Intraindividual reaction time variability is malleable: feedback- and education-related reductions in variability with age
- 1Max Planck Society-University College London Initiative for Computational Psychiatry and Aging Research (ICPAR); Center for Lifespan Psychology, Max Planck Institute for Human Development, Berlin, Germany
- 2Department of Psychology, University of Victoria, Victoria, BC, Canada
- 3Rotman Research Institute, Baycrest, Toronto, ON, Canada
- 4Department of Psychology, University of Toronto, Toronto, ON, Canada
Intraindividual variability (IIV) in trial-to-trial reaction time (RT) is a robust and stable within-person marker of aging. However, it remains unknown whether IIV can be modulated experimentally. In a sample of healthy younger and older adults, we examined the effects of motivation- and performance-based feedback, age, and education level on IIV in a choice RT task (four blocks over 15 min). We found that IIV was reduced with block-by-block feedback, particularly for highly educated older adults. Notably, the baseline difference in IIV levels between this group and the young adults was reduced by 50% by the final testing block, this advantaged older group had improved such that they were statistically indistinguishable from young adults on two of three preceding testing blocks. Our findings confirmed that response IIV is indeed modifiable, within mere minutes of feedback and testing.
Introduction
Moment-to-moment intraindividual variability (IIV) often refers to relatively rapid fluctuations in task performance (see Hultsch et al., 2008; MacDonald et al., 2009a). Particularly with regard to reaction time (RT) measured in a variety of cognitive domains (e.g., simple and choice RT tasks), older adults are typically more inconsistent than younger adults in their response patterns from trial to trial (Hultsch et al., 2008). Evidence suggests that trial-to-trial variability can offer unique predictive utility over and above mean performance level when predicting both normal (e.g., Williams et al., 2005; Lövden et al., 2007) and non-normal aging (e.g., Hultsch et al., 2000; Dixon et al., 2007). IIV is effectively a proxy measure representing a host of complex and dynamic influences and processes. Among several possible cognitive and neural [structural (e.g., lesions); functional (e.g., reduced brain signal dynamics); neuromodulatory (e.g., dopamine degradation); genetic (e.g., val variant of the catechol O-methyltransferase gene)] mechanisms mediating and moderating age-related IIV (MacDonald et al., 2006b, 2009b; Garrett et al., 2011), response variability is thought to partially reflect degradations in age-related frontal lobe-(see Stuss et al., 1994, 2003; MacDonald et al., 2009a) and broader task positive network-mediated cognitive functions (Kelly et al., 2008) such as attention allocation or cognitive control (Bunce et al., 1993; West et al., 2002; Stuss et al., 2003; Duchek et al., 2009; Jackson et al., 2012). Critically, age-based behavioral analyses of the Ex-Gaussian RT distribution suggest that the IIV effect is caused primarily by excessively slow within-person response latencies (West et al., 2002; Williams et al., 2005), possibly a result of momentary lapses in attentional control.
Findings suggesting that neural integrity and efficiency are required for consistent RT performance prompt the question as to whether it is possible to experimentally manipulate IIV in older adults, despite nervous system degradation. Given that attention/control systems are implicated in age-related IIV, these systems may be appropriate targets for attempts to reduce IIV. Evidence suggests that attention/control can improve with effective intrinsic (e.g., a participant's interest in the task) and extrinsic (e.g., external incentives such as points or money) attentional motivation or goal-direction on task (e.g., Tomporowski and Tinsley, 1996; Libera and Chelazzi, 2006; Bengtsson et al., 2009). Ongoing extrinsic motivators may be of particular interest because they can serve as an immediate source of within-task feedback, informing participants of their past and present levels of task performance, and prompting them to adjust their strategic approach if point levels are lower than desired. If IIV does reflect deficits in attention and control, employing methods that improve such deficiencies in older adults may also reduce IIV by limiting overly long response latencies. In addition, goal-directed feedback and training may serve as forms of direct external stimulation and environmental support for healthy older adults (Craik, 1983, 1986), from which task performance can improve and even approach younger adult levels (Naveh-Benjamin et al., 2005). Craik (1983, 1986) argued that by utilizing environmental support, one can alleviate demands on already limited processing/attentional resources; alleviating these demands through performance feedback could be critical for optimizing the consistency of RT responses in older adults.
Another important factor in the context of IIV, feedback, and aging may be level of education, which provides a measure of one's learning ability and intelligence, as well as one's level of cognitive reserve (Stern, 2002). “Cognitive reserve” refers to the point that higher educated older adults are often less susceptible to cognitive impairment, and thus maintain higher levels of cognitive performance compared to their less well-educated peers. Individuals with high cognitive reserve may exhibit less cognitive impairment over time, in part, because they may devise and implement alternative strategies for completing tasks when the methods they employed previously are no longer effective. Essentially, this may represent a willingness or ability to apply different approaches to the same problem. Higher educated adults may thus respond more effectively to feedback paradigms that directly impact their performance. This possible manifestation of reserve may also indicate cognitive flexibility (Lövden et al., 2010), which reflects one's ability to utilize existing functional capacities to rapidly adapt to changing environmental and cognitive demands. Further, better educated older adults may exhibit superior attentional allocation in general (e.g., Tun and Lachman, 2008), possibly yielding lower IIV (Christensen et al., 2005), and allowing a more focused and sustained response to goal-direction and feedback. It thus seems possible that feedback-related impacts on IIV may vary by education level.
In the current study, we examined the effects of goal-directed feedback, age, and education on trial-to-trial IIV over multiple blocks of a four-choice RT task. We anticipated that feedback would reduce IIV by providing motivation and focusing attentional resources on specific aspects of the task, particularly in our highly educated participants. Given older adults' typically greater level of IIV, and younger adults' already superior patterns of response consistency, we expected that older adults would benefit most from feedback. We also examined the effect of feedback, age, and education on mean speed to gauge differences between IIV and mean RTs in our paradigm. Importantly, previous research suggests that IIV and mean RT levels can improve simply through task exposure (i.e., in absence of feedback; e.g., Ram et al., 2005; Ratcliff et al., 2006; Dutilh et al., 2009; Schmiedek et al., 2009). Accordingly, all subjects in our paradigm received the same amount of task exposure, which allowed us to control for any practice-related improvements in IIV while examining the effects of age, feedback, and education level.
Materials and Methods
Participants
We recruited 41 healthy undergraduates (18–34 years) from the University of Toronto (Mage = 21.56 years, SDage = 3.70; Meducation = 14.22 years, SDeducation = 1.82) and 57 healthy, community-dwelling older adults (60–82 years) from Toronto and surrounding communities (Mage = 70.95 years, SDage = 4.94; Meducation = 16.06 years, SDeducation = 2.18; unfortunately, reliable information on ethnicity/nationality was not available for the current sample). Young adults received course credit and older adults received $15 for their participation. The Office of Research Ethics at the University of Toronto approved the current study.
Task
We administered a four-choice RT task that contained four blocks of trials, and 40 trials per block. Participants were shown four white squares (2″ × 2″ each) in a horizontal line on a black background on a 15″ laptop computer screen. When one of the squares turned red, participants were asked to press one of four buttons on a response box corresponding to the location of the red square. To encourage consistent attentional allocation throughout each block, participants were instructed to make consistently quick and accurate responses. We utilized a continuous RT task format that required correct response button presses; the next stimulus appeared immediately (and only) after a correct response was made, without any interstimulus interval. As such, accuracy for each participant was guaranteed to be 100%. Continuous RT tasks may provide more intrinsic attentional motivation than ISI-based RT tasks, as participants can progress through such tasks at a pace that matches their performance level (Hazlett et al., 2001).
Four-choice RT tasks have proved useful in models that relate IIV to both age and cognitive status (e.g., Hultsch et al., 2002; Dixon et al., 2007), and a host of other studies have also successfully employed a number of variants of the choice RT paradigm in IIV research (e.g., Shammi et al., 1998; Rabbitt et al., 2001; Murtha et al., 2002; Anstey et al., 2005; Williams et al., 2005). Our decision to use four-choice rather than the more typical two-choice paradigm was based on previous research suggesting that age differences can become more marked (i.e., greater between-group variance) when greater processing requirements are placed upon participants (West et al., 2002). Further, it was important that the task not be too difficult in order to promote participant motivation and engagement on task. The four-choice option seemed reasonable to avoid both floor and ceiling effects, while providing enough difficulty to allow improvement over blocks to occur.
Procedure and Feedback Paradigm
Half of each age group received feedback and the other half did not (participants were randomly assigned). Participants receiving feedback were told prior to the beginning of the paradigm that they would receive 10 points for each consistently quick response, lose 10 for a somewhat slow response, lose 20 for a very slow response, and lose 50 for an extremely slow response. Feedback was provided immediately after each block of 40 trials. Participants were shown three types of feedback at each feedback occasion. First, we plotted the distance of each of the 40 trials from the within-subject median of the immediately preceding block (on the first feedback block, it was necessary to use the block 1 median). Any trial on which participants responded +0.5 standard deviations (SDs) or quicker in relation to their own median, they were awarded 10 points (see green zone in Figure 1A). Participants lost 10 points for responses from +0.5 to +2 SDs (yellow zone), lost 20 points for responses from +2 to +4 SDs (orange zone), and lost 50 points for responses from +4 to +7 SDs (red zone) above their own median in the preceding block. Using the immediately preceding median provided a “moving target” that encouraged continuous improvement throughout the entire task. Importantly, although abnormally fast responses also mathematically increase indices of response inconsistency, evidence suggests that it is overly slow trials that often yield group differences in inconsistency (cf. West et al., 2002). Thus, we deliberately discouraged participants' slower responses in the current feedback paradigm by only penalizing point values for higher RTs. In any case, unrealistically quick responses were also trimmed prior to statistical model runs in the current paper (see details on RT data preparation below).
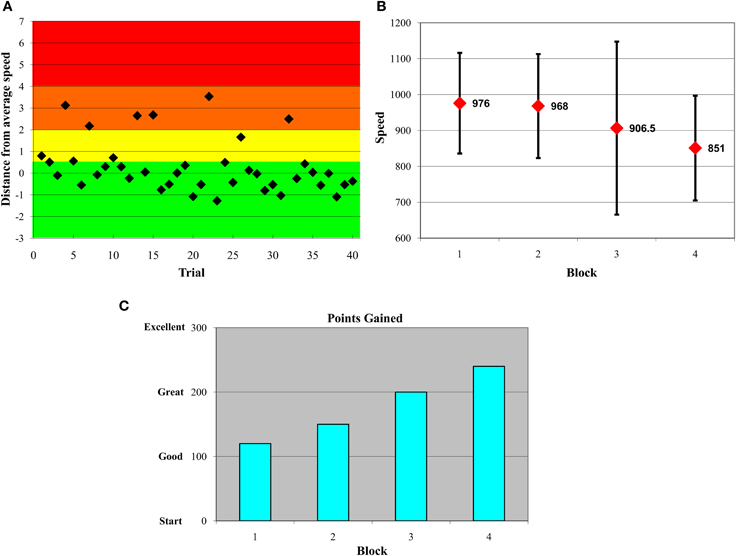
Figure 1. Variability-reduction feedback plots. All participants in the feedback condition were shown plots such as these in serial order. Plots (A–C) represent data from an example participant. (A) Within-block trial-by-trial performance feedback plot. Participants were awarded 10 points if a trial response was in the green zone, −10 if in the yellow zone, −20 if in the orange zone, and −50 if in the red zone. The y-axis represents numbers of standard deviations from a participant's own median from the previous block. (B) Feedback plot of response time medians and SDs across blocks. (C) Feedback plot of points gained across blocks.
This first feedback plot (shown in Figure 1A) also facilitated provision of feedback on overall patterns of inconsistent responses within-block and -person. For example, some participants were inconsistent at the beginning of a block of trials; in this case, we would emphasize to the participant that, on the next block of trials, they should focus their attention from the very first trial in an attempt to reduce their response variability. The second feedback graph plotted participants' median response time and their SDs for each block (see Figure 1B). This allowed participants to gauge their progress with regard to improved speed and consistency across blocks. The third feedback graph (see Figure 1C) plotted points gained across blocks, referencing the trial-by-trial points-based feedback plots shown initially during feedback (see Figure 1A). To maintain task motivation, the y-scale on this plot went from “Start” to “Good” to “Great” to “Excellent,” and was designed deliberately to avoid any negative feedback. Critically, feedback was designed to reflect within-subject performance, and this ensured that participants attempted to improve relative to their own level of functioning. Participants were also encouraged to ask questions about their performance, and to propose ideas for their own improvement (which testers commented upon); this fostered an interactive dynamic between participant, tester, and feedback material. If participants' ideas were not logical, feasible, or permitted (e.g., “should I press all buttons rapidly to ensure correct answers?”), testers dissuaded participants from proceeding in that fashion. Most often, following feedback, participants appeared relatively aware of what they could do on the next block of trials to improve; as a result, testers were more positive and supportive than dissuasive. The paradigm (four blocks of 40 trials) took approximately 5 min for the Control groups (those not receiving feedback), and 10–15 min for the Feedback groups.
RT Data Preparation
To prepare the RT data prior to ISD calculation, we adopted an approach employed previously (Hultsch et al., 2000, 2008; Dixon et al., 2007). First, extremely fast or slow responses could reflect common types of key press errors (e.g., accidental key press, interruption of the task), and thus, a lower bound for legitimate responses (150 ms) was set for each RT task on the basis of minimal RTs suggested by prior research (see MacDonald et al., 2006a; Dixon et al., 2007). An initial upper bound was determined by examining frequencies of RTs and trimming extreme outliers relative to the rest of the sample; we dropped all scores above 4000 ms. Following initial upper-bound trims, we proceeded to drop all trials exceeding within-subject block means by ± 3 SDs. The proportion of trials dropped and trimmed across the entire Persons × Trials data matrix was minimal; of 15,680 total trials, we trimmed only 187 (1.19%). The range of missing trials across subjects (range = 0.00–3.75%) was also minimal. To maintain complete data, we imputed trimmed values for outlier trials by using a regression imputation procedure (as implemented in SPSS 18.0) from which missing value estimates were based on the relationships among responses across trials from all participants.
Index of IIV
Although there are multiple indices of IIV (see Hultsch et al., 2008), we employed the ISD. Importantly, computation of the ISD permits the researcher to systematically separate confounds of relevance in aging (e.g., age and practice effects). Computing ISDs on raw scores can be problematic; significant group differences in average level of performance are typically observed, and such differences are often positively correlated with differences in raw SD values. In addition, systematic changes across trials may be present (e.g., practice, learning effects). To address these potential confounds, we used a regression procedure developed by Hultsch et al. (Hultsch et al., 2000, 2008) to residualize the RT data prior to calculating ISDs. Using a person × trial data matrix (i.e., the data were structured in person-period format), we employed multiple regression to partial age group, feedback, education, and occasion effects (trials and blocks) and all interactions by regressing four-choice RT on these potential confounding variables. Then, within-person SDs (i.e., ISDs) were computed for each block using the choice-RT trial-based residuals from our regression model.
Statistical Analyses
In a balanced design (all participants had complete data for all four blocks), we ran separate repeated-measures general linear models, in which we examined: (1) the ISD of all four blocks in relation to age group (young vs. old), feedback group (feedback vs. no feedback), years of education (continuous variable), and all interactions, and; (2) the mean RT of all four blocks in relation to the same covariates (age group, feedback, education, and all interactions). Because education was entered in our models as a continuous variable, and feedback and age group were categorical, we adapted a common approach to plotting categorical × continuous interactions (Aiken and West, 1991) for use with repeated measures modeling. Parameter estimates derived from a regression at each block (i.e., regressing ISD at each block separately on age, feedback, education, and their interactions) were utilized to plot average point estimates for specific levels within the interaction (e.g., in an Age × Feedback × Education interaction). In line with Aiken and West, all interactions that involved Education (a continuous variable) were evaluated at low (−1 SD from the sample mean, 13.42 years) and high (+1 SD from the sample mean, 17.64 years) levels of education. Then, once all point estimates were determined for each block, within-interaction-level point estimates were joined across blocks to visualize group slopes. We then proceeded to bootstrap these point estimates to derive 95% confidence intervals (CIs; percentile method; Efron and Tibshirani, 1986, 1993) using 1000 resamples (with replacement) of our data. These CIs allowed us to compare point estimates within and across blocks. For ease of reporting throughout, we refer to levels of each interaction as “groups” [e.g., an older, feedback, high educated (OFH) group] even though education (continuous) was part of the interaction and was evaluated at ±1 SD from the sample mean. SPSS 18.0 was employed for all analyses.
Results
ISD Analyses
We found several robust interactions, most notably, a Block × Age × Feedback × Education effect (see Table 1 for model results and Figure 2A for a visual depiction). To further examine this interaction, we first ran separate Block × Feedback × Education models for each age group (see Table 1). There were no significant effects in the young group (all p's > 0.48), suggesting that neither Feedback nor Education had an impact on ISD scores. However, in older adults, all effects were substantial, with estimates of effect size (partial η2) greater than 0.35 for each effect (see Table 1). To post-hoc probe differences between point estimates plotted in Figure 2A, we computed bootstrapped 95% confidence intervals (1000 model runs, using resampling with replacement) around each estimate for older adults (given significant main effects and interactions within this group), and for the younger group as a whole (given a complete absence of robust differences between point estimates across blocks). We were particularly interested in differences in ISD values at, or in relation to, Block 4, as this block represented participants' final chance at performance after the maximum amount of possible feedback exposure (i.e., for Feedback groups). Key Block 4 comparisons revealed that following the final session of performance feedback, the OFH group exhibited more consistent performance than either the older, control, low educated (OCL) or older, control, high educated (OCH) groups (i.e., bootstrapped 95% CIs did not cross over; see Figure 2A). Most importantly, the OFH group had improved by Block 4 to the extent they were statistically indistinguishable from the young group at either of Blocks 1 or 3. Despite overall reductions in ISDs across blocks, no other older group approached the young group at any Block.
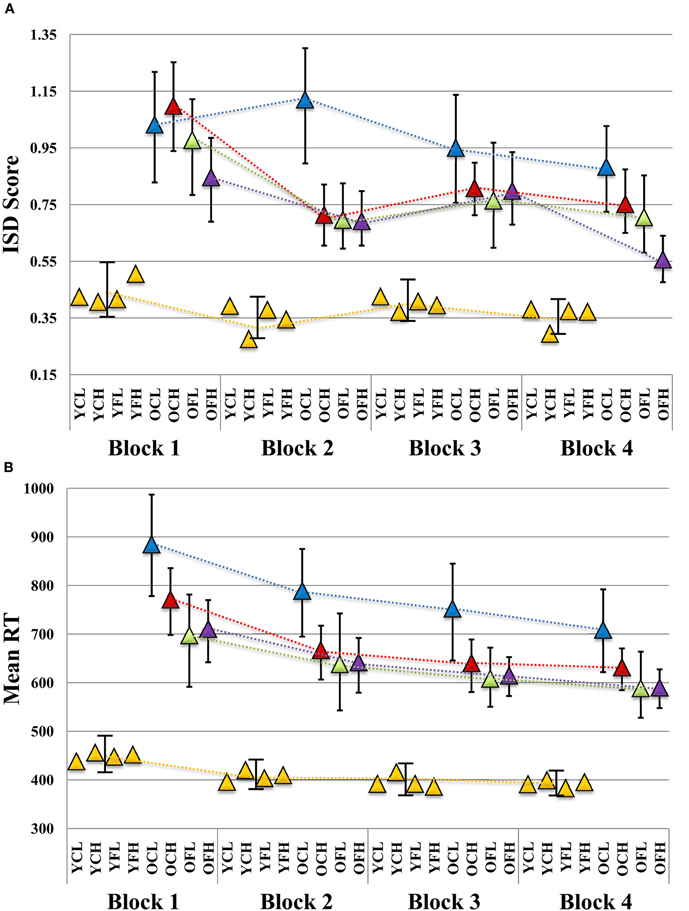
Figure 2. Plot of block-wise (A) ISD and (B) mean RT results in relation to age, feedback, and education level. ISD, intraindividual standard deviation; YCL, younger, control, lower education; YCH, younger, control, higher education; YFL, younger, feedback, lower education; YFH, younger, feedback, higher education; OCL, older, control, lower education; OCH, older, control, higher education; OFL, older, feedback, lower education; OFH, older, feedback, higher education. All slopes were plotted according to Aiken and West's (1991) method. Using betas for each block (including all main effects and interaction terms), point estimates were determined while evaluating education at +1 SD (17.64 years) and −1 SD (13.42 years) from the sample mean, and dummy coding age (young vs. old) and feedback (control vs. feedback) groups. Triangles indicate point estimate values. Error bars for each point estimate refer to bootstrapped 95% confidence intervals derived from 1000 resamples (with replacement) of our original data (N = 98). Where bars do not overlap, this indicates a robust bootstrapped difference between point estimates. (A) Given no differences between young adult subgroups in any of our results (all young model effect p's > 0.48; see Results), we provide a single young group bootstrapped CI per block for comparison to older subgroups. (B) A similar plot is provided for mean RT.
Descriptively, the OFH group closed the gap in ISD levels between them and the young group by a substantial margin by Block 4. The difference in ISDs between young and OFH groups at Block 1 was exactly 50% smaller at Block 4, nearly 11% better than the next best older group (the OCH group, see Table 2). The OFH group also showed the greatest within-group improvement between Blocks 1 and 4 (34% reduction in ISD scores) relative to other older groups (see Table 2). The OCL group was noticeably poorer, showed the least improvement across blocks (9.32%), and remained the furthest from Young adult performance of all older groups by nearly 30%.
Mean RT Analyses
Unlike for our ISD analyses, we found only a single reliable effect in our mean RT-based Block × Age Group × Feedback × Education model (see Table 1). A modest Block × Age Group interaction was present (p = 0.051; partial η2 = 0.08), which denoted a slightly increased rate of mean RT improvement over blocks for the older groups (see Figure 2B). For all blocks, all older subgroups were statistically different from young adults.
Discussion
In the current study, we examined the effect of interactive, goal-directed feedback on reductions in response time variability in younger and older adults. We anticipated that such feedback would reduce IIV by providing motivation and focusing attentional resources on task, and would specifically inhibit overly slow responses that typically underlie variability effects (West et al., 2002; Williams et al., 2005) by providing environmental support (i.e., feedback) to alleviate strains on processing resources (Craik, 1983, 1986). We also anticipated that higher educated older adults (education was used as a proxy measure for cognitive reserve; Stern, 2002) would be more likely to benefit from feedback, possibly due to their willingness to adopt different strategies on task (which our feedback paradigm could have helped provide), or due to their typically superior attentional abilities (which may have allowed a more sustained and focused response to performance feedback). Indeed, we confirmed substantial feedback-related reductions in IIV for older adults, most prominently for those with higher education. This suggests that IIV was significantly malleable for this group as a result of relatively short, incentive-based, interactive visual and auditory feedback. This effect was surprisingly strong even though feedback and task blocks took only 10–15 min to administer, effectively eliminating differences between the OFH group and young adults on two of the four blocks of measurement. Thus, although IIV can be a stable within-person trait (e.g., Hultsch et al., 2008), our findings indicate that age-related IIV is certainly modifiable, even within a remarkably short period of feedback and testing. Further, all our groups had the same amount of task exposure/practice (4 block of 40 trials each); thus, the OFH group reduction in IIV was present over and above typical practice-related improvements noted in previous work (e.g., Ram et al., 2005; Ratcliff et al., 2006; Dutilh et al., 2009; Schmiedek et al., 2009).
Our OCL group showed the least performance gains across blocks. This is interesting given that “low” education was evaluated at 13.42 years (the lower bound for our sample was 12 years), hardly low by epidemiological standards. It thus appears that reliable individual differences in ISD malleability exist even within a sample of only those with high school education or more. Also of note, young adults did not respond to feedback, and were relatively consistent across all four task blocks. It is typical and expected for young adults to perform relatively consistently on RT tasks such as the one we employed in the present study (e.g., Hultsch et al., 2002; West et al., 2002; Williams et al., 2005). The processing resources required for young adults to perform quickly and consistently on such tasks are relatively minimal compared to older adults, perhaps indicating a functional bound where feedback would have little or no effect on further ISD reductions. This is supported by previous work showing that young adults' RT variability improves relatively little with practice (e.g., Ratcliff et al., 2006). However, it is also possible that our feedback paradigm simply wasn't optimized for younger adults to improve on already excellent levels of performance, or that our choice RT task was too simple for feedback to have any notable effect. Follow-up paradigms and task types may address these issues.
ISDs vs. Mean RTs
We observed several systematic age-, feedback-, and education-related effects that could not be captured using mean RT; the mean was simply less sensitive to these block-to-block changes. In line with several previous studies, this suggests that IIV continues to offer differential and unique information regarding RT performance (see Hultsch et al., 2008; MacDonald et al., 2009a; Schmiedek et al., 2009), and can be targeted directly by feedback. In several contexts, IIV is more sensitive than mean RT when relating to a variety of phenomena, including normal aging and mild cognitive impairment (e.g., Dixon et al., 2007) and developmental increases in brain variability (McIntosh et al., 2008). In general, IIV measures may reveal theoretically important aspects of cognitive function that cannot be captured by measure of central tendency (Spieler et al., 2000), such as age-related lapses in attentional control (Bunce et al., 1993; West et al., 2002; Stuss et al., 2003; Duchek et al., 2009; Jackson et al., 2012) rather than overall psychomotor slowing. Unsurprisingly, the utility of examining IIV extends to non-cognitive domains as well. For example, recent work suggest that brain signal variability is a far more powerful and sensitive predictor of aging than is mean signal, and highlights a broad set of regions that are not detectable by examining only mean-based patterns (Garrett et al., 2010, 2011). Thus, examining IIV across scientific lines of inquiry continues to offer a variety of meaningful sources of information about the aging process that mean-based measures cannot provide.
Targeting the Cognitive and Neural Components of IIV
Given the nature and design of our paradigm, our findings give credibility to arguments that performance variability may partially reflect failures of attentional control (see Bunce et al., 1993; West et al., 2002). By specifically providing environmental support (cf. Craik, 1983, 1986) via feedback to reduce overly slow trials that presumably result from attentional lapses, we can reduce variability (for an alternative, but related theoretical account reflecting “processing efficiency” rather than attentional lapses, see Ratcliff et al., 2006, 2008; Dutilh et al., 2009). Although aging-related response variability reflects various endogenous neural mechanisms such as degraded white matter integrity (e.g., Jackson et al., 2012; Tamnes et al., 2012), reduced brain variability and dynamics (Garrett et al., 2011), and inefficient neuromodulatory transmission (see MacDonald et al., 2006b, 2009a), the rapid improvements in ISD levels we found suggest that it is possible to maximize one's existing neural substrate by providing cognitively oriented feedback and motivation on task. Unsurprisingly, higher educated (reserve) older adults were most able to maximize their functional capacity by effectively applying feedback to improve performance, perhaps through a greater level of cognitive flexibility (Lövden et al., 2010) and/or a willingness to apply different cognitive approaches to performance (Stern, 2002).
It could be argued that the rapid reductions in IIV our data are divergent from previous research indicating that performance variability is a function of nervous system integrity/efficiency. That is, if our paradigm can improve IIV over a few minutes, can nervous system integrity/efficiency really be an effective mechanistic explanation? We would argue that our results do not directly detract from IIV-nervous system links. Of course, rapid improvements in IIV would not reflect immediate changes in structural integrity (e.g., white matter) or genetic expression (e.g., val or met variants of COMT). However, changes in efficiency at the functional/network level are certainly possible over short periods. The human brain is a highly dynamic structure, within which functional networks form and change naturally from moment to moment across multiple time scales, despite the presence of a stable white matter skeleton (Honey et al., 2007, 2009). Although we do not present neuroimaging data in the current study, it is conceivable that attention/control-related functional networks (e.g., Kelly et al., 2008) may operate more efficiently over minutes (possibly as a result of top-down modulation following feedback and task exposure), particularly in our OFH group. However, whether further training blocks/task exposure would fully counteract older age- and lower education-related network inefficiencies remains unknown, but is doubtful. Functional changes will always be bounded, even if relatively liberally, by stable elements within the system (e.g., age-related degradations in brain structure). Regardless changes in IIV must be represented within the brain, and relatively rapid functional change is the most obvious candidate.
On the Non-Linear Trends Across Blocks
Three of our four older subgroups exhibited a similar non-linear trend across blocks in which an initial burst of improvement after the first feedback occasion (at Block 2) was followed by an uptick in variability at Block 3, and another reduction in variability by Block 4 (to a lesser extent, this trend was similarly noticeable in the young adult subgroups; see Figure 2A). Along a different trajectory, our poorest performing group (older low educated controls) also showed fluctuations in gains and losses across blocks. Although it may appear somewhat surprising that such fluctuations in across-block variability could occur (particularly the uptick from Blocks 2 to 3), this pattern may be expected. During the acquisition and improvement/practice of cognitive performance, greater variability can indicate an adaptive process indicative of learning, as well as strategy development, employment, and adjustment. Only when asymptotic performance is reached is further variability considered maladaptive (Siegler, 1994; Li et al., 2004). From this perspective, one could predict that our OFH group (with a combination of cognitive reserve, goal-directed feedback, and possible resulting strategy modifications) may continue to appear variable in their level of across-block ISD performance over multiple successive blocks than would other older groups. The OFH group did exhibit the most extreme change from Blocks 3 to 4, whereas the other three older groups exhibited a similarly modest change in slope across these two blocks (see Figure 2A), perhaps indicating a more rapidly approaching performance asymptote for them. In any case, across-block variability in within-block performance may be expected until an asymptote is reached, regardless of feedback paradigm, task, or sample.
Potential Caveats and Future Research Possibilities
First, the various practical implications of, and precise mechanisms driving, our results require future study. Regarding practical implications, issues central in many cognitive training/feedback studies often include: (1) the possibility of functional improvement in older adults' lives; (2) the presence of “far transfer” (i.e., that training in one cognitive domain yields gains in another domain, and; (3) the longevity of training-related gains (i.e., do gains last minutes, days, weeks, months?). Regrettably, we cannot directly address any of these issues with our present data. Our primary intention here was only to examine whether IIV was malleable in the short-term using a targeted feedback paradigm in the context of young and older adults of differing education levels. Also, because age and education are multiply determined proxy measures that represent a host of different cognitive, neural, and physical processes, the precise mechanisms driving our findings require further characterization. We thus offer our present paradigm and results as a first look at the feedback-related malleability of IIV.
Second, to fully appreciate the impact of age, feedback, and education on reductions in IIV, future studies could employ paradigms with a greater number of testing blocks. Although our brief paradigm revealed several interesting effects that were verified via 1000 unbiased, bootstrapped model runs, it would be ideal to establish the IIV asymptote for each group, and whether all older groups, or only the OFH group, ultimately approach young adult levels of performance. Previous work examining IIV on a three-back spatial working memory task over 100 daily sessions established that older adult IIV levels largely asymptote after approximately five or six sessions (Schmiedek et al., 2009); whether this same number of sessions would also produce an asymptote within a single day, multi-block, multi-group paradigm such as ours remains unknown.
Finally, to better understand how older adult IIV reduces with practice, feedback, and education levels, future work could pursue how IIV malleability is reflected in changes in brain function (as noted above). For example, previous research (Kelly et al., 2008) indicated that greater RT IIV can reflect less efficient transitions (and lower anti-correlations) between default mode (a primary resting-state network that activates largely in absence of externally demanded attention) and task positive network functioning (a network active upon externally demanded attention). It would be interesting to examine whether our across-block reductions in IIV may be reflected in greater default mode-task positive network anti-correlations. Also, recent aging-related research demonstrated that higher RT variability was robustly related to lower brain signal variability across perceptual matching, attentional cueing, and delayed match-to-sample tasks (Garrett et al., 2011). It is thus plausible that reductions in IIV across blocks may covary with increases in brain signal variability. A host of studies now support the point that greater brain variability can be an excellent indicator of well-functioning neural systems, reflecting features such as greater network complexity, system criticality, long-range functional connectivity, increased dynamic range and information transfer, and heightened signal detection (e.g., Li et al., 2006; Faisal et al., 2008; McIntosh et al., 2008, 2010; Shew et al., 2009, 2011; Garrett et al., 2010, 2011; Deco et al., 2011; Misic et al., 2011; Vakorin et al., 2011). A direct manipulation of both behavioral and brain variability would not only be an excellent test of their covariance, it would also be helpful for establishing which neural regions best exhibit adjustments in neural dynamics to brief, cognitively oriented feedback paradigms such as ours.
Concluding Remarks
In the current study, we employed a novel, goal-directed, and interactive feedback paradigm designed to attenuate IIV in response time through a hybrid of extrinsic motivation and heightened attentional allocation/control on task. Our findings suggest that response IIV is indeed modifiable, but that the beneficial effects of feedback may be specific to age group and level of education.
Conflict of Interest Statement
The authors declare that the research was conducted in the absence of any commercial or financial relationships that could be construed as a potential conflict of interest.
Acknowledgments
At various stages of this work, Douglas D. Garrett was supported by an IODE War Memorial Award, a doctoral Canada Graduate Scholarship from the Natural Sciences and Engineering Research Council of Canada, the Sir James Lougheed Award of Distinction from Alberta Scholarship Programs, the Naomi Grigg Fellowship for Postgraduate Studies in Gerontology from Soroptimist International of Toronto, and the Men's Service Group Graduate Student Fellowship from the Rotman Research Institute, Baycrest. Stuart W. S. MacDonald is supported by a Career Investigator Scholar Award from the Michael Smith Foundation for Health Research. This study was also supported by a grant from the Natural Sciences and Engineering Research Council of Canada to Fergus I. M. Craik.
References
Aiken, L. S., and West, S. G. (1991). Multiple Regression: Testing and Interpreting Interactions. Thousand Oaks, CA: Sage.
Anstey, K. J., Dear, K., Christensen, H., and Jorm, A. F. (2005). Biomarkers, health, lifestyle, and demographic variables as correlates of reaction time performance in early, middle, and late adulthood. Q. J. Exp. Psychol. A 58, 5–21.
Bengtsson, S. L., Lau, H. C., and Passingham, R. E. (2009). Motivation to do well enhances responses to errors and self-monitoring. Cereb. Cortex 19, 797–804.
Bunce, D. J., Warr, P. B., and Cochrane, T. (1993). Blocks in choice responding as a function of age and physical fitness. Psychol. Aging 8, 26–33.
Christensen, H., Dear, K. B. G., Anstey, K. J., Parslow, R. A., Sachdev, P., and Jorm, A. F. (2005). Within-occasion intraindividual variability and preclinical diagnostic status: is intraindividual variability an indicator of mild cognitive impairment? Neuropsychology 19, 309–317.
Craik, F. I. M. (1983). On the transfer of information from temporary to permanent memory. Philos. Trans. R. Soc. Lond. B Biol. Sci. 302, 341–359.
Craik, F. I. M. (1986). “A functional account of age differences in memory,” in Human Memory and Cognitive Capabilities, Mechanisms and Performance, eds F. Klix H. Hagendorf (Amsterdam: Elsevier), 409–422.
Deco, G., Jirsa, V. K., and McIntosh, A. R. (2011). Emerging concepts for the dynamical organization of resting-state activity in the brain. Nat. Rev. Neurosci. 12, 43–56.
Dixon, R. A., Garrett, D. D., Lentz, T. L., MacDonald, S. W. S., Strauss, E., and Hultsch, D. F. (2007). Neurocognitive markers of cognitive impairment: exploring the roles of speed and inconsistency. Neuropsychology 21, 381–399.
Duchek, J. M., Balota, D. A., Tse, C. S., Holtzman, D. M., Fagan, A. M., and Goate, A. M. (2009). The utility of intraindividual variability in selective attention tasks as an early marker for Alzheimer's disease. Neuropsychology 23, 746–758.
Dutilh, G., Vandekerckhove, J., Tuerlinckx, F., and Wagenmakers, E. J. (2009). A diffusion model decomposition of the practice effect. Psychon. Bull. Rev. 16, 1026–1036.
Efron, B., and Tibshirani, R. J. (1986). Bootstrap methods for standard errors, confidence intervals, and other measures of statistical accuracy. Stat. Sci. 1, 54–77.
Efron, B., and Tibshirani, R. (1993). An Introduction to the Bootstrap. Boca Raton, FL: Chapman & Hall/CRC.
Faisal, A. A., Selen, L. P., and Wolpert, D. M. (2008). Noise in the nervous system. Nat. Rev. Neurosci. 9, 292–303.
Garrett, D. D., Kovacevic, N., McIntosh, A. R., and Grady, C. L. (2010). Blood oxygen level-dependent signal variability is more than just noise. J. Neurosci. 30, 4914–4921.
Garrett, D. D., Kovacevic, N., McIntosh, A. R., and Grady, C. L. (2011). The importance of being variable. J. Neurosci. 31, 4496–4503.
Hazlett, E. A., Dawson, M. E., Schell, A. M., and Neuchterlein, K. H. (2001). Attentional stages of information processing during a continuous performance test: a startle modification analysis. Psychophysiology 38, 669–677.
Honey, C. J., Kotter, R., Breakspear, M., and Sporns, O. (2007). Network structure of cerebral cortex shapes functional connectivity on multiple time scales. Proc. Natl. Acad. Sci. U.S.A. 104, 10240–10245.
Honey, C. J., Sporns, O., Cammoun, L., Gigandet, X., Thiran, J. P., Meuli, R., and Hagmann, P. (2009). Predicting human resting-state functional connectivity from structural connectivity. Proc. Natl. Acad. Sci. U.S.A. 106, 2035–2040.
Hultsch, D. F., MacDonald, S. W. S., and Dixon, R. A. (2002). Variability in reaction time performance of younger and older adults. J. Gerontol. B. Psychol. Sci. Soc. Sci. 57B, P101–P115.
Hultsch, D. F., MacDonald, S. W. S., Hunter, M. A., Levy-Bencheton, J., and Strauss, E. (2000). Intraindividual variability in cognitive performance in older adults: comparison of adults with mild dementia, adults with arthritis, and healthy adults. Neuropsychology 14, 588–598.
Hultsch, D. F., Strauss, E., Hunter, M. A., and MacDonald, S. W. S. (2008). “Intraindividual variability, cognition, and aging,” in The Handbook of Aging and Cognition (3rd edn.), eds F. I. M. Craik and T. A. Salthouse (New York, NY: Psychology Press), 491–556.
Jackson, J. D., Balota, D. A., Duchek, J. M., and Head, D. (2012). White matter integrity and reaction time intraindividual variability in healthy aging and early-stage Alzheimer disease. Neuropsychologia 50, 357–366.
Kelly, A. M. C., Uddin, L. Q., Biswal, B. B., Castellanos, F. X., and Milham, M. P. (2008). Competition between functional brain networks mediates behavioral variability. Neuroimage 39, 527–537.
Li, S-C., Huxhold, O., and Schmiedek, F. (2004). Aging and attenuated processing robustness: evidence from cognitive and sensorimotor functioning. Gerontology 50, 28–34.
Li, S-C., van Oertzen, T., and Lindenberger, U. (2006). A neurocomputational model of stochastic resonance and aging. Neurocomputing 69, 1553–1560.
Libera, C. D., and Chelazzi, L. (2006). Visual selective attention and the effects of monetary rewards. Psychol. Sci. 17, 222–227.
Lövden, M., Bäckman, L., Lindenberger, U., Schaefer, S., and Schmiedek, F. (2010). A theoretical framework for the study of adult cognitive plasticity. Psychol. Bull. 136, 659–676.
Lövden, M., Li, S. C., Shing, Y. L., and Lindenberger, U. (2007). Within-person trial-to-trial variability precedes and predicts cognitive decline in old and very old age: longitudinal data from the Berlin Aging Study. Neuropsychologia 45, 2827–2838.
MacDonald, S. W. S., Hultsch, D. F., and Bunce, D. (2006a). Intraindividual variability in vigilance performance: does degrading visual stimuli mimic age-related “Neural Noise?”. J. Clin. Exp. Neuropsychol. 28, 655–675.
MacDonald, S. W. S., Nyberg, L., and Bäckman, L. (2006b). Intra-individual variability in behavior: links to brain structure, neurotransmission and neuronal activity. Trends Neurosci. 29, 474–480.
MacDonald, S. W. S., Li, S. C., and Backman, L. (2009a). Neural underpinnings of within-person variability in cognitive functioning. Psychol. Aging 24, 792–808.
MacDonald, S. W. S., Li, S. C., and Bäckman, L. (2009b). Neural underpinnings of within-person variability in cognitive functioning. Psychol. Aging 24, 792–808.
McIntosh, A. R., Kovacevic, N., and Itier, R. J. (2008). Increased brain signal variability accompanies lower behavioral variability in development. PLoS Comput. Biol. 4:e1000106. doi: 10.1371/journal.pcbi.1000106
McIntosh, A. R., Kovacevic, N., Lippe, S., Garrett, D. D., Grady, C. L., and Jirsa, V. (2010). The development of a noisy brain. Arch. Ital. Biol. 148, 323–337.
Misic, B., Vakorin, V. A., Paus, T., and McIntosh, A. R. (2011). Functional embedding predicts the variability of neural activity. Front. Syst. Neurosci. 5:90. doi: 10.3389/fnsys.2011.00090
Murtha, S., Cismaru, R., Waechter, R., and Chertkow, H. (2002). Increased variability accompanies frontal lobe damage in dementia. J. Int. Neuropsychol. Soc. 8, 360–372.
Naveh-Benjamin, M., Craik, F. I. M., Guez, J., and Kreuger, S. (2005). Divided attention in younger and older adults: effects of strategy and relatedness on memory performance and secondary task costs. J. Exp. Psychol. Learn. Mem. Cogn. 31, 520–537.
Rabbitt, P., Osman, P., Moore, B., and Stollery, B. (2001). There are stable individual differences in performance variability, both from moment to moment and from day to day. Q. J. Exp. Psychol. A 54A, 981–1003.
Ram, N., Rabbitt, P., Stollery, B., and Nesselroade, J. R. (2005). Cognitive performance inconsistency: intraindividual change and variability. Psychol. Aging 20, 623–633.
Ratcliff, R., Schmiedek, F., and McKoon, G. (2008). A diffusion model explanation of the worst performance rule for reaction time and IQ. Intelligence 36, 10–17.
Ratcliff, R., Thapar, A., and McKoon, G. (2006). Aging, practice, and perceptual tasks: a diffusion model analysis. Psychol. Aging 21, 353–371.
Schmiedek, F., Lövden, M., and Lindenberger, U. (2009). On the relation of mean reaction time and intraindividual reaction time variability. Psychol. Aging 24, 841–857.
Shammi, P., Bosman, E., and Stuss, D. T. (1998). Aging and variability in performance. Aging Neuropsychol. Cogn. 5, 1–13.
Shew, W. L., Yang, H., Petermann, T., Roy, R., and Plenz, D. (2009). Neuronal avalanches imply maximum dynamic range in cortical networks at criticality. J. Neurosci. 29, 15595–15600.
Shew, W. L., Yang, H., Yu, S., Roy, R., and Plenz, D. (2011). Information capacity and transmission are maximized in balanced cortical networks with neuronal avalanches. J. Neurosci. 31, 55–63.
Siegler, R. S. (1994). Cognitive variability: a key to understanding cognitive development. Curr. Dir. Psychol. Sci. 3, 1–5.
Spieler, D. H., Balota, D. A., and Faust, M. E. (2000). Levels of selective attention revealed through analyses of response time distributions. J. Exp. Psychol. Hum. Percept. Perform. 26, 506–526.
Stern, Y. (2002). What is cognitive reserve? Theory and research application of the reserve concept. J. Int. Neuropsychol. Soc. 8, 448–460.
Stuss, D. T., Murphy, K. J., Binns, M. A., and Alexander, M. P. (2003). Staying on the job: the frontal lobes control individual performance variability. Brain 126, 2363–2380.
Stuss, D. T., Pogue, J., Buckle, L., and Bondar, J. (1994). Characterization of stability of performance in patients with traumatic brain injury: variability and consistency on reaction time tests. Neuropsychology 8, 316–324.
Tamnes, C. K., Fjell, A. M., Westlye, L. T., Ostby, Y., and Walhovd, K. B. (2012). Becoming consistent: developmental reductions in intraindividual variability in reaction time are related to white matter integrity. J. Neurosci. 32, 972–982.
Tomporowski, P. D., and Tinsley, V. F. (1996). Effects of memory demand and motivation on sustained attention in young and older adults. Am. J. Psychol. 109, 187–204.
Tun, P. A., and Lachman, M. E. (2008). Age differences in reaction time and attention in a national telephone sample of adults: education, sex, and task complexity matter. Dev. Psychol. 44, 1421–1429.
Vakorin, V. A., Lippe, S., and McIntosh, A. R. (2011). Variability of brain signals processed locally transforms into higher connectivity with brain development. J. Neurosci. 31, 6405–6413.
West, R., Murphy, K. J., Armilio, M. L., Craik, F. I. M., and Stuss, D. T. (2002). Lapses of intention and performance variability reveal age-related increases in fluctuations of executive control. Brain Cogn. 49, 402–419.
Keywords: intraindividual variability, aging, reaction time, performance variability, feedback, cognitive reserve
Citation: Garrett DD, MacDonald SWS and Craik FIM (2012) Intraindividual reaction time variability is malleable: feedback- and education-related reductions in variability with age. Front. Hum. Neurosci. 6:101. doi: 10.3389/fnhum.2012.00101
Received: 23 February 2012; Accepted: 07 April 2012;
Published online: 01 May 2012.
Edited by:
Julia Karbach, Saarland University, GermanyReviewed by:
Nilam Ram, Pennsylvania State University, USADenis Gerstorf, Humboldt University Berlin, Germany
Copyright: © 2012 Garrett, MacDonald and Craik. This is an open-access article distributed under the terms of the Creative Commons Attribution Non Commercial License, which permits non-commercial use, distribution, and reproduction in other forums, provided the original authors and source are credited.
*Correspondence: Douglas D. Garrett, Max Planck Society-University College London Initiative for Computational Psychiatry and Aging Research (ICPAR); Center for Lifespan Psychology, Max Planck Institute for Human Development, Lentzeallee 94, 14195 Berlin, Germany. e-mail: garrett@mpib-berlin.mpg.de