- Department of Experimental Psychology, University of Oxford, Oxford, UK
Investigation into the neural and computational bases of decision-making has proceeded in two parallel but distinct streams. Perceptual decision-making (PDM) is concerned with how observers detect, discriminate, and categorize noisy sensory information. Economic decision-making (EDM) explores how options are selected on the basis of their reinforcement history. Traditionally, the sub-fields of PDM and EDM have employed different paradigms, proposed different mechanistic models, explored different brain regions, disagreed about whether decisions approach optimality. Nevertheless, we argue that there is a common framework for understanding decisions made in both tasks, under which an agent has to combine sensory information (what is the stimulus) with value information (what is it worth). We review computational models of the decision process typically used in PDM, based around the idea that decisions involve a serial integration of evidence, and assess their applicability to decisions between good and gambles. Subsequently, we consider the contribution of three key brain regions – the parietal cortex, the basal ganglia, and the orbitofrontal cortex (OFC) – to perceptual and EDM, with a focus on the mechanisms by which sensory and reward information are integrated during choice. We find that although the parietal cortex is often implicated in the integration of sensory evidence, there is evidence for its role in encoding the expected value of a decision. Similarly, although much research has emphasized the role of the striatum and OFC in value-guided choices, they may play an important role in categorization of perceptual information. In conclusion, we consider how findings from the two fields might be brought together, in order to move toward a general framework for understanding decision-making in humans and other primates.
Introduction
Over the past 10 years, there has been a resurgence of interest in the neural and computational mechanisms by which humans and other primates make decisions under uncertainty. This work has bridged multiple levels of description, with some researchers focusing on the contributions of individual neurons to decision-making, and others trying to map entire brain circuits for voluntary choice. Computational accounts have ranged from biophysically plausible neural network models to large-scale simulations in which the behavior of millions of neurons is captured in a single variable. Correspondingly, the techniques involved have included those focused on both local neuronal circuits, such as single-cell electrophysiology or microstimulation and global brain systems, such as functional neuroimaging, lesion studies, and pharmacological manipulations.
Curiously however, this research program has largely been carried out in two distinct but parallel streams. One stream, which is sometimes called “perceptual decision-making (PDM),” grew out of classical psychophysics, and is concerned with how humans choose an appropriate action during the detection, discrimination, or categorization of sensory information. The other stream, which we refer to as “economic decision-making (EDM),” has asked how humans choose among different options on the basis of their associated reinforcement history. To date, we would argue, researchers in either stream have been surprisingly reluctant to import concepts or approaches from the other. Instead, researchers interested in perceptual and economic choices have tended to use different classes of computational model, focused on distinct neural circuits, and have arrived at different conclusions about whether humans make good choices or not.
However, we would argue that an understanding of the computational neurobiology of voluntary choice would benefit from increased cross-fertilization between the literatures concerned with perceptual and economic decisions. It might be worth considering, for example, that all perceptual decisions are ultimately motivated by reward (or the avoidance of loss) whereas all economic decisions require perceptual appraisal of the alternatives on offer. Moreover, there is a common structure to virtually all decision-making tasks employed across the literature: an agent is required to identify one or more stimuli in a given sensory modality (what is it?), and then to select a response which will maximize the probability of positive feedback or reward (what is it worth?). In what follows, we will argue that despite the differences of approach between the two streams, one can conceive of the problem they seek to understand under one general conceptual framework, in which decisions are sensorimotor acts that depend on the integration of sensory evidence with information about reward value.
In this review, we summarize the work of computational, behavioral, and cognitive neuroscientists who have reached across the divide between the sub-fields of perceptual and EDM. Some researchers have considered, for example, how reward might influence sensory discrimination (Feng et al., 2009; Rorie et al., 2010; Serences and Saproo, 2010; Summerfield and Koechlin, 2010; Weil et al., 2010; Mulder et al., 2012), or how process models used to describe perceptual decisions might be applied in the economic domain (Basten et al., 2010; Philiastides et al., 2010; Hare et al., 2011b; Krajbich and Rangel, 2011; Krajbich et al., 2011; Hunt et al., 2012). In doing so, we emphasize a number of issues which we believe to be of key interest for researchers concerned with decision-making. In particular, we review work that has asked how perceptual decisions are biased by economic information, for example where the response options may have asymmetric costs and benefits. We also consider the mechanisms by which rewards (or informative feedback) might drive learning about sensorimotor acts. These considerations prompt a discussion about where in the primate brain information about the likely identity of a stimulus and its likely reinforcement value are combined. In conclusion, we aim to move toward a more general framework for understanding the neurobiology of decision-making, drawing upon approaches from the sub-fields of both perceptual and EDM.
Perceptual and Economic Decision-Making: Convergence and Divergence
It is beyond the scope of the current article to provide a comprehensive overview of the literatures concerned with perceptual and economic choice, and we refer the reader instead to a number of excellent summaries published in recent years (Gold and Shadlen, 2007; Heekeren et al., 2008; Kable and Glimcher, 2009; Rangel and Hare, 2010; Rushworth et al., 2011). Rather, here we aim to highlight the similarities and differences between the methods and approaches in the two fields.
Sources of Uncertainty
Perceptual decision-making is concerned with the mechanisms by which observers categorize sensory signals, and as such, tasks typically require observers to classify weak or noisy sensory information. For example, one influential paradigm called the “random-dot kinetogram” or RDK task (Britten et al., 1993) requires observers to classify the net direction of motion of a cloud of randomly moving dots. However, whilst the sensory information in these tasks is ambiguous, the reinforcement contingencies (i.e., which action leads to reward, given the identity of the stimulus) are usually clear and over-learned. Thus, it is the identity of the stimulus that is uncertain, not the value of its associated action. By contrast, EDM tasks tend to employ stimuli that are perceptually unambiguous, often in the visual domain. For example, in classic “multi-armed bandit” tasks, agents usually view two easily discriminable shapes or symbols, each associated with a distinct reward statistics (Sutton and Barto, 1998; Daw et al., 2006). However, whilst perceptual uncertainty on these tasks is negligible, the task is challenging because the reinforcement value associated with the two options may drift or jump unpredictably across the experiment (Behrens et al., 2007; Summerfield et al., 2011), or in different situations, because the agent has to choose between two or more assets whose value (learned prior to the experiment) is roughly comparable (Kable and Glimcher, 2007; Plassmann et al., 2007), and the value representations are themselves noisy. Thus, relative value of each stimulus and/or its associated action is uncertain, but its identity is known to the agent.
Computational Models
Because uncertainty in PDM tasks is owing to the identity of the stimulus itself, these experiments often take place in the “proficient” stage of task performance, where the response-reward contingencies have been either unambiguously instructed or learned through extensive training. Thus, the computational models that have been used to characterize performance have focused on the choice period itself, rather than on any reinforcement learning that occurs following feedback. One class of model that has attracted a great deal of recent interest is premised on the idea that choices depend on a serial sampling mechanism, in which evidence about the identity of the stimulus is collected and integrated until a criterial level of certainty is reached (Wald and Wolfowitz, 1948; Bogacz et al., 2006; Ratcliff and McKoon, 2008). Many variants of this model have been proposed (see Serial Sampling Models of PDM below), but they share a common advantage, namely, the ability to predict both choices and choice latencies (i.e., reaction times) in judgment tasks. A major theme of research into PDM is thus to understand the mental chronometry (i.e., the changing information processing over time) of the choice process.
Two main classes of computational model have informed the literature on EDM. One very successful class of model, that draws upon a rich literature from learning theory in experimental psychology (Rescorla and Wagner, 1972) and machine learning (Sutton and Barto, 1998), describes the mechanisms by which the value of stimuli or actions is learned (reinforcement learning or RL models). This model proposes that these values are updated according to how surprising an outcome is (a “prediction error”) scaled by a further parameter that controls the rate of learning. Models of the choice process in this field have tended to describe the weighting that agents give to different magnitudes or probabilities of reward. The other successful account, called Prospect Theory has been applied to decisions where the probabilistic information about the choice is not learned by feedback but explicitly instructed. Prospect Theory can account for proposed violations of rational economic behavior, including preference reversals, risk aversion, and susceptibility to framing effects, via appeal to non-linear weighting functions mapping objective probabilities and magnitudes of reward to their subjective, internal counterparts (Kahneman and Tversky, 1979). However, Prospect Theory describes human economic behavior without providing a normative framework for understanding choice, and offers no account of the processes that underpin decision-making. Similarly, in most reinforcement learning tasks, choices are typically modeled by assuming that agents simply choose the most valuable option (a “greedy” policy), or choose according to a sigmoidal “softmax” function that privileges the most valuable option whilst permitting some stochasticity. Below, we argue that the near-absence of process models in EDM is a major limitation to the current state of the field, and that the application of serial sampling models to economic choices may represent a fruitful avenue for future research.
Optimality
A related concern in both the fields of PDM and EDM is whether humans make good decisions or not. In EDM, the descriptive account offered by Prospect Theory catalogs the heuristics and biases that characterize human economic decisions, arriving at the conclusion that humans often make poor and irrational choices. In one classic example, it was shown that faced with an equivalent alternative, humans will favor the option to save 400/600 people from a fictitious disease, but will reject an offer to allow 200/600 people to fall ill, despite the mathematical equivalence of the two prospects (Tversky and Kahneman, 1981). Other examples abound in the behavioral economic literature (Kahneman et al., 1982). This contrasts sharply, however, with the approach taken in psychophysical investigations of perceptual choice, where a strong emphasis has been placed on the optimality of detection and categorization judgments. For example, humans integrate evidence from different sources or modalities according to its reliability, exactly as a statistically ideal observer should (Ashby and Gott, 1988; Ernst and Banks, 2002; Kording and Wolpert, 2004). Once again, however, the notion that agents are optimal for perceptual choice and suboptimal for economic choices may reflect a bias in the approach or emphasis of researchers in the two sub-fields, rather than a fundamental difference in the relevant computational mechanisms. For example, agents may approximate optimal behavior in multi-armed bandit problems (Behrens et al., 2007); on the other hand, sensory detection thresholds may typically be set too high, leading to overly conservative or poorly adjusted detection judgments (Maloney, 1991). Below, we consider the possibility that agents appear to be closer to optimal for perceptual choices mainly because we have a clearer notion of what is begin optimized in psychophysical judgment tasks (see Decision Optimality in PDM and EDM).
Neural Circuits
Researchers concerned with PDM and EDM share the goal of identifying a final common pathway for decisions, that is, a critical stage at which all decision-relevant information has been integrated, and options can be compared in a “common neural currency.” Nevertheless, researchers in the two fields have tended to pin their hopes on very different neural circuits. In PDM, where simple, over-learned sensorimotor tasks are a ubiquitous tool, the focus has been on dorsal stream cortical regions that receive inputs from the sensory cortices, but which contain at least some neurons which code information in the frame of reference of the response. For example, researchers using RDK stimuli in conjunction with a saccadic response have focused on a lateral parietal area that receives input from motion-sensitive extrastriate area MT, but which contains neurons coding for spatial targets of an eye movement (Roitman and Shadlen, 2002; Bennur and Gold, 2011). In other work, recordings have been made from frontal cortical zones with similar properties (Kim and Shadlen, 1999; de Lafuente and Romo, 2006). By contrast, in EDM, researchers have focused on structures such as the dopaminergic midbrain or orbitofrontal cortex (OFC), where neurons respond directly to the reinforcing properties of food or money (Schultz, 1986; Critchley and Rolls, 1996; O’Doherty et al., 2001), and on the structures such as the striatum or anterior cortex, where neuronal responses scale with reward prediction errors (Schultz et al., 1997; Matsumoto et al., 2007). These predilections might seem a natural reflection of the different sources of uncertainty typically manipulated in PDM and EDM tasks (about the identity of the stimulus, presumably determined in cortical circuits; and about the value of the stimulus, presumably determined in subcortical and limbic circuits and interconnected structures). However, there may also be strong reasons to suspect the involvement of cortical regions, such as the parietal cortex, in representing the expected value of a choice (Sugrue et al., 2004), as well as evidence that the OFC and BG play an important role in discrimination and categorization judgments even in the absence of explicit reward (Eacott and Gaffan, 1991; Ashby et al., 2010). Below, we review this evidence, with a view to providing an integrated account of the neural systems underlying decision-making in primates.
In summary, thus, researchers interested in perceptual and economic choices have made different assumptions, used different approaches, focused on different models and neural circuits, and, not surprisingly, drawn different conclusions. However, we can conceive of the decisions made in both perceptual and economic choice tasks under a common framework – the agent must (i) disambiguate one or more stimuli, and (ii) estimate their worth. Whilst we know much from the PDM and EDM literatures about the neural and computational mechanisms underlying these two processes separately, we know very little about how perceptual and reward information is integrated in the primate brain. In other words, we have as yet no general understanding of the mechanisms by which primates make decisions.
Computational Approaches
A renewed interest in the computational mechanisms underlying decision-making has enriched the field in recent years. In this section, we focus on the sequential sampling framework, the most prominent computational theory in PDM. Crucially, however, we also point to successful applications of serial sampling models in accounting for economic choices, and argue that such models may be promising candidates for inclusion in a unified theory of choice. We start this section with a general introduction on sequential sampling models (SSMs) of PDM, and motivate their use in theorizing value-guided behavior. We continue our discussion by contemplating computational accounts of how decision-relevant information is fed into the decision process. Finally, we attempt to provide a mechanistic overview of the decision process with regards to optimality.
Origins of the Sequential Sampling Framework
Two prominent frameworks have been proposed to account for the psychology of PDM: signal detection theory (SDT; Green and Swets, 1966) and SSMs (Laming, 1968; Ratcliff, 1978; Vickers, 1979). While SDT assumes that a decision is settled on the basis of a single sample of information, SSMs suggest that multiple samples of evidence are integrated across time up to a critical level of certainty. Because SSMs are dynamic, they have predictive power unavailable to static SDT accounts, allowing us to model not only choice behavior but also the full time-course of the deliberation process. Theoretical research in the field has explored these models’ relation to statistically optimal inference (Bogacz et al., 2006; Bogacz, 2007), and used both behavioral and neural recordings to validate and compare between models (Ratcliff et al., 2007; Kiani et al., 2008; Ditterich, 2010; Tsetsos et al., 2011). On the contrary, in EDM the focus has not been placed on developing explanatory mechanisms of the deliberative process but on ad hoc, descriptive modifications of the normative theory (Von Neumann and Morgenstern, 1944) in order to account for choice biases and apparently paradoxical behavior (Kahneman and Tversky, 1984; Gilovich et al., 2002). The absence of process models in EDM stands in sharp contrast with the state-of-the-art in PDM and poses a serious challenge for the development of a unified theory of choice. However, the recent development of dynamical models of preference formation, which build upon the tradition of SSMS, promises to establish a theoretical link between PDM and EDM (Busemeyer and Townsend, 1993; Usher and McClelland, 2004; Johnson and Busemeyer, 2005; Otter et al., 2008). We next provide an overview of serial sampling models of PDM and subsequently motivate their use in EDM.
Serial sampling models of PDM
In mathematical statistics, the optimal solution to the problem of disambiguating two competing hypotheses given a series of noisy information is provided by the sequential probability ratio test (Wald, 1947; Gold and Shadlen, 2001). For a fixed error rate, SPRT uses the minimum possible amount of evidence in order to generate a categorical decision (Wald and Wolfowitz, 1948). This is achieved by updating at each sampling step the log likelihood ratio of the evidence given the two alternative hypotheses, until it exceeds a pre-defined threshold, at which point the process is terminated and a decision occurs favoring the hypothesis with the larger likelihood. This simple, optimal process explains fundamental aspects of human choices, such as the speed-accuracy trade-off (SAT), whereby higher decision thresholds, and thus more prolonged sampling, leads to more accurate choices (Johnson, 1939). However, although the SPRT has proved able to capture many aspects of human binary choices, it assumes that the observer has perfect prior knowledge of the distributions of evidence. Thus, psychological models of PDM have attempted to approximate the sequential sampling process in more psychologically plausible and computationally feasible ways.
Two different broad classes of SSM of PDM have been proposed in the literature. The first class encompasses accumulator or race models (Figure 1A) that assume the independent integration of pieces of sensory evidence toward a common response criterion, analogous to a race among athletes running on independent tracks (Vickers, 1979; Townsend and Ashby, 1983; Brown and Heathcote, 2008). This mechanism contrasts with that of the diffusion model (Figure 1B), in which the net difference in evidence favoring either option is accumulated (Laming, 1968; Ratcliff, 1978; Ratcliff and McKoon, 2008). Thus, we can differentiate among PDM models according to whether the input to the decision process is an absolute or a relative signal (see Decision Input). A third class of PDM models has also emerged, building on mechanisms of existing mathematical models but also on principles of neural computation, such as the leaky competing accumulator model or LCA (Usher and McClelland, 2001) and the Wang model (Wang, 2002; Figure 1C). These models share with the race framework the idea that the absolute evidence for each alternative is integrated. However, similar to the diffusion, they induce competition among the alternative hypotheses in the form of lateral inhibition at the response level (see Decision Processes and their Relation to Optimality).
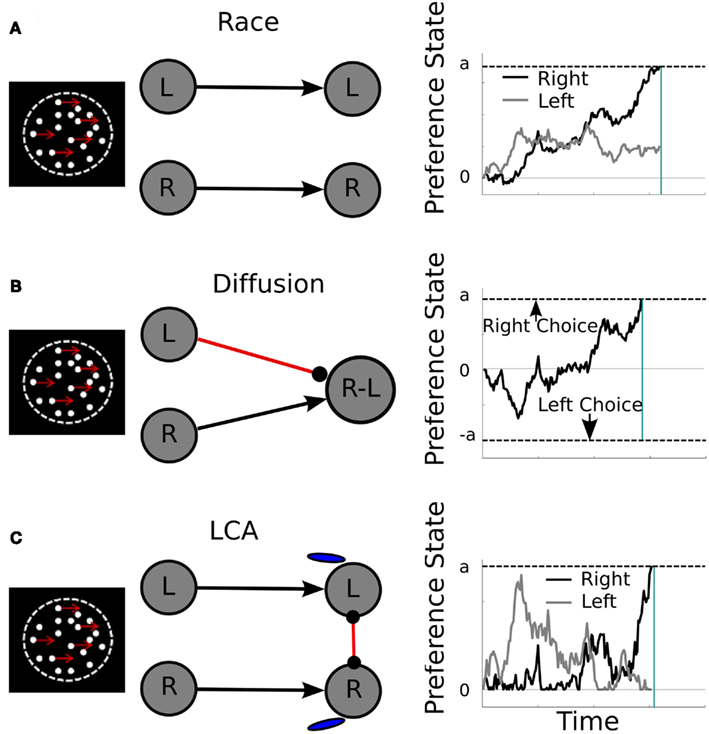
Figure 1. Computational architecture (middle panels) and representative activation trajectories (right panels) of the race (A), diffusion (B), and LCA (C) models in a motion discrimination task (left panels). Middle panels: black lines with arrowheads represent excitatory connections, and red lines terminating in filled circles correspond to inhibitory connections. Gray circles represent units encoding left (L) and right (R) responses or their difference (R-L). Blue “tears” stand for activation leakage. Right panels: representative activation over time (x-axis) in L (gray) and R (black) units, or a unit encoding their difference of activation (black, part B only). Bounds on activation level, at which a choice is initiated, are indicated by the dashed line signaled with lowercase letter a (or – a, part B only). Vertical cyan line, estimated reaction time for the representative trial. In the race model, the two options race independently toward a common upper decision boundary. In the diffusion model, choice is determined by which boundary is first reached (upper or lower). In the LCA model, the two options compete against each other toward a common response criterion.
Serial sampling models of EDM
Most PDM tasks require the observer to categorize noisy evidence presented in series. Serial sampling thus provides a natural mechanism for optimizing decisions, by averaging out the noise-driven fluctuations over time and steadily enhancing the signal-to-noise ratio. In EDM tasks, however, stimuli tend to be static and perceptually unambiguous. What benefit might be conferred by serial sampling in EDM tasks, and what might be accumulated? In EDM tasks, uncertainty is derived from variability in internal information about the expected value of each option. A growing consensus indicates that dedicating more processing time to an economic choice confers similar benefits as in PDM tasks, as if the participants were “accumulating” internal information about economic value, rather than averaging over external noise. For example, subjective values may be sampled stochastically from long-term memory, allowing a subjective value representation to be actively constructed on the basis of past experience (Sigman and Dehaene, 2005; Milosavljevic et al., 2010). These samples could be defined either with respect to the immediate context (i.e., how good is an option compared to the other alternatives), or in relation to memory contents (how good is an option relative to other similar options encountered in the past; Stewart et al., 2006). Whether this covert sampling process is governed by similar principles and mechanisms to the mental process that underlies PDM remains an open question.
One important model, called Decision Field Theory (DFT; Busemeyer and Townsend, 1993; Johnson and Busemeyer, 2005) argues that sampling of competing options is biased in part by expected reward, so that more valuable sources are sampled more frequently. In DFT, different attributes of a percept or good are sampled in turn according to where attention is oriented, such the decision variable (DV) corresponds to the attention-weighted sum of the sampled information. Attention might be oriented stochastically, or directed preferentially to a subset of the information, such as the most valuable option. DFT is able to explain preference reversals in economic behavior, such as the Allais paradox (Johnson and Busemeyer, 2005), and contextual effects in multi-attribute choice (Roe et al., 2001). A related account, Decision by Sampling (DbS), proposes that utilities are constructed afresh through sampling attribute values from both the immediate context and the long-term memory, and considering the rank of the target value within the current set of samples (Stewart et al., 2006). By assuming that the contents of memory reflect the real world distribution of decision-relevant quantities, DbS explains a range of biases such as aversion to losses, overestimation of small probabilities and underestimation of large probabilities, and hyperbolic temporal discounting. These models contrast with more descriptive accounts such as Prospect Theory (Kahneman and Tversky, 1979), that simply assume that these principles are primitives of decision behavior, rather than explaining how they occur in a plausible computational framework.
SSM summary
The serial sampling approach has been applied with success to PDM tasks where evidence is noisy and sequential. One might argue that this approach is tailored to the serial nature of the PDM tasks. However, the recent success of SSMs to explain classic puzzles and paradoxes in EDM suggests that they may offer a domain-general mechanism by which uncertainty can be reduced in decision-making, irrespective of whether that uncertainty arises from the sensory or value representation. In the next subsection we discuss what information might serve as input to the decision process, and we then overview computational accounts of how this process might work.
Decision Input
In the PDM literature a major controversy is whether decisions are settled on the basis of the relative or the absolute amount of the accumulated evidence. Race models (Figure 1A), which assume independence among the accumulated tallies of evidence, offer prima facie neurobiological plausibility, and have the virtue of being easy to extend to decisions between more than two alternatives (Bogacz et al., 2007; Furman and Wang, 2008; Tsetsos et al., 2011). By contrast, accounts based on the SPRT, such as the diffusion model (Figure 1B), offer closer approximations to statistically optimal choice behavior, and are also supported by neurophysiological evidence (see Orbitofrontal Cortex below).
Paradoxically, the same question has provoked a major debate in the field of behavioral economics over the last 60 years, but with converse claims about optimality. There, expected utility theory, the cornerstone of theories of rational choice, argues that utilities are derived in absolute terms, independent of the context, whereas relativist theories appear to provide a better empirical description of human choice behavior (see Vlaev et al., 2011 for a review). Early psychological theories proposed that the normative expected utility is modified in several ways during choice. For example, Prospect Theory assumes that values are calculated with respect to a reference point, or status quo, and introduces non-linearities to value and probability functions. Crucially however, these theories conserve the notion that the value of an option is independent of other available options (Kahneman and Tversky, 1979; Keeney and Raiffa, 1993). By contrast, relative theories of decision-making propose that option values are computed afresh in the context of each decision (Tversky and Simonson, 1993; Parducci and Fabre, 1995; Gonzalez-Vallejo, 2002). Thus, the value assigned to an option reflects not only its properties but also those of the other available alternatives. On the empirical front, context effects such as preference reversal (Simonson, 1989; Roe et al., 2001; Johnson and Busemeyer, 2005) and prospect relativity (Stewart et al., 2003) have supported the notion of relative valuation. Consider for example a hypothetical choice between two laptop computers; one is expensive and very light (A) while the other one is heavy and cheaper (B). Paradoxically, an initial tendency to favor B can be reversed by the appearance of a third option, (C) which is similar in weight to A but more expensive. This asymmetric dominance effect (Huber et al., 1982; Simonson, 1989) is representative of a class of contextual preference reversals that pose a serious challenge to independent valuation theories, which would predict that the valuation of A and B is a function of their attribute values only and that irrelevant alternatives, like option C, should not affect this valuation. This question of whether decision-relevant brain regions encode value in an absolute (“menu-invariant”) or relative framework is a major concern in neuroscientific studies of EDM (see Orbitofrontal Cortex and Absolute Stimulus Value below).
Therefore, a central question for both literatures is whether the input to the decision process is an absolute or a relative quantity. Interestingly, debate has focused on how the information is transformed before being processed by the decision mechanism, under the assumption that all available information is utilized. An alternative approach posits that the sampling process is biased by selective attention or endogenous factors (e.g., preference states). In what follows we review the literature, drawing attention to the distinction between unbiased and biased sampling of information.
Biased sampling
In most PDM tasks, choices are typically made on the basis of a single stimulus feature or dimension, and observers are instructed to hold fixation steady (but see Siegel et al., 2008 for an exception). It is thus implicitly assumed that fluctuations in visual attention are controlled for, such that information is sampled evenly for all alternatives. However, choices are known to be biased by attentional factors, such as where observers place their gaze (Russo and Rosen, 1975; Russo and Leclerc, 1994; Payne, 1976; Glockner and Herbold, 2011). This phenomenon was investigated recently on an economic decision task, in which eye movements were measured whilst hungry observers chosen between two food items displayed visually on either side of the screen (Krajbich et al., 2011). The authors compared the ability of variants of the drift-diffusion model (DDM) to account for choices and choice latencies made in the experiment, reporting that the winning model was one in which the gain of accumulation was modulated multiplicatively by the value of the currently fixated item. A follow-up study demonstrated comparable effects for trinary choices, using a multi-alternative version of the DDM (Krajbich and Rangel, 2011). The modeling aspects of the work demonstrate the applicability of serial sampling models to EDM, and highlight the importance of recognizing the capacity-limited nature of the choice process, something which has been notably absent particularly from PDM models, which prefer to emphasize that decisions are made in a strictly optimal fashion (Bogacz et al., 2006; Bogacz, 2007; van Ravenzwaaij et al., 2012). The specific interpretation of the data offered by the authors, that economic preferences depend on a stochastic sampling of the (external) world via attention, is intriguing, but another possibility is that the sampling process itself is biased by the observers’ preferences (Svenson and Benthorn, 1992; Jonas et al., 2001; Doll et al., 2011; Le Mens and Denrell, 2011). For example if the decision maker is leaning toward one alternative, she might sample from it more often, seeking for confirmatory evidence. This would be consistent with the more general observation that agents seek to confirm, rather than to disconfirm, hypotheses that they already entertain, and would suggest that momentary preference states could strongly bias the input to the evidence accumulation process in a bidirectional fashion (Holyoak and Simon, 1999; Shimojo et al., 2003).
In EDM problems whose structure is more complex, with each option varying along several dimensions, the selective allocation of resources to a subset of the choice information is more imperative. Empirical research in this area has attempted to track the regularities in information acquisition and identify what strategies people might use in order to compare options (e.g., within-option evaluation across all attributes, or across options evaluation attribute by attribute; Russo and Rosen, 1975; Dhar et al., 2000; Fellows, 2006; Glockner and Herbold, 2011). One possibility, attributable to DFT (Roe et al., 2001) is that people switch their attentional focus back and forth from attribute to attribute (Tversky, 1972). The subjective attribute values for each option are accumulated across time and a decision is initiated once a threshold is breached. This proposal is appealing as it provides a generic framework of integration across attributes, even if these attributes are incommensurate and thus cannot be represented in a “common currency.” For example, DFT has applied a similar attention switching approach to address how people’s decisions about sensory information are biased by rewards, under the assumption that observers switch their attention between sensory evidence and information about the likely payoffs (Diederich and Busemeyer, 2006; see Decision Optimality in PDM and EDM). Finally, another plausible case where biased sampling might be critical is when people are faced with multi-alternative problems either in PDM or EDM tasks (Krajbich and Rangel, 2011). These problems might be broken down into a multitude of binary comparisons which can be based on the similarity of the items in the decision space (Russo and Rosen, 1975). Further empirical work is needed to identify how people allocate their attention when confronted with a large number of options, for example when dining at a restaurant with an extensive menu.
Decision Processes and Their Relation to Optimality
We describe above the current debate regarding what information consists the input to the decision process. In this subsection we consider how this process works, namely what mechanisms are in play for transforming input into decisions. A central question in both PDM and EDM is whether the decision mechanism reflects an optimized process. However, while in PDM optimality is defined in statistical terms (optimization of SAT) in EDM it is defined as the maximization of the reward rate. In the next section we review recent attempts that examine how these two different notions of optimality are combined when making decision; and what aspects of the choice mechanisms are responsible for suboptimal biases. We next turn to another important aspect of the decision mechanism, temporal weighting, and its relation to choice optimality. Temporal weighting is often considered to be a suboptimal property leading to order effects and biases. We argue that in special cases overweighting early or late information might result in better decisions. Finally we consider response inhibition as being responsible for conflict in difficult choice problems, and we discuss the computational and descriptive merits of this mechanism.
Decision optimality in PDM and EDM
Humans’ choice behavior obeys a simple principle by which the advantages of speed and accuracy are traded off against one another (Johnson, 1939; Wickelgren, 1977; Bogacz et al., 2010). Within the sequentially sampling framework this SAT is controlled by a single parameter, the height of the decision bound (Laming, 1968); a low bound implies that decisions will be fast but overly influenced by noise fluctuations while a high bound produces accurate but delayed responses (Figure 2A). For fixed rewards rates there exists a specific response criterion value that optimizes this trade-off, and most PDM work has focused on identifying this bound or testing whether human behavior respects it (Bogacz et al., 2006). However, in both the real world and the lab, responses are often associated with unstable, asymmetric rewards, raising the question of how people maximize simultaneously their accuracy and the reward rate. For example, a radiographer examining medical scans might have to impose alternately a liberal or conservative criterion for identifying an atypicality, such as an incipient tumor, depending on the relative costs and benefits of missing the first signs of disease, or inconveniencing the patient with more tests (Diederich and Busemeyer, 2006; Feng et al., 2009; Summerfield and Koechlin, 2010; Gao et al., 2011). In such cases, participants exhibit a bias toward the high-reward option (Figures 2B,C), but the computational mechanisms by which this occurs is a topic of ongoing research. One possibility is that a higher reward changes the way the sensory evidence for the high-reward hypothesis is perceived, by increasing the drift rate (i.e., slope) of the corresponding accumulator (Figure 2B). An alternative hypothesis is that the way the input is processed remains unaffected but it is the starting point of the accumulation for the high-reward hypothesis that is shifted closer to the decision bound (Figure 2C). Specific behavioral patterns observed in humans and other primates, such as fast errors when the high-reward option was incorrect, could be captured only by the latter hypothesis (Summerfield and Koechlin, 2010; Gao et al., 2011). This shift of the starting point, prior to the onset of evidence, is independent of the decision bound and the SAT of the observer. Other accounts emphasize that human participants try to find a compromise between the perceived benefits of accuracy and overt reward (Bohil and Maddox, 2003; Simen et al., 2009).
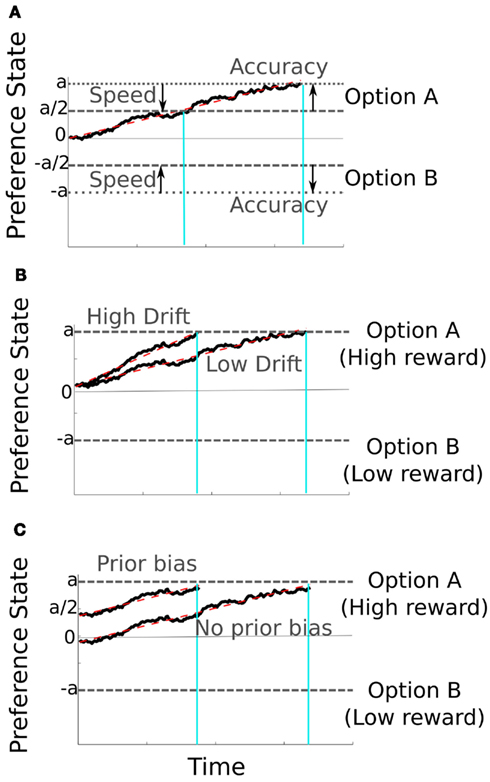
Figure 2. Representative activation trajectories from the diffusion model with noise (black traces) and without noise (dashed red traces). Decision bounds are signaled by the dashed line marked with lowercase letter a or −a). In part (A), the speed-accuracy trade-off is determined by the height of the response boundary with lower boundaries resulting in faster and less accurate decisions (and vice versa for higher boundaries). (B,C) Choice can be biased by the presence of asymmetric rewards. This is achieved either by increasing the rate of evidence accumulation (high drift trajectory in (B) for the high-reward option or by increasing its initial activation, prior to the onset of accumulation (C). Vertical cyan lines show RTs for representative trials under conditions where speed or accuracy are emphasized (A) or where decisions are biased by reward (B,C).
Thus, although PDM tasks have emphasized the optimization of accuracy, observers are swayed by factors that change the underlying reward rate (Summerfield and Koechlin, 2010; Gao et al., 2011). In EDM, optimality has tended to refer to the maximization of reward within the timeframe of the experiment, a tradition owing to neoclassical economics, where the agent is fully informed about all available alternatives and her behavior is rational when it maximizes reward rate. Failure to maximize rewards is typically considered to be irrational. In real world, however, information about the environment is not provided but must be actively sampled, leading to a trade-off between exploiting current resources and exploring new alternatives. Thus, although any organism that optimizes its fitness should jointly maximize reward and information, traditional interpretation of laboratory findings failed to consider optimality in this broader perspective. Although phenomena such as hyperbolic delay-discounting (Loewenstein and Thaler, 1989) and loss-aversion (Tversky and Kahneman, 1991) are trademarks of irrational behavior within behavioral economics, there may be circumstances in the wild where it is optimal to demonstrate such behavior – for example, losses can have fatal consequences for an organism, whereas gains may be short-lived. These concerns have been addressed in EDM by the research program of ecological rationality highlighting that choice optimality should not be assessed independently of the environmental structure and the way it influences the tradeoff between information and reward acquisition (Oaksford and Chater, 1996; Gigerenzer and Selten, 2001; Shanks et al., 2002; Behrens et al., 2007).
Temporal weighting
When people make decisions on the basis of sequential evidence, they often weigh information differentially according to its position in the sequence. For example, a judge may arrive at a hasty conclusion on the basis of the first witness (primacy bias), or a voter may judge a politician on his or her most recent debating performance (recency bias). Such order effects have been encountered in both PDM (Usher and McClelland, 2001; Kiani et al., 2008) and EDM tasks (Hogarth and Einhorn, 1992; Newell et al., 2009). The PDM literature has focused on mechanistic models where the temporal weighting of information emerges from the dynamics of evidence integration (Usher and McClelland, 2001). On the other hand, in EDM order effects have been captured mostly in a descriptive fashion, with different weights being assigned to different pieces of information (Anderson, 1981; Hogarth and Einhorn, 1992). It is important for decisions theorists to clarify what aspects of the generic (domain-independent) decision mechanism generate order effects. An appealing possibility has been put forward in biologically inspired models of PDM. There, depending on different value parameters the decision mechanism can overweight (i.e., attractor dynamics) or downweight early information (i.e., leaky integration, see also (Bogacz et al., 2007) for an extensive discussion).
What is the merit of weighing information differently at different times? Applying stronger weights to early information (attractor dynamics and primacy) is a useful mechanism that prevents endless procrastination when the information is weak or ambivalent (see also next subsection). In these cases the choice will be determined by random noise fluctuations early on, ensuring that the decision maker does not engage in excessively prolonged deliberation (Usher and McClelland, 2001). On the other hand, overweighting late information (recency) is useful when the sensory environment is volatile, because forgetting early information and emphasizing on the latest status of the world results in faster adaptation to changes that occur to the underlying statistical structure of the environment. Thus, whereas biased temporal weighting of information (either perceptual or economical) might appear suboptimal from the pure perspective of accuracy maximization, it confers benefits on reward-maximization if the decisions are challenging or if the environment is unstable.
Conflict and response competition
Choosing among two alternatives that are similar in terms of value or sensory evidence will result in response conflict, longer decision times (Laming, 1968; Ratcliff, 1978; Usher and McClelland, 2001), and less confidence associated with the final decision (Vickers, 1979; Pleskac and Busemeyer, 2010). The prolongation of deliberation in such cases might occur because the decision bound is raised to allow a clear winner to emerge among closely matched alternatives. However, adjusting the bound to the decision input requires either a priori knowledge about the difficulty of decision problem or the online adjustment of the criterion as the input unfolds. A more plausible way to produce longer decision times for more difficult problems is by introducing competition between the alternatives. In PDM this competition can be incorporated in two ways: in the diffusion model it takes place at the input level (section Decision Input and Figure 1B), while in accumulator models where the decision inputs are assumed to be independent (like in race models, Figure 1A), competitive interactions are achieved via lateral inhibition at the response level (Usher and McClelland, 2001; Wang, 2002), as illustrated in Figure 2C. Response inhibition in PDM brings some computational advantages in multi-alternative problems, where the activation in favor of poor options will be early suppressed and the decision process will continue evaluating only the strong or informative options (Bogacz et al., 2007). Additionally, response inhibition results in attractor dynamics (discussed in Temporal Weighting) which can facilitate resolving difficult decisions problems within reasonable time (Wang, 2002; Bogacz et al., 2007).
In EDM, conflict is captured in relative valuation models of preference by assuming that the input is transformed according to the value of its rivals (similar to the PDM diffusion, see also Biased Sampling). Typically the actual integration of the transformed input does not involve competitive interactions among alternatives. One exception is encountered in SSM of multi-attribute decision-making, like DFT (Roe et al., 2001) and LCA for value-based choice (Usher and McClelland, 2004), where it is assumed that different alternatives compete via response inhibition. It is noteworthy that in DFT a type of local, distance-dependent response inhibition is the key mechanism that explains a series of preference reversal effects (e.g., the asymmetric dominance effect, see Decision Input for an example). According to this account, alternatives that are similar to each other in the decision space compete more strongly while dissimilar alternatives do not interact with each other [see also (Tsetsos et al., 2010) and (Hotaling et al., 2010) for discussion of this mechanism].
Computational Models: Summary
PDM models assume that choice is the result of the accumulation of sequentially sampled sensory evidence. By contrast, EDM models have sought to define deviations from rationality but said little about the underlying mental process. The advent of process models of economic preference that capitalize on the serial sampling property of PDM, promises to provide an improved and mechanistic understanding of EDM (see Serial sampling models of EDM). In the preceding section we provided an overview of currently debated mechanistic aspects of PDM and EDM. We first reviewed how information is transformed before being processed by the decision mechanism (relative vs. absolute input, see Decision Input), highlighting also the possibility that these quantities might be actively sampled, subject to exogenous (e.g., visual attention) or endogenous (e.g., preference states) biases. We then discussed how the relevant input is transformed into a decision (see Decision Processes and their Relation to Optimality). In parallel to describing specific computational elements of choice (e.g., temporal weighting, response competition) we also discussed their relation to optimality. Although traditional views state that EDM is suboptimal while PDM optimal, we propose that this contradiction may have arisen because each literature has defined optimality in a different way.
Neural Approaches
A number of different brain regions have been proposed as key components of the circuit underlying simple decisions about visual stimuli. In the primate, three of the clearest candidates are (i) dorsal stream cortical circuits, such as the parietal and premotor cortices, (ii) the striatum and related circuitry of the basal ganglia, and (iii) the medial and lateral OFC. Other regions, such as the anterior cingulate cortex (ACC) and prefrontal cortex (PFC) clearly play important roles in decision-making too; for example, the ACC may be important for learning the value of actions (Rushworth and Behrens, 2008), and the PFC for integrating information over multiple time scales in the service of action selection (Koechlin and Summerfield, 2007). However, in the interests of brevity, we do not consider these in detail, referring the reader instead to other reviews that have considered these regions more comprehensively (Summerfield and Koechlin, 2009; Rushworth et al., 2011). Below, we consider the role of parietal/premotor, basal ganglia, and orbitofrontal structures in the decision process, with a specific focus on how each region might contribute to the processing and integration of perception and reward.
Parietal Cortex
The parietal cortex has long been implicated in the mechanisms by which sensation is converted to action, within initial debates concerning whether parietal neurons encode spatial information in the frame of reference of the stimulus or the response (Colby and Goldberg, 1999). Patients with unilateral lesions of the parietal cortex fail to orient saccades or other actions to the contralesional side of space (Robertson and Halligan, 1999), and bilateral damage provokes an inability to combine information from multiple spatial locations, for example to permit accurate judgments of similarity or dissimilarity (Friedman-Hill et al., 1995). Prominent theories of the parietal cortex suggest that it combines information across visual features (Treisman and Gelade, 1980) to generate a map of the relative salience of different locations of external space (Gottlieb, 2007). However, the parietal cortex also seems to be important for combining information across time. This is clear from neuropsychological studies, in which recently encoded spatial information is rapidly lost (Husain et al., 2001), and from neuroimaging studies which highlight increased in parietal blood-oxygen (BOLD) signals during visual short-term memory maintenance, for example in studies requiring detection of change in sequential arrays (Xu and Chun, 2006). Integration of information across a cluttered visual scene is facilitated by repeated sampling of the scene with saccadic eye movements, whose generation depend on dedicated regions of the parietal cortex (Gottlieb and Balan, 2010).
Parietal cortex and evidence integration
However, it is single-cell research conducted over the past ten years that has generated the most prominent evidence in favor of the idea that parietal neurons act as cortical integrators, and that has emphasized a role in PDM. During viewing of noisy stimulus such as an RDK, neurons in lateral intraparietal area LIP whose receptive fields (RFs) overlap with one of two saccadic choice targets exhibit firing rates that accelerate with a gain proportional to level of evidence (motion coherence) in the stimulus favoring a response at that target (Roitman and Shadlen, 2002). Initial investigations were at pains to demonstrate that activity in these cells was not a mere reflection of the sensory input, or the motor response (Gold and Shadlen, 2007). For example, the signal grows during constant stimulation, persists after the stimulus has been extinguished, and deviates from zero even when there is no motion signal in the stimulus (0% coherence trials). Similarly, the parietal activity does not predict the motor parameters of the eventual response (e.g., saccadic latency, velocity, or precision), suggesting that it is not a mere motor preparatory signal. However, more recent work in which sensory and oculomotor codes are dissociated demonstrated has demonstrated that LIP responses are more heterogenous, with some neurons responding to the motion direction, and others to the saccadic choice (Bennur and Gold, 2011). Nevertheless, at least some parietal neurons encode decision-relevant information it the frame of reference of the selected action.
One interpretation of this stereotyped increase in firing rate during perceptual judgment is that unlike earlier visual regions that encode the instantaneous sensory information, parietal neurons represent information that is integrated across a temporal window extending for many hundreds of milliseconds. The integration of serial samples of evidence is critical to optimizing a decision process, because repeated sampling of a noisy stimulus enhances the precision of the estimated information in well-described mathematical fashion. It is this intuition that informs serial sampling models of the decision process described above, and prompted the suggestion that parietal neurons might implement the accumulation-to-bound process that is known to describe decisions and decision latencies on a wide variety of perceptual choice tasks. Indeed, after an initial burst, the firing rates of neurons whose RFs overlap with the alternative, disfavored target tend to decrease with a gain proportional to the motion coherence, seemingly favoring one class of serial sampling models over all others – the DDM, in which accumulators are coupled by mutual inhibition, such that the DV represents a scalar quantity corresponding to the relative evidence in favor of either choice (see Serial Sampling Models of PDM; see though (Huk and Shadlen, 2005) p.3027 for a discussion on the possibility of attractor models (Wang, 2002 to better explain LIP responses). Moreover, response-locked analyses show that the parietal activity drops off sharply about 70 ms prior to saccade initiation, at a criterial firing rate that does not depend on the level of information in the stimulus. This satisfies another important prediction of most serial sampling models, namely that a decision is made when a criterial evidence level is reached. This work has generated a great deal of excitement, and prompted the claim that the parietal cortex plays a key role in the decision processes that underlie sensorimotor control, by integrating evidence up to a choice threshold, at which point an appropriate response is generated (Gold and Shadlen, 2007).
Cortico-cortical plasticity and reward-guided learning
One question that has received less attention, however, is how the reinforcement value of the options is integrated into the DV during perceptual choice tasks such as motion discrimination. In the experiments described above, the monkey is working to receive a liquid reward following each correct saccadic movement, but recordings are typically made during proficient performance of the task, where the monkey has been trained on many thousands of trials over which the reward contingencies have remained unchanged. Recall that most of the relevant parietal neurons encode the information in the frame of reference of the choice that will eventually be made, even when this is dissociated from the sensory information in the stimulus. In other words, the strength of synapses linking the visual and parietal cortical representations must be adjusted during training to encode information about the likely reinforcement value of each sensorimotor pairing. Recent work has shown that the improvement in perceptual performance on psychophysical tasks such as the random-dot kinetogram is better explained by plastic changes in the parietal and prefrontal regions than those in sensory cortex (Law and Gold, 2008; Kahnt et al., 2011), and that the trajectory of learning is well-described by a reinforcement learning scheme in which visuo-parietal connection weights are gradually updated according to a prediction error signal (Law and Gold, 2009). This leads to a steeper gain of accumulation after repeated feedback-mediated learning, and consequently, more sensitive perceptual judgments. However, it remains unknown whether this prediction error is computed at the cortex, or is dependent on processing of the subsequent reward in subcortical regions (Kahnt et al., 2011). One study suggests that during perceptual categorization of options that can change unpredictably, requiring constant tracking of category statistics, both cortical and subcortical mechanisms are employed. However, systems based in the striatum and medial PFC underlie optimal decisions in stable environments, whereas the dorsolateral PFC mediates decisions in fast-changing situations, where it is useful to base decisions on recently buffered information (Summerfield et al., 2011). In general, however, these mechanisms have been explored in considerably less detail for perceptual than for economic choices.
Parietal cortex and perceptual decisions biased by reward
Perceptual learning experiments chart the gradual improvement to performance that comes with extensive training and feedback. However, the costs and benefits associated with different types of perceptual error can sometimes change rapidly and unpredictably, on the basis of instructions or other contextual factors. Thus, another line of research has attempted to characterize the neural and computational mechanisms by which perceptual decisions are biased by instructions or cues that signal the relative outcomes associated with each response. As outlined above, serial sampling models in which asymmetric rewards bias the starting point of the accumulation process – an additive, a priori bias in favor of the more valuable response – fit observers’ performance better than models in which the bias influences accumulation rate in a multiplicative fashion (Whiteley and Sahani, 2008; Feng et al., 2009; Pleger et al., 2009; Simen et al., 2009; Summerfield and Koechlin, 2010; Mulder et al., 2012). Correspondingly, there is evidence from single-cell recordings that the responses of parietal neurons reflect an outcome-related bias as an additive increase in firing at or before the moment of stimulus onset (Platt and Glimcher, 1999; Rorie et al., 2010). This occurs during both challenging perceptual discriminations, for example of the RDK stimulus (Rorie et al., 2010), as well as during choices based on perceptually conspicuous information (Platt and Glimcher, 1999). Similar results have also been observed in the premotor cortex. Consistent with this finding, model-based fMRI studies have revealed that an additive reward-mediated bias is correlated with the BOLD signal in the lateral parietal cortex during signal detection (Fleming et al., 2010; Summerfield and Koechlin, 2010).
Relatedly, the responses of parietal neurons track the evolving value of an option in n-armed bandit and other economic choice tasks in which rewards are learned by feedback (Dorris and Glimcher, 2004; Sugrue et al., 2004, 2005). For example, LIP signals during a value-based choice task are well-described by a probabilistic choice model (akin to an RL model) that engages in a leaky integration of information across recent trials, producing classical “matching” behavior typically observed in bandit and reversal learning tasks (Sugrue et al., 2004). More recently, LIP neurons have been observed to correlate both with the delay-discounted value of an offer shortly after its onset, before coming to code for a choice in the build-up to action (Louie and Glimcher, 2010). These and other findings have led some authors to propose that the signal-dependent build-up of activity observed during viewing of the RDK stimulus reflects a growing expectation that an eye movement into the target field will be rewarded, an expectation that builds faster on high coherence trials, and is normalized by the value of other options (Kable and Glimcher, 2009). Thus, like brain regions classically implicated in economic choices (see below), parietal signals can adapt rapidly to reflect the changing expected value associated with a choice (Sugrue et al., 2005).
Parietal cortex: summary
There is good evidence, thus, that the parietal cortex is involved in integrating sensory information during deliberation, and that this integration occurs largely in the frame of reference of the action. However, the relevant sensorimotor contingencies are most likely learned via a reinforcement learning mechanism; and parietal signals reflect the relative reward of different perceptual alternatives, even when their value changes rapidly and unpredictably. In other words, although much work has focused on the parietal contribution to integration of evidence in PDM, single-cell responses there are strongly biased by the expected economic value of the choice-relevant response, and reward-guided visuo-motor learning rescales the responses of parietal neurons, leading to faster accumulation for more practiced choices. In subsequent sections, we contrast the involvement of the parietal cortex in the decision process to that of other candidate structures, such as the basal ganglia.
The Basal Ganglia Nuclei
The basal ganglia are a family of interconnected subcortical nuclei that have been implicated in a complex array of overlapping cognitive and motor behaviors. A detailed review of the functional architecture of the basal ganglia is beyond the scope of this review; we refer the reader to excellent accounts elsewhere (Alexander and Crutcher, 1990; Chevalier and Deniau, 1990; Doll and Frank, 2009; Redgrave et al., 2011). However, we begin by highlighting two architectural features of the basal ganglia that are of particular relevance to our understanding of their contribution to decision-making. Firstly, the striatum receives inputs from a number of cortical regions, including those concerned with processing both sensory and motor information, as well as receiving information about reward from the ventral midbrain (Alexander and Crutcher, 1990). Moreover, its neurons show gradually accelerating firing rates in response to a noisy RDK stimulus, “ramping” activity that is similar in many respects to that observed at the cortex (Ding and Gold, 2010). It is thus ideally placed to contribute to sensorimotor integration and reward-guided learning during perceptual choice tasks. Secondly, information passing through the basal ganglia is routed via one of two major conduits, known as the direct and indirect pathway, that have opposing inhibitory and disinhibitory control over the thalamus and thus on subsequent cortical motor structures such as the premotor area or superior colliculus. Striatal inputs can thus lead to selective disinhibition of the relevant motor area, a hallmark of any system engaged in efficient selection of one action over two or more competing alternatives (Chevalier and Deniau, 1990; Redgrave et al., 1999). Additionally, neuropsychological evidence also attests to the importance of the striatum in reward- and feedback-guided sensorimotor learning. Caudate lesions impair the ability to learn new visuo-motor associations from feedback (Packard et al., 1989), a phenomenon also observed following degeneration of the nigro-striatal pathway in Parkinson’s Disease (Ashby et al., 2003), whereas disconnection of all visual cortical outputs except those to the striatum spares visuo-motor discrimination (Eacott and Gaffan, 1991). In other words, sensory input to the striatum seems to be both necessary and sufficient for the learning mechanisms that lead to accurate perceptual category judgments. Together, these considerations point to the basal ganglia playing a key role in the mechanisms by which actions are selected, on the basis of integrated sensory and reward information.
Cortico-striatal plasticity and sensorimotor learning
Information from cortical integrators, such as those LIP neurons that exhibit ramping activity during discrimination judgments about randomly moving dots, is subsequently routed to the striatum (Pare and Wurtz, 2001). A consensus holds that Hebbian synaptic plasticity at the striatum depends on the presence of phasic dopaminergic inputs arising in midbrain regions sensitive to reinforcement (Wickens, 1993). Dopamine signaling from the ascending nigro-striatal pathway thus gates cortico-striatal plasticity, such that rewards occurring in a window lasting for a few seconds after an action strengthen synapses linking sensory information to the relevant action (Kerr and Wickens, 2001). The sign, amplitude and timing of these dopaminergic inputs are tightly correlated with those of hypothetical “prediction error” signals that guide simulated reinforcement learning. Intriguingly, there is evidence that both the presence and absence of dopamine is functionally significant at the striatum. Increased dopamine uptake at D1 receptors promotes potentiation of sensorimotor responses that evoke positive outcomes, whereas decreased uptake at a separate, D2-receptors mediated mechanism leads to reduced sensorimotor efficacy where the relevant pairing is followed by a punishment (Doll and Frank, 2009). Thus, patients with Parkinson’s disease, where DA signaling is chronically lowered, tend to learn better from negative than positive outcomes, and may even learn about punishment more effectively than controls (Frank et al., 2004). These “go” and “no-go” signals may be routed to the thalamus via separate direct and indirect pathways that exert inhibitory and disinhibitory control over the thalamus respectively (Frank, 2005). Thus, the architecture of the basal ganglia is well disposed to allow learning about the value of responding (or of inhibiting a response) in a given sensory context to proceed in a supervised fashion, via integration with information about unexpected reward from the ventral midbrain.
One consequence of enhanced responsiveness of cortico-striatal synapses could be to effectively reduce the level of cortical activity required to elicit a response in the striatum, and thus a disinhibition of the relative output neurons in the cortex or superior colliculus. Cortico-striatal plasticity has thus been proposed as a plausible neurobiological mechanism by which the decision threshold (for example, the “bound” in the DDM) could be lowered in order to bias responding by reward (Ito and Doya, 2011), or to adapt response times to optimize reward rate under different conditions that emphasize speed or accuracy (Bogacz et al., 2010), as discussed above. This account draws support from biologically realistic network simulations of threshold modulation in the random-dot motion task, in which dopamine-mediated cortico-striatal plasticity determines the threshold level that must be achieved for an all-or-none disinhibition of choice-appropriate neurons in the superior colliculus mediating the required saccadic response (Lo and Wang, 2006).
Cortico-striatal plasticity and decisions biased by reward
However, one caveat to this account is that adaptation of synaptic efficacy might not occur fast enough to mediate the rapid switching between speed- and accuracy-based responding, or to accommodate situations in which the rewards associated with two perceptual alternative reverse rapidly, as is required by laboratory tasks (Furman and Wang, 2008). Nevertheless, dopaminergic inputs might also act to bias action selection via direct excitatory inputs to the striatum. For example, when choosing a saccadic response conditioned on the spatial location of a target, the responses of striatal neurons reflect the integration of the action and its expected value (Hikosaka, 2007). Striatal signals reflecting a bias toward a more valuable option can be seen even in the period before stimulation begins, and thus are good candidate substrates for the offset in the accumulation of evidence toward a more rewarded choice in serial decision models such as the DDM (Lauwereyns et al., 2002; Ding and Hikosaka, 2007). However, selective dopaminergic receptor blockade abolishes the striatal responses with a corresponding effect on reaction times (Nakamura and Hikosaka, 2006), suggesting that the influence of reward on the choice process depends on direct inputs from the dopaminergic system (but see Choi et al., 2005). In a similar vein, the observed functional connectivity occurring when speed is emphasized over accuracy might reflect a selecting boosting of striatal activity by phasic input from cortical structures such as the supplementary motor area, rather than slow plastic changes at cortico-striatal synapses (Bogacz et al., 2010). In humans, support for this view is offered by the finding that cortico-striatal functional (Harsay et al., 2011) and structural (Forstmann et al., 2010) connectivity both predict individual differences in the extent to which a motivating stimulus enhances choice performance.
Response competition and action inhibition
Selectively lowering the threshold for one course of action (decision bound) is one candidate mechanism by which an economically favorable action might be privileged over other alternatives. As described above in section “Conflict and Response Competition” above, however, another possibility is that active competition occurs between rival options coupled by local inhibitory connections. This competition is proposed by several computational models of perceptual choice, including those that have attempted to describe decisions among three or more options, such as the Wang model (Wang, 2002; Wong and Wang, 2006), the LCA model (Usher and McClelland, 2001), and the MSPRT (Bogacz and Larsen, 2011). When choice alternatives conflict, an ideal observer will prolong deliberation in order to increase the chances that a single clear winner will emerge. Indeed, faced with the choice between two highly valued options, humans deliberate for longer than when choosing between a high- and a low-valued option, apparently inhibiting the impulse to respond rapidly on the basis of prior reinforcement (Ratcliff and Frank, 2012). At least two models propose that this inhibition depends on computations occurring as information flows through the basal ganglia. Bogacz and colleagues(Bogacz and Gurney, 2007; Bogacz and Larsen, 2011) suggest that restraining responses according to the cumulative evidence in favor of the alternatives might be one function of the indirect basal ganglia pathway, whereas Frank (Frank et al., 2007; Doll and Frank, 2009) has convincingly argued that this function is the province of a third, hyper-direct pathway through the basal ganglia, which links the cortex to the basal ganglia output nuclei via the subthalamic nucleus (STN). For example, patients undergoing disruptive deep-brain stimulation of the STN will respond impulsively on choices between two highly valued options, unlike healthy controls (Frank et al., 2007). Recent evidence suggests that the STN may act to raise the decision threshold under conditions of response competition by modulating activity in the medial PFC (Cavanagh et al., 2011). This consistent with a study implicating of the anterior cingulate cortex in threshold modulation, during decisions about visual stimuli linked by fixed transitional probabilities (Domenech and Dreher, 2010).
Basal ganglia: summary
There is thus compelling evidence that the basal ganglia contribute to sensorimotor learning, via dopamine-gated changes in cortico-striatal plasticity. Potentiated cortico-striatal connections might be one mechanism by which an economically advantageous alternative might be favored in a perceptual choice task such as the RDK paradigm. Relatedly, basal ganglia structures such as the STN might act to raise the decision threshold, particularly in the immediate post-stimulus period, when several competing responses simultaneously appear promising (Ratcliff and Frank, 2012). Finally, direct excitatory inputs from cortical or subcortical structures might provoke a pre-stimulus bias toward a more rewarding option that is visible in baseline levels of activity in striatal neurons, a factor that can account for the influence of reward-mediated bias on reaction times in serial sampling models such as the DDM. In the following section, we consider how we might reconcile these findings with the suggestion that parietal signals are also modulated by expected value during PDM (Sugrue et al., 2005), or that cortico-cortical plasticity is an important substrate for sensorimotor learning (Balleine et al., 2009).
Comparing the Contributions of the Cortex and Basal Ganglia to Decision-Making
The work described in sections “Parietal Cortex” and “The Basal Ganglia Nuclei” above suggests that both the parietal cortex and the basal ganglia make an important contribution to the integration perceptual evidence about the identity of a stimulus with information about its economic value. For example, (i) both parietal and striatal neurons seem to act as integrators during noisy perceptual decision tasks such as the RDK paradigm; (ii) cortico-cortical and cortico-striatal plasticity both seem good candidates for mediating reward-guided learning mechanisms during perceptual discrimination and categorization judgments, and (iii) biases toward a more economically valuable option seem to be reflected in an additive offset to pre-stimulus activity in both the parietal cortex and striatum. How can we reconcile these two accounts? Might it be that evolution has equipped primates with two mechanisms for learning the value of sensorimotor acts, and if so, why?
Complementary control systems in the striatum and neocortex
According to classic accounts, cortical and subcortical regions contribute to decisions over distinct timescales, with explicit action planning about novel response contingencies occurring in the cortex, before being consolidated to more phylogenetically ancient subcortical circuits implicated in habit-based behaviors (Dickinson and Balleine, 2002). For example, one computational model suggests that long-run average action values are “cached” in a dorsolateral striatal territories, providing a stable but inflexible representation of the value of actions that is immune to noise-driven fluctuations in the value of different actions, in contrast to more labile representations in the PFC (Daw et al., 2005). According to this and many similar proposals, slow “model-free” RL processes depend on the basal ganglia, whereas the neocortex and hippocampus provide the agent with an explicit model of the world that can be used to control behavior in a cognitively sophisticated, “model-based” fashion (Glascher et al., 2010).
However, experimental evidence suggests that the learning in the basal ganglia can, in fact, occur quite rapidly. For example, in neurophysiological recordings (Schultz et al., 1997) and functional neuroimaging studies (O’Doherty et al., 2003), ventral midbrain or striatal responses are found to track choice values even when they change over just tens of trials. Although this is slower than the moment-by-moment contextual control over action selection demonstrably afforded by the dorsolateral PFC in humans following explicit instructions (Koechlin et al., 2003), it is much faster than the practice-driven cortico-cortical changes observed in incremental perceptual learning studies. Correspondingly, although both the cortex and the striatum are the targets of ascending dopaminergic signals presumed to carry reward prediction errors, only in the striatum does dopamine reuptake occur rapidly (Cragg et al., 1997). By contrast, the neocortical dopamine response to a single reward, such as a food pellet, can still be detected many minutes later (Feenstra and Botterblom, 1996). This presumably allows striatal dopamine to reinforce punctate sensorimotor events, rather than prolonged tasks or episodes.
Thus, one possibility is that during perceptual discrimination tasks, supervised, dopamine-gated reinforcement learning occurs relatively rapidly at the striatum, allowing information about more rewarding stimuli a greater opportunity of flowing through the basal ganglia loops to drive motor output structures back in the cortex. Simultaneously, cortico-cortical learning proceeds in an unsupervised fashion, allowing sensory and motor representations that are frequently reinforced and linked through the circuitry of the basal ganglia to be associated via Hebbian principles. One way of thinking about this is that if we wish to term the subcortical system “habitual,” then this cortico-cortical system is “super-habitual” (M. J. Frank, personal communication). This theory, proposed in a number of guises over recent years (Houk and Wise, 1995; Ashby et al., 2007) and incorporated into leading models of basal ganglia function (Frank, 2005; Bogacz and Larsen, 2011) draws upon the idea familiar from theories of memory that new associations are consolidated to the cortex, providing complementary flexible and stable control over behavior (Norman and O’Reilly, 2003). The most compelling evidence in favor of this view comes from single-cell recordings in the striatum and PFC during reversal learning, which have revealed that responses in the caudate adapt earlier than in the cortex – within as little as five trials of an unpredicted switch (Pasupathy and Miller, 2005). Effective connectivity analyses of fMRI data in humans performing a discrimination task also suggest that the instantiation of connectivity between sensory regions and the frontal cortex depends on the mediating influence of the basal ganglia (den Ouden et al., 2010). Finally, a recent perceptual learning experiment demonstrated that while practice-driven gain enhancements to the gain of encoding of the DV in parietal cortex and ACC were predicted by an RL model, prediction error signals generated by the model correlated with activity in the ventral striatum (Kahnt et al., 2011). These latter studies point to striatal prediction error signals as a ubiquitous mechanism by which the cortex might learn slowly about appropriate sensorimotor contingencies, irrespective of whether they lead to explicit incentives (as in EDM) or merely informative feedback (as in PDM).
One attractive feature of this account is that it explains one dissociation (familiar from computational models) between how slow-practice-driven changes, and fast, context-dependent biases influence reward-guided choices. Perceptual learning experiments have suggested that practice enhances the gain of the DV (increased drift rate in serial sampling models), a phenomenon that is captured by a steeper slope of evidence accumulation in LIP (Law and Gold, 2009) and reflected in the scaling of the BOLD signal in corresponding regions (Kahnt et al., 2011) after extensive training on discrimination tasks. This would be a natural consequence of increased synaptic weights between sensory and higher cortical regions, such as MT and LIP during discrimination of the RDK stimulus, following a dopaminergic “teaching” signal from subcortical regions. By contrast, when the value of a choice is biased by a pre-stimulus cue or other local context, converging neural and computational evidence suggests the offset to accumulation tends to be additive, rather than multiplicative. In the context of computational models of the decision process, this is represented as a bias to the origin of the sampling process, which leads to more fast errors but does not increase discrimination sensitivity (Ratcliff and Rouder, 1998). This would be consistent with a fast, excitatory boosting of activity in parietal neurons, either by throughput from the basal ganglia or via the modulatory influence of other regions, such as the prefrontal or orbitofrontal cortices. However, more work is required to confirm this distinction.
Orbitofrontal Cortex
Given the nature of the RDK task, it is hardly surprising that researchers have focused on understanding activity in a network of interconnected regions involved in motion perception and oculomotor control. However, whilst dorsal stream cortical sites might be the recipients of information about visual motion, it is less clear that they are involved in identifying and comparing complex visual objects, such as the food items whose value we might want to judge when shopping at the local supermarket (although see Toth and Assad, 2002). Rather, complex visual objects tend to be processed in ventral stream sites along the temporal lobe, from where information is routed to limbic structures in the medial temporal lobe and OFC (Price, 2007). Below, we discuss current understanding of the OFC’s contribution to decision-making, and compare it to the circuits described above.
Orbitofrontal cortex and absolute stimulus value
An influential theory has argued that the OFC is responsible for encoding the value of sensory stimuli, and that it plays a direct role in modulating voluntary choice on the basis of absolute (or “menu-invariant”) stimulus values, via its interconnectivity with the ventral striatum (Kable and Glimcher, 2009). This theory has been bolstered by a wealth of imaging studies (O’Doherty et al., 2001; Plassmann et al., 2007) and a smaller number of highly influential single-cell recording studies (Padoa-Schioppa and Assad, 2006, 2008; Kennerley et al., 2009) demonstrating that neural signals in the OFC encode the subjective value of a prospect or gamble. For example, in some well-discussed examples, it has been shown that fMRI signals in the medial OFC scale with the monetary value that hungry participants are willing to pay for a food item (Plassmann et al., 2007) or the subjective reward associated with an erotic image (Prevost et al., 2010), and that cells in the OFC track the combined and relative value of two liquid rewards on offer (Padoa-Schioppa and Assad, 2006). Lesions studies in the non-human primate (Dias et al., 1997) and in human neuropsychological patients (Fellows and Farah, 2003) have apparently shown deficits of reward-guided learning, for example in reversal learning tasks and also decision-making tasks (Fellows and Farah, 2007).
Importantly, because OFC signals seem to be scaled by both the probability and magnitude of the potential reward associated with a choice (Knutson et al., 2005), in a fashion that is in turn modulated by a discount factor reflecting how far into the future the reward will be received (Kable and Glimcher, 2007) and an incorporating other well-known behavioral biases such as a sharper scaling for losses than gains (Tom et al., 2007), it has been argued that the OFC encodes stimulus values in a “common currency” that allows for comparison between assets whose value is in different dimensions or domains. For example, such a mechanism could be important for choosing between two courses of action, one with a low probability of a high-reward, and the other with a high probability of a low reward; or to compare consumer items that may have incommensurate advantages and disadvantages, such as a small house in a desirable neighborhood and a large house in a less sought-after area. Once value information is represented with a common metric, it can presumably be combined with an appropriate action in the striatum, in much the same way as was discussed above for simpler sensory information, such as evidence about the direction of moving dots.
Orbitofrontal cortex and goal value
However compelling this account may be, a number of recent results suggest that we might exercise caution in assuming that the OFC simply houses a bank of cells responsible for learning the value of stimuli. One important consideration is that the OFC may not be functionally homogenous. For example, the anterior and posterior OFC have been found to respond to the value of money and erotic stimuli, respectively (Sescousse et al., 2010). Secondly, the medial and lateral OFC differ sharply in their pattern of interconnectivity with the rest of the brain, and whilst it is the lateral OFC that enjoys monosynaptic links with ventral stream areas, it is the medial portion that projects to the ventral striatum (Haber et al., 2006) and that tends to be activated in concert with subjective value in fMRI studies of value-guided choice. Importantly, careful lesion studies in primates have shown that although lesions to the lateral OFC do incur a learning deficit, monkeys lacking the medial OFC learn new values normally (Noonan et al., 2010; Walton et al., 2010, 2011). Moreover, the sensitivity of the medial OFC BOLD signal to reward seems to depend on the parameters of the task. For example, fMRI correlations with willingness-to-pay evaporate when the participant views food items, but makes a choice that instructed by the computer (Plassmann et al., 2007). Similarly, when the choices are made by another agent, the observer’s medial OFC seems to track the values of that agent’s choice, even when the observer has no direct stake in the game (Cooper et al., 2010). These argue against a simple role in absolute stimulus value encoding. Indeed, medial OFC BOLD signals (as well as a subset of neuronal activity) seem to reflect the relative value of the option that is chosen and that which is not (Boorman et al., 2009). For example, when observers are responding according to the learned value associated with a stream of visual stimuli, the medial OFC signals are correlated not only to the relative value of the stimuli, but with the relative BOLD signal in visual regions encoding these stimuli (Philiastides et al., 2010). Moreover, monkeys with medial OFC lesions provoke a subtle pattern of deficits in which choices between two favored options are can be disrupted by the value of a third, lower-valued option (Noonan et al., 2010). These findings seem to point more toward a more active role in the choice process itself, rather than an automatic valuation procedure that feeds into a subsequent decision stage. Rushworth and colleagues have argued thus that the medial OFC may be involved in comparative decisions by focusing attention on the relevant dimensions of a choice, in the pursuit of a long-term goal (Rushworth et al., 2011). This account diverges from other views, in which the OFC learns stimulus values in a “menu-invariant” fashion, uninfluenced by the value of choice alternatives, and comparative choices involve the more dorsal cortico-cortical and cortico-subcortico-cortical circuits discussed above (Kable and Glimcher, 2009).
Further support for this view comes from a recent fMRI study that builds upon work described above, in which participants were free to make eye movements as they chose between food items, and their choices were found to be described by a diffusion model in which drift rates were multiplicatively scaled by the value of the currently fixated option (Krajbich et al., 2010). In the fMRI scanner, medial OFC and ventral striatal signals were found to correlate positively with the value of an option that was currently cued for fixation, and negatively with the value of the option that was not, suggesting that these regions are either involved in orienting attention to relevant attributes in turn, or are downstream from such regions (Lim et al., 2011). We might assume that one contribution of the parietal cortex is to orient attention in concert with the cue, and indeed, BOLD signals were enhanced contralateral to the cued side in this study. Intriguingly, neuropsychological evidence has revealed that when patients with medial OFC damage make decisions about stimuli based on multiple attributes, such as choosing which apartment to rent, they use a suboptimal strategy that involves comparing attribute values within rather than between stimuli, as if they were unable to engage in an active comparison process across attributes (Fellows, 2006).
Thus, as outlined above another way in which choices among perceptual stimuli might be biased by economic information is through preferred sampling of the favored option. This idea is central to DFT, the first serious attempt to understand the influence of reward on perceptual choice with a process model (Busemeyer and Townsend, 1993). In primates, the evidence above supports the view that this mechanism depends on the medial OFC. The fact that this region is thought to be phylogenetically relatively new (Hill et al., 2010) might relate to the fact that preferred sampling of choice-relevant information is most useful when maximizing value over the long-term, for example in pursuit of a complex goal. For example, focusing on the healthiness of a food item might necessitate representation of an abstract, long-term goal, such as losing weight, rather than allowing more immediate considerations, such as tastiness, to guide choice. Accordingly, signals in the medial OFC scale better with health value ratings when healthiness is emphasized, as if the health dimension were sampled more thoroughly in the comparison process (Hare et al., 2011a).
Caveats and Controversies
Much has been learned in recent years about how humans and other primates make decisions. But much remains to be understood. In this section, we point to some issues that remain controversial, and outline suggestions for future research.
Linking Computational Models and Neural Activity
In our view, key issue for future research will be to establish more convincing links between the computational mechanisms proposed by process models of learning and choice (e.g., the DDM) and neural activity recorded during voluntary choice tasks. Steps toward this goal have been made by the single-cell research in LIP in conjunction with the RDK paradigm, in which neural recordings have been used to arbitrate among competing serial sampling models of choice, such as the DDM and the LCA (Beck et al., 2008; Kiani et al., 2008; Churchland et al., 2011). Other valuable contributions have been made by using more realistic models to make predictions about patterns of observed behavior in disease, under pharmacological manipulations or during deep-brain stimulation (Doll and Frank, 2009). In this review, we have highlighted some promising new theories proposed by others, including the idea that cortico-striatal plasticity might control the decision bound (Lo and Wang, 2006), or that dopaminergic inputs might control baseline levels of excitability in the striatal integrators as a means of modulating the origin of an evidence accumulation process (Hikosaka, 2007). Similarly, the STN might provide a separate means to inhibit responding on the basis of response conflict among multiple alternatives (Frank et al., 2007), or the medial OFC might bias the sampling of decision-relevant information and thereby modulate the gain of accumulation of competing options (Lim et al., 2011).
However, attempts to use serial sampling models to make predictions about large-scale changes in BOLD activity across the cortex have relied on more speculative assumptions. For example, competing assumptions about whether evidence integration regions should scale positively (Basten et al., 2010) or negatively (Heekeren et al., 2004) with the uncertainty in the choice process have led to very different regions being implicated in this function in fMRI studies. Several recent authors have proposed that under the assumptions of the DDM, fMRI signals should correlate positively with uncertainty, because in free-response tasks the total integrated neuronal activity up to the choice will be greater when sensory signals are weak or ambiguous (Basten et al., 2010; Liu and Pleskac, 2011); indeed, parietal BOLD signals do behave in this way. But this assumption has not been subjected to rigorous investigation. For example, this view makes the strong prediction that parietal BOLD signals should vary inversely with uncertainty when viewing time is limited by the experimenter. This hypothesis remains to be tested.
A more conceptual issue concerns proposals that neural activity provides insights into the content of decision-relevant “representations” – for example, that medial OFC or parietal neurons encode the “expected value” of a choice. Applying predictions from a biophysically plausible neural network model of perceptual choice (Wong and Wang, 2006) to an economic task in which observers integrated the probability and magnitude of reward, Hunt and colleagues observed oscillatory neural activity with putative sources in the parietal and medial orbitofrontal cortices whose amplitude scaled with offer and choice value (Hunt et al., 2012). However, as they note, under the assumptions of their model the stronger oscillations for higher-valued stimuli was driven by more frequent network transitions in the face of these stimuli, not because a larger fraction of neurons were active in the relevant regions. A major goal for the future should thus be to establish a consensus about the predictions that successful computational models of choice make about neural activity, and subject these to systematic empirical investigation.
Where in the Brain are Decisions Made?
Decisions about percepts or assets involve an irrevocable commitment to one course of action over its competitors. One question that researchers interested in both PDM and EDM are anxious to address, thus, is where in the brain’s decision circuitry this commitment occurs. At this point it might be worth pausing to ask what properties, exactly, one might expect neurons in such a region to exhibit. One possibility is that neurons involved in making definitive choices might show activity that scales with the choice-relevant evidence, up to a decision threshold, such as putative integrator cells in the parietal cortex (Roitman and Shadlen, 2002) and caudate (Ding and Gold, 2010). However, it is not clear that the rapid fall-off in activity that occurs about 70 ms prior to a saccade is a signal that exerts direct control over a downstream region. Rather, it seems more likely that during evidence accumulation, the activity levels in the integrators are being constantly read out in downstream decision regions – and ultimately, in motor structures such as the superior colliculus – that show more dichotomous responses consistent with a commitment to choice (Sparks, 2002). Parietal integrators seem to equivocate, representing the decision-relevant evidence in a graded fashion that varies incrementally in a noise- and signal-driven manner, and is incommensurate with a winner-takes-all decision mechanism. The silencing of these cells immediately before a response might thus reflect corollary feedback that resets the integrators in time for a subsequent decision.
An alternative view is that neurons in regions that make decisions begin by encoding the value of an offer early on in a trial, but later come to encode the choice that the animal will eventually make. Above, we referred to neurons in the parietal cortex and medial OFC that exhibit this property (Padoa-Schioppa and Assad, 2006; Louie and Glimcher, 2010; Hunt et al., 2012). However, without a precise understanding of how information flows through the brain during perceptual or economic choices, it is hard to draw strong conclusions from this finding. For example, information about a stimulus and its corresponding action in the cortex might be sent to subcortical circuits, linked with value information, and fed back to the cortex in a manner that predicts the choice that will be made shortly after. Alternatively, other recurrent mechanisms may take place which allow the best option to be uncovered via a combination of prior information and sensory evidence (Friston, 2005). Continued careful investigation of firing properties of neurons in key decision regions, as well as measurement of their relative timing, will be required to elucidate precisely how an offer is turned into a choice.
Conclusion
In conclusion, we have tried to highlight research that has combined those techniques and concepts classically employed in the literatures concerned with perceptual and EDM. We have reviewed computational models of the decision process, mostly inspired by visual psychophysics, and described research that has attempted to apply these models to economic choices. We then focused on three brain regions – the parietal cortex, basal ganglia, and OFC – that have been implicated in decision-making by single-cell, neuroimaging, and other work, and detailed our current understanding of their relative contributions to voluntary choice. We have attempted to link key model parameters from these models – such as the decision bound, baseline evidence levels, response conflict, or biased sampling – to candidate neural substrates. Finally, we hope that this review will prompt researchers concerned with economic choices to seek new inspiration from the process models offered by PDM, and those concerned with perceptual decision-making to look beyond elementary sensorimotor circuits to understand voluntary choices.
Conflict of Interest Statement
The authors declare that the research was conducted in the absence of any commercial or financial relationships that could be construed as a potential conflict of interest.
References
Alexander, G. E., and Crutcher, M. D. (1990). Functional architecture of basal ganglia circuits: neural substrates of parallel processing. Trends Neurosci. 13, 266–271.
Ashby, F. G., Ennis, J. M., and Spiering, B. J. (2007). A neurobiological theory of automaticity in perceptual categorization. Psychol. Rev. 114, 632–656.
Ashby, F. G., and Gott, R. E. (1988). Decision rules in the perception and categorization of multidimensional stimuli. J. Exp. Psychol. Learn Mem. Cogn. 14, 33–53.
Ashby, F. G., Noble, S., Filoteo, J. V., Waldron, E. M., and Ell, S. W. (2003). Category learning deficits in Parkinson’s disease. Neuropsychology 17, 115–124.
Ashby, F. G., Turner, B. O., and Horvitz, J. C. (2010). Cortical and basal ganglia contributions to habit learning and automaticity. Trends Cogn. Sci. (Regul. Ed.) 14, 208–215.
Balleine, B. W., Liljeholm, M., and Ostlund, S. B. (2009). The integrative function of the basal ganglia in instrumental conditioning. Behav. Brain Res. 199, 43–52.
Basten, U., Biele, G., Heekeren, H. R., and Fiebach, C. J. (2010). How the brain integrates costs and benefits during decision making. Proc. Natl. Acad. Sci. U.S.A. 107, 21767–21772.
Beck, J. M., Ma, W. J., Kiani, R., Hanks, T., Churchland, A. K., Roitman, J., Shadlen, M. N., Latham, P. E., and Pouget, A. (2008). Probabilistic population codes for Bayesian decision making. Neuron 60, 1142–1152.
Behrens, T. E., Woolrich, M. W., Walton, M. E., and Rushworth, M. F. (2007). Learning the value of information in an uncertain world. Nat. Neurosci. 10, 1214–1221.
Bennur, S., and Gold, J. I. (2011). Distinct representations of a perceptual decision and the associated oculomotor plan in the monkey lateral intraparietal area. J. Neurosci. 31, 913–921.
Bogacz, R. (2007). Optimal decision-making theories: linking neurobiology with behaviour. Trends Cogn. Sci. (Regul. Ed.) 11, 118–125.
Bogacz, R., Brown, E., Moehlis, J., Holmes, P., and Cohen, J. D. (2006). The physics of optimal decision making: a formal analysis of models of performance in two-alternative forced-choice tasks. Psychol. Rev. 113, 700–765.
Bogacz, R., and Gurney, K. (2007). The basal ganglia and cortex implement optimal decision making between alternative actions. Neural Comput. 19, 442–477.
Bogacz, R., and Larsen, T. (2011). Integration of reinforcement learning and optimal decision-making theories of the basal ganglia. Neural Comput. 23, 817–851.
Bogacz, R., Usher, M., Zhang, J. X., and McClelland, J. L. (2007). Extending a biologically inspired model of choice: multi-alternatives, nonlinearity and value-based multidimensional choice. Philos. Trans. R. Soc. B Biol. Sci. 362, 1655–1670.
Bogacz, R., Wagenmakers, E. J., Forstmann, B. U., and Nieuwenhuis, S. (2010). The neural basis of the speed-accuracy tradeoff. Trends Neurosci. 33, 10–16.
Bohil, C. J., and Maddox, W. T. (2003). A test of the optimal classifier’s independence assumption in perceptual categorization. Percept. Psychophys. 65, 478–493.
Boorman, E. D., Behrens, T. E., Woolrich, M. W., and Rushworth, M. F. (2009). How green is the grass on the other side? Frontopolar cortex and the evidence in favor of alternative courses of action. Neuron 62, 733–743.
Britten, K. H., Shadlen, M. N., Newsome, W. T., and Movshon, J. A. (1993). Responses of neurons in macaque MT to stochastic motion signals. Vis. Neurosci. 10, 1157–1169.
Brown, S. D., and Heathcote, A. (2008). The simplest complete model of choice response time: linear ballistic accumulation. Cogn. Psychol. 57, 153–178.
Busemeyer, J. R., and Townsend, J. T. (1993). Decision field-theory – a dynamic cognitive approach to decision-making in an uncertain environment. Psychol. Rev. 100, 432–459.
Cavanagh, J. F., Wiecki, T. V., Cohen, M. X., Figueroa, C. M., Samanta, J., Sherman, S. J., and Frank, M. J. (2011). Subthalamic nucleus stimulation reverses mediofrontal influence over decision threshold. Nat. Neurosci. 14, 1462–1467.
Chevalier, G., and Deniau, J. M. (1990). Disinhibition as a basic process in the expression of striatal functions. Trends Neurosci. 13, 277–280.
Choi, W. Y., Balsam, P. D., and Horvitz, J. C. (2005). Extended habit training reduces dopamine mediation of appetitive response expression. J. Neurosci. 25, 6729–6733.
Churchland, A. K., Kiani, R., Chaudhuri, R., Wang, X. J., Pouget, A., and Shadlen, M. N. (2011). Variance as a signature of neural computations during decision making. Neuron 69, 818–831.
Colby, C. L., and Goldberg, M. E. (1999). Space and attention in parietal cortex. Annu. Rev. Neurosci. 22, 319–349.
Cooper, J. C., Kreps, T. A., Wiebe, T., Pirkl, T., and Knutson, B. (2010). When giving is good: ventromedial prefrontal cortex activation for others’ intentions. Neuron 67, 511–521.
Cragg, S., Rice, M. E., and Greenfield, S. A. (1997). Heterogeneity of electrically evoked dopamine release and reuptake in substantia nigra, ventral tegmental area, and striatum. J. Neurophysiol. 77, 863–873.
Critchley, H. D., and Rolls, E. T. (1996). Hunger and satiety modify the responses of olfactory and visual neurons in the primate orbitofrontal cortex. J. Neurophysiol. 75, 1673–1686.
Daw, N. D., Niv, Y., and Dayan, P. (2005). Uncertainty-based competition between prefrontal and dorsolateral striatal systems for behavioral control. Nat. Neurosci. 8, 1704–1711.
Daw, N. D., O’Doherty, J. P., Dayan, P., Seymour, B., and Dolan, R. J. (2006). Cortical substrates for exploratory decisions in humans. Nature 441, 876–879.
de Lafuente, V., and Romo, R. (2006). Neural correlate of subjective sensory experience gradually builds up across cortical areas. Proc. Natl. Acad. Sci. U.S.A. 103, 14266–14271.
den Ouden, H. E., Daunizeau, J., Roiser, J., Friston, K. J., and Stephan, K. E. (2010). Striatal prediction error modulates cortical coupling. J. Neurosci. 30, 3210–3219.
Dhar, R., Nowlis, S. M., and Sherman, S. J. (2000). Trying hard or hardly trying: an analysis of context effects in choice. J. Consum. Psychol. 9, 189–200.
Dias, R., Robbins, T. W., and Roberts, A. C. (1997). Dissociable forms of inhibitory control within prefrontal cortex with an analog of the Wisconsin Card Sort Test: restriction to novel situations and independence from on-line processing. J. Neurosci. 17, 9285–9297.
Dickinson, A., and Balleine, B. (2002). “The role of learning in motivation,” in Stevens’ Handbook of Experimental Psychology Vol. 3: Learning, Motivation and Emotion, ed. C. R. Gallistel (New York: Wiley), 497–533.
Diederich, A., and Busemeyer, J. R. (2006). Modeling the effects of payoff on response bias in a perceptual discrimination task: bound-change, drift-rate-change, or two-stage-processing hypothesis. Percept. Psychophys. 68, 194–207.
Ding, L., and Gold, J. I. (2010). Caudate encodes multiple computations for perceptual decisions. J. Neurosci. 30, 15747–15759.
Ding, L., and Hikosaka, O. (2007). Temporal development of asymmetric reward-induced bias in macaques. J. Neurophysiol. 97, 57–61.
Ditterich, J. (2010). A comparison between mechanisms of multi-alternative perceptual decision making: ability to explain human behavior, predictions for neurophysiology, and relationship with decision theory. Front. Neurosci. 4:184. doi:10.3389/fnins.2010.00184
Doll, B. B., and Frank, M. J. (2009). “The basal ganglia in reward and decision making: computational models and empirical studies,” in Handbook of Reward and Decision-Making, eds J. Dreher and L. Tremblay (Oxford: Academic Press), 399–425.
Doll, B. B., Hutchison, K. E., and Frank, M. J. (2011). Dopaminergic genes predict individual differences in susceptibility to confirmation bias. J. Neurosci. 31, 6188–6198.
Domenech, P., and Dreher, J. C. (2010). Decision threshold modulation in the human brain. J. Neurosci. 30, 14305–14317.
Dorris, M. C., and Glimcher, P. W. (2004). Activity in posterior parietal cortex is correlated with the relative subjective desirability of action. Neuron 44, 365–378.
Eacott, M. J., and Gaffan, D. (1991). The role of monkey inferior parietal cortex in visual discrimination of identity and orientation of shapes. Behav. Brain Res. 46, 95–98.
Ernst, M. O., and Banks, M. S. (2002). Humans integrate visual and haptic information in a statistically optimal fashion. Nature 415, 429–433.
Feenstra, M. G., and Botterblom, M. H. (1996). Rapid sampling of extracellular dopamine in the rat prefrontal cortex during food consumption, handling and exposure to novelty. Brain Res. 742, 17–24.
Fellows, L. K. (2006). Deciding how to decide: ventromedial frontal lobe damage affects information acquisition in multi-attribute decision making. Brain 129(Pt 4), 944–952.
Fellows, L. K., and Farah, M. J. (2003). Ventromedial frontal cortex mediates affective shifting in humans: evidence from a reversal learning paradigm. Brain 126(Pt 8), 1830–1837.
Fellows, L. K., and Farah, M. J. (2007). The role of ventromedial prefrontal cortex in decision making: judgment under uncertainty or judgment per se? Cereb. Cortex 17, 2669–2674.
Feng, S., Holmes, P., Rorie, A., and Newsome, W. T. (2009). Can monkeys choose optimally when faced with noisy stimuli and unequal rewards? PLoS Comput. Biol. 5, e1000284. doi:10.1371/journal.pcbi.1000284
Fleming, S. M., Whiteley, L., Hulme, O. J., Sahani, M., and Dolan, R. J. (2010). Effects of category-specific costs on neural systems for perceptual decision-making. J. Neurophysiol. 103, 3238–3247.
Forstmann, B. U., Anwander, A., Schafer, A., Neumann, J., Brown, S., Wagenmakers, E. J., Bogacz, R., and Turner, R. (2010). Cortico-striatal connections predict control over speed and accuracy in perceptual decision making. Proc. Natl. Acad. Sci. U.S.A. 107, 15916–15920.
Frank, M. J. (2005). Dynamic dopamine modulation in the basal ganglia: a neurocomputational account of cognitive deficits in medicated and nonmedicated Parkinsonism. J. Cogn. Neurosci. 17, 51–72.
Frank, M. J., Samanta, J., Moustafa, A. A., and Sherman, S. J. (2007). Hold your horses: impulsivity, deep brain stimulation, and medication in parkinsonism. Science 318, 1309–1312.
Frank, M. J., Seeberger, L. C., and O’Reilly, C. R. (2004). By carrot or by stick: cognitive reinforcement learning in parkinsonism. Science 306, 1940–1943.
Friedman-Hill, S. R., Robertson, L. C., and Treisman, A. (1995). Parietal contributions to visual feature binding: evidence from a patient with bilateral lesions. Science 269, 853–855.
Friston, K. (2005). A theory of cortical responses. Philos. Trans. R. Soc. Lond. B Biol. Sci. 360, 815–836.
Furman, M., and Wang, X. J. (2008). Similarity effect and optimal control of multiple-choice decision making. Neuron 60, 1153–1168.
Gao, J. A., Tortell, R., and McClelland, J. L. (2011). Dynamic integration of reward and stimulus information in perceptual decision-making. PLoS ONE 6, e16749. doi:10.1371/journal.pone.0016749
Gigerenzer, G., and Selten, R. (2001). Bounded Rationality: The Adaptive Toolbox. Cambridge: MIT Press, 37–50.
Gilovich, T., Griffin, D. W., and Kahneman, D. (2002). Heuristics and Biases: The Psychology of Intuitive Judgement. New York: Cambridge University Press.
Glascher, J., Daw, N., Dayan, P., and O’Doherty, J. P. (2010). States versus rewards: dissociable neural prediction error signals underlying model-based and model-free reinforcement learning. Neuron 66, 585–595.
Glockner, A., and Herbold, A. K. (2011). An eye-tracking study on information processing in risky decisions: evidence for compensatory strategies based on automatic processes. J. Behav. Decis. Mak. 24, 71–98.
Gold, J. I., and Shadlen, M. N. (2001). Neural computations that underlie decisions about sensory stimuli. Trends Cogn. Sci. (Regul. Ed.) 5, 10–16.
Gold, J. I., and Shadlen, M. N. (2007). The neural basis of decision making. Annu. Rev. Neurosci. 30, 535–574.
Gonzalez-Vallejo, C. (2002). Making trade-offs: a probabilistic and context-sensitive model of choice behavior. Psychol. Rev. 109, 137–154.
Gottlieb, J. (2007). From thought to action: the parietal cortex as a bridge between perception, action, and cognition. Neuron 53, 9–16.
Gottlieb, J., and Balan, P. (2010). Attention as a decision in information space. Trends Cogn. Sci. (Regul. Ed.) 14, 240–248.
Haber, S. N., Kim, K. S., Mailly, P., and Calzavara, R. (2006). Reward-related cortical inputs define a large striatal region in primates that interface with associative cortical connections, providing a substrate for incentive-based learning. J. Neurosci. 26, 8368–8376.
Hare, T. A., Malmaud, J., and Rangel, A. (2011a). Focusing attention on the health aspects of foods changes value signals in vmPFC and improves dietary choice. J. Neurosci. 31, 11077–11087.
Hare, T. A., Schultz, W., Camerer, C. F., O’Doherty, J. P., and Rangel, A. (2011b). Transformation of stimulus value signals into motor commands during simple choice. Proc. Natl. Acad. Sci. U.S.A. 108, 18120–18125.
Harsay, H. A., Cohen, M. X., Oosterhof, N. N., Forstmann, B. U., Mars, R. B., and Ridderinkhof, K. R. (2011). Functional connectivity of the striatum links motivation to action control in humans. J. Neurosci. 31, 10701–10711.
Heekeren, H. R., Marrett, S., Bandettini, P. A., and Ungerleider, L. G. (2004). A general mechanism for perceptual decision-making in the human brain. Nature 431, 859–862.
Heekeren, H. R., Marrett, S., and Ungerleider, L. G. (2008). The neural systems that mediate human perceptual decision making. Nat. Rev. Neurosci. 9, 467–479.
Hikosaka, O. (2007). Basal ganglia mechanisms of reward-oriented eye movement. Ann. N. Y. Acad. Sci. 1104, 229–249.
Hill, J., Inder, T., Neil, J., Dierker, D., Harwell, J., and Van Essen, D. (2010). Similar patterns of cortical expansion during human development and evolution. Proc. Natl. Acad. Sci. U.S.A. 107, 13135–13140.
Hogarth, R. M., and Einhorn, H. J. (1992). Order effects in belief updating – the belief-adjustment model. Cogn. Psychol. 24, 1–55.
Holyoak, K. J., and Simon, D. (1999). Bidirectional reasoning in decision making by constraint satisfaction. J. Exp. Psychol. Gen. 128, 3–31.
Hotaling, J. M., Busemeyer, J. R., and Li, J. Y. (2010). Theoretical developments in decision field theory: comment on Tsetsos, Usher, and Chater (2010). Psychol. Rev. 117, 1294–1298.
Houk, J. C., and Wise, S. P. (1995). Distributed modular architectures linking basal ganglia, cerebellum, and cerebral cortex: their role in planning and controlling action. Cereb. Cortex 5, 95–110.
Huber, J., Payne, J. W., and Puto, C. (1982). Adding asymmetrically dominated alternatives – violations of regularity and the similarity hypothesis. J. Consum. Res. 9, 90–98.
Huk, A. C., and Shadlen, M. N. (2005). Neural activity in macaque parietal cortex reflects temporal integration of visual motion signals during perceptual decision making. J. Neurosci. 25, 10420–10436.
Hunt, L. T., Kolling, N., Soltani, A., Woolrich, M. W., Rushworth, M. F., and Behrens, T. E. (2012). Mechanisms underlying cortical activity during value-guided choice. Nat. Neurosci. 15, 470–476.
Husain, M., Mannan, S., Hodgson, T., Wojciulik, E., Driver, J., and Kennard, C. (2001). Impaired spatial working memory across saccades contributes to abnormal search in parietal neglect. Brain 124(Pt 5), 941–952.
Ito, M., and Doya, K. (2011). Multiple representations and algorithms for reinforcement learning in the cortico-basal ganglia circuit. Curr. Opin. Neurobiol. 21, 368–373.
Johnson, D. M. (1939). Confidence and Speed in the Two Category judgment. New York: Columbia University, 52.
Johnson, J. G., and Busemeyer, J. R. (2005). A dynamic, stochastic, computational model of preference reversal phenomena. Psychol. Rev. 112, 841–861.
Jonas, E., Schulz-Hardt, S., and Frey, D. (2001). Confirmation bias in information seeking under simultaneous vs. sequential information presentation. Z. Exp. Psychol. 48, 239–247.
Kable, J. W., and Glimcher, P. W. (2007). The neural correlates of subjective value during intertemporal choice. Nat. Neurosci. 10, 1625–1633.
Kable, J. W., and Glimcher, P. W. (2009). The neurobiology of decision: consensus and controversy. Neuron 63, 733–745.
Kahneman, D., Slovic, P., and Tversky, A. (1982). Judgment Under Uncertainty: Heuristics and Biases. New York: Cambridge University Press.
Kahneman, D., and Tversky, A. (1979). Prospect theory: an analysis of decision under risk. Econometrica 47, 263–291.
Kahnt, T., Grueschow, M., Speck, O., and Haynes, J. D. (2011). Perceptual learning and decision-making in human medial frontal cortex. Neuron 70, 549–559.
Keeney, R. L., and Raiffa, H. (1993). Decisions with Multiple Objectives: Preferences and Value Tradeoffs. Cambridge: Cambridge University Press.
Kennerley, S. W., Dahmubed, A. F., Lara, A. H., and Wallis, J. D. (2009). Neurons in the frontal lobe encode the value of multiple decision variables. J. Cogn. Neurosci. 21, 1162–1178.
Kerr, J. N., and Wickens, J. R. (2001). Dopamine D-1/D-5 receptor activation is required for long-term potentiation in the rat neostriatum in vitro. J. Neurophysiol. 85, 117–124.
Kiani, R., Hanks, T. D., and Shadlen, M. N. (2008). Bounded integration in parietal cortex underlies decisions even when viewing duration is dictated by the environment. J. Neurosci. 28, 3017–3029.
Kim, J. N., and Shadlen, M. N. (1999). Neural correlates of a decision in the dorsolateral prefrontal cortex of the macaque. Nat. Neurosci. 2, 176–185.
Knutson, B., Taylor, J., Kaufman, M., Peterson, R., and Glover, G. (2005). Distributed neural representation of expected value. J. Neurosci. 25, 4806–4812.
Koechlin, E., Ody, C., and Kouneiher, F. (2003). The architecture of cognitive control in the human prefrontal cortex. Science 302, 1181–1185.
Koechlin, E., and Summerfield, C. (2007). An information theoretical approach to prefrontal executive function. Trends Cogn. Sci. (Regul. Ed.) 11, 229–235.
Kording, K. P., and Wolpert, D. M. (2004). Bayesian integration in sensorimotor learning. Nature 427, 244–247.
Krajbich, I., Armel, C., and Rangel, A. (2010). Visual fixations and the computation and comparison of value in simple choice. Nat. Neurosci. 13, 1292–1298.
Krajbich, I., Armel, C., and Rangel, A. (2011). Visual fixations and the computation and comparison of value in simple choice. Nat. Neurosci. 14, 1217–1217.
Krajbich, I., and Rangel, A. (2011). Multialternative drift-diffusion model predicts the relationship between visual fixations and choice in value-based decisions. Proc. Natl. Acad. Sci. U.S.A. 108, 13852–13857.
Lauwereyns, J., Watanabe, K., Coe, B., and Hikosaka, O. (2002). A neural correlate of response bias in monkey caudate nucleus. Nature 418, 413–417.
Law, C. T., and Gold, J. I. (2008). Neural correlates of perceptual learning in a sensory-motor, but not a sensory, cortical area. Nat. Neurosci. 11, 505–513.
Law, C. T., and Gold, J. I. (2009). Reinforcement learning can account for associative and perceptual learning on a visual-decision task. Nat. Neurosci. 12, 655–663.
Le Mens, G., and Denrell, J. (2011). Rational learning and information sampling: on the naivety assumption in sampling explanations of judgment biases. Psychol. Rev. 118, 379–392.
Lim, S. L., O’Doherty, J. P., and Rangel, A. (2011). The decision value computations in the vmPFC and striatum use a relative value code that is guided by visual attention. J. Neurosci. 31, 13214–13223.
Liu, T., and Pleskac, T. J. (2011). Neural correlates of evidence accumulation in a perceptual decision task. J. Neurophysiol. 106, 2383–2398.
Lo, C. C., and Wang, X. J. (2006). Cortico-basal ganglia circuit mechanism for a decision threshold in reaction time tasks. Nat. Neurosci. 9, 956–963.
Loewenstein, G., and Thaler, R. H. (1989). Anomolies – intertemporal choice. J. Econ. Perspect. 3, 181–193.
Louie, K., and Glimcher, P. W. (2010). Separating value from choice: delay discounting activity in the lateral intraparietal area. J. Neurosci. 30, 5498–5507.
Maloney, L. T. (1991). Distributional assumptions and observed conservatism in the theory of signal detectability. J. Math. Psychol. 35, 443–470.
Matsumoto, M., Matsumoto, K., Abe, H., and Tanaka, K. (2007). Medial prefrontal cell activity signaling prediction errors of action values. Nat. Neurosci. 10, 647–656.
Milosavljevic, M., Malmaud, J., Huth, A., Koch, C., and Rangel, A. (2010). The drift diffusion model can account for the accuracy and reaction time of value-based choices under high and low time pressure. Judgm. Decis. Mak. 5, 437–449.
Mulder, M. J., Wagenmakers, E. J., Ratcliff, R., Boekel, W., and Forstmann, B. U. (2012). Bias in the brain: a diffusion model analysis of prior probability and potential payoff. J. Neurosci. 32, 2335–2343.
Nakamura, K., and Hikosaka, O. (2006). Role of dopamine in the primate caudate nucleus in reward modulation of saccades. J. Neurosci. 26, 5360–5369.
Newell, B. R., Wong, K. Y., Cheung, J. C. H., and Rakow, T. (2009). Think, blink or sleep on it? The impact of modes of thought on complex decision making. Q. J. Exp. Psychol. 62, 707–732.
Noonan, M. P., Walton, M. E., Behrens, T. E., Sallet, J., Buckley, M. J., and Rushworth, M. F. (2010). Separate value comparison and learning mechanisms in macaque medial and lateral orbitofrontal cortex. Proc. Natl. Acad. Sci. U.S.A. 107, 20547–20552.
Norman, K. A., and O’Reilly, R. C. (2003). Modeling hippocampal and neocortical contributions to recognition memory: a complementary-learning-systems approach. Psychol. Rev. 110, 611–646.
Oaksford, M., and Chater, N. (1996). Rational explanation of the selection task. Psychol. Rev. 103, 381–391.
O’Doherty, J., Kringelbach, M. L., Rolls, E. T., Hornak, J., and Andrews, C. (2001). Abstract reward and punishment representations in the human orbitofrontal cortex. Nat. Neurosci. 4, 95–102.
O’Doherty, J. P., Dayan, P., Friston, K., Critchley, H., and Dolan, R. J. (2003). Temporal difference models and reward-related learning in the human brain. Neuron 38, 329–337.
Otter, T., Johnson, J., Rieskamp, J., Allenby, G. M., Brazell, J. D., Diederich, A., Hutchinson, J. W., MacEachern, S., Ruan, S. L., and Townsend, J. (2008). Sequential sampling models of choice: some recent advances. Mark. Lett. 19, 255–267.
Packard, M. G., Hirsh, R., and White, N. M. (1989). Differential effects of fornix and caudate nucleus lesions on two radial maze tasks: evidence for multiple memory systems. J. Neurosci. 9, 1465–1472.
Padoa-Schioppa, C., and Assad, J. A. (2006). Neurons in the orbitofrontal cortex encode economic value. Nature 441, 223–226.
Padoa-Schioppa, C., and Assad, J. A. (2008). The representation of economic value in the orbitofrontal cortex is invariant for changes of menu. Nat. Neurosci. 11, 95–102.
Parducci, A., and Fabre, J. M. (1995). Contextual effects in judgment and choice. Adv. Consum. Res. 16, 97–109.
Pare, M., and Wurtz, R. H. (2001). Progression in neuronal processing for saccadic eye movements from parietal cortex area lip to superior colliculus. J. Neurophysiol. 85, 2545–2562.
Pasupathy, A., and Miller, E. K. (2005). Different time courses of learning-related activity in the prefrontal cortex and striatum. Nature 433, 873–876.
Payne, J. W. (1976). Task complexity and contingent processing in decision making: an information search and protocol analysis. Organ. Behav. Hum. Perform. 16, 366–387.
Philiastides, M. G., Biele, G., and Heekeren, H. R. (2010). A mechanistic account of value computation in the human brain. Proc. Natl. Acad. Sci. U.S.A. 107, 9430–9435.
Plassmann, H., O’Doherty, J., and Rangel, A. (2007). Orbitofrontal cortex encodes willingness to pay in everyday economic transactions. J. Neurosci. 27, 9984–9988.
Platt, M. L., and Glimcher, P. W. (1999). Neural correlates of decision variables in parietal cortex. Nature 400, 233–238.
Pleger, B., Ruff, C. C., Blankenburg, F., Kloppel, S., Driver, J., and Dolan, R. J. (2009). Influence of dopaminergically mediated reward on somatosensory decision-making. PLoS Biol. 7, e1000164. doi:10.1371/journal.pbio.1000164
Pleskac, T. J., and Busemeyer, J. R. (2010). Two-stage dynamic signal detection: a theory of choice, decision time, and confidence. Psychol. Rev. 117, 864–901.
Prevost, C., Pessiglione, M., Metereau, E., Clery-Melin, M. L., and Dreher, J. C. (2010). Separate valuation subsystems for delay and effort decision costs. J. Neurosci. 30, 14080–14090.
Price, J. L. (2007). Definition of the orbital cortex in relation to specific connections with limbic and visceral structures and other cortical regions. Ann. N. Y. Acad. Sci. 1121, 54–71.
Rangel, A., and Hare, T. (2010). Neural computations associated with goal-directed choice. Curr. Opin. Neurobiol. 20, 262–270.
Ratcliff, R., and Frank, M. J. (2012). Reinforcement-based decision making in corticostriatal circuits: mutual constraints by neurocomputational and diffusion models. Neural Comput. 24, 1186–1229.
Ratcliff, R., Hasegawa, Y. T., Hasegawa, R. P., Smith, P. L., and Segraves, M. A. (2007). Dual diffusion model for single-cell recording data from the superior colliculus in a brightness-discrimination task. J. Neurophysiol. 97, 1756–1774.
Ratcliff, R., and McKoon, G. (2008). The diffusion decision model: theory and data for two-choice decision tasks. Neural Comput. 20, 873–922.
Ratcliff, R., and Rouder, J. N. (1998). Modeling response times for two-choice decisions. Psychol. Sci. 9, 347–356.
Redgrave, P., Prescott, T. J., and Gurney, K. (1999). The basal ganglia: a vertebrate solution to the selection problem? Neuroscience 89, 1009–1023.
Redgrave, P., Vautrelle, N., and Reynolds, J. N. (2011). Functional properties of the basal ganglia’s re-entrant loop architecture: selection and reinforcement. Neuroscience 198, 138–151.
Rescorla, R. A., and Wagner, A. R. (1972). “A theory of Pavlovian conditioning: variations in the effectiveness of reinforcement and nonreinforcement,” in Classical Conditioning II: Current Research and Theory, eds A. H. Black and W. F. Prokasy (New York: Appleton Century Crofts), 64–99.
Robertson, I. H., and Halligan, P. W. (1999). Spatial Neglect: A Clinical Handbook for Diagnosis and Treatment. Hove: Erlbaum.
Roe, R. M., Busemeyer, J. R., and Townsend, J. T. (2001). Multialternative decision field theory: a dynamic connectionist model of decision making. Psychol. Rev. 108, 370–392.
Roitman, J. D., and Shadlen, M. N. (2002). Response of neurons in the lateral intraparietal area during a combined visual discrimination reaction time task. J. Neurosci. 22, 9475–9489.
Rorie, A. E., Gao, J., McClelland, J. L., and Newsome, W. T. (2010). Integration of sensory and reward information during perceptual decision-making in lateral intraparietal cortex (LIP) of the macaque monkey. PLoS ONE 5, e9308. doi:10.1371/journal.pone.0009308
Rushworth, M. F., and Behrens, T. E. (2008). Choice, uncertainty and value in prefrontal and cingulate cortex. Nat. Neurosci. 11, 389–397.
Rushworth, M. F., Noonan, M. P., Boorman, E. D., Walton, M. E., and Behrens, T. E. (2011). Frontal cortex and reward-guided learning and decision-making. Neuron 70, 1054–1069.
Russo, J., and Rosen, L. (1975). An eye fixation analysis of multialternative choice. Mem. Cognit. 3, 267–276.
Russo, J. E., and Leclerc, F. (1994). An eye-fixation analysis of choice processes for consumer nondurables. J. Consum. Res. 21, 274–290.
Schultz, W. (1986). Responses of midbrain dopamine neurons to behavioral trigger stimuli in the monkey. J. Neurophysiol. 56, 1439–1461.
Schultz, W., Dayan, P., and Montague, P. R. (1997). A neural substrate of prediction and reward. Science 275, 1593–1599.
Serences, J. T., and Saproo, S. (2010). Population response profiles in early visual cortex are biased in favor of more valuable stimuli. J. Neurophysiol. 104, 76–87.
Sescousse, G., Redoute, J., and Dreher, J. C. (2010). The architecture of reward value coding in the human orbitofrontal cortex. J. Neurosci. 30, 13095–13104.
Shanks, D. R., Tunney, R. J., and McCarthy, J. D. (2002). A re-examination of probability matching and rational choice. J. Behav. Decis. Mak. 15, 233–250.
Shimojo, S., Simion, C., Shimojo, E., and Scheier, C. (2003). Gaze bias both reflects and influences preference. Nat. Neurosci. 6, 1317–1322.
Siegel, M., Donner, T. H., Oostenveld, R., Fries, P., and Engel, A. K. (2008). Neuronal synchronization along the dorsal visual pathway reflects the focus of spatial attention. Neuron 60, 709–719.
Sigman, M., and Dehaene, S. (2005). Parsing a cognitive task: a characterization of the mind’s bottleneck. PLoS Biol. 3, e37. doi:10.1371/journal.pbio.0030037
Simen, P., Contreras, D., Buck, C., Hu, P., Holmes, P., and Cohen, J. D. (2009). Reward rate optimization in two-alternative decision making: empirical tests of theoretical predictions. J. Exp. Psychol. Hum. Percept. Perform. 35, 1865–1897.
Simonson, I. (1989). Choice based on reasons – the case of attraction and compromise effects. J. Consum. Res. 16, 158–174.
Sparks, D. L. (2002). The brainstem control of saccadic eye movements. Nat. Rev. Neurosci. 3, 952–964.
Stewart, N., Chater, N., Stott, H. P., and Reimers, S. (2003). Prospect relativity: how choice options influence decision under risk. J. Exp. Psychol. Gen. 132, 23–46.
Sugrue, L. P., Corrado, G. S., and Newsome, W. T. (2004). Matching behavior and the representation of value in the parietal cortex. Science 304, 1782–1787.
Sugrue, L. P., Corrado, G. S., and Newsome, W. T. (2005). Choosing the greater of two goods: neural currencies for valuation and decision making. Nat. Rev. Neurosci. 6, 363–375.
Summerfield, C., Behrens, T. E., and Koechlin, E. (2011). Perceptual classification in a rapidly changing environment. Neuron 71, 725–736.
Summerfield, C., and Koechlin, E. (2009). “Decision-making and prefrontal executive function,” in The Cognitive Neurosciences, Vol. IV, ed. M. S. Gazzaniga (Cambridge: MIT Press), 1019–1030.
Summerfield, C., and Koechlin, E. (2010). Economic value biases uncertain perceptual choices in the parietal and prefrontal cortices. Front. Hum. Neurosci. 4:208. doi:10.3389/fnhum.2010.00208
Svenson, O., and Benthorn, L. J. (1992). Consolidation processes in decision-making – post-decision changes in attractiveness of alternatives. J. Econ. Psychol. 13, 315–327.
Tom, S. M., Fox, C. R., Trepel, C., and Poldrack, R. A. (2007). The neural basis of loss aversion in decision-making under risk. Science 315, 515–518.
Toth, L. J., and Assad, J. A. (2002). Dynamic coding of behaviourally relevant stimuli in parietal cortex. Nature 415, 165–168.
Townsend, J. T., and Ashby, F. G. (1983). The Stochastic Modeling of Elementary Psychological Processes. Cambridge: Cambridge University Press.
Treisman, A. M., and Gelade, G. (1980). A feature-integration theory of attention. Cogn. Psychol. 12, 97–136.
Tsetsos, K., Usher, M., and Chater, N. (2010). Preference reversal in multiattribute choice. Psychol. Rev. 117, 1275–1293.
Tsetsos, K., Usher, M., and McClelland, J. L. (2011). Testing multi-alternative decision models with non-stationary evidence. Front. Neurosci. 5:63. doi:10.3389/fnins.2011.00063
Tversky, A., and Kahneman, D. (1981). The framing of decisions and the psychology of choice. Science 211, 453–458.
Tversky, A., and Kahneman, D. (1991). Loss aversion in riskless choice – a reference-dependent model. Q. J. Econ. 106, 1039–1061.
Usher, M., and McClelland, J. L. (2001). The time course of perceptual choice: the leaky, competing accumulator model. Psychol. Rev. 108, 550–592.
Usher, M., and McClelland, J. L. (2004). Loss aversion and inhibition in dynamical models of multialternative choice. Psychol. Rev. 111, 757–769.
van Ravenzwaaij, D., van der Maas, H. L. J., and Wagenmakers, E. J. (2012). Optimal decision making in neural inhibition models. Psychol. Rev. 119, 201–215.
Vlaev, I., Chater, N., Stewart, N., and Brown, G. D. A. (2011). Does the brain calculate value? Trends Cogn. Sci. (Regul. Ed.) 15, 546–554.
Von Neumann, J., and Morgenstern, O. (1944). Theory of Games and Economic Behavior. Princeton: Princeton university press.
Wald, A., and Wolfowitz, J. (1948). Optimum character of the sequential probability ratio test. Ann. Math. Stat. 19, 326–339.
Walton, M. E., Behrens, T. E., Buckley, M. J., Rudebeck, P. H., and Rushworth, M. F. (2010). Separable learning systems in the macaque brain and the role of orbitofrontal cortex in contingent learning. Neuron 65, 927–939.
Walton, M. E., Behrens, T. E., Noonan, M. P., and Rushworth, M. F. (2011). Giving credit where credit is due: orbitofrontal cortex and valuation in an uncertain world. Ann. N. Y. Acad. Sci. 1239, 14–24.
Wang, X. J. (2002). Probabilistic decision making by slow reverberation in cortical circuits. Neuron 36, 955–968.
Weil, R. S., Furl, N., Ruff, C. C., Symmonds, M., Flandin, G., Dolan, R. J., Driver, J., and Rees, G. (2010). Rewarding feedback after correct visual discriminations has both general and specific influences on visual cortex. J. Neurophysiol. 104, 1746–1757.
Whiteley, L., and Sahani, M. (2008). Implicit knowledge of visual uncertainty guides decisions with asymmetric outcomes. J. Vis. 8, 1–15.
Wickelgren, W. A. (1977). Speed-accuracy tradeoff and information-processing dynamics. Acta Psychol. (Amst.) 41, 67–85.
Wong, K. F., and Wang, X. J. (2006). A recurrent network mechanism of time integration in perceptual decisions. J. Neurosci. 26, 1314–1328.
Keywords: decision-making, reward, psychophysics, basal ganglia, orbitofrontal cortex, parietal cortex
Citation: Summerfield C and Tsetsos K (2012) Building bridges between perceptual and economic decision-making: neural and computational mechanisms. Front. Neurosci. 6:70. doi: 10.3389/fnins.2012.00070
Received: 05 March 2012; Accepted: 26 April 2012;
Published online: 24 May 2012.
Edited by:
Leendert Van Maanen, University of Amsterdam, NetherlandsReviewed by:
Jean-Claude Dreher, National Scientific Research Center, FranceChristian Ruff, University of Zurich, Switzerland
Copyright: © 2012 Summerfield and Tsetsos. This is an open-access article distributed under the terms of the Creative Commons Attribution Non Commercial License, which permits non-commercial use, distribution, and reproduction in other forums, provided the original authors and source are credited.
*Correspondence: Christopher Summerfield, Department Experimental Psychology, University of Oxford, South Parks Road, Oxford OX1 3UD, UK. e-mail: christopher.summerfield@psy.ox.ac.uk