Functional differences between global pre- and postsynaptic inhibition in the Drosophila olfactory circuit
- 1 RIKEN Brain Science Institute, Wako, Saitama, Japan
- 2 Department of Complexity Science and Engineering, The University of Tokyo, Kashiwa, Chiba, Japan
The Drosophila antennal lobe is subdivided into multiple glomeruli, each of which represents a unique olfactory information processing channel. In each glomerulus, feedforward input from olfactory receptor neurons (ORNs) is transformed into activity of projection neurons (PNs), which represent the output. Recent investigations have indicated that lateral presynaptic inhibitory input from other glomeruli controls the gain of this transformation. Here, we address why this gain control acts “pre”-synaptically rather than “post”-synaptically. Postsynaptic inhibition could work similarly to presynaptic inhibition with regard to regulating the firing rates of PNs depending on the stimulus intensity. We investigate the differences between pre- and postsynaptic gain control in terms of odor discriminability by simulating a network model of the Drosophila antennal lobe with experimental data. We first demonstrate that only presynaptic inhibition can reproduce the type of gain control observed in experiments. We next show that presynaptic inhibition decorrelates PN responses whereas postsynaptic inhibition does not. Due to this effect, presynaptic gain control enhances the accuracy of odor discrimination by a linear decoder while its postsynaptic counterpart only diminishes it. Our results provide the reason gain control operates “pre”-synaptically but not “post”-synaptically in the Drosophila antennal lobe.
Introduction
Nervous systems need to correctly interpret sensory stimuli robustly across a wide variety of intensities. One strategy to accomplish this robust interpretation is gain control. Gain control properly regulates the response magnitude of sensory neurons in accordance with the stimulus intensity. Gain control is in action in a wide variety of modalities. However, the biophysical mechanisms of even the most intensively studied form of gain control in the primate primary visual cortex, is still under debate (Carandini and Heeger, 2011).
Detailed cellular and synaptic mechanisms of such a gain control in vivo were recently reported in the Drosophila antennal lobe, an analog of the vertebrate olfactory bulb (Olsen and Wilson, 2008; Root et al., 2008; Olsen et al., 2010). The fruit fly Drosophila is increasingly recognized as an excellent model animal to study the neural basis of sensory processing in general. Olfactory processing is particularly attractive given the detailed wiring diagram of the circuit as well as genetic and physiological accessibility to identified neurons forming the circuit. The antennal lobe is divided into discrete neuropil structures called glomeruli, each of which represents a unique information processing unit (Stocker et al., 1990). Olfactory receptor neurons (ORNs) expressing the same olfactory receptors converge to a single glomerulus where they synapse onto the projection neurons (PNs) (Figure 1; Gao et al., 2000; Vosshall et al., 2000). Feedforward ORN input is non-linearly transformed into PN output in a glomerulus (Wilson et al., 2004; Bhandawat et al., 2007; Kazama and Wilson, 2008). Independent studies have shown that local neurons (LNs) interconnecting glomeruli mediate lateral inhibition and control the gain of this intraglomerular transformation (Olsen and Wilson, 2008; Root et al., 2008). The strength of lateral inhibition roughly scales with total feedforward input to the antennal lobe (Olsen and Wilson, 2008). This global inhibition appropriately scales PN responses in accordance with the stimulus intensity and is considered to enable PNs to efficiently encode olfactory information across a wide range of odorant concentrations within their limited dynamic rage.
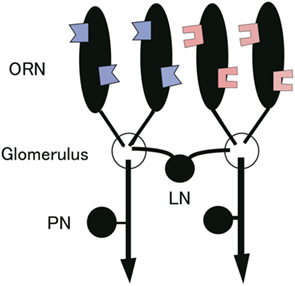
Figure 1. Schematic of the Drosophila antennal lobe. ORNs expressing the same odorant receptor converge to the same glomerulus and connect to PNs. PNs send information to higher order brain regions. LNs interconnecting glomeruli mediate lateral inhibition.
Interestingly, in the Drosophila olfactory system, the gain control mechanism acts at a presynaptic locus of ORN-PN synapses (Olsen and Wilson, 2008; Root et al., 2008). This experimental observation is the main concern of this paper. Postsynaptic inhibition can equally control the gain in terms of regulating the overall firing rate of PNs in accordance with the stimulus intensity. If this is so, why does the gain control act at a presynaptic locus in the antennal lobe? The physiological difference between pre- and postsynaptic inhibition is that presynaptic inhibition indirectly inhibits the activity of PNs by regulating the release probability of the ORN-PN synapses while postsynaptic inhibition directly inhibits the activity of PNs by hyperpolarizing the membrane potential of PNs. We hypothesized that there should be some functional reasons global inhibition acts presynaptically but not postsynaptically and that these reasons are related to a fundamental task of an olfactory system, i.e., discriminating between different odors across a wide range of concentrations.
To test this hypothesis, we investigated how different gain control mechanisms contribute to the discriminability of PN odor responses by simulating a network model of the Drosophila antennal lobe with experimental data. To clarify the functional difference between pre- and postsynaptic inhibitory gain control mechanisms, we constructed two types of model networks. One emulates the actual olfactory circuit where global inhibition acts presynaptically. The other is a hypothetical circuit model where global inhibition acts postsynaptically. By comparing the two network models, we addressed whether qualitative differences exist between pre- and postsynaptic gain control in terms of odor discriminability.
We first showed that presynaptic inhibition horizontally scales the input-output (I-O) relationship between ORNs and PNs as has been observed in experiments (Olsen and Wilson, 2008; Olsen et al., 2010). On the other hand, postsynaptic inhibition rather vertically scales the I-O relationship and does not emulate the experimentally observed scaling. These results confirmed that presynaptic inhibition is the actual mechanism mediating the gain control in the Drosophila antennal lobe. We next showed that presynaptic global inhibition sharpens the odor tuning of PNs whereas postsynaptic global inhibition does not. This means that presynaptic inhibition can reduce the similarity of mean PN responses to various odors, which would be beneficial for odor discrimination. By applying a classification technique developed in machine learning, we further demonstrated that presynaptic inhibition actually enhances the accuracy of odor discrimination whereas postsynaptic inhibition only diminishes it. Our results provide a reason presynaptic but not postsynaptic gain control is used in the Drosophila antennal lobe.
Materials and Methods
Model of Drosophila Olfactory Circuit
Here we provide an overview of the Drosophila antennal lobe circuit that we investigate in this study (Figure 1). The antennal lobe consists of characteristic compartmental structures termed glomeruli (Stocker et al., 1990; Laissue et al., 1999). ORNs expressing the same odorant receptor converge to the same glomerulus (Gao et al., 2000; Vosshall et al., 2000) and connect to PNs. The dendritic arbors of individual PNs are confined within a single glomerulus (Jefferis et al., 2001; Marin et al., 2002; Wong et al., 2002). The glomeruli therefore constitute discrete processing channels. The interaction between these discrete channels is mediated by LNs. The transformation of neural responses from ORNs to PNs is determined by the sum of intraglomerular processing at ORN-PN synapses and interglomerular interaction through LNs. Although both excitatory and inhibitory LNs exist in the antennal lobe (Stocker et al., 1990; Ng et al., 2002; Wilson and Laurent, 2005; Olsen et al., 2007; Root et al., 2007, 2008; Shang et al., 2007; Das et al., 2008; Lai et al., 2008; Okada et al., 2009; Chou et al., 2010; Huang et al., 2010; Yaksi and Wilson, 2010), we only consider the LNs providing global, non-specific inhibition across all glomeruli because these are the mediators of gain control (Olsen and Wilson, 2008; Root et al., 2008), which is the main focus of this study. Moreover, lateral input from LNs is overall inhibitory (Olsen and Wilson, 2008; Asahina et al., 2009). Also, there has been so far no evidence supporting the contribution of excitatory LNs to gain control. Moreover, lateral input from LNs is overall inhibitory (Olsen and Wilson, 2008; Asahina et al., 2009). From these results, we restricted our analysis to inhibitory LNs.
Model of ORNs
First, we describe the model of ORNs. We assumed that ORNs fire in accordance with the Poisson process with time-independent rates that depend on types of odors. When the firing rate of an ORN is f, the probability that the ORN fires within infinitesimal time interval Δt is given by fΔt. Note that the stochastic firing of ORNs according to the Poisson statistics is the only source of noise in the network because we do not assume any other noise sources. To determine the ORN rates to various odors, we used Hallem and Carlson’s (2006) data that measured responses of 24 types of ORNs to more than 100 odors. In our network model, we considered these 24 types of ORNs. Because approximately only 50 glomeruli are in Drosophila (Laissue et al., 1999), our model takes into account about half the glomeruli engaged in olfactory processing.
We used two sets of data reported in Hallem and Carlson (2006) for simulations (Figures 2A,B). The first (data 1) contains the ORN responses to 110 different odors (Figure 2A). The second (data 2) contains the ORN responses to 19 different odors at three different concentrations (Figure 2B).
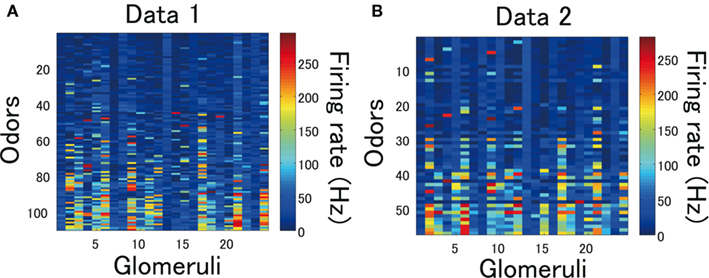
Figure 2. ORN data from Hallem and Carlson (2006). (A) 24 Types of ORN responses to 110 different odors. (B) 24 Types of ORN responses to 19 different odors at three different concentrations.
Model of PNs
Second, we describe the model of PNs. A PN is modeled as the standard leaky integrate-and-fire neuron. The membrane potential of a PN at time t is determined by the following differential equation,

where τm is the membrane time constant and V0 is the resting potential. When the membrane potential of a PN exceeds the threshold value Vth, the PN emits a spike and the value of the membrane potential is reset to Vreset. After the spike emission, the PN enters an absolute refractory period that lasts for tr. The membrane potential of a PN stays at Vreset during the refractory period. The parameters in the leaky integrate-and-fire neuron model are set as follows: τm= 5 ms, V0 = −60 mV, Vth= − 45 mV, Vreset= − 80 mV, and tr= 1 ms. These parameter values do not qualitatively affect the results of this study.
IpostLN is the postsynaptic input from LNs, and the details will be described in the next section. IORN is the sum of the input from ORNs within a glomerulus and is given by

where gi is the synaptic conductance between the ith ORN and a PN within a glomerulus, Vi is the membrane potential of the ith ORN and VE is the reversal potential. In accordance with the experimental findings (Kazama and Wilson, 2008, 2009), we assumed that all the ORNs are connected to all the PNs within a glomerulus. We set the number of ORNs and PNs in each glomerulus to 30 and 1, respectively. The actual numbers are reported to be about 40 and 3, respectively (Stocker, 1994; de Bruyne et al., 1999, 2001; Vosshall et al., 1999). For the sake of computational costs, we slightly reduced the numbers of ORNs and PNs. The summation in equation (2) runs through all ORNs that belong to the same glomerulus. When the ith ORN spikes, the conductance gi(t) increases as follows,

where Ni(t) is the number of releasable vesicles at the ORN axon, p is the probability of vesicular release and q is the quantal size. We introduced Ni(t) and p to model short-term synaptic depression at ORN-PN synapses (Kazama and Wilson, 2008). Although the actual vesicular release is stochastic, we assumed for simplicity that the ratio of released vesicles is constant, p. The synaptic conductance in the absence of ORN spikes decays exponentially with a synaptic decay time τORN,

Because the number of releasable vesicles decreases by Ni(t)p every time an ORN fires,

vesicles are depleted when the firing rate of an ORN is high. This depletion of releasable vesicles causes synaptic depression. The recovery process is modeled by the exponential relaxation,

where τN is the recovery time constant and N0 is the maximal number of releasable vesicles. This is the simplest known model of short-term depression (Liley and North, 1952; Betz, 1970; Zucker and Regehr, 2002).
The parameters N0, q, τORN, τN determine the strength of transmission at ORN-PN synapses. The parameters are chosen so that the PN firing rates are saturated at about 200 Hz (Figure 4) as can be seen in the actual data (Bhandawat et al., 2007; Olsen and Wilson, 2008). The parameter values are N0 = 51, q = 1.07, τORN= 2 ms, τN= 100 ms.
Model of Global Pre- and Postsynaptic Inhibition
Recent experiments showed that lateral input from LNs to PNs is mainly inhibitory and this scales with total feedforward input from ORNs (Olsen and Wilson, 2008; Olsen et al., 2010). These experimental result suggested that the odor tuning of inhibitory input to each glomerulus is approximately similar. This global inhibitory input across all glomeruli is considered to mediate gain control of olfactory processing. In what follows, we describe how to model such a gain control mechanism.
Importantly, this global inhibition mechanism acts at a presynaptic locus (Olsen and Wilson, 2008; Root et al., 2008). Thus, LNs indirectly inhibit PNs by reducing the release probability at the ORN terminal. We model the decrease in the release probability in accordance with total ORN activity as

where pmax is the release probability without inhibition, Kpre is the parameter that determines the strength of presynaptic inhibition, and ftot(s) is the total ORN activity evoked by odor s. ftot(s) is described as,

where fk(s) is the firing rate of ORNs belonging to the kth glomerulus when odor s is presented. pmax determines the degree of synaptic depression. We set pmax to 0.79 by referring to experimentally observed values (Kazama and Wilson, 2008).
To compare it with presynaptic gain control, we also considered the case of global postsynaptic inhibition, in which LNs directly inhibit PNs by hyperpolarizing the membrane potential of PNs. Postsynaptic inhibitory input is modeled as IpostLN(s) in equation (1),

where Kpost is a parameter that determines the strength of postsynaptic inhibition and ftot(s) is given by equation (8). When we consider the postsynaptic gain control model, the release probability is fixed at p = pmax.
It is known that the degree of inhibition approximately linearly scales with the total ORN activity (Olsen and Wilson, 2008). To properly compare pre- and postsynaptic inhibition, we emulated this experimental observation in both pre- and postsynaptic inhibition models by arbitrarily determining the functional forms of pre- and postsynaptic gain control in equations (7) and (9). Figures 3A,B show how much PN responses are inhibited by pre- and postsynaptic inhibition. Pre- and postsynaptic gain control similarly work in terms of regulating the dynamic range of PN rate as we intended. The major results of this study do not depend on the particular forms of pre- and postsynaptic gain control in equations (7) and (9) (data not shown). As will be discussed in detail in the Results section, what matters is whether pre- or postsynaptic inhibition decorrelate PN responses or not.
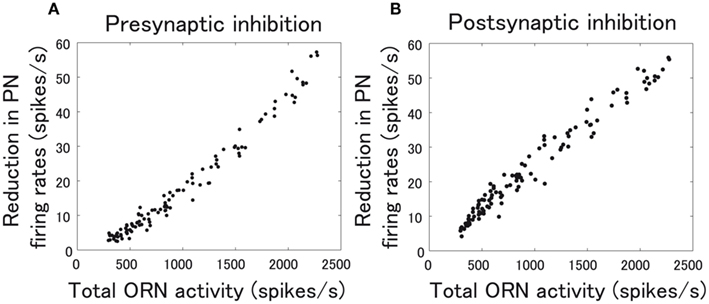
Figure 3. Relationship between total ORN activity and reduction in PN firing rates. ORN responses to 110 odors in data 1 (Figure 2A) were fed to our model network. The reduction in firing rates is averaged over the all types of PNs. Each point corresponds to a different odor. (A) The case of presynaptic inhibition. The strength of presynaptic inhibition, Kpre in equation (7), is set to Kpre= 0.35. (B) The case of postsynaptic inhibition. The strength of postsynaptic inhibition, Kpost in equation (9), is set to Kpost= 3.0.
Evaluation of Odor Discriminability
We evaluated the odor discriminability of PN responses as follows. We ran simulations of the olfactory network using the data of ORN responses (Figure 2A or B) as input. This yielded simulated PN responses to various odors. By using half the simulated PN responses, we trained support vector machine (SVM) classifiers. Subsequently, we tested the performance of SVM classifiers by using the other half of the simulated data. The number of simulation trials was 100, and the simulation time was set to 10 ms. A library for support vector machines (LIBSVM) was used to implement the SVM classifiers (Chang and Lin, 2001).
The task of SVM classifiers is to determine correctly which odor is presented when PN responses are given. We used the one-against-one method for this multiclass classification (Hsu and Lin, 2002). This method constructs 2-class SVM classifiers for all possible pairs of classes. When there are K classes, K(K − 1)/2 2-class classifiers are constructed. The values of K are 110 or 19 when we use data 1 and data 2, respectively. We classified given data by majority votes of these K(K − 1)/2 classifiers.
Results
Effects of Pre- and Postsynaptic Inhibition on Input-Output Function
We investigated how global pre- and postsynaptic inhibition modulate PN responses to different odors. We started by examining how inhibition affects the input-output (I-O) function of a single glomerulus, which describes the relationship between ORN and PN firing rates. Without any global inhibition, ORN responses are non-linearly transformed in PNs by preferentially amplifying weak ORN inputs (Figure 4). This low-pass filter is shaped by the combination of short-term depression expressed at ORN-PN synapses and the relative refractory period of PN firing (Kazama and Wilson, 2008). We introduced presynaptic inhibition by decreasing the probability of vesicular release, p, in equation (3). As the probability of release is lowered by presynaptic inhibition, the shape of this filter was linearized (Figure 4A).
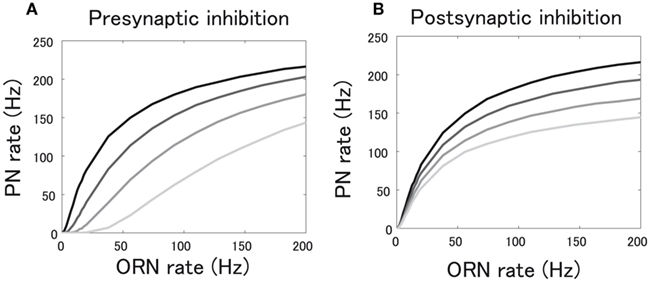
Figure 4. Effects of pre- and postsynaptic inhibition on input-output relationship between ORN and PN firing rates. (A) The case of presynaptic inhibition. The release probability is decreased from the top to the bottom line. The value of release probability is 0.79 in the top line and 0.2 in the bottom line. (B) The case of postsynaptic inhibition. The postsynaptic inhibitory input is increased from the top to the bottom line. The values of postsynaptic inhibitory input are 0 in the top line (no inhibition) and 8 in the bottom line.
On the other hand, postsynaptic inhibition does not change the shape of the I-O relationship because the probability of release is kept constant (Figure 4B). Postsynaptic inhibition just vertically scales the I-O relationship whereas presynaptic inhibition horizontally scales it (Figures 4A,B). Horizontal and vertical scaling are termed as input and output gain control, respectively (Olsen et al., 2010). Our simulation directly demonstrated that input gain control is implemented by presynaptic inhibition and response gain control is implemented by postsynaptic inhibition. This is consistent with two pieces of experimental evidence collected separately that inhibition mainly acts at a presynaptic locus and scaling of PN responses is accurately explained by input gain control (Olsen and Wilson, 2008; Olsen et al., 2010).
Pre- and postsynaptic inhibition differently affect noise of PN responses. Because our ORN neurons fire according to the Poisson statistics, noise of ORN responses gets larger as the firing rate of ORNs increases. Accordingly, PN noise tends to be larger as the firing rate increases. However, PN noise also depends on the relationship between ORN and PN firing rates. As the slope of the I-O relationship gets steep, PN noise increases. As shown in Figure 5A, presynaptic inhibition suppresses the amplification of weak ORN input more strongly than postsynaptic inhibition. As a consequence, PN noise quantified as standard deviation of PN firing rate is smaller with presynaptic inhibition especially when ORN firing rate is low (below 50 Hz) (Figure 5B). Given that more than a half of ORN responses are weaker than 50 Hz (Figure 5C), this preferential noise reduction by presynaptic inhibition is likely to be beneficial.
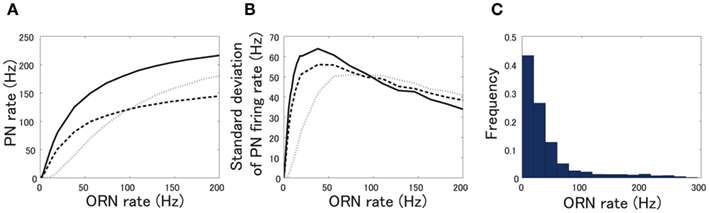
Figure 5. Noise reduction in PN responses by pre- and postsynaptic inhibition. (A) PN firing rate when pre- or postsynaptic inhibition is applied. (B) Standard deviation of PN firing rate when pre- or postsynaptic inhibition is applied. Solid line represents the standard deviation of PN firing rate without inhibition, dotted line represents that with presynaptic inhibition, and dashed line represents that with postsynaptic inhibition. (C) Histogram of ORN firing rate.
Decorrelation by Global Presynaptic Inhibition
Before we consider PN responses to actual odors, we demonstrate the differential effects of pre- and postsynaptic inhibition on PN responses by using two hypothetical odor responses (Figure 6). We assumed that the two odors evoke non-overlapping ORN responses (Figure 6A) and calculated the PN responses in our network model, first without considering lateral inhibition (Figure 6B). We found that the response curves of PNs are broader than those of ORNs. This broadening is produced by non-linear amplification of ORN responses in a glomerulus. When we introduced pre- or postsynaptic inhibition, we found that presynaptic inhibition sharpens the response curve whereas postsynaptic inhibition does not (Figures 6B,C). The normalized response curve with postsynaptic inhibition almost entirely overlaps the normalized curve without inhibition (Figure 6C). This is because postsynaptic inhibition just vertically scales the I-O relationship of a glomerulus while presynaptic inhibition horizontally scales it (Figure 4). This sharpening effect of presynaptic inhibition on odor responses may enhance the odor discriminability between PN responses.
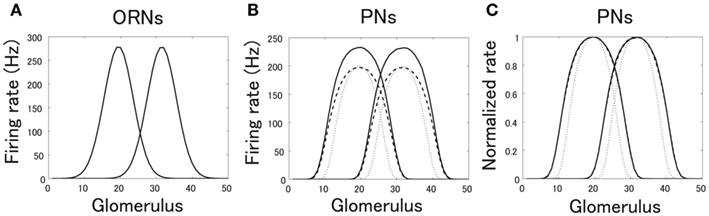
Figure 6. ORN and PN responses to two hypothetical odors. The horizontal axis represents the glomerulus identity. We assumed bell-shaped ORN responses over hypothetical glomeruli. (A) ORN responses. (B,C) PN responses (B) and normalized PN responses (C). PN responses were obtained by simulating the network with the ORN input in (A). Solid line represents the PN response without inhibition, dotted line represents that with presynaptic inhibition, and dashed line represents that with postsynaptic inhibition.
We next quantified how pre- and postsynaptic inhibition change the similarity of odor-evoked PN responses (data 1 in Figure 2) by computing the “overlap” (Linster and Cleland, 2002, 2004). Overlap is a normalized scalar product of two average PN responses. When PN responses to one odor are r1, which is a multi-dimensional vector whose components represent the firing rate of PNs, and PN responses to the other odor are r2, and the overlap ρ is computed as

If we compute the overlaps of two PN responses in Figure 6B, the values are 0.335 in the case without inhibition, 0.132 in the case with presynaptic inhibition, and 0.333 in the case with postsynaptic inhibition. This analysis confirmed that presynaptic inhibition significantly reduces the similarity of PN responses while postsynaptic inhibition essentially does not change it as shown in Figure 6C.
We calculated the overlaps of ORN and PN responses for all pairs of odors in data 1. As discussed before, because of the effect of non-linear amplification in a glomerulus, PN responses have larger overlaps than ORN responses (Figures 7A,B). Importantly, we found that presynaptic inhibition decreases the overlaps of PN responses whereas postsynaptic inhibition does not (Figures 7C,D). The decrease in the overlaps caused by presynaptic inhibition could be beneficial for odor discrimination. However, we note that the decrease in overlaps does not ensure an increase in the odor discriminability because the overall firing rate is also suppressed by inhibition, which basically reduces the information of stimuli contained in neural responses per fixed time period. To enhance the odor discriminability, the beneficial effect of sharpening odor response curves needs to overcome the detrimental effect of decreasing the firing rate. We therefore conducted a network simulation to see whether presynaptic inhibition can actually enhance the odor discriminability.
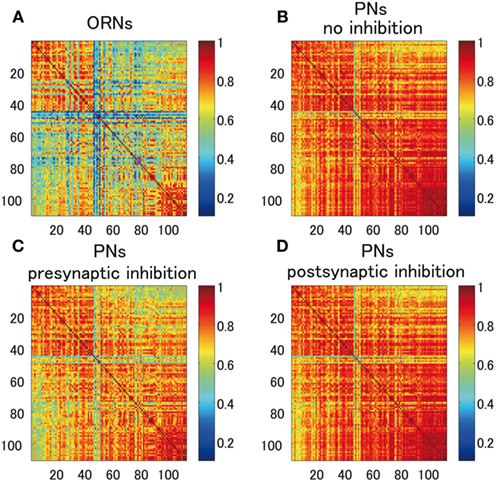
Figure 7. Overlaps of ORN and PN responses for all pairs of odors in data 1 in Figure 2. (A) Overlap of ORN responses. (B) Overlap of PN responses without inhibition. (C) Overlap of PN responses with presynaptic inhibition. The strength of presynaptic inhibition is set to Kpre= 0.35. (D) Overlap of PN responses with postsynaptic inhibition. The strength of postsynaptic inhibition is set to Kpost= 3.0.
Enhancement of Odor Discriminability by Global Presynaptic Inhibition
PN responses from different glomeruli are integrated by third-order neurons, namely Kenyon cells in the mushroom body and cells in the lateral horn. These neurons are considered to discriminate odors by integrating odor information from different glomeruli. To evaluate the odor discriminability, we used a linear classifier used in previous studies (Luo et al., 2010; Olsen et al., 2010) and specifically chose a support vector machine (SVM) classifier (see Materials and Methods for details). We tested several kernels for the SVM classifier but the linear kernel gave the best classification performance. The SVM classifier was trained to identify one out of 110 odors in data 1 when a particular PN response was given.
We found that the correct classification rate increases when presynaptic inhibition is applied and peaks at a certain level of inhibition (Figure 8A1). Meanwhile, presynaptic inhibition decreases the firing rate of PNs averaged over all types of PNs and all stimuli (Figure 8A2). The correct rate increases in spite of the large decrease in firing rate because presynaptic inhibition decorrelates the PN responses to odor stimuli (Figure 7). When the mean firing rate of PNs decreases excessively, the correct classification rate naturally decreases due to this detrimental effect.
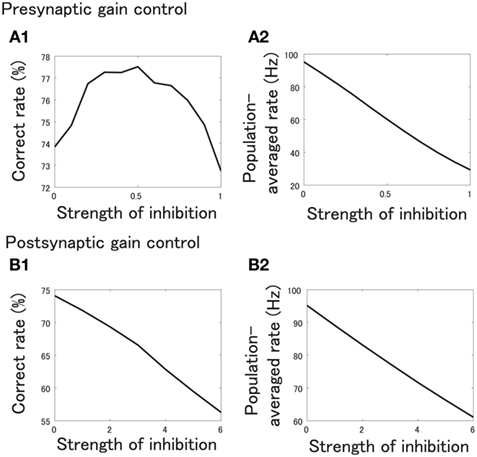
Figure 8. (A1,B1) Correct odor classification rate of SVM classifiers in the case of presynaptic gain control (A1) and postsynaptic gain control (B1). The horizontal axis shows the strength of inhibition, Kpre in (A1), and Kpost in (B1). (A2,B2) Population averaged firing rate of PNs when strength of inhibition, Kpre(A2) or Kpost (B2), is varied.
On the other hand, when inhibition is postsynaptic, the correct classification rate just monotonically decreases as the inhibition becomes stronger (Figures 8B1,B2). This is because postsynaptic inhibition does not change the overlaps of odor-evoked PN responses. Together, we conclude that decorrelation by presynaptic inhibition is a key to enhance the odor discriminability.
We also examined how the correct classification rate changes with the strength of presynaptic inhibition when the value of basal release probability, pmax [equation (7)], is varied. As long as the release probability is higher than a certain value (∼0.4), presynaptic inhibition increases the odor classification rate (Figure 9). This result suggests that the beneficial effects of presynaptic inhibition are robust to moderate changes in the release probability.
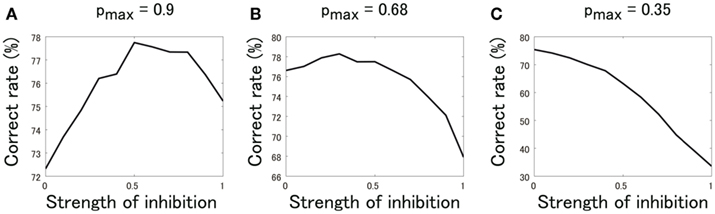
Figure 9. Correct odor classification rate of SVM classifiers when the release probability, pmax, and the strength of presynaptic inhibition, Kpre, are varied. (A) pmax = 0.9. (B) pmax = 0.68. (C) pmax = 0.35.
Discrimination Over a Range of Concentrations
We investigated whether global inhibition beneficially works for discriminating between odors across a range of concentrations by applying a procedure similar to that in the previous section. We used data 2, which describes responses of ORNs to 19 odors at three different concentrations (Figure 2B). We therefore have 57 different ORN responses in total. By using this data, we investigated how accurately the odor identity is determined from simulated PN responses even when the concentration is varied. To be accurate, when we trained the SVM classifiers with these 57 different ORN responses, PN responses evoked by the same odor at three different concentrations were considered to belong to the same class. Thus, the number of classes is only 19. Similar evaluation was done in a previous study (Olsen et al., 2010).
We note that global inhibition should equalize the magnitudes of PN responses evoked at different concentrations because the strength of inhibition depends on the overall activity of ORNs. For example, as the concentration increases, inhibition becomes larger and counteracts the increasing feedforward input. This equalization of PN response magnitudes is expected to be helpful for a classifier to correctly classify the odors across a wide range of concentrations. In fact, both pre- and postsynaptic inhibition equalize the PN response magnitudes (Figures 10A3,B3). One can therefore expect that both types of inhibition could be beneficial for discrimination. However, this is not the case.
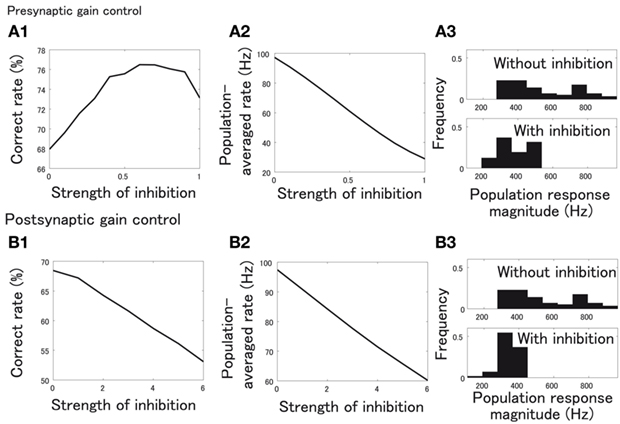
Figure 10. Effects of global presynaptic and postsynaptic inhibition on discrimination between odors over a range of concentrations. (A1,B1) Correct rate of SVM classifiers in the case of presynaptic gain control (A1) and postsynaptic gain control (B1). The horizontal axis shows the strength of inhibition, Kpre in (A1) and Kpost in (B1). (A2,B2) Population averaged rate of PNs when strength of inhibition, Kpre(A2) or Kpost (B2), is varied. (A3,B3) Histogram of PN population response magnitude with inhibition (upper panel) and without inhibition (lower panel). In upper panels, Kpre= 0 (A3) and Kpost= 0 (B3), and in lower panels, Kpre= 0.5 (A3) and Kpost= 5 (B3).
Presynaptic inhibition robustly enhances the correct classification rate even though the firing rate of PNs is largely decreased (Figures 10A1,A2). On the other hand, postsynaptic inhibition only deteriorates the correct classification rate as it decreases the firing rate of PNs (Figures 10B1,B2). These results suggest that response magnitude equalization is not enough for discrimination of odors: what is needed is further decorrelation caused by presynaptic inhibition.
Discussion
By using Drosophila antennal lobe as a model system, we investigated how different neural mechanisms of gain control can confer the olfactory system the ability to discriminate better between odors across a wide range of stimulus intensities. Previous studies suggested that horizontal scaling of the I-O relationship between ORNs and PNs within a glomerulus would be more advantageous than the vertical scaling (Luo et al., 2010; Olsen et al., 2010). Our study demonstrated that presynaptic inhibition is the mechanism that enables horizontal scaling and postsynaptic mechanism enables vertical scaling.
This differential effect of pre- and postsynaptic inhibition stems from the non-linearity of the I-O relationship. If the I-O relationship is non-linear as is shown in Figure 4, PNs receiving weak ORN inputs are more strongly inhibited by presynaptic inhibition than PNs receiving strong ORN inputs. Due to the preferential inhibition, presynaptic inhibition could beneficially act on PNs in terms of noise reduction because the fluctuation of PN firing rate is higher when a PN receives weaker ORN input (Figure 5). The noise source that we considered in this study is only the stochastic firing of ORNs whose variance depends on the firing rate. We note that if we consider a different type of noise whose strength is independent of PN firing rate, this preferential suppression of low ORN input by presynaptic inhibition could be harmful because weaker PN response is more susceptible to noise.
However, the critical effect of presynaptic inhibition is to sharpen the odor tuning by preferentially inhibiting PNs receiving weak ORN inputs. In contrast, postsynaptic inhibition more uniformly inhibits PNs independently of the strength of ORN inputs. This uniform inhibition does not sharpen the odor tuning. If the I-O relationship were perfectly linear, there would be no clear qualitative difference between pre- and postsynaptic inhibition.
The origin of non-linearity of the I-O relationship is at least in part synaptic depression at synapses between ORNs and PNs (Kazama and Wilson, 2008). This non-linear transformation is considered to be helpful for PNs to effectively use their dynamic range and encode information more efficiently (Laughlin, 1981; Bhandawat et al., 2007; Satoh et al., 2010). However, the transformation comes at the cost of elevating the correlation between PN responses (Figure 7B). We found that presynaptic inhibition counteracts this effect and decorrelates PN responses, which was unattainable with postsynaptic inhibition (Figures 7C,D). Decorrelation should tend to make the discrimination between odor-evoked PN responses easier. To test this idea, we examined the performance of support vector machines to classify different PN responses with or without global inhibition. As expected, presynaptic global inhibition increased the odor discriminability whereas postsynaptic global inhibition did not (Figure 8).
Presynaptic inhibition was similarly effective at discriminating between odors over a range of concentrations (Figure 10). Theoretically, equalizing the PN response magnitude is beneficial for a linear discriminator to distinguish between the odors (Luo et al., 2010). We found that this equalization was accomplished by both pre- and postsynaptic inhibition (Figure 10). However, the latter deteriorated the odor discriminability by SVM classifiers (Figure 10). This suggests that decorrelation of PN responses by presynaptic inhibition is the critical factor for accurate odor discrimination.
Our results are consistent with recent studies on the functional role of non-linear ORN-PN transformation and normalization of PN responses (Luo et al., 2010; Olsen et al., 2010). What we have shown further is the specific neuronal mechanisms that give rise to these effects by creating a computational model. We have demonstrated that the combination of ORN-PN synaptic depression and presynaptic inhibition enables PNs to encode olfactory information in a highly separable manner. The strength of synaptic depression and global presynaptic inhibition may be appropriately adjusted in the actual Drosophila olfactory circuit for the animal to discriminate between a wide variety of odors.
Finally, the gain control mechanisms in other modalities could be similarly understood from the viewpoint of efficient information coding (Schwartz and Simoncelli, 2001). Different types of gain control mechanisms could be more advantageous for other modalities because the strategy to code sensory information efficiently depends on the statistics of natural signals (light, sound, etc.) that the organism receives (Barlow, 1989; Olshausen and Field, 1996; Lewicki, 2002). The approach we took here with the Drosophila olfactory circuit may serve as a guide to decipher the neural mechanisms and the functional implications of gain control in other systems.
Conflict of Interest Statement
The authors declare that the research was conducted in the absence of any commercial or financial relationships that could be construed as a potential conflict of interest.
Acknowledgments
This work was supported by a Grant-in-Aid for JSPS Fellows (11J06477) to M. Oizumi. H. Kazama was supported by a grant from RIKEN. M. Okada was supported by Grants-in-Aid for Scientific Research (20240020) from the Ministry of Education, Culture, Sports, Science and Technology of Japan.
References
Asahina, K., Louis, M., Piccinotti, S., and Vosshall, L. B. (2009). A circuit supporting concentration-invariant odor perception in Drosophila. J. Biol. 8, 9.
Betz, W. J. (1970). Depression of transmitter release at the neuromuscular junction of the frog. J. Physiol. (Lond.) 206, 629–644.
Bhandawat, V., Olsen, S. R., Schlief, M. L., Gouwens, N. W., and Wilson, R. I. (2007). Sensory processing in the Drosophila antennal lobe increases reliability and separability of ensemble odor representations. Nat. Neurosci. 10, 1474–1482.
Carandini, M., and Heeger, D. J. (2011). Normalization as a canonical neural computation. Nat. Rev. Neurosci. 13, 51–62.
Chang, C., and Lin, C. J. (2001). LIBSVM: A Library for Support Vector Machines. Available at: http://www.csie.ntu.edu.tw/cjlin/libsvm
Chou, Y. H., Spletter, M. L., Yaksi, E., Leong, J. C. S., Wilson, R. I., and Luo, L. (2010). Diversity and wiring variability of olfactory local interneurons in the Drosophila antennal lobe. Nat. Neurosci. 13, 439–449.
Das, A., Sen, S., Lichtneckert, R., Okada, R., Ito, K., Rodrigues, V., and Reichert, H. (2008). Drosophila olfactory local interneurons and projection neurons derive from a common neuroblast lineage specified by the empty spiracles gene. Neural Dev. 3, 33.
de Bruyne, M., Clyne, P. J., and Carlson, J. R. (1999). Odor coding in a model olfactory organ: the Drosophila maxillary palp. J. Neurosci. 19, 4520–4532.
de Bruyne, M., Foster, K., and Carlson, J. R. (2001). Odor coding in the Drosophila antenna. Neuron 30, 537–552.
Gao, Q., Yuan, B., and Chess, A. (2000). Convergent projection of Drosophila olfactory neurons to specific glomeruli in the antennal lobe. Nat. Neurosci. 3, 780–785.
Hallem, E. A., and Carlson, J. R. (2006). Coding of odors by a receptor repertoire. Cell 125, 143–160.
Hsu, C. W., and Lin, C. J. (2002). A comparison of methods for multi-class support vector machines. IEEE Trans. Neural Netw. 13, 415–425.
Huang, J., Zhang, W., Qiao, W., Hu, A., and Wang, Z. (2010). Functional connectivity and selective odor responses of excitatory local interneurons in Drosophila antennal lobe. Neuron 67, 1021–1033.
Jefferis, G., Marin, E. C., Stocker, R. F., and Luo, L. (2001). Target neuron prespecification in the olfactory map of Drosophila. Nature 414, 204–208.
Kazama, H., and Wilson, R. I. (2008). Homeostatic matching and nonlinear amplification at identified central synapses. Neuron 58, 401–413.
Kazama, H., and Wilson, R. I. (2009). Origins of correlated activity in an olfactory circuit. Nat. Neurosci. 12, 1136–1144.
Lai, S. L., Awasaki, T., Ito, K., and Lee, T. (2008). Clonal analysis of Drosophila antennal lobe neurons: diverse neuronal architectures in the lateral neuroblast lineage. Development 135, 2883–2893.
Laissue, P. P., Reiter, C., Hiesinger, P., Halter, S., Fischbach, K., and Stocker, R. F. (1999). Three dimensional reconstruction of the antennal lobe in Drosophila melanogaster. J. Comp. Neurol. 405, 543–552.
Laughlin, S. (1981). A simple coding procedure enhances a neuron’s information capacity. Z. Naturforsch. C 36, 910–912.
Liley, A. W., and North, K. A. K. (1952). An electrical investigation of effects of repetitive stimulation on mamalian neuromuscular junction. J. Neurophysiol. 16, 509–527.
Linster, C., and Cleland, T. A. (2002). Cholinergic modulation of sensory representations in the olfactory bulb. Neural. Netw. 15, 709–717.
Linster, C., and Cleland, T. A. (2004). Configurational and elemental odor mixture perception can arise from local inhibition. J. Comput. Neurosci. 16, 39–47.
Luo, S. X., Axel, R., and Abbott, L. F. (2010). Generating sparse and selective third-order responses in the olfactory system of the fly. Proc. Natl. Acad. Sci. U.S.A. 107, 10713–10718.
Marin, E. C., Jefferis, G., Komiyama, T., Zhu, H., and Luo, L. (2002). Representation of the glomerular olfactory map in the Drosophila brain. Cell 109, 243–255.
Ng, M., Roorda, R. D., Lima, S. Q., Zemelman, B. V., Morcillo, P., and Miesenböck, G. (2002). Transmission of olfactory information between three populations of neurons in the antennal lobe of the fly. Neuron 36, 463–474.
Okada, R., Awasaki, T., and Ito, K. (2009). Gamma-aminobutyric acid (GABA)-mediated neural connections in the Drosophila antennal lobe. J. Comp. Neurol. 514, 74–91.
Olsen, S. R., Bhandawat, V., and Wilson, R. I. (2007). Excitatory interactions between olfactory processing channels in the Drosophila antennal lobe. Neuron 54, 89–103.
Olsen, S. R., Bhandawat, V., and Wilson, R. I. (2010). Divisive normalization in olfactory population codes. Neuron 66, 287–299.
Olsen, S. R., and Wilson, R. I. (2008). Lateral presynaptic inhibition mediates gain control in an olfactory circuit. Nature 452, 956–960.
Olshausen, B. A., and Field, D. J. (1996). Emergence of simple cell receptive field properties by learning a sparse code for natural images. Nature 381, 607–609.
Root, C. M., Masuyama, K., Green, D. S., Enell, L. E., Nässel, D. R., Lee, C. H., and Wang, J. W. (2008). A presynaptic gain control mechanism fine-tunes olfactory behavior. Neuron 59, 311–321.
Root, C. M., Semmelhack, J. L., Wong, A. M., Flores, J., and Wang, J. W. (2007). Propagation of olfactory information in Drosophila. Proc. Natl. Acad. Sci. U.S.A. 104, 11826–11831.
Satoh, R., Oizumi, M., Kazama, H., and Okada, M. (2010). Mechanisms of maximum information preservation in the Drosophila antennal lobe. PLoS ONE 5, e10644. doi:10.1371/journal.pone.0010644
Schwartz, O., and Simoncelli, E. P. (2001). Natural signal statistics and sensory gain control. Nat. Neurosci. 4, 819–825.
Shang, Y., Claridge-Chang, A., Sjulson, L., Pypaert, M., and Miesenbock, G. (2007). Excitatory local circuits and their implications for olfactory processing in the fly antennal lobe. Cell 128, 601–612.
Stocker, R. F. (1994). The organization of the chemosensory system in Drosophila melanogaster: a review. Cell Tissue Res. 275, 3–26.
Stocker, R. F., Lienhard, M. C., Borst, A., and Fischbach, K. F. (1990). Neuronal architecture of the antennal lobe in Drosophila melanogaster. Cell Tissue Res. 262, 9–34.
Vosshall, L. B., Amrein, H., Morozov, P. S., Rzhetsky, A., and Axel, R. (1999). A spatial map of olfactory receptor expression in the Drosophila antenna. Cell 96, 725–736.
Vosshall, L. B., Wong, A. M., and Axel, R. (2000). An olfactory sensory map in the fly brain. Cell 102, 147–159.
Wilson, R. I., and Laurent, G. (2005). Role of GABAergic inhibition in shaping odor-evoked spatiotemporal patterns in the Drosophila antennal lobe. J. Neurosci. 25, 9069–9079.
Wilson, R. I., Turner, G. C., and Laurent, G. (2004). Transformation of olfactory representations in the Drosophila antennal lobe. Science 303, 366–370.
Wong, A. M., Wang, J. W., and Axel, R. (2002). Spatial representation of the glomerular map in the Drosophila protocerebrum. Cell 109, 229–241.
Yaksi, E., and Wilson, R. I. (2010). Electrical coupling between olfactory glomeruli. Neuron 67, 1034–1047.
Keywords: Drosophila, antennal lobe, odor discriminability, presynaptic inhibition, postsynaptic inhibition, gain control, decorrelation, concentration invariance
Citation: Oizumi M, Satoh R, Kazama H and Okada M (2012) Functional differences between global pre- and postsynaptic inhibition in the Drosophila olfactory circuit. Front. Comput. Neurosci. 6:14. doi: 10.3389/fncom.2012.00014
Received: 06 September 2011; Accepted: 26 February 2012;
Published online: 21 March 2012.
Edited by:
Hava T. Siegelmann, Rutgers University, USAReviewed by:
Germán Mato, Centro Atomico Bariloche, ArgentinaChristiane Linster, Cornell University, USA
John R. Nambu, Florida Atlantic University, USA
Copyright: © 2012 Oizumi, Satoh, Kazama and Okada. This is an open-access article distributed under the terms of the Creative Commons Attribution Non Commercial License, which permits non-commercial use, distribution, and reproduction in other forums, provided the original authors and source are credited.
*Correspondence: Masafumi Oizumi, Laboratory for Mathematical Neuroscience, RIKEN Brain Science Institute, 2-1 Hirosawa, Wako City, Saitama 351-0198, Japan. e-mail: oizumi@brain.riken.jp