a metagenomic perspective of the aging process in the human gut: they introduce human beings as “meta-organisms” with a more holistic view of the aging process and the interaction between environment, intestinal microbiota and host taken into consideration. Age-related physiological changes of the GIT, the lifestyle, nutritional behavior, and the host’s immune system affect the gut microbial ecosystem. Biagi et al. (2011) review the current knowledge of gut microbial changes in aging people and propose age-related gut microbial unbalances to be involved in “inflamm-aging” and immunosenescence. In view of the importance of gut microbiota homeostasis for host health they consider medical and nutritional applications based on probiotic and prebiotic preparations specific for the elderly. They also review the few clinical intervention trials reporting the use of pre-/probiotics in the elderly.
Ng et al. (2010) investigated the relationship between human intestinal dendritic cells (DCs), gut microbiota, and Crohn’s disease (CD) activity. They found that intestinal DC IL-6 production is increased in CD patients and correlated with disease activity and C-reactive protein (CRP). In terms of host–microbe interaction, they suggest that bacterially driven local IL-6 production by host intestinal DC may result in unopposed effector function and tissue damage. This would mean that intestinal DC function can be influenced by the commensal microbiota composition.
Pruteanu et al. (2011) studied the degradation of the extracellular matrix components by bacterial-derived metalloproteases and possible implications for inflammatory bowel disease (IBD). Proteolytic degradation of the extracellular matrix is a hallmark of mucosal homeostasis and tissue renewal, but also contributes to complications of intestinal inflammation. It is not known, whether this is entirely host-derived, or, in part, exhibited by the gut microbiota. Therefore, Pruteanu et al. (2011) screened fecal bacterial colonies from 20 healthy controls, 23 subjects with ulcerative colitis (UC), and 18 CD patients for gelatinolytic activity. Metalloprotease genes were detected by PCR. The physiological significance and potential implications of such microbe-driven matrix degradation were confirmed by reduced transepithelial resistance (TER) after exposure of rat distal colon to culture supernatants of C. perfringens. The group concluded that microbial proteolytic activity has the capacity to contribute to mucosal homeostasis and may participate in the pathogenesis of IBD.
The Human Genome
Healthy adult humans vary considerably in their appearance, physical, and cognitive performance and also in their food preferences and requirements. Nutrition science has recognized that different life situations and phases require adequately adapted food intake: so do pregnant women, active athletes, and elderly people have very distinct and specific dietary requirements. Individual food choices and personalized solutions have a long history of being a consequence of preference, culture, life stage; and also, as more recently discovered, life style, disease, and genetics.
Genetics to Clarify Pre-Disposition to Diet and Health
Nutrigenetics investigates the genetic pre-disposition of an individual with regard to its response to diet. Single-nucleotide polymorphisms (SNPs) are single-base mutations in genes and are considered today as the most common and important inter-individual genetic differences at gene sequence level (Zhao et al., 2004). This said, also other genetic modifications such as deletions (Smith et al., 2008), insertions (Payton et al., 2005) and copy number variants (CNVs; Need et al., 2009), are important and contribute to inter-individual variability in humans. SNPs can occur in both exons and introns, i.e., in coding and non-coding DNA regions, and some have important functional consequences (Nelson et al., 2004). We believe that hypothesis-driven approaches to assess variants of specific, metabolically relevant genes in subjects enrolled in nutritional interventions can improve study design and readout. One such promising scenario is based on probing genetic variants in G-proteins (heterotrimeric guanine – binding proteins), for the following reasons:
G-proteins are expressed in all cells of the human body. Their main function is to translate signals from the cell surface into a cellular response (Bourne, 1997). They represent a signaling funnel of some 30 proteins, positioned between thousands of extracellular signals, hundreds of membrane receptors and again thousands of intracellular effector molecules. G-proteins are heterotrimers and consist of α-, β-, and γ -subunits encoded by different genes (Downes and Gautam, 1999). This α-, β-, γ-subunit composition determines their receptor and effector specificity (Gautam et al., 1998). Given the crucial role of G-proteins in virtually all intracellular signal transduction processes, it has been shown that mutations altering the expression or structure of these proteins can contribute to or even cause health disorders (Siffert, 2005).
Metabolically relevant SNPs in G-proteins have been extensively characterized (Gutersohn et al., 2000; Wenzel et al., 2002; Hauner et al., 2003; Nurnberger et al., 2003; Holtmann et al., 2004; Siffert, 2005; Mitchell et al., 2006; Frey et al., 2008). G protein gene variants have been shown to directly impact weight regulation (Gutersohn et al., 2000; Hauner et al., 2003; Frey et al., 2008): Hauner et al., 2003 genotyped 111 participants of a randomized placebo-controlled clinical trial for the GNB3 C825T polymorphism and analyzed associations of genotypes with outcome of a structured weight loss program with administration of either placebo or 15 mg Sibutramine daily for 54 weeks; the C825T polymorphism in the β3-subunit encoded by the gene GNB3 could predict whether a weight reduction under Sibutramine therapy would be successful or not. A similar observation has been made for the GNAS gene that mediates cAMP increase in several cell types after hormonal stimulation. The GNAS G(-1211) A genotype, too, influences the individual response to Sibutramine-induced weight loss intervention. Lastly, the GNA11 G(-659)C genotype in the G protein Ga11 has also a strong influence on the Sibutramine intervention (Frey et al., 2008). Taken together these results suggest that G protein-directed genotyping may render nutritional intervention more specific and powerful.
Most G protein studies in Caucasian populations reveal a positive association between 825T allele carrier status in the GNB3 subunit gene on the one hand and increased risk for hypertension on the other hand (Nurnberger et al., 2003). This has been shown in particular for large populations genotyped within a German (Siffert, 2005) and Belgian study. Moreover, evidence emerges that the 825T allele frequency is increased in hypertensive patients with typical features of the metabolic syndrome (Siffert, 2005): young, lean, normotensive male 825T allele carriers already reveal first signs of the metabolic syndrome, such as increased total cholesterol and uric acid concentration, but not yet insulin resistance (similar metabolic derangements were found in older Europeans and Japanese; Siffert, 2005). In older hypertensive subjects possessing the TT or TC genotype of GNB3, insulin resistance seems to be more frequent than in CC genotype individuals. Overall, the studies discussed above raise the question whether hypertension associated with the C825T polymorphism may be just one facet of the metabolic syndrome. The 825T association with increased risk for type 2 diabetes mellitus (T2DM) remains to be proved (Siffert, 2005).
In view of the evidence outlined above, genotyping healthy adult volunteers enrolled in weight management trials at the G protein level appears promising and complementary to probing other metabolically relevant genes for subject characterization and cohort stratification.
A more medical and disease-preventive nutrigenetic context is given by the genetic basis of neural tube defects (NTD), cancer and their correlation with folate and methionine metabolism: a C → T substitution in the gene encoding methylene-tetrahydrofolate-reductase (MTHFR), the enzyme that produces 5-methyl-tetrahydrofolate (5-methyl-THF) required for the conversion of homocysteine to methionine, is quite common. Individuals with the T/T genotype display functional metabolic effects, e.g., changes in one-carbon folate derivatives, elevated plasma homocysteine and different response to folic acid supplementation compared with normal (C/C) or heterozygous (C/T) genotypes. These metabolic changes associated with the T/T genotype can modify risk for chronic disease (e.g., vascular disease and cancer) and NTD if they coincide with folate deficiency (Bailey and Gregory III, 1999). Bailey and Gregory III (1999) discussed MTHFR polymorphisms in terms of metabolic significance, risk, and impact on folate requirement. From the observed modulation of these metabolic deviations by increasing folate intake they conclude that folate requirements may be different in affected individuals (T/T) relative to normal (C/C) or heterozygous (C/T) individuals.
Effective folate supplementation prevents NTD occurrence. Together with the high blood homocysteine levels in NTD children’s mothers, this suggests folate genes [methylene-tetrahydrofolate-reductase (MTHFR) and -dehydrogenase (MTHFD)] and homocysteine metabolism genes (methionine synthase; methionine synthase reductase; cystathionine synthase) to be involved in NTD etiology. Gos Jr. and Szpecht-Potocka, 2002 reviewed this topic and presented a list of folate and homocysteine metabolism genes known to be involved in NTD with an emphasis on primary and secondary NTD prevention.
Epigenetics to Understand Metabolic Programming
Epigenetics means “above the genetics” and alludes to mechanisms that control gene expression without changing the DNA sequence. Epigenetic changes encompass molecular modifications to both DNA and chromatin (Klose and Bird, 2006; Talbert and Henikoff, 2006). DNA methylation and changes to chromatin packaging of DNA by post-translational modifications of histone proteins are the most extensively investigated mechanisms (Klose and Bird, 2006; Talbert and Henikoff, 2006). Other epigenetic events include regulation by non-coding RNAs, such as microRNAs, and mechanisms that control the higher-level organization of chromatin within the nucleus (Jirtle and Skinner, 2007). Apart from the relevance for environmental programming, epigenetic mechanisms are first and foremost central elements of development and differentiation: epigenetic regulation is involved in tissue-specific gene expression and silencing (Slotkin and Martienssen, 2007).
Epigenetics provides a biochemical framework for long-term effects of environmental experiences, metabolic programming and adaptive development (Jirtle and Skinner, 2007). Epigenetic changes happen most commonly during gestation, neonatal development, puberty, and old age. During early embryogenesis, the mammalian genome is “cleaned” of most epigenetic marks, with the latter then being progressively re-established during embryonic development (Gluckman et al., 2009). The epigenome is therefore environmentally most vulnerable during embryogenesis. Epigenetic marks may be inherited from one generation to the next, either directly by preservation through meiosis or, indirectly in the next generation, through replication of the conditions, in which the epigenetic change occurred in the first place (Gluckman et al., 2009). These observations pile up to substantial evidence for the developmental origins of health and disease with underlying epigenetic mechanisms (Gluckman et al., 2009).
Characterizing the expression profiles of epigenetically controlled genes should reveal early biomarkers for disease, exposure, intervention, and efficacy. In a second phase, these markers should translate into early diagnosis of individuals with a propensity for adult-onset disease and may ultimately deliver novel therapeutic approaches that prevent and treat diseases before classical symptoms become visible. This contrasts with current human disease management, which typically aims at treating and curing disease after its onset. In this regard, epigenetics can be expected to play a key role in nutrition: modern nutrition research focuses on disease prevention and health preservation. Ultimately, comprehensive knowledge of the human epigenome will be required, as the epigenome is not only tissue and stage-of-life dependent, but also varies markedly between individuals and species.
Current mechanistic evidence for environmental epigenetic programming, by nutritional or other routes, has been mainly generated in animal studies. But also human epidemiology increasingly highlights associations between environmental impact – especially prenatal and early postnatal – and long-term epigenetic modifications manifesting in health and disease phenotypes (Yajnik, 2004; Painter et al., 2005). Developmental plasticity generally manifests when environmental exposure produces a broad range of adult phenotypes from a single genotype by epigenetically altering gene expression (Boney et al., 2005; Singhal, 2006); but developmental plasticity does not only allow an organism to adapt to environmental signals during early life; it can also increase the risk of chronic diseases, namely in the case of a mismatch between the early perceived environment and the one encountered later in adulthood.
Developing organisms appear to be particularly susceptible to epigenetic changes. The effects of suboptimal nutrition during the peri-conceptional period shows the “epigenetic sensitivity” of this developmental phase (Sinclair et al., 2007; Heijmans et al., 2008), in which widespread re-programming of the epigenome occurs (Nafee et al., 2008). But also nutritional constraints later in pregnancy (Vickers et al., 2000; Painter et al., 2005), postnatal over-nutrition leading to rapid growth (Vickers et al., 2000; Singhal, 2006), and maternal–fetal over-nutrition (Schaefer-Graf et al., 2005) can cause metabolic deregulation later in life. For each of these situations, epigenetic changes have been reported (Waterland et al., 2006; Gluckman et al., 2007; Aagaard-Tillery et al., 2008). The importance of the peri-conceptional period, when suboptimal nutrition can have long-lasting effects without affecting birth weight (Painter et al., 2005; Heijmans et al., 2008), underscores the need for healthy nutrition during the pre-pregnancy period, when nutrition may often be unbalanced, even for women in wealthy countries (Robinson et al., 2004). So does the related finding of (im)balanced intake of micronutrients involved in one-carbon metabolism during pregnancy affecting the subsequent metabolic health of the offspring (Yajnik et al., 2008) have implications for nutritional supplementation. Research in animal models went beyond analysis and has demonstrated that such adverse effects of impaired early life nutrition and the associated epigenetic changes can be even prevented (Lillycrop et al., 2005) or reversed (Vickers et al., 2005; Gluckman et al., 2007) by nutritional interventions (such as folate supplementation) or endocrinological interventions (such as neonatal leptin administration).
In our view, epigenetics in nutrition should play an important role in investigating mechanistic underpinnings of long-term human health effects of nutrients, ingredients and, possibly, entire diets. In more fundamental studies relying on animal models, the epigenetic dimension of, e.g., nutritionally promoting lean body mass and of dietary mimicking the life-prolonging effects of caloric restriction could be explored.
Conclusion
The food genomes interact with the human genome, either directly or mediated by the interfacing gut microbial metagenome. Polyunsaturated fatty acids for example directly bind to particular transcription factors (such as PPARs) that in turn switch on a gene expression machinery – a most direct interaction between a food component and the host genome. On the other hand, human milk-derived oligosaccharides favor the colonization of the infant gut by health-beneficial bacteria that in turn impact the host’s metabolism – an indirect, but powerful gut microbiota-mediated interaction between a food compound class and the host genome resulting in a symbiotic relationship between food stuff and intestinal bacteria.
This perspective and these examples illustrate that we need to understand the regulation and variability of the human genome as a response to its exposure to the food genomes (f. ex. bioactive dairy peptides) in conjunction with the host’s gut microbiome:
– Functional food with added health value starts with a holistic, genomic perspective of how we can leverage the richness of bioactives derived from food sources, with milk-derived bioactive peptides being a prominent example – this refers to the bioinformatic and proteomic exploitation of the food genomes.
– Then we need to see where, when, and how these bioactives interact with the human body, either directly, or metabolized by either the host or the gut microbiome, and how these interactions translate into physiological consequences – this refers to the Omics-level elucidation of nutritional health effects.
– As an important dimension of this food–host interaction, we need to take the human gut microbiome as a metabolic organ into account, a perspective which ultimately requires a metagenomic approach.
– In a further step, the inter-individual differences in human responses to diet should be understood – this can be partly addressed through genotyping for metabolically relevant genes.
– We should monitor long-term health consequences of (early) nutrition and try to understand, in which tissues and at which molecular level these effects may be mediated by epigenetic mechanisms.
Whereas gene and protein expression studies as well as metabolite profiling in humans investigating (rather acute) responses to nutritional interventions have become standard for mechanistic nutritional research (and are reviewed elsewhere by us and others), both validation and translation of the results are still challenging. There are emerging examples of functionally impacting polymorphisms in metabolically relevant genes that determine whether or not, or to which extent an individual benefits from a specific diet or ingredient. However, nutrigenetics-based foods are still to be developed. Finally, we are only at the beginning to appreciate how epigenetic programming is not only a natural part of development and differentiation but also a mechanism of long-term gene expression changes that result in “metabolic memory.”
In our view, current and future molecular food research should ask precise nutritional questions; answer them in highly defined and controlled studies all across from well-defined diets and ingredients to standardized genomic readouts; and, most importantly, assess the outcomes with a multitude of holistic genomic and genetic tools, combined with extensive phenotyping.
Conflict of Interest Statement
The authors declare that the research was conducted in the absence of any commercial or financial relationships that could be construed as a potential conflict of interest.
Acknowledgments
We thank Catherine Ngom-Bru and Alexandre Panchaud for their expert advice on bioactive peptide discovery.
References
Aagaard-Tillery, K. M., Grove, K., Bishop, J., Ke, X., Fu, Q., McKnight, R., and Lane, R.H. (2008). Developmental origins of disease and determinants of chromatin structure: maternal diet modifies the primate fetal epigenome. J. Mol. Endocrinol. 41, 91–102.
Astle, J., Ferguson, J. T., German, J. B., Harrigan, G. G., Kelleher, N. L., Kodadek, T., Parks, B. A., Roth, M. J., Singletary, K. W., Wenger, C. D., and Mahady, G. B. (2007). Characterization of proteomic and metabolomic responses to dietary factors and supplements. J. Nutr. 137, 2787–2793.
Autelitano, D. J., Rajic, A., Smith, A. I., Berndt, M. C., Ilag, L. L., and Vadas, M. (2006). The cryptome: a subset of the proteome, comprising cryptic peptides with distinct bioactivities. Drug Discov. Today 11, 306–314.
Backhed, F., Ley, R. E., Sonnenburg, J. L., Peterson, D. A., and Gordon, J. I. (2005). Host-bacterial mutualism in the human intestine. Science 307, 1915–1920.
Bailey, L. B., and Gregory, J. F. III. (1999). Polymorphisms of methylenetetrahydrofolate reductase and other enzymes: metabolic significance, risks and impact on folate requirement. J. Nutr. 129, 919–922.
Beermann, C., Euler, M., Herzberg, J., and Stahl, B. (2009). Anti-oxidative capacity of enzymatically released peptides from soybean protein isolate. Eur. Food Res. Technol. 229, 637–644.
Biagi, E., Candela, M., Fairweather-Tait, S., Franceschi, C., and Brigidi, P. (2011). Ageing of the human metaorganism: the microbial counterpart. Age (Dordr.) doi:10.1007/s11357-011-9217-5. [Epub ahead of print].
Boney, C. M., Verma, A., Tucker, R., and Vohr, B. R. (2005). Metabolic syndrome in childhood: association with birth weight, maternal obesity, and gestational diabetes mellitus. Pediatrics 115, e290–e296.
Bourlioux, P., Koletzko, B., Guarner, F., and Braesco, V. (2003) The intestine and its microflora are partners for the protection of the host: report on the Danone symposium “the intelligent intestine,” held in Paris, June 14, 2002. Am. J. Clin. Nutr. 78, 675–683.
Bry, L., Falk, P. G., Midtvedt, T., and Gordon, J. I. (1996). A model of host-microbial interactions in an open mammalian ecosystem. Science 273, 1380–1383.
Casado, B., Affolter, M., and Kussmann, M. (2009). OMICS-rooted studies of milk proteins, oligosaccharides and lipids. J. Proteomics 73, 196–208.
Crouch, S. P., Slater, K. J., and Fletcher, J. (1992). Regulation of cytokine release from mononuclear cells by the iron-binding protein lactoferrin. Blood 80, 235–240.
Danielsen, M., Hornshoej, H., Siggers, R. H., Jensen, B. B., van Kessel, A. G., and Bendixen, E. (2007). Effects of bacterial colonization on the porcine intestinal proteome. J. Proteome Res. 6, 2596–2604.
Dumas, M. E., Barton, R. H., Toye, A., Cloarec, O., Blancher, C., Rothwell, A., Fearnside, J., Tatoud, R., Blanc, V., Lindon, J. C., Mitchell, S. C., Holmes, E., McCarthy, M. I., Scott, J., Gauguier, D., and Nicholson, J. K. (2006). Metabolic profiling reveals a contribution of gut microbiota to fatty liver phenotype in insulin-resistant mice. Proc. Natl. Acad. Sci. U.S.A. 103, 12511–12516.
Erdmann, K., Cheung, B. W., and Schroder, H. (2008). The possible roles of food-derived bioactive peptides in reducing the risk of cardiovascular disease. J. Nutr. Biochem. 19, 643–654.
Falk, P. G., Hooper, L. V., Midtvedt, T., and Gordon, J. I. (1998). Creating and maintaining the gastrointestinal ecosystem: what we know and need to know from gnotobiology. Microbiol. Mol. Biol. Rev. 62, 1157–1170.
Fang, N., Yu, S., and Badger, T. M. (2004). Comprehensive phytochemical profile of soy protein isolate. J. Agric. Food Chem. 52, 4012–4020.
Farnaud, S., and Evans, R. W. (2003). Lactoferrin-a multifunctional protein with antimicrobial properties. Mol. Immunol. 40, 395–405.
Fong, B. Y., Norris, C. S., and Palmano, K. P. (2008). Fractionation of bovine whey proteins and characterisation by proteomic techniques. Int. Dairy J. 18, 23–46.
Frey, U. H., Hauner, H., Jockel, K. H., Manthey, I., Brockmeyer, N., and Siffert, W. (2008). A novel promoter polymorphism in the human gene GNAS affects binding of transcription factor upstream stimulatory factor 1, Galphas protein expression and body weight regulation. Pharmacogenet. Genomics 18, 141–151.
Gao, L., Wang, A., Li, X., Dong, K., Wang, K., Appels, R., Ma, W., and Yan, Y. (2009). Wheat quality related differential expressions of albumins and globulins revealed by two-dimensional difference gel electrophoresis (2-D DIGE). J. Proteomics 73, 279–296.
Gautam, N., Downes, G. B., Yan, K., and Kisselev, O. (1998). The G-protein betagamma complex. Cell. Signal. 10, 447–455.
Geleijnse, J. M., and Engberink, M. F. (2010). Lactopeptides and human blood pressure. Curr. Opin. Lipidol. 21, 58–63.
German, J. B., and Dillard, C. J. (2006). Composition, structure and absorption of milk lipids: a source of energy, fat-soluble nutrients and bioactive molecules. Crit. Rev. Food Sci. Nutr. 46, 57–92.
Gluckman, P. D., Hanson, M. A., Buklijas, T., Low, F. M., and Beedle, A. S. (2009). Epigenetic mechanisms that underpin metabolic and cardiovascular diseases. Nat. Rev. Endocrinol. 5, 401–408.
Gluckman, P. D., Lillycrop, K. A., Vickers, M. H., Pleasants, A. B., Phillips, E. S., Beedle, A. S., Burdge, G. C., and Hanson, M. A. (2007). Metabolic plasticity during mammalian development is directionally dependent on early nutritional status. Proc. Natl. Acad. Sci. U.S.A. 104, 12796–12800.
Gos, M. Jr., and Szpecht-Potocka, A. (2002). Genetic basis of neural tube defects. II. Genes correlated with folate and methionine metabolism. J. Appl. Genet. 43, 511–524.
Grigorov, M. G., and van Bladeren, P. J. (2007). Functional peptides by genome reverse engineering. Curr. Opin. Drug Discov. Devel. 10, 341–346.
Gutersohn, A., Naber, C., Muller, N., Erbel, R., and Siffert, W. (2000). G protein beta3 subunit 825 TT genotype and post-pregnancy weight retention. Lancet 355, 1240–1241.
Hamady, M., and Knight, R. (2009). Microbial community profiling for human microbiome projects: tools, techniques, and challenges. Genome Res. 19, 1141–1152.
Hauner, H., Meier, M., Jockel, K. H., Frey, U. H., and Siffert, W. (2003). Prediction of successful weight reduction under sibutramine therapy through genotyping of the G-protein beta3 subunit gene (GNB3) C825T polymorphism. Pharmacogenetics 13, 453–459.
Heijmans, B. T., Tobi, E. W., Stein, A. D., Putter, H., Blauw, G. J., Susser, E. S., Slagboom, P. E., and Lumey, L. H. (2008). Persistent epigenetic differences associated with prenatal exposure to famine in humans. Proc. Natl. Acad. Sci. U.S.A. 105, 17046–17049.
Holmes, E., Loo, R. L., Stamler, J., Bictash, M., Yap, I. K., Chan, Q., Ebbels, T., De Iorio, M., Brown, I. J., Veselkov, K. A., Daviglus, M. L., Kesteloot, H., Ueshima, H., Zhao, L., Nicholson, J. K., and Elliott, P. (2008). Human metabolic phenotype diversity and its association with diet and blood pressure. Nature 453, 396–400.
Holtmann, G., Siffert, W., Haag, S., Mueller, N., Langkafel, M., Senf, W., Zotz, R., and Talley, N. J. (2004). G-protein beta 3 subunit 825 CC genotype is associated with unexplained (functional) dyspepsia. Gastroenterology 126, 971–979.
Hooper, L. V., and Gordon, J. I. (2001). Commensal host-bacterial relationships in the gut. Science 292, 1115–1118.
Hooper, L. V., Midtvedt, T., and Gordon, J. I. (2002). How host-microbial interactions shape the nutrient environment of the mammalian intestine. Annu. Rev. Nutr. 22, 283–307.
Hooper, L. V., Wong, M. H., Thelin, A., Hansson, L., Falk, P. G., and Gordon, J. I. (2001). Molecular analysis of commensal host-microbial relationships in the intestine. Science 291, 881–884.
Huyghe, A., Francois, P., Charbonnier, Y., Tangomo-Bento, M., Bonetti, E. J., Paster, B. J., Bolivar, I., Baratti-Mayer, D., Pittet, D., and Schrenzel, J. (2008). Novel microarray design strategy to study complex bacterial communities. Appl. Environ. Microbiol. 74, 1876–1885.
Jirtle, R. L., and Skinner, M. K. (2007). Environmental epigenomics and disease susceptibility. Nat. Rev. Genet. 8, 253–262.
Klose, R. J., and Bird, A. P. (2006). Genomic DNA methylation: the mark and its mediators. Trends Biochem. Sci. 31, 89–97.
Kussmann, M., Affolter, M., and Fay, L. B. (2005). Proteomics in nutrition and health. Comb. Chem. High Throughput. Screen. 8, 679–696.
Kussmann, M., and Fay, L. B. (2008). Nutrigenomics and personalized nutrition: science and concept. Per. Med. 5, 447–455.
Kussmann, M., Panchaud, A., and Affolter, M. (2010). Proteomics in nutrition: status quo and outlook for biomarkers and bioactives. J. Proteome Res. 9, 4876–4887.
Kussmann, M., Raymond, F., and Affolter, M. (2006). OMICS-driven biomarker discovery in nutrition and health. J. Biotechnol. 124, 758–787.
Labeta, M. O., Vidal, K., Nores, J. E., Arias, M., Vita, N., Morgan, B. P., Guillemot, J. C., Loyaux, D., Ferrara, P., Schmid, D., Affolter, M., Borysiewicz, L. K., Donnet-Hughes, A., and Schiffrin, E. J. (2000). Innate recognition of bacteria in human milk is mediated by a milk-derived highly expressed pattern recognition receptor, soluble CD14. J. Exp. Med. 191, 1807–1812.
Lampreave, F., Pineiro, A., Brock, J. H., Castillo, H., Sanchez, L., and Calvo, M. (1990). Interaction of bovine lactoferrin with other proteins of milk whey. Int. J. Biol. Macromol. 12, 2–5.
LeBouder, E., Rey-Nores, J. E., Rushmere, N. K., Grigorov, M., Lawn, S. D., Affolter, M., Griffin, G. E., Ferrara, P., Schiffrin, E. J., Morgan, B. P., and Labéta, M. O. (2003). Soluble forms of Toll-like receptor (TLR)2 capable of modulating TLR2 signaling are present in human plasma and breast milk. J. Immunol. 171, 6680–6689.
Ley, R. E., Turnbaugh, P. J., Klein, S., and Gordon, J. I. (2006). Microbial ecology: human gut microbes associated with obesity. Nature 444, 1022–1023.
Lillycrop, K. A., Phillips, E. S., Jackson, A. A., Hanson, M. A., and Burdge, G. C. (2005). Dietary protein restriction of pregnant rats induces and folic acid supplementation prevents epigenetic modification of hepatic gene expression in the offspring. J. Nutr. 135, 1382–1386.
Macpherson, A. J., and Harris, N. L. (2004). Interactions between commensal intestinal bacteria and the immune system. Nat. Rev. Immunol. 4, 478–485.
Madureira, A. R., Pereira, C. I., Gomes, A.-M. P., Pintado, M. E., and Malcata, F. X. (2007). Bovine whey proteins – overview on their main biological properties. Food Res. Int. 40, 1197–1211.
Martin, F. P., Dumas, M. E., Wang, Y., Legido-Quigley, C., Yap, I. K., Tang, H., Zirah, S., Murphy, G. M., Cloarec, O., Lindon, J. C., Sprenger, N., Fay, L. B., Kochhar, S., van Bladeren, P., Holmes, E., and Nicholson, J. K. (2007). A top-down systems biology view of microbiome-mammalian metabolic interactions in a mouse model. Mol. Syst. Biol. 3, 112.
Martin, F. P., Verdu, E. F., Wang, Y., Dumas, M. E., Yap, I. K., Cloarec, O., Bergonzelli, G. E., Corthesy-Theulaz, I., Kochhar, S., Holmes, E., Lindon, J. C., Collins, S. M., and Nicholson, J. K. (2006). Transgenomic metabolic interactions in a mouse disease model: interactions of Trichinella spiralis infection with dietary Lactobacillus paracasei supplementation. J. Proteome Res. 5, 2185–2193.
Martin, F. P., Wang, Y., Sprenger, N., Yap, I. K., Lundstedt, T., Lek, P., Rezzi, S., Ramadan, Z., van Bladeren, P., Fay, L. B., Kochhar, S., Lindon, J. C., Holmes, E., and Nicholson, J. K. (2008). Probiotic modulation of symbiotic gut microbial-host metabolic interactions in a humanized microbiome mouse model. Mol. Syst. Biol. 4, 157.
Masuda, O., Nakamura, Y., and Takano, T. (1996). Antihypertensive peptides are present in aorta after oral administration of sour milk containing these peptides to spontaneously hypertensive rats. J. Nutr. 126, 3063–3068.
Meisel, H., and Bockelmann, W. (1999). Bioactive peptides encrypted in milk proteins: proteolytic activation and thropho-functional properties. Antonie Van Leeuwenhoek 76, 207–215.
Minkiewicz, P., Dziuba, J., Iwaniak, A., Dziuba, M., and Darewicz, M. (2008). BIOPEP database and other programs for processing bioactive peptide sequences. J. AOAC Int. 91, 965–980.
Mitchell, A., Rushentsova, U., Siffert, W., Philipp, T., and Wenzel, R. R. (2006). The angiotensin II receptor antagonist valsartan inhibits endothelin 1-induced vasoconstriction in the skin microcirculation in humans in vivo: influence of the G-protein beta3 subunit (GNB3) C825T polymorphism. Clin. Pharmacol. Ther. 79, 274–281.
Moller, N. P., Scholz-Ahrens, K. E., Roos, N., and Schrezenmeir, J. (2008). Bioactive peptides and proteins from foods: indication for health effects. Eur. J. Nutr. 47, 171–182.
Nafee, T. M., Farrell, W. E., Carroll, W. D., Fryer, A. A., and Ismail, K. M. (2008). Epigenetic control of fetal gene expression. BJOG 115, 158–168.
Need, A. C., Attix, D. K., McEvoy, J. M., Cirulli, E. T., Linney, K. L., Hunt, P., Ge, D., Heinzen, E. L., Maia, J. M., Shianna, K. V., Weale, M. E., Cherkas, L. F., Clement, G., Spector, T. D., Gibson, G., and Goldstein, D. B. (2009). A genome-wide study of common SNPs and CNVs in cognitive performance in the CANTAB. Hum. Mol. Genet. 18, 4650–4661.
Nelson, M. R., Marnellos, G., Kammerer, S., Hoyal, C. R., Shi, M. M., Cantor, C. R., and Braun, A. (2004). Large-scale validation of single nucleotide polymorphisms in gene regions. Genome Res. 14, 1664–1668.
Ng, S. C., Benjamin, J. L., McCarthy, N. E., Hedin, C. R., Koutsoumpas, A., Plamondon, S., Price, C. L., Hart, A. L., Kamm, M. A., Forbes, A., Knight, S. C., Lindsay, J. O., Whelan, K., and Stagg, A. J. (2010). Relationship between human intestinal dendritic cells, gut microbiota, and disease activity in Crohn’s disease. Inflamm. Bowel Dis. doi: 10.1002/ibd.21590. [Epub ahead of print].
Nicholls, A. W., Mortishire-Smith, R. J., and Nicholson, J. K. (2003). NMR spectroscopic-based metabonomic studies of urinary metabolite variation in acclimatizing germ-free rats. Chem. Res. Toxicol. 16, 1395–1404.
Nicholson, J. K., Holmes, E., and Wilson, I. D. (2005). Gut microorganisms, mammalian metabolism and personalized health care. Nat. Rev. Microbiol. 3, 431–438.
Nicholson, J. K., and Wilson, I. D. (2003). Opinion: understanding “global” systems biology: metabonomics and the continuum of metabolism. Nat. Rev. Drug Discov. 2, 668–676.
Ninonuevo, M. R., Youmie, P., Hongfeng, Y., Jinhua, Z., Ward, R. E., Clowers, B. H., German, J. B., Freeman, S. L., Killeen, K., Grimm, R., and Lebrilla, C. B. (2006). A strategy for annotating the human milk glycome. J. Agric. Food Chem. 54, 7471–7480.
Nurnberger, J., Dammer, S., Mitchell, A., Siffert, W., Wenzel, R. R., Gossl, M., Philipp, T., Michel, M. C., and Schäfers, R. F. (2003). Effect of the C825T polymorphism of the G protein beta 3 subunit on the systolic blood pressure-lowering effect of clonidine in young, healthy male subjects. Clin. Pharmacol. Ther. 74, 53–60.
Painter, R. C., Roseboom, T. J., and Bleker, O. P. (2005). Prenatal exposure to the Dutch famine and disease in later life: an overview. Reprod. Toxicol. 20, 345–352.
Panchaud, A., Affolter, M., Moreillon, P., and Kussmann, M. (2008). Experimental and computational approaches to quantitative proteomics: status quo and outlook. J. Proteomics 71, 19–33.
Park, J. H., Jeong, H. J., and de Lumen, B. O. (2005) Contents and bioactivities of lunasin, bowman-birk inhibitor, and isoflavones in soybean seed. J. Agric. Food Chem. 53, 7686–7690.
Payton, A., Gibbons, L., Davidson, Y., Ollier, W., Rabbitt, P., Worthington, J., Pickles, A., Pendleton, N., and Horan, M. (2005). Influence of serotonin transporter gene polymorphisms on cognitive decline and cognitive abilities in a nondemented elderly population. Mol. Psychiatry 10, 1133–1139.
Pruteanu, M., Hyland, N. P., Clarke, D. J., Kiely, B., and Shanahan, F. (2011). Degradation of the extracellular matrix components by bacterial-derived metalloproteases: implications for inflammatory bowel diseases. Inflamm. Bowel Dis. 17, 1189–1200.
Rabot, S., Membrez, M., Bruneau, A., Gerard, P., Harach, T., Moser, M., Raymond, F., Mansourian, R., and Chou, C. J. (2010). Germ-free C57BL/6J mice are resistant to high-fat-diet-induced insulin resistance and have altered cholesterol metabolism. FASEB J. 24, 4948–4959.
Robinson, S. M., Crozier, S. R., Borland, S. E., Hammond, J., Barker, D. J., and Inskip, H. M. (2004). Impact of educational attainment on the quality of young women’s diets. Eur. J. Clin. Nutr. 58, 1174–1180.
Sakata, K., Ohyanagi, H., Nobori, H., Nakamura, T., Hashiguchi, A., Nanjo, Y., Mikami, Y., Yunokawa, H., and Komatsu, S. (2009). Soybean proteome database: a data resource for plant differential omics. J. Proteome Res. 8, 3539–3548.
Sanchez, L., Calvo, M., and Brock, J. H. (1992). Biological role of lactoferrin. Arch. Dis. Child. 67, 657–661.
Savage, D. C. (1977). Microbial ecology of the gastrointestinal tract. Annu. Rev. Microbiol. 31, 107–133.
Savage, D. C. (1986). Gastrointestinal microflora in mammalian nutrition. Annu. Rev. Nutr. 6, 155–178.
Schack-Nielsen, L., and Michaelsen, K. F. (2007). Advances in our understanding of the biology of human milk and its effects on the offspring. J. Nutr. 137, 503S–510S.
Schaefer-Graf, U. M., Pawliczak, J., Passow, D., Hartmann, R., Rossi, R., Buhrer, C., Harder, T., Plagemann, A., Vetter, K., and Kordonouri, O. (2005). Birth weight and parental BMI predict overweight in children from mothers with gestational diabetes. Diabetes Care 28, 1745–1750.
Schlimme, E., and Meisel, H. (1995). Bioactive peptides derived from milk proteins. Structural, physiological and analytical aspects. Nahrung 39, 1–20.
Severin, S., and Xia, W. (2005). Milk biologically active components as nutraceuticals: review. Crit. Rev. Food Sci. Nutr. 45, 645–656.
Siffert, W. (2005). G protein polymorphisms in hypertension, atherosclerosis, and diabetes. Annu. Rev. Med. 56, 17–28.
Sinclair, K. D., Allegrucci, C., Singh, R., Gardner, D. S., Sebastian, S., Bispham, J., Thurston, A., Huntley, J. F., Rees, W. D., Maloney, C. A., Lea, R. G., Craigon, J., McEvoy, T. G., and Young, L. E. (2007). DNA methylation, insulin resistance, and blood pressure in offspring determined by maternal periconceptional B vitamin and methionine status. Proc. Natl. Acad. Sci. U.S.A. 104, 19351–19356.
Slotkin, R. K., and Martienssen, R. (2007). Transposable elements and the epigenetic regulation of the genome. Nat. Rev. Genet. 8, 272–285.
Smith, E. D., Tsuchiya, M., Fox, L. A., Dang, N., Hu, D., Kerr, E. O., Johnston, E. D., Tchao, B. N., Pak, D. N., Welton, K. L., Promislow, D. E., Thomas, J. H., Kaeberlein, M., and Kennedy, B. K. (2008). Quantitative evidence for conserved longevity pathways between divergent eukaryotic species. Genome Res. 18, 564–570.
Sonnenburg, J. L., Chen, C. T., and Gordon, J. I. (2006). Genomic and metabolic studies of the impact of probiotics on a model gut symbiont and host. PLoS Biol. 4, e413. doi:10.1371/journal.pbio.0040413
Sonnenburg, J. L., Xu, J., Leip, D. D., Chen, C. H., Westover, B. P., Weatherford, J., Buhler, J. D., and Gordon, J. I. (2005). Glycan foraging in vivo by an intestine-adapted bacterial symbiont. Science 307, 1955–1959.
Talbert, P. B., and Henikoff, S. (2006). Spreading of silent chromatin: inaction at a distance. Nat. Rev. Genet. 7, 793–803.
Turnbaugh, P. J., and Gordon, J. I. (2009). The core gut microbiome, energy balance and obesity. J. Physiol. 587, 4153–4158.
Turnbaugh, P. J., Hamady, M., Yatsunenko, T., Cantarel, B. L., Duncan, A., Ley, R. E., Sogin, M. L., Jones, W. J., Roe, B. A., Affourtit, J. P., Egholm, M., Henrissat, B., Heath, A. C., Knight, R., and Gordon, J. I. (2009). A core gut microbiome in obese and lean twins. Nature 457, 480–484.
Turnbaugh, P. J., Ley, R. E., Mahowald, M. A., Magrini, V., Mardis, E. R., and Gordon, J. I. (2006). An obesity-associated gut microbiome with increased capacity for energy harvest. Nature 444, 1027–1031.
VerBerkmoes, N. C., Russell, A. L., Shah, M., Godzik, A., Rosenquist, M., Halfvarson, J., Lefsrud, M. G., Apajalahti, J., Tysk, C., Hettich, R. L., and Jansson, J. K. (2009). Shotgun metaproteomics of the human distal gut microbiota. ISME J. 3, 179–189.
Vickers, M. H., Breier, B. H., Cutfield, W. S., Hofman, P. L., and Gluckman, P. D. (2000). Fetal origins of hyperphagia, obesity, and hypertension and postnatal amplification by hypercaloric nutrition. Am. J. Physiol. Endocrinol. Metab. 279, E83–E87.
Vickers, M. H., Gluckman, P. D., Coveny, A. H., Hofman, P. L., Cutfield, W. S., Gertler, A., Breier, B. H., and Harris, M. (2005). Neonatal leptin treatment reverses developmental programming. Endocrinology 146, 4211–4216.
Vidal, K., Labeta, M. O., Schiffrin, E. J., and Donnet-Hughes, A. (2001). Soluble CD14 in human breast milk and its role in innate immune responses. Acta Odontol. Scand. 59, 330–334.
Walker, W. A., Goulet, O., Morelli, M., and Antoine, J. M. (2006). Progress in the science of probiotics: from cellular microbiology and applied immunology to clinical nutrition. Eur. J. Nutr. 45, 1–18.
Waterland, R. A., Lin, J. R., Smith, C. A., and Jirtle, R. L. (2006). Post-weaning diet affects genomic imprinting at the insulin-like growth factor 2 (Igf2) locus. Hum. Mol. Genet. 15, 705–716.
Wenzel, R. R., Siffert, W., Bruck, H., Philipp, T., and Schafers, R. F. (2002). Enhanced vasoconstriction to endothelin-1, angiotensin II and noradrenaline in carriers of the GNB3 825T allele in the skin microcirculation. Pharmacogenetics 12, 489–495.
Xu, J., and Gordon, J. I. (2003). Inaugural article: honor thy symbionts. Proc. Natl. Acad. Sci. U.S.A. 100, 10452–10459.
Yajnik, C. S. (2004). Early life origins of insulin resistance and type 2 diabetes in India and other Asian countries. J. Nutr. 134, 205–210.
Yajnik, C. S., Deshpande, S. S., Jackson, A. A., Refsum, H., Rao, S., Fisher, D. J., Bhat, D. S., Naik, S. S., Coyaji, K. J., Joglekar, C. V., Joshi, N., Lubree, H. G., Deshpande, V. U., Rege, S. S., and Fall, C. H. (2008). Vitamin B12 and folate concentrations during pregnancy and insulin resistance in the offspring: the Pune maternal nutrition study. Diabetologia 51, 29–38.
Zhao, X. J., Li, C., Paez, J. G., Chin, K., Janne, P. A., Chen, T. H., Girard, L., Minna, J., Christiani, D., Leo, C., Gray, J. W., Sellers, W. R., and Meyerson, M. (2004). An integrated view of copy number and allelic alterations in the cancer genome using single nucleotide polymorphism arrays. Cancer Res. 64, 3060–3071.
Keywords: nutrigenomics, nutrigenetics, epigenetics, personalized nutrition, biomarker, bioactive, gut microbiota
Citation: Kussmann M and Van Bladeren PJ (2011) The extended nutrigenomics – understanding the interplay between the genomes of food, gut microbes, and human host. Front. Gene. 2:21. doi: 10.3389/fgene.2011.00021
Received: 21 March 2011;
Accepted: 29 April 2011;
Published online: 20 May 2011.
Edited by:
Patrick John Stover, Cornell University, USAReviewed by:
Sander Kersten, Wageningen University, NetherlandsAmanda J. Macfarlane, Health Canada, Canada
Xu Lin, Chinese Academy of Sciences, China
Copyright: © 2011 Kussmann and Bladeren. This is an open-access article subject to a non-exclusive license between the authors and Frontiers Media SA, which permits use, distribution and reproduction in other forums, provided the original authors and source are credited and other Frontiers conditions are complied with.
*Correspondence: Martin Kussmann, Nestlé Institute of Health Sciences, Avenue Nestlé 55, 1800 Vevey, Lausanne, Switzerland. e-mail: martin.kussmann@nestle.com