TurtleCam: A “Smart” Autonomous Underwater Vehicle for Investigating Behaviors and Habitats of Sea Turtles
- 1Biology Department, Woods Hole Oceanographic Institution, Woods Hole, MA, United States
- 2Oceanographic Systems Laboratory, Applied Ocean Physics and Engineering Department, Woods Hole Oceanographic Institution, Woods Hole, MA, United States
- 3Massachusetts Division of Marine Fisheries, New Bedford, MA, United States
Sea turtles inhabiting coastal environments routinely encounter anthropogenic hazards, including fisheries, vessel traffic, pollution, dredging, and drilling. To support mitigation of potential threats, it is important to understand fine-scale sea turtle behaviors in a variety of habitats. Recent advancements in autonomous underwater vehicles (AUVs) now make it possible to directly observe and study the subsurface behaviors and habitats of marine megafauna, including sea turtles. Here, we describe a “smart” AUV capability developed to study free-swimming marine animals, and demonstrate the utility of this technology in a pilot study investigating the behaviors and habitat of leatherback turtles (Dermochelys coriacea). We used a Remote Environmental Monitoring UnitS (REMUS-100) AUV, designated “TurtleCam,” that was modified to locate, follow and film tagged turtles for up to 8 h while simultaneously collecting environmental data. The TurtleCam system consists of a 100-m depth rated vehicle outfitted with a circular Ultra-Short BaseLine receiver array for omni-directional tracking of a tagged animal via a custom transponder tag that we attached to the turtle with two suction cups. The AUV collects video with six high-definition cameras (five mounted in the vehicle nose and one mounted aft) and we added a camera to the animal-borne transponder tag to record behavior from the turtle's perspective. Since behavior is likely a response to habitat factors, we collected concurrent in situ oceanographic data (bathymetry, temperature, salinity, chlorophyll-a, turbidity, currents) along the turtle's track. We tested the TurtleCam system during 2016 and 2017 in a densely populated coastal region off Cape Cod, Massachusetts, USA, where foraging leatherbacks overlap with fixed fishing gear and concentrated commercial and recreational vessel traffic. Here we present example data from one leatherback turtle to demonstrate the utility of TurtleCam. The concurrent video, localization, depth and environmental data allowed us to characterize leatherback diving behavior, foraging ecology, and habitat use, and to assess how turtle behavior mediates risk to impacts from anthropogenic activities. Our study demonstrates that an AUV can successfully track and image leatherback turtles feeding in a coastal environment, resulting in novel observations of three-dimensional subsurface behaviors and habitat use, with implications for sea turtle management and conservation.
Introduction
Sea turtles inhabiting coastal environments routinely encounter anthropogenic hazards, including fisheries, vessel traffic, pollution, dredging, and drilling (Lutcavage et al., 1996). These urbanized habitats often require successful navigation of multiple threats to avoid injury or mortality. While there is evidence that sea turtles can modify their behavior based on variable environmental conditions (Hays et al., 2006; Schofield et al., 2009), there is currently little information on behavioral adaptations relative to transient human activities. Sea turtle mortalities in coastal habitats frequently show evidence of human interaction that suggests they are unable to avoid some anthropogenic threats, including vessel strike (Hazel and Gyuris, 2006; Tomás et al., 2008), fishery interactions (Peckham et al., 2007; Casale et al., 2010; Hamelin et al., 2017), and debris ingestion (Bjorndal et al., 1994; Mrosovsky et al., 2009). Furthermore, some turtle behaviors (e.g., shallow water diving, affinity for nearshore habitat) may actually exacerbate risk (Hazel et al., 2009). To develop effective mitigation strategies, we need to understand how turtle behavior and habitat choice mediate risk in coastal feeding grounds.
To investigate fine-scale movements and behaviors of sea turtles, researchers have employed a variety of tools and techniques, including direct subsurface observations (Schofield et al., 2006), radio tags (Avens et al., 2003; Brooks et al., 2009), acoustic tags (van Dam and Diez, 1998; Seminoff et al., 2002), archival tags (Southwood et al., 1999; Fossette et al., 2010), animal-borne camera tag packages (Heithaus et al., 2002; Reina et al., 2005), remotely operated vehicles (ROVs) (Patel et al., 2016), and various combinations of these technologies. While these approaches have resulted in a richer understanding of turtle movements and behaviors in foraging and breeding habitats, few studies have concurrently investigated fine-scale turtle behavior and measured in situ habitat characteristics. Since animal behavior is responsive to environmental conditions, accurate interpretation of turtle behavior requires an understanding of their bio-physical habitat.
Recent advancements in autonomous underwater vehicles (AUVs) now make it possible to directly observe subsurface behaviors and concurrently sample the habitats of marine megafauna (Skomal et al., 2015; Kukulya et al., 2016). Here, we describe a “smart” AUV developed to follow and film free-swimming marine animals, and demonstrate the utility of this technology in a pilot study investigating the subsurface behaviors and habitat of leatherback sea turtles (Dermochelys coriacea) in a high-risk coastal environment. We adapted the SharkCam REMUS-100 AUV developed at the Oceanographic Systems Laboratory (OSL) at the Woods Hole Oceanographic Institution (WHOI) (Packard et al., 2013), and coined it “TurtleCam.” TurtleCam's animal-following algorithms were modified to continuously locate, follow and film a tagged turtle while simultaneously collecting environmental data along the turtle's track. We conducted our pilot study off Cape Cod, Massachusetts, USA, where leatherbacks are resident during boreal summer and fall months (Lazell, 1980; Dodge et al., 2014). In Massachusetts' coastal waters, the leatherbacks' spatially restricted movements, particularly in bays, sounds, and shoal/ledge habitat, coincide with high densities of fixed fishing gear (e.g., weighted gear set on the sea floor), concentrated commercial and recreational vessel traffic, subtidal aquaculture, and renewable energy operations and development (2015 Massachusetts Ocean Management Plan, http://www.mass.gov/eea/docs/eea/oceans/ocean-plan/2015-ocean-plan-v1-complete.pdf). Anthropogenic impacts to leatherback turtles in this region are well-documented by the Greater Atlantic Region Sea Turtle Stranding and Disentanglement Networks, with the primary sources of leatherback mortality attributed to vessel strikes and entanglement in fixed fishing gear (Dwyer et al., 2002; Sampson, 2012). We used the TurtleCam system to understand how leatherback behavior and habitat choice mediated risk to anthropogenic threats, with the ultimate goal of incorporating this information into regional conservation and management plans.
REMUS TurtleCam System
The REMUS TurtleCam system used in this study is custom built at WHOI and available to the research community though collaboration with the WHOI OSL. The system consists of a 25-kHz cylindrical transponder tag (7.6 × 38 cm, 1.7 kg in air, ~58 g buoyancy in seawater), a 100-m depth rated AUV (203 × 19 cm, 48 kg in air), and a shipboard tracking system for tracking the animal independent of the AUV if desired. The 25-kHz frequency of the TurtleCam transponder tag is well above the known underwater hearing range and sensitivity of leatherback turtle hatchlings (Dow-Piniak et al., 2012). While hearing range and sensitivity is unknown for adult leatherbacks, studies involving adult sea turtles of other species also found that they detected sounds in the low frequency sound range (e.g., Bartol et al., 1999), and we do not expect leatherbacks to perceive the higher frequency sound of the transponder. The transponder is attached to the turtle via a custom-built mechanism with two suction cups, and has an embedded acoustic release system that can be activated via an acoustic signal from the tracking boat or by a preprogrammed depth. Once attached to the turtle, the tag remains in a listening-only standby mode, awaiting coded acoustic signals (pings) from either the vehicle or shipboard tracking system. Depending on the ping duty cycle, the tag can remain in a low power state lasting for up to 3 weeks.
TurtleCam is launched (typically within minutes) after a turtle is tagged, and immediately dives and swims to the tagging location programmed by the vehicle operator between tagging and deployment. While transiting to the last known position, the vehicle interrogates the transponder tag every 3 s using its onboard acoustic system, including a 360° Ultra-Short BaseLine (USBL) receiver array, and follows the tag using navigational algorithms for tracking a randomly moving animal. The transponder tag “listens” for a coded signal (interrogation ping) and replies back with two coded signals. The round-trip travel time of the response is calculated by the vehicle and used to determine the range to the turtle. This response is then beam-formed to determine the turtle's bearing relative to the vehicle, and the vehicle's compass (Model Sparton AHRS-8) is used to transform this into an absolute bearing. From these range and bearing estimates, the turtle's location in earth coordinates (latitude/longitude) can be determined. A second response from the tag is time delayed proportional to depth. The time difference between the two responses is used to determine the depth of the turtle. This combination allows precise location of a tagged turtle in three-dimensional space (Figure 1) (Kukulya et al., 2015).
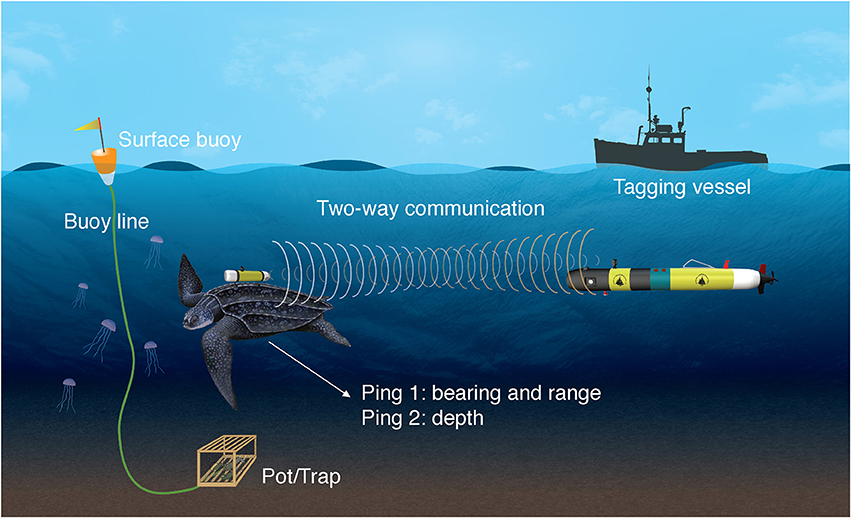
Figure 1. Graphic representation of the TurtleCam system. Continuous, two-way communication between the leatherback turtle's transponder and the vehicle enables the vehicle to locate, follow and film the tagged turtle while simultaneously collecting habitat data along the turtle's path. A camera on the turtle-borne transponder collects video from the turtle's perspective. Turtles were tracked in areas with fixed fishing gear.
In addition to tracking with TurtleCam, the tagged turtle can be tracked from the boat using the shipboard tracking system (STS). The STS is a “vehicle in a box” that uses a shipboard-mounted USBL array, transducer, ship's global positioning system (GPS) receiver, and compass to independently track the tagged turtle. The vehicle pilot also uses the STS to communicate in real time with the TurtleCam vehicle using bidirectional acoustic modems (Model 1.3, acomms.whoi.edu). The STS enables the real-time positions of the turtle, vehicle and boat to be plotted on the operator's laptop, giving the research team immediate visual feedback on how the system is working and what the turtle is doing. Moreover, the turtles surfaced frequently in our study, and direct visual observations could be made from the boat to corroborate the estimated turtle positions derived from the TurtleCam and STS.
The architecture of the animal-following algorithm allows an operator to change the vehicle's position relative to an animal in real time. The algorithm allows multiple parameters that are preprogrammed and then modified during the mission, including speed near the animal (within 10 m), speed far from animal (beyond 10 m), the distance to the animal above, below, forward, behind, port and starboard (in meters). Since individual foraging and swimming behaviors can be highly variable, having the ability to manipulate the position of the vehicle relative to an animal in real time is critical. Real-time visualization via the STS allowed the operator to make mission parameter changes as needed. The duration of TurtleCam missions are limited by the batteries in the cameras (230 min mean battery life). If desired, the research team can recover the vehicle to change out the batteries and SD cards on the cameras, and then relaunch the vehicle for a second mission. While the vehicle is out of the water, the tagged turtle can be tracked with the STS. Upon mission completion, the tag is acoustically commanded to release from the animal using the STS; the suction cups on the turtle are flooded upon receipt of this command, and the slightly buoyant tag floats to the surface where it can be located via the STS or a VHF radio receiver and recovered.
While tracking the tagged turtle, the vehicle collects video with six high definition cameras (Model HERO3+, GoPro, Inc.; www.gopro.com). Five cameras are mounted on the nose and one rear-facing camera is mounted on the top. We also added a camera (Model HERO Session, GoPro, Inc.; www.gopro.com) to the animal-borne transponder to record behavior from the turtle's perspective. The vehicle can be outfitted with different environmental sensors to collect bio-physical habitat data along the animal's track, depending on the mission objectives. Sensors for our study included a 1,200-kHz up-down looking acoustic Doppler current profiler (ADCP) (Teledyn RDI; www.rdinstruments.com) for altimetry, water current data, and speed over ground measurements, as well as a conductivity-temperature (CT) probe (YSI; www.ysi.com), magnetic heading sensor, pressure sensor, and an environmental characterization optics sensor (ECO puck, SeaBird Scientific; www.seabird.com/eco-puck) that measured chlorophyll fluorescence and turbidity.
Pilot Study: Tracking Leatherback Sea Turtles With TurtleCam
Between September 2016 and September 2017, we tagged, tracked and filmed nine leatherback turtles with the TurtleCam system off Cape Cod, Massachusetts, USA. The full results of this pilot study will be reported elsewhere. Here, we present representative data from a single turtle to demonstrate how the TurtleCam system can be successfully used to simultaneously investigate turtle behavior and habitat characteristics in a gear-dense, highly trafficked, coastal feeding ground.
On 7 October, 2016, we worked with a spotter pilot to locate a subadult leatherback turtle in Nantucket Sound. The turtle was found in an area with fixed fishing gear (traps/pots) intersected by shipping/ferry lanes, with a minimum of eight other leatherbacks. We approached the turtle by boat and tagged it at the surface with the transponder using a pole applicator (Figure 2). The transponder was attached to the turtle's carapace with two suction cups, eliminating the need to capture or handle the turtle and potentially impact its behavior (Heaslip et al., 2012). Turtles in our study dove immediately post-tagging, but they appear to quickly resume feeding dives (usually within minutes based on video footage), suggesting that surface application of suction cup tags has a minimal impact on the turtles' natural behavior (Heaslip et al., 2012; Wallace et al., 2015). The total weight of the transponder, camera and attachment materials was less than 1% of the turtle's minimum estimated body weight of 200 kg. We estimated the turtle's carapace length relative to the known length of the tagging vessel, and used published values of mass vs. curved carapace length (James et al., 2005) to estimate a conservative body weight for the turtle.
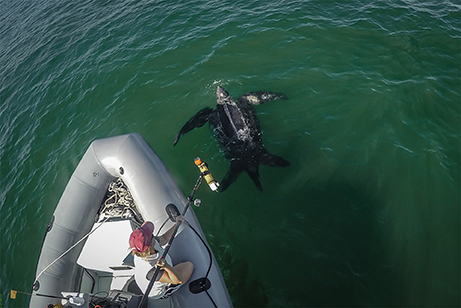
Figure 2. A researcher prepares to tag a leatherback turtle at the surface with a pole-deployed transponder off Cape Cod, Massachusetts, USA. Photo: Sean P. Whelan, used with permission.
We tagged the turtle with the transponder at 14:33 GMT and deployed the TurtleCam AUV at 14:39 GMT. We tracked the turtle until 20:29 GMT, recovering the AUV briefly (from 18:27 to 18:45 GMT) to swap out batteries and memory cards in the vehicle cameras. For this mission, the TurtleCam AUV was equipped with an ADCP, CT probe, pressure sensor, and magnetic heading sensor. For a subset of the total mission (16:32 to 20:29), we also deployed a second REMUS-100 AUV “Edgar” that was equipped with a CTD (Model, SBD 49 FastCAT sensor; www.seabird.com), combo flurometer-turbidity (Model, ECO-Triplet; www.seabird.com) ECO puck sensor, up/down 1,200-kHz RDI ADCP, and 900/1,800-kHz dual frequency sidescan sonar (www.marinesonic.com) to sample throughout the entire water column in the vicinity of the tagged turtle. Our mission generated over 5 h of turtle localization data and video, and 2 h of turtle-borne camera footage. The TurtleCam AUV collected over 5 h of biophysical oceanographic data along the turtle's track, while Edgar collected 4 h of environmental data from the surface to the sea floor near the turtle's path (Figure 3). At the end of the mission, the transponder tag successfully released from the turtle on acoustic command from the STS and was recovered.
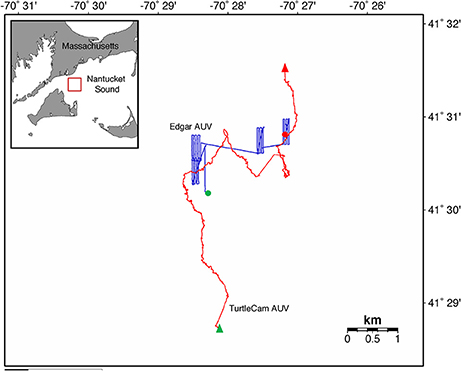
Figure 3. Leatherback turtle track (red line) from beginning (green triangle) to end (red triangle) in Nantucket Sound, Massachusetts, USA in October, 2016. Turtle track reconstructed from the TurtleCam AUV localization data. Track of second AUV “Edgar” (blue line) from beginning (green circle) to end (red circle) near the tracked turtle's path.
Sensor Data
Turtle localization, depth and habitat data were extracted from the AUV using algorithms developed at the WHOI Oceanographic Systems Lab, and sensor data was analyzed in R (R Core Team, 2016). During the 5.5-h tracking period, the turtle's estimated horizontal movements covered approximately 16 km (Figure 3). In this shallow, coastal environment, the turtle dove continuously from the surface to the seafloor, occupying a depth range of 0–20 m (mean ± SD = 8.1 ± 4.7 m) (Figure 4). The seafloor along the turtle's path was a mix of shoal and channel habitat, with bathymetry ranging from 7.2 to 30.7 m (mean ± SD = 15.5 ± 2.9 m) (Figure 4). The water column was well-mixed, with relatively uniform temperature (mean ± SD = 18.4 ± 0.1°C) and salinity (mean ± SD = 32.07 ± 0.03 psu), and a weak or absent mixed layer (Figure 5). We also measured high values of chlorophyll a (mean ± SD = 488.7 ± 58.9 μg/L) and turbidity (mean ± SD = 242.0 ± 24.6 NTU) near the path of the tagged turtle with the second AUV.
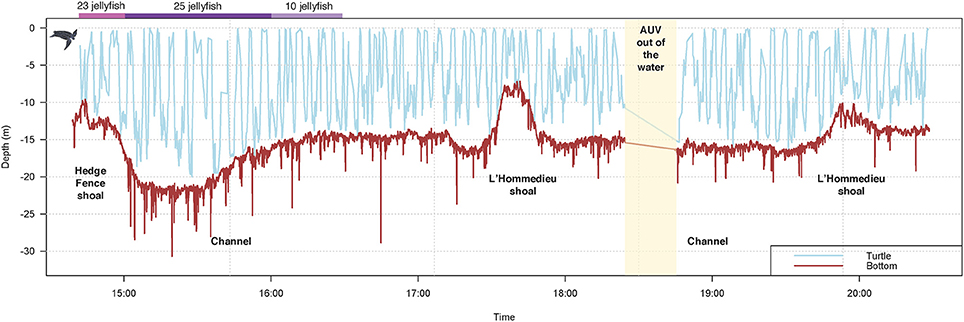
Figure 4. Leatherback turtle depth data, concurrent bathymetry, and jellyfish consumption during a TurtleCam AUV mission in Nantucket Sound in October, 2016. Turtle depth (blue line) was reconstructed from the TurtleCam AUV localization data and pressure sensor. Bathymetry (brown line) along the turtle's track was measured by the TurtleCam AUV with the ADCP (altimeter) and pressure sensor. Jellyfish captures were calculated from turtle-borne camera footage during the first 2 h of the deployment. The AUV was recovered part-way through the mission (yellow column) to replace camera batteries and memory cards.
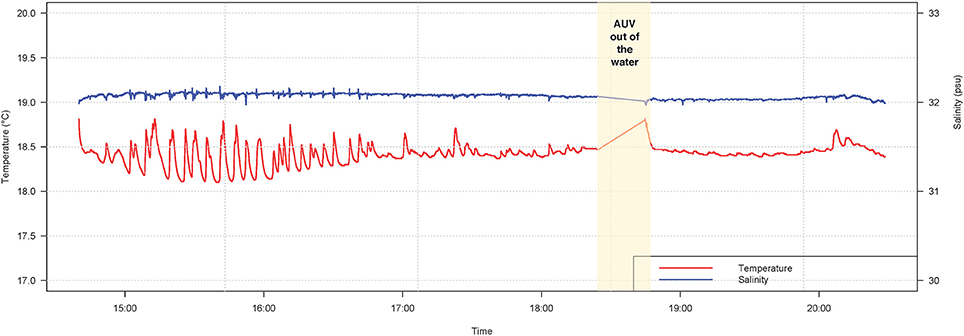
Figure 5. Temperature (red line) and salinity (blue line) during a TurtleCam AUV mission in Nantucket Sound in October, 2016. Environmental data was sampled along the tagged leatherback turtle's path by the AUV with a conductivity-temperature (CT) probe. The AUV was recovered part-way through the mission (yellow column) to replace camera batteries and memory cards.
Video Footage
The video footage from the turtle-borne transponder and AUV cameras was downloaded and backed up on duplicate drives after each mission. The AUV footage from six different cameras was stitched together into a single video mosaic for review (Figure 6). High turbidity in our study site resulted in poor visibility (frequently <1 m), limiting footage of the turtle from the AUV cameras (<1% total time). For this reason, we focused our analysis on the turtle-borne camera footage (Figure 7). We built an ethogram and coded the turtle-borne camera footage using the open-source event-logging software BORIS (Friard and Gamba, 2016). This analysis resulted in a detailed time-activity budget and included state events (frequency and duration) and point events (frequency only). We included modifiers for some parameters to capture additional detail from our observations. Parameters in our ethogram can be found in the Time Diagram (Figure 8).
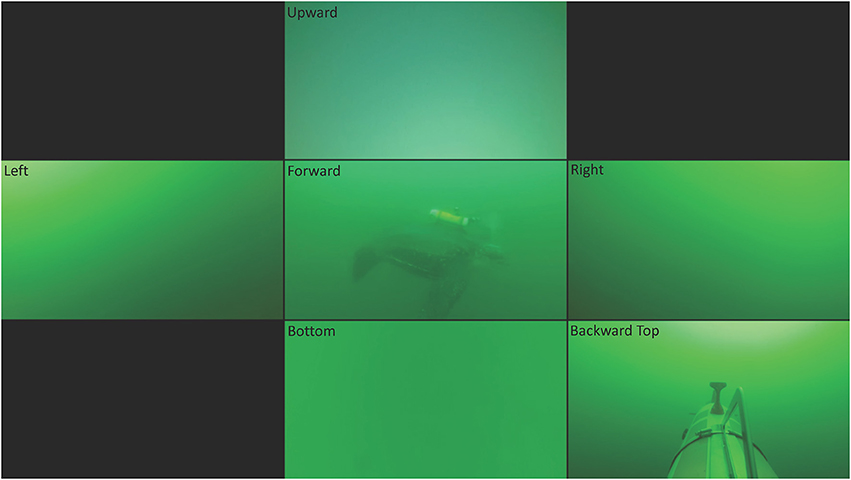
Figure 6. AUV imagery of the tagged leatherback turtle feeding on a sea nettle in the water column in highly turbid conditions. The image mosaic represents the views from all six AUV video cameras, including the cameras in the vehicle nose (upward, forward, bottom, right, and left), and the backward facing camera that was mounted on the vehicle top.
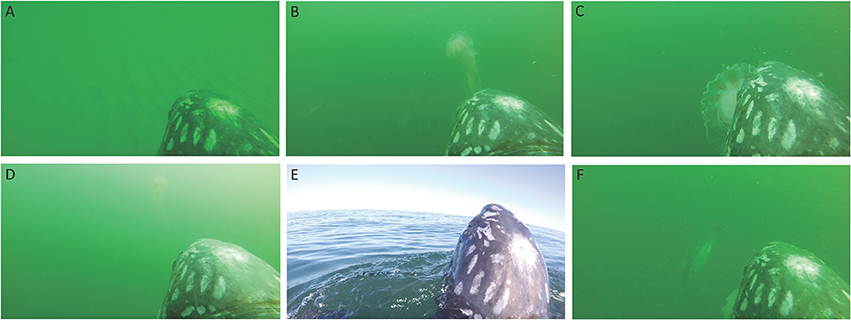
Figure 7. Images from the turtle-borne transponder camera on a leatherback sea turtle. (A) Swimming just above the bottom over sand waves in shoal habitat. (B) Swimming toward a sea nettle jellyfish. (C) Capturing and consuming a sea nettle jellyfish. (D) Silhouetting a sea nettle jellyfish with surface light. (E) Breaking the surface for a breath. (F) False albacore making a close approach after the turtle captures a jellyfish.
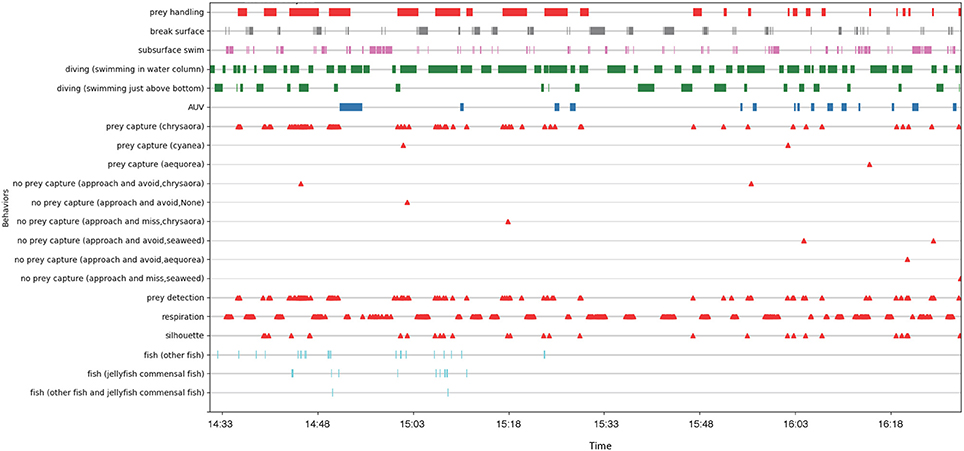
Figure 8. Time diagram for 2 h of turtle-borne camera video footage from one leatherback turtle. Behavior parameters include state events (frequency and duration) and point events (frequency only).
During the ~2 h recording period of the turtle-borne camera, the turtle spent most of its time diving (68%), making 71 dives (Figure 8). Consistent with our turtle depth and bathymetry measurements from the AUV, we observed the turtle using the entire water column, spending almost 16% of its time swimming just above the sea floor (Figure 7A) and occasionally feeding on jellyfish at or near the bottom. We recorded high feeding rates (over 30 jellyfish per hour), with prey captures consisting mostly (95%) of Atlantic sea nettle (Chrysaora quinquecirrha) (Figures 7B,C, 8). Out of the 78 prey detections recorded, 63 jellyfish were successfully captured and the turtle spent 29% of its time handling jellyfish (Figure 7C). The turtle silhouetted its prey 36% of the time by diving to the bottom or just above the bottom, and then looking up toward the surface light to locate prey (Figure 7D). The turtle spent less of its time at the surface (15%) and swimming just under the surface (17%) (Figure 8). It broke the surface 103 times during the tracking period, and surface time was always associated with respirations (n = 180) (Figures 7E, 8).
We also coded the video data for the turtle's reaction, if any, to the AUV, and presence of non-prey species (Figure 8). We recorded 15 apparent reactions to the AUV, which included brief cessation of feeding and movement away (toward the surface if it occurred at depth and toward the bottom if it occurred at the surface), as well as defensive postures (turning its carapace toward the AUV). The first observed reaction was the longest at 3.5 min, while the subsequent reactions were brief (mean = 0.6 min) with the turtle recovering quickly and resuming its diving and feeding behavior (Figure 8). This suggests the turtle may have acclimated to the presence of the AUV over time. We recorded the presence of fish, identified mostly as false albacore Euthynnus alletteratus (Figure 7F) and jellyfish-commensal larval butterfish Peprilus triacanthus, but this was infrequent (<4% of the recording time) (Figure 8). Although we tracked and filmed the turtle within meters of pot gear, the turbidity and limited visibility precluded collection of underwater footage of the gear.
AUV Applications
To mitigate threats to animals in the marine environment, it is critical to identify behaviors that exacerbate risk. Characterization of sea turtle behavior is often based on indirect measurements and inference, resulting in an incomplete and potentially inaccurate picture of behavior (Seminoff et al., 2006). Direct visual observations are critical to improve and validate interpretation of indirect behavior measurements (Schofield et al., 2006; Seminoff et al., 2006). To correctly interpret habitat-driven behaviors, we also need to concurrently observe and sample habitat during behavior studies. Autonomous underwater vehicles can efficiently meet all of these objectives, resulting in a more holistic picture of marine animal behavior (Packard et al., 2013; Kukulya et al., 2015, 2016; Skomal et al., 2015). The pilot study described here demonstrates proof of concept for using an AUV to study leatherback turtle behavior and habitat in a densely populated, high-risk coastal environment, and it can be easily adapted for other species and habitats with similar conservation concerns.
The “smart” AUV SharkCam has already demonstrated the utility of using versatile autonomous vehicles to study the behavior of large pelagic animals such as great white sharks (Carcharodon carcharius) (Skomal et al., 2015). Data collected from previous SharkCam studies showed that great white sharks spend the majority of their time swimming in a straight line at a constant speed (Kukulya et al., 2015, 2016; Skomal et al., 2015). Our leatherback turtle study demonstrated that REMUS AUV technology is also capable of making observations of an obligate air breather that dives frequently, is less predictable in its swimming trajectory, and frequently surfaces and hovers. “Smart” AUVs like SharkCam and TurtleCam, which can track a randomly moving target, film it and collect a variety of oceanographic data, offer a revolutionary tool to scientists investigating the subsurface behaviors and habitat of marine megafauna. Future applications for “smart” AUVs include behavior and habitat studies of whales, seals, rays, skates, tuna, and a variety of sea turtles and sharks. As AUV technology improves and tags become miniaturized, the demand and applications for animal-following AUVs will continue to grow and evolve, transforming the ways that scientists can study cryptic marine species.
Summary and Conclusions
We successfully used the TurtleCam system to simultaneously measure and observe leatherback turtle habitat and behavior. Our findings have direct implications for conservation and management of leatherback turtles off Massachusetts and in regions with similar bio-physical oceanographic conditions. The combination of sensor and video data demonstrated that the tagged turtle fed in productive, turbid, and well-mixed habitat associated with shallow (<35 m) shoal and channel bathymetry. In these conditions, the turtle used the entire water column and fed on jellyfish from the seafloor to just under the surface. Jellyfish are known to accumulate around physical gradients (Graham et al., 2001), and the absence of a pycnocline in our well-mixed study site is consistent with jellyfish distribution throughout the water column. In foraging areas with a well-defined pycnocline (e.g., Atlantic Canada), leatherbacks appear to limit their diving to the upper mixed layer, and depth-specific fishing gear modifications may reduce sea turtle entanglement in buoy lines (Hamelin et al., 2014). In the shallow well-mixed habitat off Cape Cod, leatherback turtles are likely to feed throughout the entire water column and can encounter fishing gear anywhere from the surface to the sea floor, making depth-specific fishing gear modifications to reduce sea turtle interactions ineffective. The tagged turtle also exhibited prey-silhouetting behavior, which has been documented in great white sharks (Klimley et al., 1996; Strong, 1996; Skomal et al., 2015), and in leatherback turtles off Atlantic Canada (Wallace et al., 2015). While sharks silhouette their evasive pinniped prey as an ambush tactic, visual predators like leatherbacks may silhouette jellyfish with surface light in response to the murky, turbid conditions in our study area. Prey silhouetting could potentially increase the risk of entanglement in buoy lines of fixed fishing gear if the turtle mistakes a surface buoy or submerged float for their jellyfish prey. While the leatherback was able to correctly identify jellyfish the majority of the time, it did make a close approach to seaweed on three occasions (Figure 8). Entanglement risk may be exacerbated by poor visibility coupled with the turtles' primary focus on prey capture/handling.
The tagged turtle only spent 15% of its time at the surface, but the frequency of surfacing (>100 times in ~2 h) may increase its probability of boat strike. Surface and subsurface swimming (within the top 2 m) accounted for about one third of the turtle's observed behaviors, putting the turtle within easy striking distance of the hull and/or propeller of a range of watercraft (Hazel and Gyuris, 2006). Vessels operating in our study area range from shallow draft (≤1.5 m) recreational vessels to medium draft (3–5 m) passenger ferries, fishing vessels, and yachts (reviewed in https://energy.gov/sites/prod/files/DOE-EIS-0470-Cape_Wind_FEIS_2012.pdf). This is consistent with annual documentation of boat-struck leatherbacks in our study region (Dwyer et al., 2002), though forensic analysis is needed to identify the primary source(s) of vessel-related mortalities (e.g., Rommel et al., 2007). Interestingly, the tagged turtle did not appear to show an overt behavioral response to the presence (visual or acoustic) of the tracking boat or other vessels in the area, though this assessment was subjective. To quantify behavioral response to sound, future deployments should include an acoustic tag (e.g., Tyson et al., 2017) and incorporate behavioral response studies. More research is needed on the behavioral response of sea turtles to anthropogenic underwater sounds, especially field studies that measure behavioral response experimentally as has been done for marine mammals (Southall et al., 2016).
Data from TurtleCam can also be used to improve energy budget calculations for leatherback turtles and to help define fine-scale foraging habitat requirements. Video imagery can be used to quantify flipper beat frequency, or stroke rate (Reina et al., 2005), which can be a proxy of energy expenditure in some marine animals (Williams et al., 2004; Jeanniard-du-Dot et al., 2016). Stroke rate data can be combined with video-derived time-activity budgets to estimate activity-specific energy budgets for leatherbacks. In addition to stroke rate, TurtleCam also monitored feeding behavior, including prey composition, consumption rate, and abundance, key parameters in bioenergetics models (Chipps and Wahl, 2008). TurtleCam continuously collected imagery and sensor information on the turtle's immediate environment, including both biotic (chlorophyll a, turbidity) and abiotic (bathymetry, temperature, salinity) properties, allowing us to identify and characterize leatherback habitat requirements within a coastal feeding ground.
Limitations of the current TurtleCam system include relatively short tracking durations (≤8 h), the size of the transponder, and the potential to affect the focal animal's behavior. Larger AUVs (e.g., the REMUS-600; depth-rated to 600 m, 211 × 32 cm, 235 kg in air) have greater battery power and can carry more instruments and lights for overnight deployments, but the larger vehicle size increases the costs associated with deployment and operation. The REMUS-100 can be deployed by two individuals from the side of a small vessel, making it a versatile and cost-effective choice for field studies with limited budgets. The current transponder size (7.6 × 38 cm, 1.7 kg in air, ~58 g buoyancy in seawater) limits its application to relatively large marine animals that can handle the added weight and drag of the instrument. The size and associated drag of the transponder may also impact swimming and diving behavior (Jones et al., 2013). The WHOI Oceanographic Systems Laboratory is in the process of re-engineering the transponder to be more compact and hydrodynamic (~60% reduction in size and weight), reducing potential impact to natural behaviors and increasing its suitability for other species. Future improvements to the transponder also include incorporating scientific sensors and onboard data storage.
In our study, the attachment of the transponder via suction cups did not appear to have any measurable impact on subsequent behavior, but for species that require handling for tag attachment, there may be a “capture” stress response that can last for several hours (Heaslip et al., 2012; Thomson and Heithaus, 2014). The video footage allowed us to objectively measure the turtle's response to the presence of the AUV. Given the turbidity and limited visibility in our study site, we used a follow distance of 1–2 m between the turtle and the AUV to maximize footage of the turtle. This small distance likely resulted in heightened turtle response to the presence of the AUV, with the turtle appearing to react to the AUV during close follows by modifying its behavior to avoid it by swimming away and/or presenting a defensive posture (turning its carapace toward it). However, the turtle appeared to recover quickly from these events and resume normal diving and feeding behavior. In less turbid conditions with better visibility, a larger follow distance can be maintained and would likely result in fewer reactions from the turtle.
The TurtleCam AUV system is a unique platform that enables researchers to directly observe and study the subsurface behaviors and habitats of marine megafauna. It is a highly versatile platform that can be customized to meet different study objectives through its range of sensors and adaptability for different animal behaviors (e.g., high frequency diving vs. horizontal swimming). In densely populated coastal habitats where endangered and threatened species overlap with multiple anthropogenic threats, understanding animal behavior and habitat is critical to designing and implementing effective mitigation strategies. Our study demonstrates that an AUV can successfully track and image leatherback sea turtles feeding in a coastal environment, resulting in new observations of three-dimensional subsurface behaviors and habitat use, with direct implications for sea turtle management and conservation.
Ethics Statement
This study was carried out in accordance with the recommendations of the National Marine Fisheries Service Endangered Species Act Section 10 Permit no. 15672-02. The protocol was approved by the Woods Hole Oceanographic Institution Institutional Animal Care and Use Committee (IACUC) no. 21668.
Author Contributions
KD and AK conceived and designed the study. KD, AK, EB, and MB contributed to obtaining funding, the acquisition, analysis, or interpretation of the data, and to drafts and revisions of the manuscript.
Funding
This research was funded by National Oceanic and Atmospheric Administration Grant #NA16NMF4720074 to the Massachusetts Division of Marine Fisheries under the Species Recovery Grants to States program. Additional funding was provided by Jean Tempel, Hydroid Inc., and over 100 Project WHOI donors.
Conflict of Interest Statement
The authors declare that the research was conducted in the absence of any commercial or financial relationships that could be construed as a potential conflict of interest.
Acknowledgments
For assistance in the field, we thank George Purmont, Michael Dodge, Sean Whelan, Ken Kostel, and Daniel Cojanu. We thank Roger Stokey and the Oceanographic Systems Laboratory for providing REMUS AUV support. We are grateful to Karen Moore Dourdeville and Mass Audubon Wellfleet Bay Wildlife Sanctuary for providing real-time sea turtle sightings information during our field seasons (www.seaturtlesightings.org). We thank Jack Cook and Natalie Renier (WHOI Graphic Services) for assistance with Figure 1. The authors acknowledge the use of SEATURTLE.ORG's Maptool program (www.seaturtle.org/maptool/). Special thanks to Jean Tempel, Hydroid, Inc., and over 100 Project WHOI donors who provided the seed funding for this work. We thank Larisa Avens and Sabrina Fossette for helpful comments that improved an earlier draft of this manuscript.
References
Avens, L., Braun-McNeill, J., Epperly, S., and Lohmann, K. J. (2003). Site fidelity and homing behavior in juvenile loggerhead sea turtles (Caretta caretta). Mar. Biol. 143, 211–220. doi: 10.1007/s00227-003-1085-9
Bartol, S. M., Musick, J. A., and Lenhardt, M. L. (1999). Auditory evoked potentials of the loggerhead sea turtle (Caretta caretta). Copeia 1999, 836–840. doi: 10.2307/1447625
Bjorndal, K. A., Bolten, A. B., and Lagueux, C. J. (1994). Ingestion of marine debris by juvenile sea turtles in coastal Florida habitats. Mar. Poll. Bull. 28, 154–158. doi: 10.1016/0025-326X(94)90391-3
Brooks, L. B., Harvey, J. T., and Wallace, W. J. (2009). Tidal movements of the East Pacific green turtles Chelonia mydas at a foraging ground in Baja California Sur, México. Mar. Ecol. Prog. Ser. 386, 263–274. doi: 10.3354/meps08061
Casale, P., Affronte, M., Insacco, G., Freggi, D., Vallini, C., D'Astore, P. P., et al. (2010). Sea turtle strandings reveal high anthropogenic mortality in Italian waters. Aquatic Conserv. 20, 611–620. doi: 10.1002/aqc.1133
Chipps, S. R., and Wahl, D. H. (2008). Bioenergetics modeling in the 21st century: reviewing new insights and revisiting old constraints. Trans. Am. Fish. Soc. 137, 298–313. doi: 10.1577/T05-236.1
Dodge, K. L., Galuardi, B., Miller, T. J., and Lutcavage, M. E. (2014). Leatherback turtle movements, dive behavior and habitat characteristics in ecoregions of the Northwest Atlantic Ocean. PLoS ONE 9:e91726. doi: 10.1371/journal.pone.0091726
Dow-Piniak, W. E., Eckert, S. A., Harms, C. A., and Stringer, E. M. (2012). Underwater Hearing Sensitivity of the Leatherback Sea Turtle (Dermochelys Coriacea): Assessing the Potential Effect of Anthropogenic Noise. Herndon, VA: U.S. Department of the Interior, Bureau of Ocean Energy Management, Headquarters, OCS Study BOEM 2012-01156, 35.
Dwyer, K. L., Ryder, C. E., and Prescott, R. (2002). “Anthropogenic mortality of leatherback turtles in Massachusetts waters,” in Proceedings of the Twenty-Second Annual Symposium on Sea Turtle Biology and Conservation (Miami, FL: United States Department of Commerce, NOAA Technical Memorandum NMFS-SEFSC-503), 260.
Fossette, S., Gleiss, A. C., Myers, A. E., Garner, S., Liebsch, N., Whitney, N. M., et al. (2010). Behaviour and buoyancy regulation in the deepest-diving reptile: the leatherback turtle. J. Exp. Biol. 213, 4074–4083. doi: 10.1242/jeb.048207
Friard, O., and Gamba, M. (2016). BORIS: a free, versatile open-source event-logging software for video/audio coding and live observations. Methods Ecol. Evol. 7, 1325–1330. doi: 10.1111/2041-210X.12584
Graham, W. M., Pages, F., and Hammer, W. M. (2001). A physical context for gelatinous zooplankton aggregations: a review. Hydrobiologia 451, 199–212. doi: 10.1023/A:1011876004427
Hamelin, K. M., James, M. C., Ledwell, W., Huntington, J., and Martin, K. (2017). Incidental capture of leatherback sea turtles in fixed fishing gear off Atlantic Canada. Aquatic Conserv. 27, 631–642. doi: 10.1002/aqc.2733
Hamelin, K. M., Kelley, D. E., Taggart, C. T., and James, M. C. (2014). Water mass characteristics and solar illumination influence leatherback turtle dive patterns at high latitudes. Ecosphere 5:19. doi: 10.1890/ES13-00158.1
Hays, G. C., Hobson, V. J., Metcalfe, J. D., Righton, D., and Sims, D. W. (2006). Flexible foraging movements of leatherback turtles across the North Atlantic ocean. Ecology 87, 2647–2656. doi: 10.1890/0012-9658(2006)87[2647:FFMOLT]2.0.CO;2
Hazel, J., and Gyuris, E. (2006). Vessel-related mortality of sea turtles in Queensland, Australia. Wildl. Res. 33, 149–154. doi: 10.1071/WR04097
Hazel, J., Lawler, I. R., and Hamann, M. (2009). Diving at the shallow end: green turtle behavior in near-shore foraging habitat. J. Exp. Mar. Biol. Ecol. 371, 84–92. doi: 10.1016/j.jembe.2009.01.007
Heaslip, S. G., Iverson, S. J., Bowen, W. D., and James, M. C. (2012). Jellyfish support high energy intake of leatherback sea turtles (Dermochelys coriacea): video evidence from animal-borne cameras. PLoS ONE 7:e33259. doi: 10.1371/journal.pone.0033259
Heithaus, M. R., McLash, J. J., Frid, A., Dill, L. M., and Marshall, G. J. (2002). Novel insights into green sea turtle behaviour using animal-borne video cameras. J. Mar. Bol. Ass. U.K. 82, 1049–1050. doi: 10.1017/S0025315402006689
James, M. C., Ottensmeyer, C. A., and Myers, R. A. (2005). Identification of high-use habitat and threats to leatherback sea turtles in northern waters: new directions for conservation. Ecol. Lett. 8, 195–201. doi: 10.1111/j.1461-0248.2004.00710.x
Jeanniard-du-Dot, T., Trites, A. W., Arnould, J. P., Speakman, J. R., and Guinet, C. (2016). Flipper strokes can predict energy expenditure and locomotion costs in free-ranging northern and Antarctic fur seals. Sci. Rep. 6:33912. doi: 10.1038/srep33912
Jones, T. T., Van Houtan, K. S., Bostrom, B. L., Ostafichuk, P., Mikkelsen, J., Tezcan, E., et al. (2013). Calculating the ecological impacts of animal-borne instruments on aquatic organisms. Methods Ecol. Evol. 4, 1178–1186. doi: 10.1111/2041-210X.12109
Klimley, A. P., Pyle, P., and Anderson, S. D. (1996). “The behaviour of white sharks and their pinniped prey during predatory attacks,” in Great White Sharks: the Biology of Carcharodon carcharias, eds A. P. Klimley and D. G. Ainley (San Diego, CA: Academic Press), 175–191.
Kukulya, A. L., Stokey, R., Fiester, C., Hoyos-Padilla, E. M., and Skomal, G. (2016). “Multi-vehicle autonomous tracking and filming of white sharks Carcharodon carcharias,” in Autonomous Underwater Vehicles (AUV), 2016 IEEE/OES (Tokyo), 423–430. doi: 10.1109/AUV.2016.7778707
Kukulya, A. L., Stokey, R., Jaffre, F., Hoyos-Padilla, M. E., and Skomal, G. B. (2015). “3D real-time tracking, following and imaging of white sharks with an autonomous underwater vehicle,” in Proceedings of Oceans'15 (Genova), 18–21.
Lazell, J. D. (1980). New England waters: critical habitat for marine turtles. Copeia 1980, 290–295. doi: 10.2307/1444006
Lutcavage, M. E., Plotkin, P., Witherington, B., and Lutz, P. (1996). “Human impacts on sea turtle survival,” in The Biology of Sea Turtles, eds P. Lutz and J. Musick (Boca Raton, FL: CRC Press), 387–409.
Mrosovsky, N., Ryan, G. D., and James, M. C. (2009). Leatherback turtles: the menace of plastic. Mar. Poll. Bull. 58, 287–289. doi: 10.1016/j.marpolbul.2008.10.018
Packard, G. E., Kukulya, A., Austin, T., Dennett, M., Littlefield, R., Packard, G., et al. (2013). “Continuous autonomous tracking and imaging of white sharks and basking sharks using a REMUS-100 AUV,” in Proceedings of Oceans'13 (San Diego, CA).
Patel, S. H., Dodge, K. L., Haas, H. L., and Smolowitz, R. J. (2016). Videography reveals in-water behavior of loggerhead turtles (Caretta caretta) at a foraging ground. Front. Mar. Sci. 3:254. doi: 10.3389/fmars.2016.00254
Peckham, S. H., Maldonado Diaz, D., Walli, A., Ruiz, G., Crowder, L. B., and Nichols, W. J. (2007). Small-scale fisheries bycatch jeopardizes endangered Pacific loggerhead turtles. PLoS ONE 2:e1041. doi: 10.1371/journal.pone.0001041
R Core Team (2016). R: A Language and Environment for Statistical Computing. Vienna: R Foundation for Statistical Computing. Available online at: https://www.r-project.org/
Reina, R. D., Abernathy, K. J., Marshall, G. J., and Spotila, J. R. (2005). Respiratory frequency, dive behaviour and social interactions of leatherback turtles, Dermochelys coriacea during the inter-nesting interval. J. Exp. Mar. Ecol. Biol. 316, 1–16. doi: 10.1016/j.jembe.2004.10.002
Rommel, S. A., Costidis, A. M., and Pitchford, T. D. (2007). Forensic methods for characterizing watercraft from watercraft-induced wounds on the Florida manatee (Trichechus manatus latirostris). Mar. Mamm. Sci. 23, 110–132. doi: 10.1111/j.1748-7692.2006.00095.x
Sampson, K. A. (2012). “Entanglement of sea turtles in vertical lines of fixed gear fisheries: a summary of the geographic range, seasonality, involved species and nature of reported entanglement events in the Northeast region of the United States,” in Proceedings of the Thirty-First Annual Symposium on Sea Turtle Biology and Conservation (San Diego, CA: United States Department of Commerce, NOAA Technical Memorandum NMFS-SEFSC-631), 288.
Schofield, G., Bishop, C. M., Katselidis, K. A., Dimopoulos, P., Pantis, J. D., and Hays, G. C. (2009). Microhabitat selection by sea turtles in a dynamic thermal marine environment. J. Anim. Ecol. 78, 14–21. doi: 10.1111/j.1365-2656.2008.01454.x
Schofield, G., Katselidis, K. A., Dimopoulos, P., Pantis, J. D., and Hays, G. C. (2006). Behaviour analysis of the loggerhead sea turtle Caretta caretta from direct in-water observation. Endang. Spec. Res. 2, 71–79. doi: 10.3354/esr002071
Seminoff, J. A., Jones, T. T., and Marshall, G. J. (2006). Underwater behaviour of green turtles monitored with video-time-depth recorders: what's missing from dive profiles? Mar. Ecol. Prog. Ser. 322, 269–280. doi: 10.3354/meps322269
Seminoff, J. A., Resendiz, A., and Nichols, W. J. (2002). Home range of green turtles Chelonia mydas at a coastal foraging area in the Gulf of California, Mexico. Mar. Ecol. Prog. Ser. 242, 253–265. doi: 10.3354/meps242253
Skomal, G. B., Hoyos-Padilla, E. M., Kukulya, A., and Stokey, R. (2015). Subsurface observations of white shark Carcharodon carcharias predatory behaviour using an autonomous underwater vehicle. J. Fish Biol. 87, 1293–1312. doi: 10.1111/jfb.12828
Southall, B. L., Nowacek, D. P., Miller, P. J. O., and Tyack, P. L. (2016). Experimental field studies to measure behavioral responses of cetaceans to sonar. Endang. Species Res. 31, 293–315. doi: 10.3354/esr00764
Southwood, A. L., Andrews, R. D., Lutcavage, M. E., Paladino, F. V., West, N. H., George, R. H., et al. (1999). Heart rates and diving behavior of leatherback sea turtles in the eastern Pacific ocean. J. Exp. Biol. 202, 1115–1125.
Strong, W. R. Jr. (1996). “Shape discrimination and visual predatory tactics in white sharks,” in Great White Sharks: The Biology of Carcharodon carcharias, eds A. P. Klimley and D. G. Ainley (San Diego, CA: Academic Press), 175–191.
Thomson, J. A., and Heithaus, M. R. (2014). Animal-borne video reveals seasonal activity patterns of green sea turtles and the importance of accounting for capture stress in short-term biologging. J. Exp. Mar. Biol. Ecol. 450, 15–20. doi: 10.1016/j.jembe.2013.10.020
Tomás, J., Gozalbes, P., Raga, J. A., and Godley, B. J. (2008). Bycatch of loggerhead sea turtles: insights from 14 years of stranding data. Endang. Species Res. 5, 161–169. doi: 10.3354/esr00116
Tyson, R. B., Piniak, W. E. D., Domit, C., Mann, D., Hall, M., Nowacek, D. P., et al. (2017). Novel bio-logging tool for studying fine-scale behaviors of marine turtles in response to sound. Front. Mar. Sci. 4:219. doi: 10.3389/fmars.2017.00219
van Dam, R. P., and Diez, C. E. (1998). Home range of immature hawksbill turtles (Eretmochelys imbricata) (Lannaeus) at two Caribbean islands. J. Exp. Mar. Biol Ecol. 220, 15–24. doi: 10.1016/S0022-0981(97)00080-4
Wallace, B. P., Zolkewitz, M., and James, M. C. (2015). Fine-scale foraging ecology of leatherback turtles. Front. Ecol. Evol. 3:15. doi: 10.3389/fevo.2015.00015
Keywords: autonomous underwater vehicle AUV, CTD, entanglement, habitat, foraging behavior, jellyfish, leatherback sea turtle, video camera
Citation: Dodge KL, Kukulya AL, Burke E and Baumgartner MF (2018) TurtleCam: A “Smart” Autonomous Underwater Vehicle for Investigating Behaviors and Habitats of Sea Turtles. Front. Mar. Sci. 5:90. doi: 10.3389/fmars.2018.00090
Received: 03 January 2018; Accepted: 05 March 2018;
Published: 20 March 2018.
Edited by:
Lisa Marie Komoroske, University of Massachusetts Amherst, United StatesReviewed by:
Larisa Avens, Southeast Fisheries Science Center (NOAA), United StatesSabrina Fossette, National Oceanic and Atmospheric Administration (NOAA), United States
Copyright © 2018 Dodge, Kukulya, Burke and Baumgartner. This is an open-access article distributed under the terms of the Creative Commons Attribution License (CC BY). The use, distribution or reproduction in other forums is permitted, provided the original author(s) and the copyright owner are credited and that the original publication in this journal is cited, in accordance with accepted academic practice. No use, distribution or reproduction is permitted which does not comply with these terms.
*Correspondence: Kara L. Dodge, kdodge@whoi.edu