- Department of Health Technology and Informatics, The Hong Kong Polytechnic University, Hong Kong, China
MicroRNAs are a class of non-coding RNAs and the dysregulated expression of these short RNA molecules was frequently observed in cancer cells. The steady state level of microRNA concentration may differentiate the biological function of the cells between normal and impaired. To understand the steady state or equilibrium of microRNAs, their interactions with transcription factors and target genes need to be explored and visualized through prediction and network analysis algorithms. This article discusses the application of mathematical model for simulating the dynamics of network feedback loop so as to decipher the mechanism of microRNA regulation.
Regulatory Functions of microRNAs
MicroRNAs (miRNAs) constitute a set of non-coding RNAs that are short in length, around 21–25 nucleotides, and endogenous. Protein expression is repressed or the coding message is degraded when miRNAs are bound to the 3′-untranslated regions (UTRs) of the target messenger RNAs (mRNAs) with imperfect complementarity (Baek et al., 2008). In mammals, over 800 miRNAs have been identified and those are capable to simultaneously regulate hundreds to thousands of genes and thus their biological networks (Wu et al., 2009). It was reported that miR-7 downregulates significantly the EGFR expression in various malignancies, including glioblastoma, breast cancer, prostate cancer, and lung cancer (Webster et al., 2009). This finding was further confirmed by the miR-7 target prediction using TargetScan, an in silico analysis pipeline. Through the prediction, three putative target sites at the 3′-UTR of EGFR transcript were identified and two of them ranked very high due to the fact that they are proximal to AU-rich sequences. Indeed, diminished EGFR expression leads to the cell cycle arrest and the cell growth reduction. It was also shown that multiple associated members of EGFR downstream signaling pathway were regulated by miR-7 in cancer cell lines, including protein kinase B and extracellular signal-regulated kinase (ERK) 1/2 (Webster et al., 2009).
A miRNA could regulate the expression of hundreds of genes. The proteins expressed from these genes targeted by a particular miRNA tend to be hubs and bottlenecks in the protein interactions network (Hsu et al., 2008). The biological functions of this specific subset of proteins are thus altered by an individual miRNA. Conversely, a gene could be a common target of many miRNAs. A systematic search performed at 10 representative resources, including prediction pipelines and curated databases as listed in Table 1, revealed that 138 miRNAs potentially target EGFR transcript according to various identification criteria. In this set of potential miRNAs, 25 of them were predicted by at least 3 prediction pipelines and confirmed by at least 1 curated database (Chan et al., 2011). This observation implicated a comprehensive post-transcriptional regulatory network mediated by miRNAs but the underlying mechanism and conditions of the gene regulation by miRNAs is still unclear.
Transcriptional Regulation of miRNA Expression
Dysregulation of miRNA expression have long been hypothesized as the pathogenesis of cancers. The stimulation of miR-7 expression in an ERK suggested that miR-7 expression is promoted by EGFR through the Ras/ERK/Myc signaling cascade, which is found in the non-small cell lung cancer (NSCLC) pathway (Chou et al., 2010). Most of the miRNAs are transcribed in independent units, which are distant from the annotated genes (Bartel, 2004). Such miRNAs have their own promoter regions where the transcription factors (TFs) regulate their expression via TF binding sites (TFBS) within the promoter region of miRNA genes. Some of the miRNAs are processed from the introns of the protein coding genes. The expression of this class of miRNAs is regulated by the TFs via TFBS at the promoter region of the host gene of the miRNAs. Public database has been developed for modeling the interactions between TFs and TFBSs (Matys et al., 2003). TFBSs are usually organized in DNA footprints, called cis-regulatory modules (CRMs). CRMs are featured by the evolutionary conservation of the TFBS sequences and the co-expression of the corresponding TFs (Blanchette et al., 2006). A TF is predicted when the corresponding TFBS(s) are found within the upstream 5 kbp of a miRNA gene or within the promoter region of the host gene (Yu et al., 2008). Such predictions implicate higher complexity and variability in the regulatory network topology as miRNAs are not only regulators of protein expression but also regulated by proteins. Feedback loops (FBLs) become frequently observed patterns in the gene regulatory networks.
Genome-Wide miRNA Regulation Analysis
Biological processes are typically regulated by the dynamic interactions of biomolecules and genetic materials, including genes, TFs, and miRNAs, between or within the living cells, due to the cell cycle or the external stress. Regulatory networks provide framework for understanding the cascade of these molecular interactions and exploring the biologically relevant motifs. The alteration of these molecular interaction networks leads to localized or systemic malignancy in living organisms, especially human sapiens. Many computational algorithms and databases have been developed for generating and analyzing comprehensive models of the regulatory interactions at genome-wide scale (Su et al., 2010; Béchec et al., 2011). In those resources, miRNAs are regarded as a class of key actors interplaying with the other key actors, such as genes and TFs. Network motifs with the enrichment of gene ontology (GO) could give insight into the underlying biological functions performed by the “teamwork” of these actors.
Feedback Loop for miRNA Homeostasis
Many miRNAs are related to cancer pathogenesis and thus regarded as “onco-miRs.” The over-expressions of such miRNAs were frequently observed in cancer cell lines. Conversely, there exist cancer suppressor miRNAs that post-transcriptionally repress the expression of oncogenes and their under-expressions were also found in cancer cells. Therefore, the fluctuation in miRNA expression level does not favor the normal biological processes. It is still unclear how the steady state of miRNA expression is achieved.
The expression of miR-591 was found in colorectal tumor cells but not in the normal cells through miRNA serial analysis of gene expression (miRAGE; Cummins et al., 2006). It is meaningful to decipher the mechanism, under which the miRNAs expression is maintained at a low level in the normal cells. MiRNA and TF analysis network (MIR@NT@N) was used to predict the TFs and gene targets of miR-591 and to construct the regulatory network among them (Béchec et al., 2011). Nine TFs were found with full score. More interestingly, the algorithm predicts the gene expressions of two of these TFs are repressed by miR-591. The regulatory network of miR-591 is shown in Figure 1. MZF1 and FOXA1 are two TFs, which regulate miR-591, but their gene expressions are also repressed by miR-591. These reciprocal interactions, highlighted in the right-top box of Figure 1, are referred to as FBLs of biological systems. The balance between miR-591, MZF1, and FOXA1 can be automatically attained through the FBLs. Further, MZF1 is also the TF of the other TFs of miR-591 except FOXL1. Thus, the retarded expression of MZF1 will also suppress the transcription of miR-591 indirectly. Such direct and indirect FBLs may explain why and how onco-miR expression level is low in normal cells.
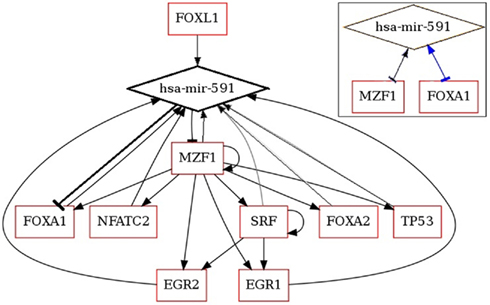
Figure 1. Regulatory network of miR-591 generated by MIR@NT@N (Béchec et al., 2011).
Mathematical modeling could be applied to elucidate the reciprocal regulatory responses of the actors in the FBLs. The state-space model was used to simulate the concentrations and their rates of a panel of TFs (Yu et al., 2008). As miRNAs are also key actors of the regulation, the TFs and miRNAs could constitute the state vector of the model so to understand the homeostasis of miRNA expression in normal cells and the effect of perturbed miRNA expression in cancer cells with the future research endeavors.
Conclusion
Based on the identified feedback mechanism, differential equation system can be established to model biological programs contributed by miRNAs. The response of miRNA expression due to the dysregulation of interacting genes can be simulated using the model, providing useful information for therapeutic planning.
Conflict of Interest Statement
The author declares that the research was conducted in the absence of any commercial or financial relationships that could be construed as a potential conflict of interest.
References
Baek, D., Villén, J., Shin, C., Camargo, F. D., Gygi, S. P., and Bartel, D. P. (2008). The impact of microRNAs on protein output. Nature 455, 64–71.
Béchec, A. L., Portales-Casamar, E., Vetter, G., Moes, M., Zindy, P.-J., Saumet, A., Arenillas, D., Theillet, C., Wasserman, W. W., Lecellier, C.-H., and Friederich, E. (2011). MIR@NT@N: a framework integrating transcription factors, microRNAs and their targets to identify sub-network motifs in a meta-regulation network model. BMC Bioinformatics 12, 67. doi:10.1186/1471-2105-12-67
Blanchette, M., Bataille, A. R., Chen, X., Poitras, C., Laganière, J., Lefèbvre, C., Deblois, G., Giguère, V., Ferretti, V., Bergeron, D., Coulombe, B., and Robert, F. (2006). Genome-wide computational prediction of transcriptional regulatory modules reveals new insights into human gene expression. Genome Res. 16, 656–668.
Chan, L. W. C., Wang, F. F., and Cho, W. C. S. (2011). Genomic sequence analysis of EGFR regulation by microRNAs in lung cancer. [Manuscript submitted to Current Topics in Medicinal Chemistry for review].
Chou, Y.-T., Lin, H.-H., Lien, Y.-C., Wang, Y.-H., Hong, C.-F., Kao, Y.-R., Lin, S.-C., Chang, Y.-C., Lin, S.-Y., Chen, S.-J., Chen, H.-C., Yeh, S.-D., and Wu, C.-W. (2010). EGFR promotes lung tumorigenesis by activating miR-7 through a Ras/ERK/Myc pathway that targets the Ets2 transcriptional repressor ERF. Cancer Res. 70, 8822–8831.
Cummins, J. M., He, Y., Leary, R. J., Pagliarini, R., Diaz, L. A., Sjoblom, T., Barad, O., Bentwich, Z., Szafranska, A. E., Labourier, E., Raymond, C. K., Roberts, B. S., Juhl, H., Kinzler, K. W., Vogelstein, B., and Velculescu, V. E. (2006). The colorectal microRNAome. Proc. Natl. Acad. Sci. U.S.A. 103, 3687–3692.
Hsu, C.-W., Juan, H.-F., and Huang, H.-C. (2008). Characterization of microRNA-regulated protein-protein interaction network. Proteomics 8, 1975–1979.
Matys, V., Fricke, E., Geffers, R., Göß ling, E., Haubrock, M., Hehl, R., Hornischer, K., Karas, D., Kel, A. E., Kel-Margoulis, O. V., Kloos, D.-U., Land, S., Lewicki-Potapov, B., Michael, H., Münch, R., Reuter, I., Rotert, S., Saxel, H., Scheer, M., Thiele, S., and Wingender, E. (2003). TRANSFAC: transcriptional regulation, from patterns to profiles. Nucleic Acids Res. 31, 374–378.
Su, N., Wang, Y., Qian, M., and Deng, M. (2010). Combinatorial regulation of transcription factors and microRNAs. BMC Syst. Biol. 4, 150. doi:10.1186/1752-0509-4-150
Webster, R. J., Giles, K. M., Price, K. J., Zhang, P. M., Mattick, J. S., and Leedman, P. J. (2009). Regulation of epidermal growth factor receptor signaling in human cancer cells by microRNA-7. J. Biol. Chem. 284, 5731–5741.
Wu, X., Piper-Hunter, M. G., Crawford, M., Nuovo, G. J., Marsh, C. B., Otterson, G. A., and Nana-Sinkam, S. P. (2009). MicroRNAs in the pathogenesis of lung cancer. J. Thorac. Oncol. 4, 1028–1034.
Keywords: microRNA, feedback, regulation, equilibrium
Citation: Chan LWC (2011) Modeling equilibrium of microRNA expression. Front. Gene. 2:35. doi: 10.3389/fgene.2011.00035
Received: 27 March 2011;
Paper pending published: 12 April 2011;
Accepted: 04 June 2011;
Published online: 16 June 2011.
Edited by:
Michael Rossbach, Genome Institute of Singapore, SingaporeReviewed by:
Michael Rossbach, Genome Institute of Singapore, SingaporePanagiotis Alexiou, Alexander Fleming Biomedical Sciences Research Center, Greece
Amelia Cimmino, Consiglio Nazionale delle Ricerche, Italy
Copyright: © 2011 Chan. This is an open-access article subject to a non-exclusive license between the authors and Frontiers Media SA, which permits use, distribution and reproduction in other forums, provided the original authors and source are credited and other Frontiers conditions are complied with.
*Correspondence: Lawrence W. C. Chan, Department of Health Technology and Informatics, The Hong Kong Polytechnic University, Room Y934, 9/F, Y Block, Hung Hom, Kowloon, Hong Kong, China. e-mail: wing.chi.chan@inet.polyu.edu.hk