- Centre for Public Health, Queen’s University Belfast, Belfast, United Kingdom
Chronic kidney disease (CKD) was the 12th leading cause of death globally in 2017 with the prevalence of CKD estimated at ~9%. Early detection and intervention for CKD may improve patient outcomes, but standard testing approaches even in developed countries do not facilitate identification of patients at high risk of developing CKD, nor those progressing to end-stage kidney disease (ESKD). Recent advances in CKD research are moving towards a more personalised approach for CKD. Heritability for CKD ranges from 30% to 75%, yet identified genetic risk factors account for only a small proportion of the inherited contribution to CKD. More in depth analysis of genomic sequencing data in large cohorts is revealing new genetic risk factors for common diagnoses of CKD and providing novel diagnoses for rare forms of CKD. Multi-omic approaches are now being harnessed to improve our understanding of CKD and explain some of the so-called ‘missing heritability’. The most common omic analyses employed for CKD are genomics, epigenomics, transcriptomics, metabolomics, proteomics and phenomics. While each of these omics have been reviewed individually, considering integrated multi-omic analysis offers considerable scope to improve our understanding and treatment of CKD. This narrative review summarises current understanding of multi-omic research alongside recent experimental and analytical approaches, discusses current challenges and future perspectives, and offers new insights for CKD.
Introduction
Within the last decade, studies have generated a wealth of biological data by exploring the human ‘omes’; from genomics and epigenomics which explore gene variation and modification, transcriptomics which explores gene expression, proteomics and metabolomics which explore the abundance of key biological molecules, to phenomics which explores the potential outcomes or consequences of such biological changes. The valuable insights gained by integrating multiple omic technologies (via multi-omics) have improved our fundamental understanding of complex cellular processes, and highlighted how these processes become disrupted during disease. Multi-omic studies have facilitated exploratory analysis of human ‘omes’, improved our basic understanding of their individual function and highlighted important intricate interactions. This knowledge has been harnessed to aid the development of disease biomarkers, the diagnosis of rare disease, the identification of novel drug targets, the design of precision or personalised medicine, and the prediction of disease risk at a population level (1–5).
Multi-omic analyses have been harnessed to improve our understanding of chronic kidney disease (CKD) (Figure 1). CKD is a non-communicable disease with increasing prevalence worldwide. In 1990, CKD was the 17th leading cause of death, rising to the 12th leading cause of death by 2017, with 697.5 million cases globally that year (6, 7). Further increases in CKD prevalence are expected, with this disease predicted to become the 5th leading cause of death by 2040 (8). In 2017, diabetic kidney disease (DKD) was the leading cause of CKD (7), and in 2018, diabetes accounted for 40% of incident end-stage kidney disease (ESKD) cases in the USA (9). Diabetes is also increasing in incidence worldwide (10, 11), and this, together with increasing prevalence of CKD, is reflective of the aging global population (6). Goal 3 of the United Nations sustainable development goals includes an aim to reduce premature mortality from non-communicable diseases by one third by 2030, with decreasing CKD disease burden highlighted as an important factor in reaching that target (6, 12).
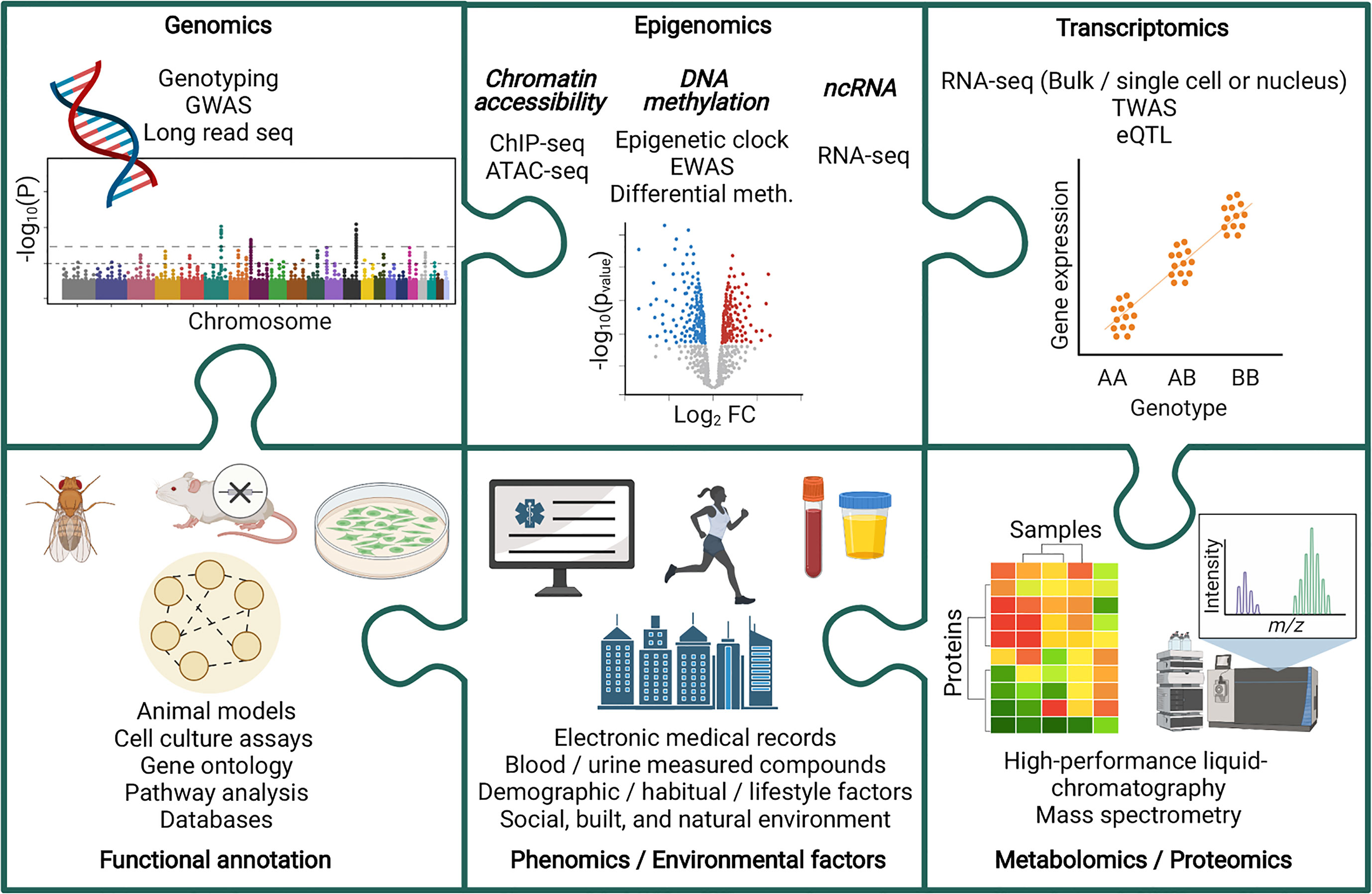
Figure 1 Piecing together the multi-omic puzzle to facilitate the study of chronic kidney disease. ATAC-seq, Assay for Transposase-Accessible Chromatin sequencing; ChIP-seq, Chromatin immunoprecipitation sequencing; eQTL, Expression quantitative trait loci; EWAS, Epigenome wise association study; GWAS, Genome wide association study; Meth, Methylation; ncRNA, non-coding RNA; TWAS, Transcriptome wide association study.
Harnessing a multi-omic approach for the study of CKD is valuable due to the sophisticated contributions from many factors, such as genetic, biological, environmental, lifestyle, social and demographic, to the onset and progression of this disease. Moreover, whilst studying each class of factors individually can provide meaningful insights, proper integration of datasets via multi-omic analyses advances our understanding how this network of factors interact to ultimately disrupt biological pathways and influence disease pathology. Advancing our understanding of these complex systems, and how they become disrupted during CKD, has provided opportunities to improve diagnostics, advance treatment strategies, and deepened our fundamental understanding of the causes and effects of CKD, ultimately leading towards our ability to significantly reduce the global impact of this disease.
Multi-Omics in the Study of Chronic Kidney Disease
The typical five-stage CKD classification system highlights the heterogeneity of this disease, with both genetic and environmental (i.e. encompassing built, natural and social environments factors) influencing CKD onset and progression. Multi-omic analyses have been harnessed to improve our understanding of CKD pathogenesis and progression, as well as how these processes vary between patients, with the ultimate goal of advancing patient care, prioritising resources, and improving patient outcomes.
Measuring the Influence of Genetic Variation on CKD Pathogenesis and Progression via Genomic Analysis
CKD heritability has been estimated between 30% and 75%, depending on the measure of kidney function analysed, such as Glomerular filtration rate (GFR), creatinine clearance or albuminuria; varying also due to the influence of kidney disease risk factors, such as diabetes and hypertension (13–16). Moreover, heritability estimates can vary between studies due to the differences in ethnicity, measurement methods and environment (17). Zhang et al. recently highlighted a knowledge gap in the determination of heritability estimates for CKD (17), identifying that most estimates were determined using familial aggregation in late stage CKD cases only (18–21). These authors presented a large (approximately 150,000 participants of predominantly European ancestry), family-based study of kidney function and carried out the first familial clustering analysis of CKD to include early stages of disease, reporting narrow-sense (additive) heritability estimates ranging from approximately 20% to 50% (17). Interestingly, these authors also reported that those with an affected first-degree relative presented a 3-fold higher risk of CKD compared to the general population, independent of risk factors such as hypertension or diabetes. Those with an affected spouse presented a 1.56-fold higher risk (17). This study highlighted the influence of genetic factors on CKD risk, as well as contributions from environmental influences.
Heritability analyses are useful for guiding the estimates of how much phenotypic variation can be attributed to genetic changes (17). Up to approximately 30% of CKD cases have been attributed to monogenic (single-gene) mutations with strong phenotypic effects; often resulting in early-onset disease (22). More common forms of CKD have been attributed to polygenetic (multiple gene) mutations which cumulatively contribute to kidney function decline; with these patients often presenting with adult-onset disease, subject to variation due to environmental influences (22). Large numbers of genome-wide association studies (GWAS) have been carried out to identify significant associations between specific genetic variants (often single nucleotide polymorphisms, or SNPs) and kidney function or disease (23), recently reviewed by Tin and Köttgen (24). These studies have identified over 250 highly reproducible genetic loci, in both European and non-European populations, associated with GFR (24, 25). Additional GWAS have since been published reporting further genetic variants associated with kidney function (26, 27). For example, Stanzick et al. harnessed a dataset of over 1.2 million individuals to expand the number of genetic loci associated with GFR to 424, with variation within these loci explaining 9.8% variance observed in GFR measurements (26). Zhang et al., however, estimated GFR heritability to be 44% (17), highlighting the phenomenon of missing heritability.
Missing heritability in CKD has recently been discussed by Cañadas-Garre et al. (16) and Anderson et al. (28), with these authors exploring how genomic features such as rare variants, copy number variation (CNV), telomeres, mitochondrial DNA and sex chromosome variation may also contribute to the onset and progression of CKD, accounting for some of this missing heritability. These features have been less extensively studied compared to common autosomal genetic variants. This is largely because the GWAS method is most appropriate for identifying common variants (present in more than 1% to 5% of the population) with moderate effect sizes, which cumulatively contribute towards common phenotypic changes (29, 30). This ‘common disease, common variant’ hypothesis is a fundamental basis of GWAS (29, 31–33). Additionally, rare variants or variants on sex chromosomes have decreased coverage in sequencing arrays commonly used for GWAS analyses, with the added limitation of decreased power when performing sex-specific or rare variant analyses (29, 34). More recently, long read sequencing has provided a promising opportunity to undertake more comprehensive exploration of missing heritability, providing insights into structural and rare variants (35, 36), as well as facilitating adaptive sampling to enrich for sex chromosome analysis (37). Zuk et al., however, propose that due to the influence of genetic interactions, which are often not considered during heritability calculations, the level of missing heritability may in fact be over-estimated (38). This is an important consideration when undertaking genomic analysis, however, this does not invalidate the search for further genetic variants significantly associated with a particular phenotype, but it does highlight the importance of determining the biological function of genetic variants in influencing health and disease, to ultimately improve prevention, diagnosis and treatment (38).
Identifying genetic variants significantly associated with kidney function and disease has also unlocked the potential to explore the causal effects of modifiable risk factors with known genetic associations on these kidney function outcomes. Using Mendelian Randomization methods (39), causal associations between kidney function and factors such as telomere length (40–42), hormone levels (43, 44), coffee consumption (45), macronutrient intake (46), physical activity or sedentary behaviours (47), and education have been identified (48). These studies have great scope to inform about behavioural and environmental changes which may reduce the risk of CKD, or slow disease progression; potentially aiding the discovery of novel ways to moderate the global impact of this disease.
Epigenetic Modifications Provide an Additional Layer of Variation Influencing CKD Pathogenesis and Progression
Beyond the study of genetic variants which contribute to the onset and progression of diseases such as CKD, the contribution of epigenetic variation has been investigated (23, 49, 50). Epigenetics classically defines changes in gene expression which are not the result of gene mutations but are heritable in the absence of the signal which initiated the change (51). Epigenetics is a broad term commonly used to describe the study of DNA methylation, histone modifications, and non-coding RNA (ncRNA); however, no mechanistic evidence currently exists to confirm the heritability or self-perpetuating capabilities of histone modifications or ncRNA (51–53). DNA methylation of the fifth position of cytosine (5mC) is the most commonly studied epigenetic modification, with these changes having the potential to be both heritable and dynamic in response to stimuli (54–56).
DNA methylation is commonly found within, but not limited to, CpG sites; regions of DNA where a Cytosine residue is followed by a Guanine residue. Of the approximate 29 million CpG sites in the human genome, 60 to 80% are methylated (56). CpG sites are not evenly distributed across the human genome, they accumulate in two types of regions, with 25% of CpGs present within Alu retrotransposons, and 2% within CpG islands (CGIs) (57–59). In vertebrate genomes, over 50% of genes contain CGIs, with other regions of the genome generally CpG-depleted (60). CGIs are present in the promoter region of around 60% of human genes, with these CGIs remaining largely unmethylated (60). CGIs and their methylation status are highly conserved between species (61), for example, 40% of promoter CGIs and 64% of intragenic non-promoter CGIs presented orthologous methylation patterns between mice and humans (62), highlighting the functional importance of these configurations. Indeed, DNA methylation is essential for processes such as X chromosome inactivation (for X chromosome dosage compensation in females) (63, 64), genomic imprinting (65), embryonic development (66), and tissue-specific gene expression (67).
A range of enzymatic factors have been shown to be involved in DNA methylation and demethylation, for example, the DNA methyltransferases, Dnmt3a and Dnmt3b (68). A range of human diseases, such as leukaemia, lymphoma, Tatton-Brown-Rahman syndrome and autosomal dominant cerebellar ataxia have been associated with genetic mutations within the genes encoding these enzymes (66), highlighting the functional importance of DNA methylation. Changes in DNA methylation status have been associated with a wide range of diseases, including cancer, metabolic disorders, autoimmune diseases, and neurological disorders, reviewed by Jin and Liu (69). Environmental factors such as nutritional intake, chemical exposure (pollutants or toxins), and lifestyle can influence epigenetic status (70). Whilst these environmental factors can disrupt epigenetic signals to cause disease, disease states can initiate feedback mechanisms to further alter epigenetic status. For example, when metabolism becomes altered during diabetes (hyperglycaemia) or CKD (uraemia), epigenetic changes can occur which result in altered gene expression, potentially increasing the risk of disease complications; with these processes referred to as metabolic, hyperglycaemic, uremic or inflamed “memory” (71–78).
It is known that advancing age is a risk factor for chronic diseases such as diabetes and CKD (79–86). DNA methylation is an estimator of biological age, as highlighted by the introduction of epigenetic clocks by Steve Horvath and the use of this epigenetic biomarker across multiple tissue and cell types to provide predictions of lifespan and healthspan (87–89). Evidence suggests that accelerated epigenetic aging (an increased difference between chronological and epigenetically predicted age) is associated with CVD, diabetes, Alzheimer’s disease, cancer and kidney disease (87, 90–99). Matías-García et al. performed a trans-ethnic meta-analysis of up to seven populations, investigating five kidney traits (GFR, prevalent CKD, urine albumin-to-creatinine ratio (uACR), microalbuminuria and serum urate) and seven DNA methylation-based age/lifespan predictors (91). These authors identified 23 significant associations between several kidney traits and epigenetic clock age/lifespan predictors; 6 replicated across ethnic groups, and 16 replicated in an ethnic-specific manor (91). Different epigenetic clocks probe different aspects of aging, for example, the extrinsic epigenetic age acceleration (EEAA) clock is a measure of immune system aging (100, 101), whilst mortality risk scores (MRSs) have been associated with oxidative stress (102). CKD is associated with both increased inflammation and oxidative stress (103–108), potentially explaining the strong predictive associations obtained when using these methods in the study of kidney function decline (91).
An alternative method to study epigenetic features of CKD is by harnessing an epigenome-wide association study (EWAS) approach (23, 71). Much like the SNP arrays harnessed for GWAS analysis, commercial arrays have been developed to facilitate the reproducible and high-throughput study of CpG sites across the human genome, for example, the Illumina MethylationEPIC BeadChip Infinium array investigates 853,307 CpG (850K) sites, with increased coverage of regulatory regions compared to previous methylation arrays (109, 110). Alternative forms of epigenetic regulation, such as ncRNA or chromatin modifications, can be analysed via methods such as quantitative polymerase chain reaction (qPCR), RNA sequencing (RNA-seq) and chromatin immunoprecipitation sequencing (ChIP-seq) (111, 112), recently reviewed by Walters and Cox (113). Interestingly, computational methods have facilitated the direct detection of epigenetic modifications during Oxford Nanopore genome sequencing (114), identifying a potential avenue for future kidney disease research to intricately integrate and streamline genetic and epigenetic analyses.
Epigenetic variation is associated with CKD and DKD across multiple populations (16, 23, 71, 111, 115–126). Functional annotation of these epigenetic variations has highlighted the potential association of these variations with processes such as haemostasis, endocrine or metabolic control, mitochondrial function, apoptotic cell clearance, immune cell activation, or regulation of cell shape (117, 118, 121, 125, 127). Due to the dynamic and reversible nature of epigenetic medications, such studies may provide attractive targets for therapeutic interventions (128–132).
Functional effects of epigenetic modifications on kidney conditions have been confirmed via studies harnessing mouse models, validating effects in vivo (133, 134). Park et al. determined that differential methylation of tumor necrosis factor alpha (TNF-α) resulted in altered gene expression, with increased TNF expression in diabetic mice increasing the severity of kidney disease (123). Chen et al. determined that promoter regions of mammalian target of rapamycin (mTOR) regulators were differentially methylated in patients with diabetes (135). These authors highlighted the role of the DNA methyltransferase, DNMT1, in controlling the methylation of mTOR regulator genes, with DNMT1 expression positively correlated with inflammatory activity of peripheral blood mononuclear cells (PBMCs) from diabetic patients (135). Harnessing mouse models, Chen et al. also determined that mTOR dysregulation in diabetic immune cells resulted in kidney inflammation associated with DKD (135).
Specific DNA methylation patterns have also been associated with kidney disease progression and co-morbidities (136, 137), with different methylation profiles observed for early versus late stages of DKD (116), Gluck et al. have shown improved estimations of renal function in DKD patients upon the incorporation of methylation status at CpG sites significantly associated with renal function decline (124). Onishi et al. determined that urine levels of 5-Methyl-2′-deoxycytidine (5MedC), a by-product of DNA methylation, was significantly associated with late-stage CKD prediction (138). Similarly, Marumo et al. determined that SMTNL2 (Smoothelin Like 2) methylation levels in urine sediment significantly correlated with renal function decline and when incorporated into models to predict faster GFR decline in diabetics, provided a more successful prediction method (120). In a recent study by Dritsoula et al., the relationship between CKD and cardiovascular disease (CVD), a common CKD co-morbidity, was explored in the context of methylation. These authors identified changes in DNA methylation in the arterial wall of CKD patients and uncovered interesting targets for future study to advance our understanding of the molecular dysfunctions occurring in CKD which may result in cardiovascular damage (137). These studies highlight the diagnostic potential of methylation status determination for both disease pathogenesis and progression.
A recent longitudinal study has also explored the effect of various kidney disease treatment methods on methylation levels, in 23 individuals (and 24 controls) with 1 year of follow-up data. Witasp et al. recently identified that the number of significantly differentially methylated CpG sites (compared to healthy controls) fell from approximately 12,000 and 19,000 pre-treatment, to approximately 300 and 400 12 months post-treatment, for dialysis and transplant respectively (139). These authors also noted distinct localisation patterns for differentially methylated CpG sites for dialysis and transplant patients, and highlighted that the methylation status of regions associated with cellular aging or metabolism were particularly altered 12 months post-treatment, to become more in line with healthy control participants (139). 413 differentially methylated genes present in both dialysis and transplant patients remained unaltered 12 months post-treatment, identifying potentially distinct and robust CKD markers warranting future study (139).
Emerging Insights From Transcriptomic Analysis of CKD
In order to gain additional functional insights into the effects of genetic or epigenetic variants, studies have harnessed transcriptomics, the study of RNA transcripts via technologies such as microarrays, qPCR or RNA-seq (140). These investigations have identified gene expression profiles and determined how they differ during health and disease. Many transcriptomic studies investigating kidney disease have focused on examining messenger RNA (mRNA) or the ncRNA subtype, micro RNA (miRNA) (23); however, attention has turned to the contributions made by other ncRNAs such as, ribosomal RNA (rRNA), transfer RNA (tRNA), small nuclear RNA (snRNA), small nucleolar RNA (snoRNA) or long non-coding RNA (lncRNA), with this area of research undergoing recent reviews (141–143). Potential exists to harness RNAs as novel biomarkers for kidney disease pathogenesis or progression, with a distinct focus on urinary RNAs, particularly those contained with extracellular vesicles (protective membrane bound carriers released by cells), as a less invasive and robust means of disease diagnosis (144–148).
A 2018 review summarised literature investigating transcriptomic analysis in the context of kidney disease (23), with a number of additional investigations published in recent years (146, 148–159). An interesting study by Fan et al. carried out RNA-seq analysis of kidney biopsies from early DKD, advanced DKD, or control patients, to reveal gene expression changes from healthy to disease states (158). Gene ontology analysis highlighted that genes involved in iron transport and cell differentiation were positively associated with GFR, whilst genes involved in fibrosis and immune response were negatively associated with GFR (158). Moreover, harnessing kidney single-cell RNA-seq datasets (160, 161), Fan et al. deconvolved their dataset to estimate the relative fraction of different kidney cell types within their samples, reporting a significant increase in macrophages, monocytes, fibroblasts, and myofibroblasts in advanced DKD stages, along with a reduction in proximal tubular endothelial cells (158). This transcriptomic analysis reflected results obtained from studies harnessing alternative methods, such as histological examination, which reported increased inflammation and fibrosis during DKD, alongside tubular cell injury (162). Indeed, harnessing single cell RNA-seq has rapidly advanced the field of kidney disease research, recently reviewed by Jiang et al. (163). A greater understanding of how cell heterogeneity changes during kidney disease advances our ability to identify cellular pathways of disease, develop advanced or personalised therapies and improve disease diagnosis or classification (151, 160, 164–166).
Harnessing Metabolomic and Proteomic Analyses to Aid CKD Diagnosis and Treatment Planning
Alternative methods to study the molecular pathways disrupted during kidney disease involve the investigation of metabolomic and proteomic profiles, recently reviewed by Cañadas-Garre et al. (167). Whilst proteomics assesses the enzymatic, structural protein, antibody, hormonal, DNA-associated or receptor protein profiles, metabolomics assesses the sugar, amino acid, lipid, organic compound or nucleotide profile, which can be impacted by diet or the microbiome (168). These profiles are dynamic and can provide insights into functional changes which occur during kidney disease over time, with genetic, epigenetic or transcriptomic alterations potentially impacting the downstream protein and metabolite landscape. A recent review by Dubin et al. summarised insights gained from the proteomic and metabolomic study of kidney disease, highlighting that because these methods can be easily applied to human blood or urine samples, there is now considerable scope to develop novel biomarkers for disease detection or treatment planning using these approaches (168). Dubin et al. do, however, highlight the challenge of interpreting metabolomic and proteomic studies, with the direction of influence difficult to ascertain (i.e. does the disease cause the protein/metabolite level to change, or vice versa), and the need for downstream functional studies to confirm causative associations (168).
Metabolomic and proteomic studies have resulted in advanced tools to aid patient classification into CKD or DKD stages. Chen et al. utilised ultra-performance liquid chromatography-tandem mass spectrometry to identify five metabolites which explained 94.1% of variation observed between CKD stages (169). Further animal model and cell culture investigations into 5-methoxytryptophan (5-MTP), which presented increased levels in serum as CKD progressed, determined that this metabolite presented strong anti-fibrotic and anti-inflammatory effects, and targeting its regulatory enzyme tryptophan hydroxylase-1 (TPH-1) might prove an effective therapeutic strategy to mitigate CKD progression (169). Fan et al. utilised mass spectrometry to assess the urine proteomic profile of CKD patients without diabetes, DKD patients and diabetic patients without nephropathy, identifying 509 disease specific differentially excreted proteins, and the related pathways, such as late endosomal microautophagy and insulin-like growth factor (IGF) transport regulation in diabetes, and immune system or platelet activation in both DKD and CKD (170). Strong correlations were identified between kidney function measures, such as GFR or uACR, and 46 protein abundance levels, with these authors harnessing urine proteomes to develop models capable of distinguishing between various DKD stages and diabetes (170), with potential future applications within diagnostic testing.
Proteomic or metabolomic studies have been harnessed to assess patient responses to treatment over time, aiding the development of effective and appropriate treatment regimens, unique to each patient. Zhu et al. compared the metabolomic profile of pre-dialysis, haemodialysis and peritoneal dialysis patients, identifying 42 metabolites significantly altered among these three groups (171). Pathway and functional annotation highlighted that haemodialysis and peritoneal dialysis patients had potentially increased risk of infection, increased cardiovascular risk and increased oxidative stress (171). Additionally, Hu et al. carried out metabolomic analysis in haemodialysis patients who had a cardiac death within 1 year of study enrolment, compared to haemodialysis patients surviving after 1 year, with these authors identifying that greater odds for cardiac death were associated with higher levels of several lipid metabolites, an amino acid metabolite and phosphate (172). As highlighted previously, urinary extracellular vesicles have proved useful for the identification of excreted RNAs significantly associated with kidney disease. Proteomic analysis of urinary extracellular vesicles, carried out by Braun et al., identified phosphoenolpyruvate carboxykinase 2 (PCK2) as an early predictive marker of transplant outcome after 1 year (173).
These studies highlight how metabolomic and proteomic studies have uncovered biomarkers to advance the assessment of risk factors and advance the development of non-invasive clinical tests for CKD. Moreover, these studies have advanced CKD treatment planning to aid personalised medicine and improve patient outcomes. With a greater understanding of the functional changes which occur during CKD, metabolomic and proteomic studies have uncovered novel targets to guide future therapeutic development.
Insights Gained From Environmental Datasets in the Study of Kidney Function and Disease
Beyond the blood, urine or biopsy-derived omic changes associated with kidney disease, researchers have also explored the impact of external factors on CKD. Studies have determined the influence of natural or built environments, pollution and social disparities on CKD. Poverty can impact the development of CKD by modifying health behaviour (due to limited information regarding disease prevention or management), reducing access to healthcare, impacting nutritional intake and increasing exposure to risk factors such as stress, infectious diseases and pollutants (174–177). Closer proximity to open or green spaces has been associated with higher kidney function (178, 179), with the distance to green space shown to increase with social deprivation (180). Closer proximity to open and green spaces may result in improved air quality, increased access to physical recreation and relaxation areas, or reduced noise pollution, with these factors each individually associated with improved kidney function (178, 181–184). Many of these factors change as a result of urbanisation, with urbanisation also shown to modify the presence of potentially toxic elements (PTEs) within the soil, with such PTEs associated with CKD incidence, including CKD attributed to unknown aetiology (185, 186). Urbanisation also modifies the association between air pollutants (namely fine particulate matter and nitrogen dioxide) and CKD (187). Interestingly, the strongest associations between air pollutants and CKD were found in medium-urbanised areas, likely because urbanisation not only results in negative consequences, but also brings positive effects, such as improved healthcare access or higher socio-economic status (187). Scope exists to harness the “PROGRESS” framework (place of residence, race/ethnicity/culture/language, occupation, gender/sex, religion, education, socioeconomic status, and social capital) to study complex environmental, social and demographics interactions, to highlight differences in CKD burden in disadvantaged populations and to identify potential interventions to reach health equity (188).
A number of studies have harnessed a systematic, population level approach to studying environmental impacts on CKD, via environment-wide association studies (EnvWAS). Lee at al. assessed bio-monitored chemicals (262 chemicals, measured in blood or urine samples) in participants from the United States National Health and Nutrition Examination Survey (NHANES, 46,748 participants), and identified significant associations with multiple kidney function outcomes; 24 (9%) chemical levels were associated with reduced eGFR, five (2%) with albuminuria, and nine (3%) with composite CKD outcomes (albuminuria or reduced eGFR) (189). These authors determined that increased blood lead and cadmium levels were significantly associated with reduced kidney function, in line with previous studies (189, 190). Interestingly, Yimthiang et al. identified a significant association between simultaneous exposure to cadmium and lead and increased risk of high fasting plasma glucose and kidney function decline, also highlighting the impact of this exposure on DKD progression (191). Cadmium and lead exposure has been associated with processes such as oxidative stress, inflammation and fibrosis within the kidneys (192), with a recent histological analysis by Barregard et al. determining that even low levels of cadmium in the kidney can induce tubular atrophy (193). Here, histological and experimental analyses have be utilised to highlight the biological plausibility of significant EnvWAS associations.
Zheng et al. recently reviewed the strategy and challenges of EnvWAS, highlighting that whilst EnvWAS can provide insights into factors influencing phenotypic changes, care must be taken when drawing associations via statistical inference, with further study required to determine the biological function or molecular mechanism of these associations (194). These authors also highlight aspects, such as biomarker or chemical half-lives, spatial or temporal heterogeneity of the environment, detection or quantifications limits of technical methods, and between-factor associations, which can potentially influence EnvWAS outcomes and must be carefully considered during each analysis (194). These studies highlight how a range of data sources can be used to deepen our understanding of the causes and effects of kidney disease, improving our ability to target molecular pathways in novel therapies and advise on the importance of minimising environmental or occupational exposure to specific chemical agents to lower disease risk. This is especially important due to the impact of environmental variation on epigenetic status, with the subsequent cellular effects of these changes potentially resulting in disease or impacting future generations via transgenerational epigenetic inheritance (195).
Challenges Which Remain in the Multi-Omic Study of CKD
Each of the omic analyses discussed above bring their own challenges, such as the effect of confounders or co-variables, the requirement for adequate sample sizes to draw meaningful associations, analytical considerations (significance thresholds, false discovery rates and handling large multi-dimensional datasets). Effective harmonisation and standardised quality control are particular issues for multi-centre studies or those using historical datasets, as well as variations between platform technologies and batch effects. Variability also exists in terms of CKD outcomes, patient characteristics, disease progression and response to treatment, which makes the development of accurate predictive models for CKD prognosis and prediction a challenge (196, 197). Provenzano et al. reviewed the impact of such variability on the study of CKD and highlighted a number of statistical methods and adapted clinical trial designs which can be harnessed to advance the development and assessment of predictive CKD models, and build a more individualised focus to CKD treatment (196, 197). These authors highlight the importance of selecting an appropriate population for model design, which is transferable for use in CKD patients. Moreover, these authors highlight the need for more longitudinal studies with larger datasets to ensure long-term outcomes which may take years to present, such as ESKD or mortality, can be properly assessed (196, 197). Overall, rigorous multi-omic methodologies will advance our ability identify disrupted biological pathways, stratify patients based on risk, prioritise resources, and deliver a personalised treatment approach, ultimately improving patient care and outcomes.
An important consideration for the study of multi-omics is the presence of ‘dark matter,’ consisting of those features which go undetected via current methodologies, or those which remain unannotated due to limited prior knowledge (198). For example, coding regions make up only part of human genome (199), with studies now turning to non-coding regions to gain a deeper understanding of the impact of genetic, epigenetic or transcriptomic changes on health and disease (200). Additionally, the Human Metabolite Database (version 5.0) describes 253,243 metabolites; however, as of March 2022, only 24,309 (9.6%) have been detected (with or without quantification) experimentally (201), highlighting a deficit with current experimental metabolomic methods. Interestingly, Odenkirk et al. recently reviewed the application of artificial intelligence methods to advance the annotation of unknowns and improve the estimation of undetected features to improve statistical analysis and interpretation in multi-omic datasets; however, these authors emphasise the need for sufficient model training and downstream validation to ensure confident conclusions can be drawn (198).
Functional Annotation of Features Significantly Associated With CKD
Functional annotation of those variants or features identified during upstream omic analysis is an important step in the multi-omic pipeline; translating information gained into knowledge which is useful for biological validation, therapeutic development or real-world applications. This is often achieved via gene ontology or pathway analysis, with these processes providing insights into functional commonalities and differences, even across different methodologies and cohorts (202–204). Challenges remain in achieving standardisation of pathway analysis annotations between studies and databases, in the unification of similar ontologies to streamline analyses and in advancing annotation coverage (204). An interesting advancement came with the development of an ontology hierarchy annotating the Human Metabolite Database (201, 205), bringing metabolomic studies more in line with the annotations available for genomic and proteomic studies (202, 206, 207). Moreover, text and database mining methods provide additional opportunities to increase the confidence in annotations, and reduce the burden of manual curation of ontology or genotype-phenotype interaction databases (208, 209).
Molecular quantitative trait loci (MolQTL), such as expression (eQTL), methylation (mQTL), splicing (sQTL) or chromatin assembly (caQTL) are genetic variants associated with specific molecular traits. MolQTL were reviewed by Sullivan et al. in the context of CKD, with these authors highlighting how MolQTL are integrated with GWAS to prioritise target genes (210). The Genotype–Tissue Expression (GTEx) project has generated eQTL datasets for 49 human tissues (as of March 2022, release version 8), including the kidney, allowing tissue-specific gene eQTLs to be identified (211). Xu et al. harnessed the GTEx datasets to determine which transcriptionally active SNPs, previously identified via GWAS to be associated with CKD disease-defining traits, were associated with kidney-specific or ubiquitous expression (212). This facilitated the prioritisation of genes for downstream functional validation and Mendelian Randomization analysis, which resulted in the discovery that alternatively spliced MUC1 mRNA isoform expression was causally related to GFR; with alternative splicing a potential allele specific effect (212). This study is an excellent example of how the integration of multiple methodologies can provide biological insights and refine hypotheses to direct downstream studies, such as animal model or cell culture assays, to explore the molecular mechanisms of disease.
Challenges Faced During the Full Integration of Multiple Omic Datasets
A significant challenge in the field of multi-omics is the full integration of several omic datasets. A number of CKD studies outlined above have carried out single-level analysis, for example, carrying out a separate GWAS, EWAS or TWAS. For multi-level analysis, integration of these datasets must occur, with data integration taking either the form of integrating the same datatype from multiple studies (horizontal integration), or the study of different ‘omes’ within the same cohort (vertical integration) (213, 214). Vertical integration allows a range of methods to be harnessed, each reflecting different aspects of disrupted biological function during disease. For example, proteomics may provide a better understanding of disrupted protein interactions and binding, whereas metabolomics may provide better insights into dysregulated chemical processes. Moreover, ‘ome’ interactions, such as allele specific methylation (215), allele specific chromatin assembly or gene expression (216, 217), and non-coding RNA modulation of protein or gene expression (218), may be overlooked unless a fully integrated multi-omic approach is utilised, meaning vital insights into the molecular mechanisms of kidney disease may go undiscovered.
Data integration can take multiple forms, for example, individual omics can be processed separately and integrated later in the pipeline, or alternatively, omic datasets can be concatenated into a single matrix to be processed and analysed together (219). Merging multiple complex and highly variable biological datasets brings with it many challenges, summarised in Figure 2; with many tools now available to optimise this process (220). Researchers must ensure the most appropriate and optimal approach is taken, depending on the biological question and the omic datasets available. Table 1 highlights a number of studies which have utilised an integrated multi-omic approach to study kidney disease.
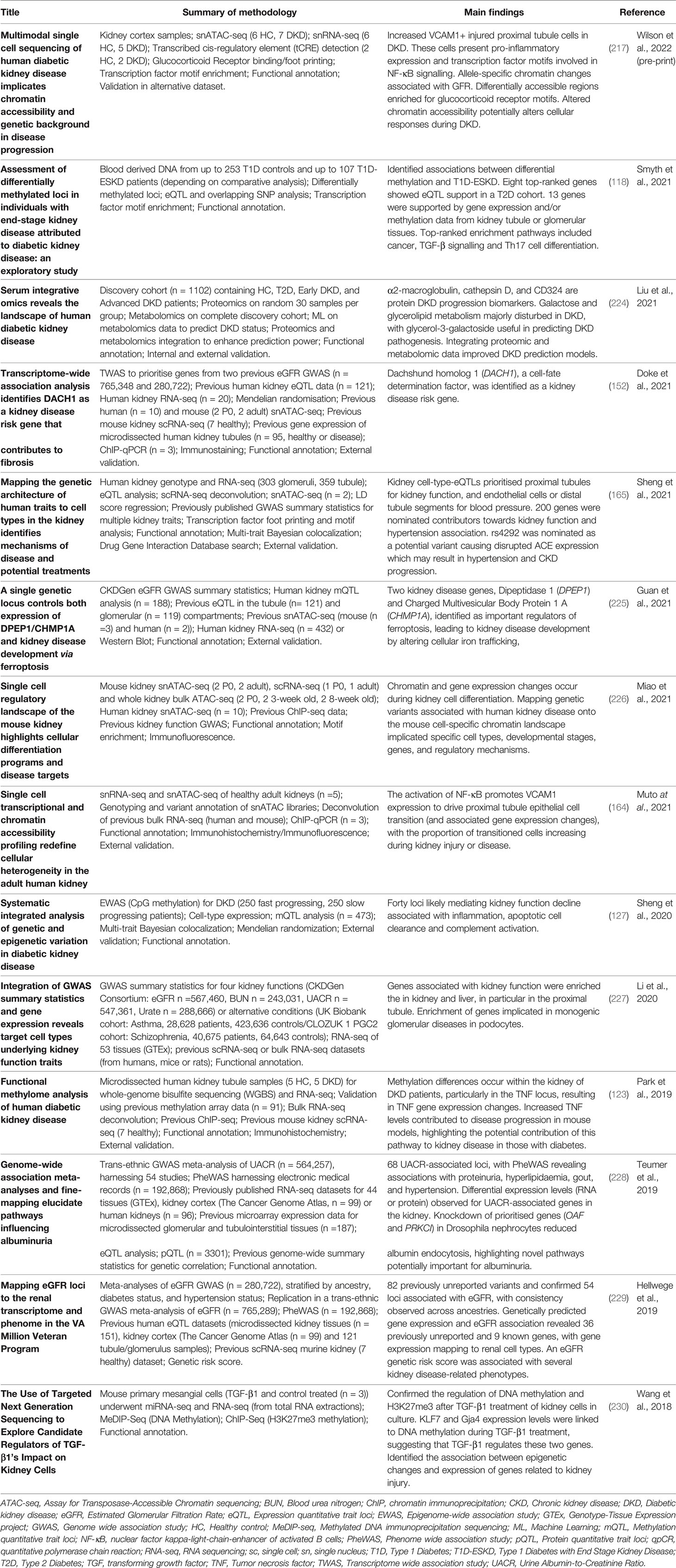
Table 1 Examples of kidney disease studies which have harnessed a more integrated multi-omic analysis approach from 2018.
Improving Cohort Phenotype Information to Improve Multi-Omic Analyses of CKD
An additional aspect of multi-omic studies which holds potential to advance the analysis and interpretation of data is carefully phenotyped cohorts. For multi-omic studies, particularly those carried out between cohorts or consortia, lack of standardisation in the measures and classification of kidney function or co-variables can limit the Findability, Accessibility, Interoperability, and Reuse (FAIR) of datasets (231). Clinical, epidemiological and demographical records provide a wealth of data, and with careful and proper integration into multi-omic datasets, they can provide advanced phenotypic information, recently reviewed by de Maturana et al. (232). Shang et al. provide an excellent demonstration of harnessing clinical records to study CKD; developing an algorithm capable of classifying patients into CKD stages based on electronic clinical records (233). Via an observational study, these authors determined the presence of co-morbidities across CKD stages, identifying novel associations, such as the prevalence of several psychiatric comorbidities among patients with mild CKD compared to those patients with normal renal function, independent of age and sex (233). These authors also utilised their algorithm in the Electronic Medical Records and Genomic (eMERGE) network, carrying out a GWAS analyses of CKD (Stage 3 or greater) which identified significant associations with UMOD and APOL1 (233, 234). An additional Phenome-wide association study (PheWAS) identified further associations for these genes with a number of additional kidney associated phenotypes, such as kidney transplantation, ESKD, and dialysis (233). The methods developed by Shang et al. have the potential to support the provision of personalised medicine for those living with kidney disease; facilitating risk stratification for optimised treatment planning. These authors do highlight the need for further investigations to be conducted in adult and child cohorts, across diverse ancestral backgrounds (233), to ensure that the tools developed are appropriate for use across all patients, in a fair and equitable manner.
Considering Sex Imbalances in the Multi-Omic Study of Kidney Disease
Whilst future studies must incorporate the assessment of CKD across diverse ancestral backgrounds, work is also required to assess CKD between sexes. In 2017, the age-standardised prevalence of CKD was higher in females (9.5%) compared to males (7.3%), whereas the age-standardised incidence of dialysis and transplantation was higher in males (13.7 per 100 000 population) compared to females (8.6 per 100 000 population) (7). Moreover, the global age-standardised CKD mortality rate was 1.39 times greater in males compared to females (7), suggesting that whilst more females are diagnosed with CKD, males may have faster disease progression. Sex-specific variations in omic datasets are potentially overlooked when both sexes are analysed in a single analysis. Bond et al. highlight that sex biases within curated databases may also result in uninformative or lower significance results in scenarios where sex differences exist (235). These authors provide action points for scientists, databases and funding agencies to tackle this problem, adding that including sex as a covariate in a mixed cohort analysis is insufficient to account for this fundamental variable, with the need for analyses to be run in both mixed and sex-stratified groups to optimise data analysis and interpretation (235).
A small number of sex-stratified analyses of the healthy kidney and kidney disease have been carried out (236–238), however, more work is needed in this area. Graham et al. recently determined that SLC47A1 (also known as MATE1 (multidrug toxin and extrusion protein 1)) was significantly associated with GFR in females but not in males (239). This gene presented cell-type specific expression in the mouse kidney proximal tubule, with previous studies also supporting hormonal regulation of this gene (239–241), highlighting biological plausibility that this gene may influence sex-specific features of kidney disease. Sex chromosomes may also contribute to sex-specific pathogenesis and progression of CKD; however, more advanced sex chromosome imputation and wider inclusion of sex chromosome genes on genotyping or methylation arrays is required to gain further insights from these largely ignored chromosomes (16, 28). Mosaic loss of the Y chromosome (mLOY), which increases with age and has been strongly associated with both diabetes and cardiovascular disease (242), two common CKD comorbidities, has been explored in the context of renal cancers (243, 244), but not CKD. This work highlights an interesting focus for future study, which may uncover novel sex-specific mechanisms of CKD pathogenesis, potentially useful for the development of novel diagnostics or personalised therapeutic targets.
Linking Causes and Consequences of CKD Together
This review highlights multiple forms of omic analyses which have been conducted to investigate the factors influencing CKD, such as genomics, epigenomics, transcriptomics, metabolomics and proteomics, phenomics, as well as exploring social and environmental impacts. The visual representation shown in Figure 3 summarises influences and consequences of multi-omic biomarkers on CKD and associated variables. Whilst many studies have explored single-level omic analysis, significant value can be achieved by harnessing multi-level analysis by combining numerous omic datasets. Various challenges exist when fully integrating multiple omic datasets for the study of CKD, such as optimising data merging, incorporating the study of sex-biases, and improving nomenclature and phenotyping. These processes must be properly considered for each analysis to enhance data analysis and improve interpretation. Moreover, by understanding these challenges prior to experimental design, more effective data and meta-data generation can be achieved, aiding data sharing and accessibility between studies, ultimately accelerating this research field.
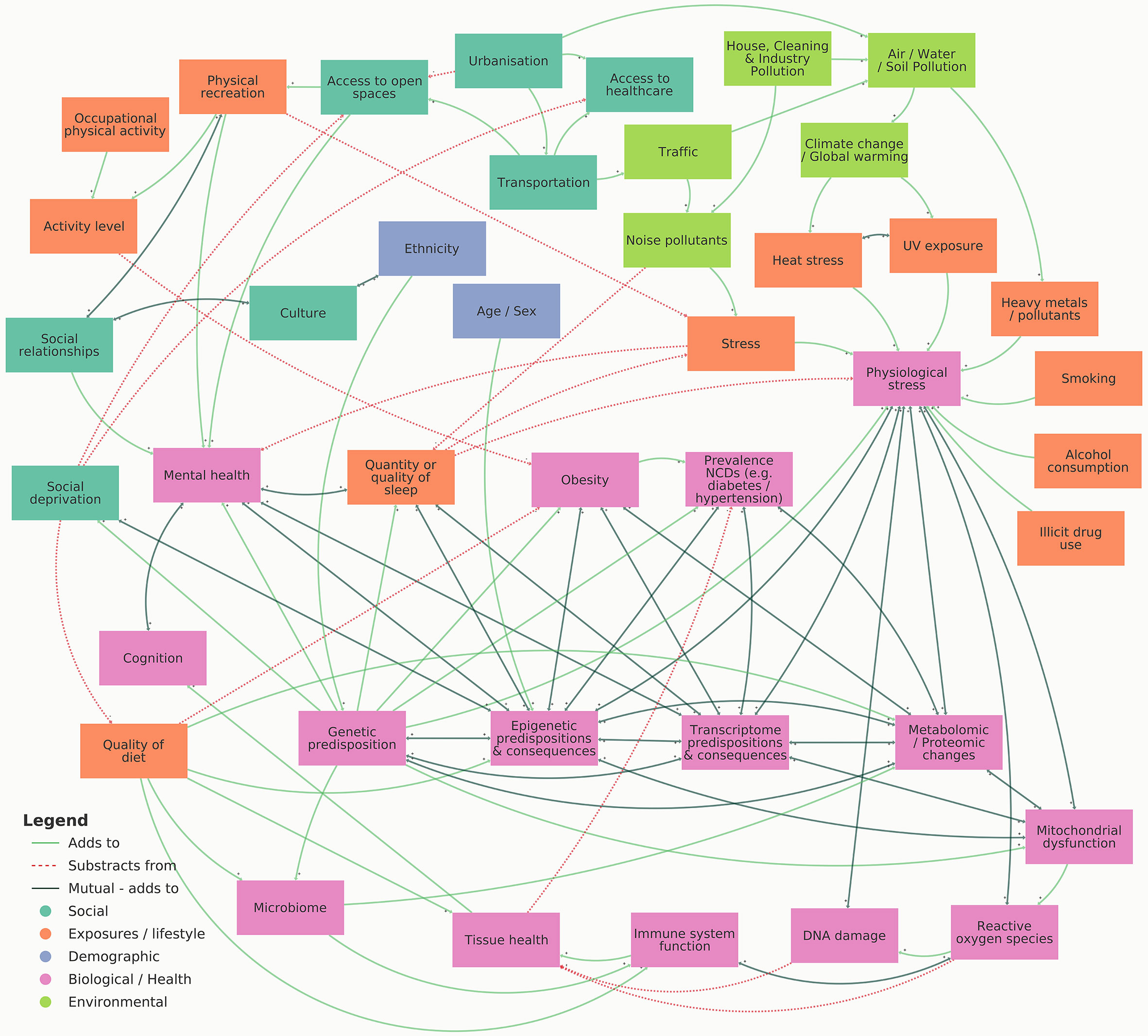
Figure 3 Visual representation illustrating the influences and consequences of multi-omic biomarkers on CKD. An interactive version of this map can be viewed online at https://embed.kumu.io/4ac1a6b9dfef3b8bc1ffdf165737c6c4.
Conclusion
CKD is a heterogeneous disease presenting a significant impact on global healthcare budgets. Decreasing the burden of CKD has been identified as an important factor in achieving the United Nations sustainable development goal of reducing premature mortality from non-communicable diseases by one third by 2030. In order to achieve this target, a range of approaches have been taken to better understand CKD pathogenesis and progression. Multi-omic studies have facilitated the discovery of novel genetic and epigenetic variants significantly associated with CKD, with gene ontology or pathway analysis, as well as data mining, facilitating the prioritisation of those targets significantly associated with kidney function decline. The functional consequences of such variations have been assessed via transcriptomic, proteomic, metabolomic and phenomic analyses, as well as by harnessing in vitro and in vivo models. Whilst challenges remain in the comprehensive integration of such complex and multifaceted biological datasets, a multi-level approach to combining such datasets has facilitated the discovery of novel pathways associated with CKD pathology, provided insights into the biological effect of different treatment routes, and improved our understanding of disease progression at an individual patient level. Many opportunities remain in the field of multi-omics, with improved machine learning, DNA or RNA sequencing, molecule detection, data analysis and statistical tools being developed, applicable for studying a range of human diseases, including CKD. The multi-omic study of CKD has thus far aided the identification of new therapeutic targets, paved the way towards personalised treatment plans and advanced our knowledge of risk factors for CKD and its progression, with the ultimate goal of improving patient care and outcomes.
Author Contributions
CH & AMK conceived the review. CH initially drafted the review with input from AMK. AM provided critical clinical oversight while IA-P and RH supported the development of Figure 3. All authors contributed to the article, reviewed the manuscript for important intellectual content and approved the final version for submission.
Funding
This research is supported by the HSC R&D division (STL/5569/19) and UKRI (MRC MC_PC_20026). CH is supported by funding from a Science Foundation Ireland and Department for the Economy, Northern Ireland partnership award (15/IA/3152). IA-P is supported by funding from the UKRI Industrial Strategy Challenge Fund (ESRC, SBDRP, ES/V016075/1).
Conflict of Interest
The authors declare that the research was conducted in the absence of any commercial or financial relationships that could be construed as a potential conflict of interest.
Publisher’s Note
All claims expressed in this article are solely those of the authors and do not necessarily represent those of their affiliated organizations, or those of the publisher, the editors and the reviewers. Any product that may be evaluated in this article, or claim that may be made by its manufacturer, is not guaranteed or endorsed by the publisher.
Acknowledgments
Figures 1 and 2 were created using Biorender.com. Figure 3 was created using Kumu relationship mapping software [https://kumu.io (April 2022)].
References
1. Kerr K, McAneney H, Smyth LJ, Bailie C, McKee S, McKnight AJ. A Scoping Review and Proposed Workflow for Multi-Omic Rare Disease Research. Orphanet J Rare Dis (2020) 15(1):107. doi: 10.1186/s13023-020-01376-x
2. Zielinski JM, Luke JJ, Guglietta S, Krieg C. High Throughput Multi-Omics Approaches for Clinical Trial Evaluation and Drug Discovery. Front Immunol (2021) 12:590742. doi: 10.3389/fimmu.2021.590742
3. Sha Q, Lyu J, Zhao M, Li H, Guo M, Sun Q. Multi-Omics Analysis of Diabetic Nephropathy Reveals Potential New Mechanisms and Drug Targets. Front Genet (2020) 11:616435. doi: 10.3389/fgene.2020.616435
4. Kerr K, McAneney H, Flanagan C, Maxwell AP, McKnight AJ. Differential Methylation as a Diagnostic Biomarker of Rare Renal Diseases: A Systematic Review. BMC Nephrol (2019) 20(1):320. doi: 10.1186/s12882-019-1517-5
5. Olivier M, Asmis R, Hawkins GA, Howard TD, Cox LA. The Need for Multi-Omics Biomarker Signatures in Precision Medicine. Int J Mol Sci (2019) 20(19):4781. doi: 10.3390/ijms20194781
6. Carney EF. The Impact of Chronic Kidney Disease on Global Health. Nat Rev Nephrol (2020) 16(5):251. doi: 10.1038/s41581-020-0268-7
7. Bikbov B, Purcell CA, Levey AS, Smith M, Abdoli A, Abebe M, et al. Global, Regional, and National Burden of Chronic Kidney Disease, 1990–2017: A Systematic Analysis for the Global Burden of Disease Study 2017. Lancet (2020) 395(10225):709–33. doi: 10.1016/S0140-6736(20)30045-3
8. Foreman KJ, Marquez N, Dolgert A, Fukutaki K, Fullman N, McGaughey M, et al. Forecasting Life Expectancy, Years of Life Lost, and All-Cause and Cause-Specific Mortality for 250 Causes of Death: Reference and Alternative Scenarios for 2016–40 for 195 Countries and Territories. Lancet (2018) 392(10159):2052–90. doi: 10.1016/S0140-6736(18)31694-5
9. United States Renal Data System. In: USRDS Annual Data Report: Epidemiology of Kidney Disease in the United States. (2020) Bethesda, MD: National Institutes of Health, National Institute of Diabetes and Digestive and Kidney Diseases. Available at: https://adr.usrds.org/2020/suggested-citation#:~:text=Suggested%20citation%20for%20this%20report,%2C%20Bethesda%2C%20MD%2C%202020.
10. Lin X, Xu Y, Pan X, Xu J, Ding Y, Sun X, et al. Global, Regional, and National Burden and Trend of Diabetes in 195 Countries and Territories: An Analysis From 1990 to 2025. Sci Rep (2020) 10(1):14790. doi: 10.1038/s41598-020-71908-9
11. International Diabetes Federation. IDF Diabetes Atlas. 10th ed. Brussels, Belgium: International Diabetes Federation Diabetes Atlas (2021). Available at: https://diabetesatlas.org/en/.
12. United Nations. UN General Assembly, Transforming Our World: The 2030 Agenda for Sustainable Development. (2015). New York
13. Regele F, Jelencsics K, Shiffman D, Paré G, McQueen MJ, Mann JFE, et al. Genome-Wide Studies to Identify Risk Factors for Kidney Disease With a Focus on Patients With Diabetes. Nephrol Dial Transplant (2015) 30(Issue suppl_4):iv26–34. doi: 10.1093/ndt/gfv087
14. Satko SG, Sedor JR, Iyengar SK, Freedman BI. Familial Clustering of Chronic Kidney Disease. Semin Dial (2007) 20(3):229–36. doi: 10.1111/j.1525-139X.2007.00282.x
15. O’Seaghdha CM, Fox CS. Genetics of Chronic Kidney Disease. Nephron Clin Pract (2011) 118(1):c55–63. doi: 10.1159/000320905
16. Cañadas-Garre M, Anderson K, Cappa R, Skelly R, Smyth LJ, McKnight AJ, et al. Genetic Susceptibility to Chronic Kidney Disease - Some More Pieces for the Heritability Puzzle. Front Genet (2019) 10:453. doi: 10.3389/fgene.2019.00453
17. Zhang J, Thio CHL, Gansevoort RT, Snieder H. Familial Aggregation of CKD and Heritability of Kidney Biomarkers in the General Population: The Lifelines Cohort Study. Am J Kidney Dis (2021) 77(6):869–78. doi: 10.1053/j.ajkd.2020.11.012
18. Lei HH, Perneger TV, Klag MJ, Whelton PK, Coresh J. Familial Aggregation Study of Renal Disease in a Population-Based Case-Control Study. J Am Soc Nephrol (1998) 9(7):1270–6. doi: 10.1681/ASN.V971270
19. Madeira EPQ, da Rosa Santos O, Santos SFF, da Silva LA, Innocenzi AM, Santoro-Lopes G. Familial Aggregation of End-Stage Kidney Disease in Brazil. Nephron (2002) 91(4):666–70. doi: 10.1159/000065029
20. Wu HH, Kuo CF, Li IJ, Weng CH, Lee CC, Tu KH, et al. Family Aggregation and Heritability of ESRD in Taiwan: A Population-Based Study. Am J Kidney Dis (2017) 70(5):619–26. doi: 10.1053/j.ajkd.2017.05.007
21. Skrunes R, Svarstad E, Reisæter AV, Vikse BE. Article Familial Clustering of ESRD in the Norwegian Population. Clin J Am Soc Nephrol (2014) 9(10):1692–700. doi: 10.2215/CJN.01680214
22. Connaughton DM, Hildebrandt F. Personalized Medicine in Chronic Kidney Disease by Detection of Monogenic Mutations. Nephrol Dial Transplant (2020) 35(3):390–7. doi: 10.1093/ndt/gfz028
23. Cañadas-Garre M, Anderson K, McGoldrick J, Maxwell AP, McKnight AJ. Genomic Approaches in the Search for Molecular Biomarkers in Chronic Kidney Disease. J Transl Med (2018) 16(1):292. doi: 10.1186/s12967-018-1664-7
24. Tin A, Köttgen A. Genome-Wide Association Studies of CKD and Related Traits. Clin J Am Soc Nephrol (2020) 15(11):1643–56. doi: 10.2215/CJN.00020120
25. Wuttke M, Li Y, Li M, Sieber KB, Feitosa MF, Gorski M, et al. A Catalog of Genetic Loci Associated With Kidney Function From Analyses of a Million Individuals. Nat Genet (2019) 51(6):957–72. doi: 10.1038/s41588-019-0407-x
26. Stanzick KJ, Li Y, Schlosser P, Gorski M, Wuttke M, Thomas LF, et al. Discovery and Prioritization of Variants and Genes for Kidney Function in >1.2 Million Individuals. Nat Commun (2021) 12(1):4350. doi: 10.1038/s41467-021-24491-0
27. Gorski M, Jung B, Li Y, Matias-Garcia PR, Wuttke M, Coassin S, et al. Meta-Analysis Uncovers Genome-Wide Significant Variants for Rapid Kidney Function Decline. Kidney Int (2021) 99(4):926–39. doi: 10.1016/j.kint.2020.09.030
28. Anderson K, Cañadas-Garre M, Chambers R, Maxwell AP, McKnight AJ. The Challenges of Chromosome Y Analysis and the Implications for Chronic Kidney Disease. Front Genet (2019) 10:781. doi: 10.3389/fgene.2019.00781
29. Manolio TA, Collins FS, Cox NJ, Goldstein DB, Hindorff LA, Hunter DJ, et al. Finding the Missing Heritability of Complex Diseases. Nature (2009) 461(7265):747–53. doi: 10.1038/nature08494
30. McCarthy MI, Abecasis GR, Cardon LR, Goldstein DB, Little J, Ioannidis JPA, et al. Genome-Wide Association Studies for Complex Traits: Consensus, Uncertainty and Challenges. Nat Rev Genet (2008) 9(5):356–69. doi: 10.1038/nrg2344
31. Reich DE, Lander ES. On the Allelic Spectrum of Human Disease. Trends Genet (2001) 17(9):502–10. doi: 10.1016/S0168-9525(01)02410-6
32. Pritchard JK. Are Rare Variants Responsible for Susceptibility to Complex Diseases? Am J Hum Genet (2001) 69(1):124–37. doi: 10.1086/321272
33. Collins F, Guyer M, Charkravarti A. Variations on a Theme: Cataloging Human DNA Sequence Variation. Science (1997) 278(5343):1580–1. doi: 10.1126/science.278.5343.1580
34. Wise AL, Gyi L, Manolio TA. EXclusion: Toward Integrating the X Chromosome in Genome-Wide Association Analyses. Am J Hum Genet (2013) 92(5):643–7. doi: 10.1016/j.ajhg.2013.03.017
35. De Coster W, Weissensteiner MH, Sedlazeck FJ. Towards Population-Scale Long-Read Sequencing. Nat Rev Genet (2021) 22(9):572–87. doi: 10.1038/s41576-021-00367-3
36. Ebert P, Audano PA, Zhu Q, Rodriguez-Martin B, Porubsky D, Jan Bonder M, et al. Haplotype-Resolved Diverse Human Genomes and Integrated Analysis of Structural Variation. Science (2021) 372(6537):eabf7117. doi: 10.1126/science.abf7117
37. Martin S, Heavens D, Lan Y, Horsfield S, Clark MD, Leggett RM. Nanopore Adaptive Sampling: A Tool for Enrichment of Low Abundance Species in Metagenomic Samples. Genome Biol (2022) 23(11):1–27. doi: 10.1186/s13059-021-02582-x
38. Zuk O, Hechter E, Sunyaev SR, Lander ES. The Mystery of Missing Heritability: Genetic Interactions Create Phantom Heritability. Proc Natl Acad Sci U S A (2011) 109(4):1193–8. doi: 10.1073/pnas.1119675109
39. Bowden J, Holmes MV. Meta-Analysis and Mendelian Randomization: A Review. Res Synth Methods (2019) 10(4):486–96. doi: 10.1002/jrsm.1346
40. Gurung RL, Dorajoo R, M Y, Wang L, Liu S, Liu J-J, et al. Association of Leukocyte Telomere Length With Chronic Kidney Disease in East Asians With Type 2 Diabetes: A Mendelian Randomization Study. Clin Kidney J (2021) 14(11):2371–6. doi: 10.1093/ckj/sfab067
41. Park S, Lee S, Kim Y, Cho S, Kim K, Kim YC, et al. A Mendelian Randomization Study Found Causal Linkage Between Telomere Attrition and Chronic Kidney Disease. Kidney Int (2021) 100(5):1063–70. doi: 10.1016/j.kint.2021.06.041
42. Taub MA, Conomos MP, Keener R, Pankratz N, Reiner AP, Mathias RA. Genetic Determinants of Telomere Length From 109,122 Ancestrally Diverse Whole-Genome Sequences in TOPMed. Cell Genomics (2022) 2(1):100084. doi: 10.1016/j.xgen.2021.100084
43. Zhao JV, Schooling CM. Sex-Specific Associations of Sex Hormone Binding Globulin With CKD and Kidney Function: A Univariable and Multivariable Mendelian Randomization Study in the UK Biobank. J Am Soc Nephrol (2021) 32(3):686–94. doi: 10.1681/ASN.2020050659
44. Zhao JV, Schooling CM. The Role of Testosterone in Chronic Kidney Disease and Kidney Function in Men and Women: A Bi-Directional Mendelian Randomization Study in the UK Biobank. BMC Med (2020) 18(1):122. doi: 10.1186/s12916-020-01594-x
45. Kennedy OJ, Pirastu N, Poole R, Fallow JA, Hayes PC, Grzeszkowiak EJ, et al. Coffee Consumption and Kidney Function: A Mendelian Randomization Study. Am J Kidney Dis (2020) 75(5):753–61. doi: 10.1053/j.ajkd.2019.08.025
46. Park S, Lee S, Kim Y, Lee Y, Kang MW, Kim K, et al. Causal Effects of Relative Fat, Protein, and Carbohydrate Intake on Chronic Kidney Disease: A Mendelian Randomization Study. Am J Clin Nutr (2021) 113(4):1023–31. doi: 10.1093/ajcn/nqaa379
47. Park S, Lee S, Kim Y, Lee Y, Kang MW, Kim K, et al. Causal Effects of Physical Activity or Sedentary Behaviors on Kidney Function: An Integrated Population-Scale Observational Analysis and Mendelian Randomization Study. Nephrol Dial Transplant (2021) 37(6):1059–68. doi: 10.1093/ndt/gfab153.
48. Park S, Lee S, Kim Y, Lee Y, Kang MW, Kim K, et al. Causal Effects of Education on Chronic Kidney Disease: A Mendelian Randomization Study. Clin Kidney J (2020) 14(8):1932–8. doi: 10.1093/ckj/sfaa240
49. Zhang L, Cao W. Histone Deacetylase 3 (HDAC3) as an Important Epigenetic Regulator of Kidney Diseases. J Mol Med (2021) 100(1):43–51. doi: 10.1007/s00109-021-02141-8
50. Ingrosso D, Perna AF. DNA Methylation Dysfunction in Chronic Kidney Disease. Genes (Basel) (2020) 11(7):811. doi: 10.3390/genes11070811
51. Ptashne M. On the Use of the Word ‘Epigenetic’. Curr Biol (2007) 17(7):R233–6. doi: 10.1016/j.cub.2007.02.030
52. Greally JM, Drake AJ. The Current State of Epigenetic Research in Humans Promise and Reality. JAMA Pediatr (2017) 171(2):103–4. doi: 10.1001/jamapediatrics.2016.3508
53. Ptashne M. Epigenetics: Core Misconcept. Proc Natl Acad Sci U S A (2013) 110(18):7101–3. doi: 10.1073/pnas.1305399110
54. Kim M, Costello J. DNA Methylation: An Epigenetic Mark of Cellular Memory. Exp Mol Med (2017) 49(4):e322. doi: 10.1038/emm.2017.10
55. Smith ZD, Meissner A. DNA Methylation: Roles in Mammalian Development. Nat Rev Genet (2013) 14(3):204–20. doi: 10.1038/nrg3354
56. Lister R, Pelizzola M, Dowen RH, Hawkins RD, Hon G, Tonti-filippini J, et al. Human DNA Methylomes at Base Resolution Show Widespread Epigenomic Differences. Nature (2009) 462(7271):315–22. doi: 10.1038/nature08514
57. Luo Y, Lu X, Xie H. Dynamic Alu Methylation During Normal Development, Aging, and Tumorigenesis. BioMed Res Int (2014) 2014:784706. doi: 10.1155/2014/784706
58. Deaton M, Bird A. CpG Islands and the Regulation of Transcription. Genes Dev (2011) 25(10):1010–22. doi: 10.1101/gad.2037511
59. Babenko VN, Chadaeva IV, Orlov YL. Genomic Landscape of CpG Rich Elements in Human. BMC Evol Biol (2017) 17(Suppl 1):19. doi: 10.1186/s12862-016-0864-0
60. Jones PA. Functions of DNA Methylation: Islands, Start Sites, Gene Bodies and Beyond. Nat Rev Genet (2012) 13(7):484–92. doi: 10.1038/nrg3230
61. Zhou J, Sears RL, Xing X, Zhang B, Li D, Rockweiler NB, et al. Tissue-Specific DNA Methylation is Conserved Across Human, Mouse, and Rat, and Driven by Primary Sequence Conservation. BMC Genomics (2017) 18(1):724. doi: 10.1186/s12864-017-4115-6
62. Illingworth RS, Gruenewald-Schneider U, Webb S, Kerr ARW, James KD, Turner DJ, et al. Orphan CpG Islands Identify Numerous Conserved Promoters in the Mammalian Genome. PloS Genet (2010) 6(9):e1001134. doi: 10.1371/journal.pgen.1001134
63. Fang H, Disteche CM, Berletch JB. X Inactivation and Escape: Epigenetic and Structural Features. Front Cell Dev Biol (2019) 7:219. doi: 10.3389/fcell.2019.00219
64. Lyon MF. Gene Action in the X-Chromosome of the Mouse (Mus Musculus L.). Nature (1961) 190:372–3. doi: 10.1038/190372a0
65. Peters J. The Role of Genomic Imprinting in Biology and Disease: An Expanding View. Nat Rev Genet (2014) 15(8):517–30. doi: 10.1038/nrg3766
66. Greenberg MVC, Bourc’his D. The Diverse Roles of DNA Methylation in Mammalian Development and Disease. Nat Rev Mol Cell Biol (2019) 20(10):590–607. doi: 10.1038/s41580-019-0159-6
67. Han H, Cortez CC, Yang X, Nichols PW, Jones PA, Liang G. DNA Methylation Directly Silences Genes With non-CpG Island Promoters and Establishes a Nucleosome Occupied Promoter. Hum Mol Genet (2011) 20(22):4299–310. doi: 10.1093/hmg/ddr356
68. Okano M, Bell DW, Haber DA, Li E. DNA Methyltransferases Dnmt3a and Dnmt3b Are Essential for De Novo Methylation and Mammalian Development. Cell (1999) 99(3):247–57. doi: 10.1016/S0092-8674(00)81656-6
69. Jin Z, Liu Y. DNA Methylation in Human Diseases. Genes Dis (2018) 5(1):1–8. doi: 10.1016/j.gendis.2018.01.002
70. Tiffon C. The Impact of Nutrition and Environmental Epigenetics on Human Health and Disease. Int J Mol Sci (2018) 19(11):3425. doi: 10.3390/ijms19113425
71. Smyth LJ, Duffy S, Maxwell AP, McKnight AJ. Genetic and Epigenetic Factors Influencing Chronic Kidney Disease. Am J Physiol Renal Physiol (2014) 307(7):F757–76. doi: 10.1152/ajprenal.00306.2014
72. Golestaneh L, Melamed ML, Hostetter TH. Uremic Memory: The Role of Acute Kidney Injury in Long-Term Outcomes. Kidney Int (2009) 76(8):813–4. doi: 10.1038/ki.2009.314
73. Keating ST, El-Osta A. Glycemic Memories and the Epigenetic Component of Diabetic Nephropathy. Curr Diabetes Rep (2013) 13(4):574–81. doi: 10.1007/s11892-013-0383-y
74. Pirola L, Balcerczyk A, Okabe J, El-Osta A. Epigenetic Phenomena Linked to Diabetic Complications. Nat Rev Endocrinol (2010) 6(12):665–75. doi: 10.1038/nrendo.2010.188
75. Villeneuve LM, Natarajan R. The Role of Epigenetics in the Pathology of Diabetic Complications. Am J Physiol Renal Physiol (2010) 299(1):F14–25. doi: 10.1152/ajprenal.00200.2010
76. Villeneuve LM, Reddy MA, Lanting LL, Wang M, Meng L, Natarajan R. Epigenetic Histone H3 Lysine 9 Methylation in Metabolic Memory and Inflammatory Phenotype of Vascular Smooth Muscle Cells in Diabetes. Proc Natl Acad Sci U S A (2008) 105(26):9047–52. doi: 10.1073/pnas.0803623105
77. Chen XJ, Zhang H, Yang F, Liu Y, Chen G. DNA Methylation Sustains “Inflamed” Memory of Peripheral Immune Cells Aggravating Kidney Inflammatory Response in Chronic Kidney Disease. Front Physiol (2021) 12:637480. doi: 10.3389/fphys.2021.637480
78. Kato M, Natarajan R. Epigenetics and Epigenomics in Diabetic Kidney Disease and Metabolic Memory. Nat Rev Nephrol (2019) 15(6):327–45. doi: 10.1038/s41581-019-0135-6
79. Zhao J, Miao K, Wang H, Ding H, Wang DW. Association Between Telomere Length and Type 2 Diabetes Mellitus: A Meta-Analysis. PloS One (2013) 8(11):e79993. doi: 10.1371/journal.pone.0079993
80. Jeanclos E, Krolewski A, Skurnick J, Kimura M, Aviv H, Warram JH, et al. Shortened Telomere Length in White Blood Cells of Patients With IDDM. Diabetes (1998) 47(3):482–6. doi: 10.2337/diabetes.47.3.482
81. Adaikalakoteswari A, Balasubramanyam M, Mohan V. Telomere Shortening Occurs in Asian Indian Type 2 Diabetic Patients. Diabetes Med (2005) 22(9):1151–6. doi: 10.1111/j.1464-5491.2005.01574.x
82. Testa R, Olivieri F, Sirolla C, Spazzafumo L, Rippo MR, Marra M, et al. Leukocyte Telomere Length is Associated With Complications of Type 2 Diabetes Mellitus. Diabetes Med (2011) 28(11):1388–94. doi: 10.1111/j.1464-5491.2011.03370.x
83. White WE, Yaqoob M, Harwood SM. Aging and Uremia: Is There Cellular and Molecular Crossover? World J Nephrol (2015) 4(1):19–30. doi: 10.5527/wjn.v4.i1.19
84. Guo J, Zheng HJ, Zhang W, Lou W, Xia C, Han XT, et al. Accelerated Kidney Aging in Diabetes Mellitus. Oxid Med Cell Longev (2020) 2020:1234059. doi: 10.1155/2020/1234059
85. Liu J, Yang JR, Chen XM, Cai GY, Lin LR, He YN. Impact of ER Stress-Regulated ATF4/p16 Signaling on the Premature Senescence of Renal Tubular Epithelial Cells in Diabetic Nephropathy. Am J Physiol Cell Physiol (2015) 308(8):C621–30. doi: 10.1152/ajpcell.00096.2014
86. Verzola D, Gandolfo MT, Gaetani G, Ferraris A, Mangerini R, Ferrario F, et al. Accelerated Senescence in the Kidneys of Patients With Type 2 Diabetic Nephropathy. Am J Physiol Renal Physiol (2008) 295(5):F1563–1573. doi: 10.1152/ajprenal.90302.2008
87. Levine ME, Lu AT, Quach A, Chen BH, Assimes TL, Bandinelli S, et al. An Epigenetic Biomarker of Aging for Lifespan and Healthspan. Ageing (2018) 10(4):573–91. doi: 10.18632/aging.101414
88. Lu AT, Quach A, Wilson JG, Reiner AP, Aviv A, Raj K, et al. DNA Methylation GrimAge Strongly Predicts Lifespan and Healthspan. Aging (Albany NY) (2019) 11(2):303–27. doi: 10.18632/aging.101684
89. Horvath S. DNA Methylation Age of Human Tissues and Cell Types. Genome Biol (2013) 14(10):R15. doi: 10.1186/gb-2013-14-10-r115
90. Horvath S, Raj K. DNA Methylation-Based Biomarkers and the Epigenetic Clock Theory of Ageing. Nat Rev Genet (2018) 19(6):371–84. doi: 10.1038/s41576-018-0004-3
91. Matías-García PR, Ward-Caviness CK, Raffield LM, Gao X, Zhang Y, Wilson R, et al. DNAm-Based Signatures of Accelerated Aging and Mortality in Blood are Associated With Low Renal Function. Clin Epigenetics (2021) 13(1):121. doi: 10.1186/s13148-021-01082-w
92. Levine ME, Lu AT, Bennett DA, Horvath S. Epigenetic Age of the Pre-Frontal Cortex is Associated With Neuritic Plaques, Amyloid Load, and Alzheimer’s Disease Related Cognitive Functioning. Aging (Albany NY) (2015) 7(12):1198–211. doi: 10.18632/aging.100864
93. Lu AT, Hannon E, Levine ME, Crimmins EM, Lunnon K, Mill J, et al. Genetic Architecture of Epigenetic and Neuronal Ageing Rates in Human Brain Regions. Nat Commun (2017) 8:15353. doi: 10.1038/ncomms15353
94. Levine ME, Hosgood HD, Chen B, Absher D, Assimes T, Horvath S. DNA Methylation Age of Blood Predicts Future Onset of Lung Cancer in the Women’s Health Initiative. Aging (Albany NY) (2015) 7(9):690–700. doi: 10.18632/aging.100809
95. Zheng Y, Joyce BT, Colicino E, Liu L, Zhang W, Dai Q, et al. Blood Epigenetic Age may Predict Cancer Incidence and Mortality. EBioMedicine (2016) 5:68–73. doi: 10.1016/j.ebiom.2016.02.008
96. Dugué PA, Bassett JK, Joo JHE, Jung CH, Ming Wong E, Moreno-Betancur M, et al. DNA Methylation-Based Biological Aging and Cancer Risk and Survival: Pooled Analysis of Seven Prospective Studies. Int J Cancer (2018) 142(8):1611–9. doi: 10.1002/ijc.31189
97. Ambatipudi S, Horvath S, Perrier F, Cuenin C, Hernandez- H, Le Calvez-kelm F, et al. DNA Methylome Analysis Identifies Accelerated Epigenetic Ageing Associated With Postmenopausal Breast Cancer Susceptibility. Eur J Cancer (2018) 75:299–307. doi: 10.1016/j.ejca.2017.01.014
98. Grant CD, Jafari N, Hou L, Li Y, Stewart JD, Zhang G, et al. A Longitudinal Study of DNA Methylation as a Potential Mediator of Age-Related Diabetes Risk. GeroScience (2017) 39(5–6):475–89. doi: 10.1007/s11357-017-0001-z
99. Roshandel D, Chen Z, Canty AJ, Bull SB, Natarajan R, Paterson AD. DNA Methylation Age Calculators Reveal Association With Diabetic Neuropathy in Type 1 Diabetes. Clin Epigenetics (2020) 12(1):52. doi: 10.1186/s13148-020-00840-6
100. Bressler J, Fornage M, Studenski S, Vandiver AR, Tanaka T, Kiel DP, et al. DNA Methylation - Based Measures of Biological Age: Meta - Analysis Predicting Time to Death. Aging (Albany NY) (2016) 8(9):1844–65. doi: 10.18632/aging.101168
101. Quach A, Levine ME, Tanaka T, Lu AT, Chen BH, Ferrucci L, et al. Epigenetic Clock Analysis of Diet, Exercise, Education, and Lifestyle Factors. Aging (Albany NY) (2017) 29(1):20–1. doi: 10.18632/aging.101168
102. Gao X, Gào X, Zhang Y, Holleczek B, Schöttker B, Brenner H. Oxidative Stress and Epigenetic Mortality Risk Score: Associations With All-Cause Mortality Among Elderly People. Eur J Epidemiol (2019) 34(5):451–62. doi: 10.1007/s10654-019-00493-7
103. Charlton A, Garzarella J, Jandeleit-Dahm KAM, Jha JC. Oxidative Stress and Inflammation in Renal and Cardiovascular Complications of Diabetes. Biology (Basel) (2021) 10(1):18. doi: 10.3390/biology10010018
104. Rapa SF, Di Iorio BR, Campiglia P, Heidland A, Marzocco S. Inflammation and Oxidative Stress in Chronic Kidney Disease—Potential Therapeutic Role of Minerals, Vitamins and Plant-Derived Metabolites. Int J Mol Sci (2019) 21(1):263. doi: 10.3390/ijms21010263
105. Libetta C, Sepe V, Esposito P, Galli F, Dal Canton A. Oxidative Stress and Inflammation: Implications in Uremia and Hemodialysis. Clin Biochem (2011) 44(14–15):1189–98. doi: 10.1016/j.clinbiochem.2011.06.988
106. Popolo A, Autore G, Pinto A, Marzocco S. Oxidative Stress in Patients With Cardiovascular Disease and Chronic Renal Failure. Free Radic Res (2013) 47(5):346–56. doi: 10.3109/10715762.2013.779373
107. Tecklenborg J, Clayton D, Siebert S, Coley SM. The Role of the Immune System in Kidney Disease. Clin Exp Immunol (2018) 192(2):142–50. doi: 10.1111/cei.13119
108. Kooman JP, Kotanko P, Schols AMWJ, Shiels PG, Stenvinkel P. Chronic Kidney Disease and Premature Ageing. Nat Rev Nephrol (2014) 10(12):732–42. doi: 10.1038/nrneph.2014.185
109. Pidsley R, Zotenko E, Peters TJ, Lawrence MG, Risbridger GP, Molloy P, et al. Critical Evaluation of the Illumina MethylationEPIC BeadChip Microarray for Whole-Genome DNA Methylation Profiling. Genome Biol (2016) 17(1):208. doi: 10.1186/s13059-016-1066-1
110. Moran S, Arribas C, Esteller M. Validation of a DNA Methylation Microarray for 850,000 CpG Sites of the Human Genome Enriched in Enhancer Sequences. Epigenomics (2016) 8(3):389–99. doi: 10.2217/epi.15.114
111. Sharifian R, Okamura DM, Denisenko O, Zager RA, Johnson A, Gharib SA, et al. Distinct Patterns of Transcriptional and Epigenetic Alterations Characterize Acute and Chronic Kidney Injury. Sci Rep (2018) 8(1):17870. doi: 10.1038/s41598-018-35943-x
112. Flanagin S, Nelson JD, Castner DG, Denisenko O, Bomsztyk K. Microplate-Based Chromatin Immunoprecipitation Method, Matrix ChIP: A Platform to Study Signaling of Complex Genomic Events. Nucleic Acids Res (2008) 36(3):e17. doi: 10.1093/nar/gkn001
113. Walters BJ, Cox BC. Approaches for the Study of Epigenetic Modifications in the Inner Ear and Related Tissues. Hear Res (2019) 376:69–85. doi: 10.1016/j.heares.2019.01.007
114. Liu Y, Rosikiewicz W, Pan Z, Jillette N, Wang P, Taghbalout A, et al. DNA Methylation-Calling Tools for Oxford Nanopore Sequencing: A Survey and Human Epigenome-Wide Evaluation. Genome Biol (2021) 22(1):295. doi: 10.1186/s13059-021-02510-z
115. Smyth LJ, Patterson CC, Swan EJ, Maxwell AP, McKnight AJ. DNA Methylation Associated With Diabetic Kidney Disease in Blood-Derived DNA. Front Cell Dev Biol (2020) 8:561907. doi: 10.3389/fcell.2020.561907
116. Lecamwasam A, Novakovic B, Meyer B, Ekinci EI, Dwyer KM, Saffery R. DNA Methylation Profiling Identifies Epigenetic Differences Between Early Versus Late Stages of Diabetic Chronic Kidney Disease. Nephrol Dial Transplant (2021) 36(11):2027–38. doi: 10.1093/ndt/gfaa226
117. Schlosser P, Tin A, Matias-Garcia PR, Thio CHL, Joehanes R, Liu H, et al. Meta-Analyses Identify DNA Methylation Associated With Kidney Function and Damage. Nat Commun (2021) 12(1):7174. doi: 10.1038/s41467-021-27234-3
118. Smyth LJ, Kilner J, Nair V, Liu H, Brennan E, Kerr K, et al. Assessment of Differentially Methylated Loci in Individuals With End-Stage Kidney Disease Attributed to Diabetic Kidney Disease: An Exploratory Study. Clin Epigenetics (2021) 13(1):99. doi: 10.1186/s13148-021-01081-x
119. Breeze CE, Batorsky A, Lee MK, Szeto MD, Xu X, McCartney DL, et al. Epigenome-Wide Association Study of Kidney Function Identifies Trans-Ethnic and Ethnic-Specific Loci. Genome Med (2021) 13(1):74. doi: 10.1186/s13073-021-00877-z
120. Marumo T, Hoshino J, Kawarazaki W, Nishimoto M, Ayuzawa N, Hirohama D, et al. Methylation Pattern of Urinary DNA as a Marker of Kidney Function Decline in Diabetes. BMJ Open Diabetes Res Care (2020) 8(1):e001501. doi: 10.1136/bmjdrc-2020-001501
121. Chu AY, Tin A, Schlosser P, Ko Y, Qiu C, Yao C, et al. Epigenome-Wide Association Studies Identify DNA Methylation Associated With Kidney Function. Nat Commun (2017) 8(1):1286. doi: 10.1038/s41467-017-01297-7
122. Smyth LJ, Maxwell AP, Benson KA, Kilner J, McKay GJ, McKnight AJ. Validation of Differentially Methylated microRNAs Identified From an Epigenome-Wide Association Study; Sanger and Next Generation Sequencing Approaches. BMC Res Notes (2018) 11(1):767. doi: 10.1186/s13104-018-3872-x
123. Park J, Guan Y, Sheng X, Gluck C, Seasock MJ, Ari Hakimi A, et al. Functional Methylome Analysis of Human Diabetic Kidney Disease. JCI Insight (2019) 4(11):e128886. doi: 10.1172/jci.insight.128886
124. Gluck C, Qiu C, Han SY, Palmer M, Park J, Ko Y, et al. Kidney Cytosine Methylation Changes Improve Renal Function Decline Estimation in Patients With Diabetic Kidney Disease. Nat Commun (2019) 10(1):2461. doi: 10.1038/s41467-019-10378-8
125. Qiu C, Hanson RL, Fufaa G, Kobes S, Gluck C, Huang J, et al. Cytosine Methylation Predicts Renal Function Decline in American Indians. Kidney Int (2019) 93(6):1417–31. doi: 10.1016/j.kiny.2018.01.036
126. Chen J, Huang Y, Hui Q, Mathur R, Gwinn M, So-Armah K, et al. Epigenetic Associations With Estimated Glomerular Filtration Rate Among Men With Human Immunodeficiency Virus Infection. Clin Infect Dis (2020) 70(4):667–73. doi: 10.1093/cid/ciz240
127. Sheng X, Qiu C, Liu H, Gluck C, Hsu JY, He J, et al. Systematic Integrated Analysis of Genetic and Epigenetic Variation in Diabetic Kidney Disease. Proc Natl Acad Sci U S A (2020) 117(46):29013–24. doi: 10.1073/pnas.2005905117
128. Fontecha-Barriuso M, Martin-Sanchez D, Ruiz-Andres O, Poveda J, Sanchez-Niño MD, Valiño-Rivas L, et al. Targeting Epigenetic DNA and Histone Modifications to Treat Kidney Disease. Nephrol Dial Transplant (2018) 33(11):1875–86. doi: 10.1093/ndt/gfy009
129. Martinez-Moreno JM, Fontecha-Barriuso M, Martin-Sanchez D, Guerrero-Mauvecin J, Goma-Garces E, Fernandez-Fernandez B, et al. Epigenetic Modifiers as Potential Therapeutic Targets in Diabetic Kidney Disease. Int J Mol Sci (2020) 21(11):4113. doi: 10.3390/ijms21114113
130. Tanemoto F, Mimura I. Therapies Targeting Epigenetic Alterations in Acute Kidney Injury-To-Chronic Kidney Disease Transition. Pharmaceuticals (2022) 15(2):123. doi: 10.3390/ph15020123
131. Zinellu A, Sotgia S, Sotgiu E, Assaretti S, Baralla A, Mangoni AA, et al. Cholesterol Lowering Treatment Restores Blood Global DNA Methylation in Chronic Kidney Disease (CKD) Patients. Nutr Metab Cardiovasc Dis (2017) 27(9):822–9. doi: 10.1016/j.numecd.2017.06.011
132. Larkin BP, Glastras SJ, Chen H, Pollock CA, Saad S. DNA Methylation and the Potential Role of Demethylating Agents in Prevention of Progressive Chronic Kidney Disease. FASEB J (2018) 32(10):5215–26. doi: 10.1096/fj.201800205R
133. Shan Q, Zheng G, Zhu A, Cao L, Lu J, Wu D, et al. Epigenetic Modification of miR-10a Regulates Renal Damage by Targeting CREB1 in Type 2 Diabetes Mellitus. Toxicol Appl Pharmacol (2016) 306:134–43. doi: 10.1016/j.taap.2016.06.010
134. Marumo T, Yagi S, Kawarazaki W, Nishimoto M. Diabetes Induces Aberrant DNA Methylation in the Proximal Tubules of the Kidney. J Am Soc Nephrol (2015) 26(10):2388–97. doi: 10.1681/ASN.2014070665
135. Chen G, Chen H, Ren S, Xia M, Zhu J, Liu Y, et al. Aberrant DNA Methylation of mTOR Pathway Genes Promotes Inflammatory Activation of Immune Cells in Diabetic Kidney Disease. Kidney Int (2019) 96(2):409–20. doi: 10.1016/j.kint.2019.02.020
136. Li Z, Li N. Epigenetic Modification Drives Acute Kidney Injury-To-Chronic Kidney Disease Progression. Nephron (2021) 145(6):737–47. doi: 10.1159/000517073
137. Dritsoula A, Kislikova M, Oomatia A, Webster AP, Beck S, Ponticos M, et al. Epigenome-Wide Methylation Profile of Chronic Kidney Disease-Derived Arterial DNA Uncovers Novel Pathways in Disease-Associated Cardiovascular Pathology. Epigenetics (2021) 16(7):718–28. doi: 10.1080/15592294.2020.1819666
138. Onishi A, Sugiyama H, Kitagawa M, Yamanari T, Tanaka K, Ogawa-Akiyama A, et al. Urine 5medc, a Marker of DNA Methylation, in the Progression of Chronic Kidney Disease. Dis Markers (2019) 2019:5432453. doi: 10.1155/2019/5432453
139. Witasp A, Luttropp K, Qureshi AR, Barany P, Heimbürger O, Wennberg L, et al. Longitudinal Genome − Wide DNA Methylation Changes in Response to Kidney Failure Replacement Therapy. Sci Rep (2022) 12(1):470. doi: 10.1038/s41598-021-04321-5
140. Lowe R, Shirley N, Bleackley M, Dolan S, Shafee T. Transcriptomics Technologies. PloS Comput Biol (2017) 13(5):1–23. doi: 10.1371/journal.pcbi.1005457
141. Moreno JA, Hamza E, Guerrero-Hue M, Rayego-Mateos S, García-Caballero C, Vallejo-Mudarra M, et al. Non-Coding RNAs in Kidney Diseases: The Long and Short of Them. Int J Mol Sci (2021) 22(11):6077. doi: 10.3390/ijms22116077
142. Ren H, Wang Q. Non-Coding RNA and Diabetic Kidney Disease. DNA Cell Biol (2021) 40(4):553–67. doi: 10.1089/dna.2020.5973
143. Liu M, Ren J. Long Noncoding RNAs in Renal Diseases. ExRNA (2019) 1:27. doi: 10.1186/s41544-019-0033-x
144. Khurana R, Ranches G, Schafferer S, Lukasser M, Rudnicki M, Mayer G, et al. Identification of Urinary Exosomal Noncoding RNAs as Novel Biomarkers in Chronic Kidney Disease. RNA (2017) 23(2):142–52. doi: 10.1261/rna.058834.116
145. Sun IO, Lerman LO. Urinary microRNA in Kidney Disease: Utility and Roles. Am J Physiol Renal Physiol (2019) 316(5):F785–93. doi: 10.1152/ajprenal.00368.2018
146. Fukuda A, Minakawa A, Kikuchi M, Sato Y, Nagatomo M, Nakamura S, et al. Urinary Podocyte mRNAs Precede Microalbuminuria as a Progression Risk Marker in Human Type 2 Diabetic Nephropathy. Sci Rep (2020) 10(1):18209. doi: 10.1038/s41598-020-75320-1
147. Eissa S, Matboli M, Aboushahba R, Bekhet MM, Soliman Y. Urinary Exosomal microRNA Panel Unravels Novel Biomarkers for Diagnosis of Type 2 Diabetic Kidney Disease. J Diabetes Complications (2016) 30(8):1585–92. doi: 10.1016/j.jdiacomp.2016.07.012
148. Zhou LT, Lv LL, Qiu S, Yin Q, Li ZL, Tang TT, et al. Bioinformatics-Based Discovery of the Urinary BBOX1 mRNA as a Potential Biomarker of Diabetic Kidney Disease. J Transl Med (2019) 17(1):59. doi: 10.1186/s12967-019-1818-2
149. Wen L, Peng H. Whole Transcriptome Analysis of Diabetic Nephropathy in the Db/Db Mouse Model of Type 2 Diabetes. J Cell Biochem (2019) 120(10):17520–33. doi: 10.1002/jcb.29016
150. Liu L, Ma F, Hao Y, Yi Z, Yu X, Xu B, et al. Integrative Informatics Analysis of Transcriptome and Identification of Interacted Genes in the Glomeruli and Tubules in CKD. Front Med (2021) 7:615306. doi: 10.3389/fmed.2020.615306
151. Dhillon P, Park J, Hurtado del Pozo C, Li L, Doke T, Huang S, et al. The Nuclear Receptor ESRRA Protects From Kidney Disease by Coupling Metabolism and Differentiation. Cell Metab (2021) 33(2):379–394.e8. doi: 10.1016/j.cmet.2020.11.011
152. Doke T, Huang S, Qiu C, Liu H, Guan Y, Hu H, et al. Transcriptome-Wide Association Analysis Identifies DACH1 as a Kidney Disease Risk Gene That Contributes to Fibrosis. J Clin Invest (2021) 131(10):e141801. doi: 10.1172/JCI141801
153. Cao Y, Wang Y, Peng N, Xiao J, Wang S, Fu C. The Ratio of Urinary TREM-1/TREM-2 mRNA Expression in Chronic Kidney Disease and Renal Fibrosis. Ann Med (2021) 53(1):1010–8. doi: 10.1080/07853890.2021.1912384
154. Gu X, Yang H, Sheng X, Ko YA, Qiu C, Park J, et al. Kidney Disease Genetic Risk Variants Alter Lysosomal Beta-Mannosidase (MANBA) Expression and Disease Severity. Sci Transl Med (2021) 13(576):eaaz1458. doi: 10.1126/scitranslmed.aaz1458
155. Park J, Dhillon P, Hurtado C, Li L, Zhao J, Kang HM, et al. Renal Proximal Tubule Cell State and Metabolism are Coupled by Nuclear Receptors. bioRxiv (2020) 33(2):379–4.e8. doi: 10.1101/2020.09.21.307231
156. Chung KW, Dhillon P, Huang S, Sheng X, Shrestha R, Qiu C, et al. Mitochondrial Damage and Activation of the STING Pathway Lead to Renal Inflammation and Fibrosis. Cell Metab (2019) 30(4):784–799.e5. doi: 10.1016/j.cmet.2019.08.003
157. Miguel V, Tituaña J, Ignacio Herrero J, Herrero L, Serra D, Cuevas P, et al. Renal Tubule Cpt1a Overexpression Protects From Kidney Fibrosis by Restoring Mitochondrial Homeostasis. J Clin Invest (2021) 131(5):e140695. doi: 10.1172/JCI140695
158. Fan Y, Yi Z, Agati VDD, Sun Z, Zhong F, Zhang W, et al. Comparison of Kidney Transcriptomic Profiles of Early and Advanced Diabetic Nephropathy Reveals Potential New Mechanisms for Disease Progression. Diabetes (2019) 68(12):2301–14. doi: 10.2337/db19-0204
159. Levin A, Reznichenko A, Witasp A, Liu P, Greasley PJ, Sorrentino A, et al. Novel Insights Into the Disease Transcriptome of Human Diabetic Glomeruli and Tubulointerstitium. Nephrol Dial Transplant (2020) 35(12):2059–72. doi: 10.1093/ndt/gfaa121
160. Park J, Shrestha R, Qiu C, Kondo A, Huang S, Werth M, et al. Single-Cell Transcriptomics of the Mouse Kidney Reveals Potential Cellular Targets of Kidney Disease. Science (2018) 360(6390):758–63. doi: 10.1126/science.aar2131
161. Wu H, Malone AF, Donnelly EL, Kirita Y, Uchimura K, Ramakrishnan SM, et al. Single-Cell Transcriptomics of a Human Kidney Allograft Biopsy Specimen Defines Diverse Inflammatory Response. J Am Soc Nephrol (2018) 29(8):2069–80. doi: 10.1681/ASN.2018020125
162. Chang J, Yan J, Li X, Liu N, Zheng R, Zhong Y. Update on the Mechanisms of Tubular Cell Injury in Diabetic Kidney Disease. Front Med (2021) 8:661076. doi: 10.3389/fmed.2021.661076
163. Jiang M, Chen H, Guo G. Studying Kidney Diseases at the Single-Cell Level. Kidney Dis (2021) 7(5):335–42. doi: 10.1159/000517130
164. Muto Y, Wilson PC, Ledru N, Wu H, Dimke H, Waikar SS, et al. Single Cell Transcriptional and Chromatin Accessibility Profiling Redefine Cellular Heterogeneity in the Adult Human Kidney. Nat Commun (2021) 12(1):2190. doi: 10.1038/s41467-021-22368-w
165. Sheng X, Guan Y, Ma Z, Wu J, Liu H, Qiu C, et al. Mapping the Genetic Architecture of Human Traits to Cell Types in the Kidney Identifies Mechanisms of Disease and Potential Treatments. Nat Genet (2021) 53(9):1322–33. doi: 10.1038/s41588-021-00909-9
166. Gerhardt LMS, Liu J, Koppitch K, Cippà PE, McMahon AP. Single-Nuclear Transcriptomics Reveals Diversity of Proximal Tubule Cell States in a Dynamic Response to Acute Kidney Injury. Proc Natl Acad Sci U S A (2021) 118(27):e2026684118. doi: 10.1073/pnas.2026684118
167. Cañadas-Garre M, Anderson K, McGoldrick J, Maxwell AP, McKnight AJ. Proteomic and Metabolomic Approaches in the Search for Biomarkers in Chronic Kidney Disease. J Proteomics (2019) 193:93–122. doi: 10.1016/j.jprot.2018.09.020
168. Dubin RF, Rhee EP. Proteomics and Metabolomics in Kidney Disease, Including Insights Into Etiology, Treatment, and Prevention. Clin J Am Soc Nephrol (2020) 15(3):404–11. doi: 10.2215/CJN.07420619
169. Chen D, Cao G, Chen H, Argyopoulos CP, Yu H, Su W, et al. Identification of Serum Metabolites Associating With Chronic Kidney Disease Progression and Anti-Fibrotic Effect of 5-Methoxytryptophan. Nat Commun (2019) 10(1):1476. doi: 10.1038/s41467-019-09329-0
170. Fan G, Gong T, Lin Y, Wang J, Sun L, Wei H, et al. Urine Proteomics Identifies Biomarkers for Diabetic Kidney Disease at Different Stages. Clin Proteomics (2021) 18(1):32. doi: 10.1186/s12014-021-09338-6
171. Zhu S, Zhang F, Shen A-W, Sun B, Xia T-Y, Chen W-S, et al. Metabolomics Evaluation of Patients With Stage 5 Chronic Kidney Disease Before Dialysis, Maintenance Hemodialysis, and Peritoneal Dialysis Patients and Study Design. Front Physiol (2021) 11:630646. doi: 10.3389/fphys.2020.630646
172. Hu J, Grams ME, Coresh J, Hwang S, Kovesdy CP, Rhee EP, et al. Serum Metabolites and Cardiac Death in Patients on Hemodialysis. Clin J Am Soc Nephrol (2019) 14:747–9. doi: 10.2215/CJN.12691018
173. Braun F, Bachurski D, Buchner D, Bohl K, Antczak P, Späth MR, et al. The Proteomic Landscape of Small Urinary Extracellular Vesicles During Kidney Transplantation. J Extracell Vesicles (2020) 10(1):e12026. doi: 10.1002/jev2.12026
174. Bruce MA, Beech BM, Sims M, Brown TN, Wyatt SB, Taylor HA, et al. Social Environmental Stressors, Psychological Factors, and Kidney Disease. J Investig Med (2009) 57(4):583–9. doi: 10.2310/JIM.0b013e31819dbb91
175. Robles-Osorio ML, Sabath E. Social Disparities, Risk Factors and Chronic Kidney Disease. Nefrologia (2016) 36(5):577–9. doi: 10.1016/j.nefro.2016.05.004
176. Crews DC, Novick T. Social Determinants of CKD Hotspots. Semin Nephrol (2020) 39(3):256–62. doi: 10.1016/j.semnephrol.2019.02.003
177. Lapedis CJ, Mariani LH, Jang BJ, Hodgin J, Hicken MT. Understanding the Link Between Neighborhoods and Kidney Disease. Kidney360 (2020) 1(8):845–54. doi: 10.34067/KID.0001202019
178. Jung J, Park JY, Kim YC, Lee H, Kim E, Kim YS, et al. Effects of Air Pollution on Mortality of Patients With Chronic Kidney Disease: A Large Observational Cohort Study. Sci Total Environ (2021) 786:147471. doi: 10.1016/j.scitotenv.2021.147471
179. Chien JW, Yang YR, Chen SY, Chang YJ, Chan CC. Urban Open Space is Associated With Better Renal Function of Adult Residents in New Taipei City. Int J Environ Res Public Health (2019) 16(13):2436. doi: 10.3390/ijerph16132436
180. Hoffimann E, Barros H, Ribeiro AI. Socioeconomic Inequalities in Green Space Quality and Accessibility — Evidence From a Southern European City. Environ Res Public Heal (2017) 14:916. doi: 10.3390/ijerph14080916
181. Dzhambov AM, Tokmakova MP, Gatseva PD, Zdravkov NG, Gencheva DG, Ivanova NG, et al. Community Noise Exposure and its Effect on Blood Pressure and Renal Function in Patients With Hypertension and Cardiovascular Disease. Folia Med (Plovdiv) (2017) 59(3):344–56. doi: 10.1515/folmed-2017-0045
182. Kuźma Ł, Małyszko J, Bachórzewska-Gajewska H, Kralisz P, Dobrzycki S. Exposure to Air Pollution and Renal Function. Sci Rep (2021) 11(1):11419. doi: 10.1038/s41598-021-91000-0
183. Kim YJ, Choi WJ, Ham S, Kang SK, Lee W. Association Between Occupational or Environmental Noise Exposure and Renal Function Among Middle-Aged and Older Korean Adults: A Cross-Sectional Study. Sci Rep (2021) 11(1):24127. doi: 10.1038/s41598-021-03647-4
184. Hwang SY, Jeong S, Choi S, Kim DH, Kim SR, Lee G, et al. Association of Air Pollutants With Incident Chronic Kidney Disease in a Nationally Representative Cohort of Korean Adults. Int J Environ Res Public Health (2021) 18(7):3775. doi: 10.3390/ijerph18073775
185. McKinley JM, Mueller U, Atkinson PM, Ofterdinger U, Jackson C, Cox SF, et al. Investigating the Influence of Environmental Factors on the Incidence of Renal Disease With Compositional Data Analysis Using Balances. Appl Comput Geosci (2020) 6:100024. doi: 10.1016/j.acags.2020.100024
186. McKinley JM, Muelle U, Atkinson P, Ofterdinger U, Cox S, Doherty R, et al. Chronic Kidney Disease of Unknown Origin is Associated With Environmental Urbanisation in Belfast, UK. J Environ Geochem Heal (2021) 43(7):2597–614. doi: 10.1007/s10653-020-00618-y
187. Liang Z, Wang W, Wang Y, Ma L, Liang C, Li P, et al. Urbanization, Ambient Air Pollution, and Prevalence of Chronic Kidney Disease: A Nationwide Cross-Sectional Study. Environ Int (2021) 156:106752. doi: 10.1016/j.envint.2021.106752
188. Neill JO, Tabish H, Welch V, Petticrew M, Pottie K, Clarke M, et al. Applying an Equity Lens to Interventions: Using PROGRESS Ensures Consideration of Socially Stratifying Factors to Illuminate Inequities in Health. J Clin Epidemiol (2014) 67(1):56–64. doi: 10.1016/j.jclinepi.2013.08.005
189. Lee J, Oh S, Kang H, Kim S, Lee G, Li L, et al. Environment-Wide Association Study of CKD. Clin J Am Soc Nephrol (2020) 15(6):766–75. doi: 10.2215/CJN.06780619
190. Satarug S, Gobe GC, Vesey DA, Phelps KR. Cadmium and Lead Exposure, Nephrotoxicity, and Mortality. Toxics (2020) 8(4):86. doi: 10.3390/toxics8040086
191. Yimthiang S, Pouyfung P, Khamphaya T, Kuraeiad S. Effects of Environmental Exposure to Cadmium and Lead on the Risks of Diabetes and Kidney Dysfunction. Int J Environ Res Public Health (2022) 19(4):2259. doi: 10.3390/ijerph19042259
192. Orr SE, Bridges CC. Chronic Kidney Disease and Exposure to Nephrotoxic Metals. Int J Mol Sci (2017) 18(5):1039. doi: 10.3390/ijms18051039
193. Barregard L, Sallsten G, Lundh T, Johan M. Low-Level Exposure to Lead, Cadmium and Mercury, and Histopathological Findings in Kidney Biopsies. Environ Res (2022) 211:113119. doi: 10.1016/j.envres.2022.113119
194. Zheng Y, Chen Z, Pearson T, Zhao J, Hu H, Prosperi M. Design and Methodology Challenges of Environment-Wide Association Studies: A Systematic Review. Environ Res (2020) 183:109275. doi: 10.1016/j.envres.2020.109275
195. Cavalli G, Heard E. Advances in Epigenetics Link Genetics to the Environment and Disease. Nature (2019) 571:489–99. doi: 10.1038/s41586-019-1411-0
196. Provenzano M, De Nicola L, Pena MJ, Capitoli G, Garofalo C, Borrelli S, et al. Precision Nephrology Is a Non-Negligible State of Mind in Clinical Research: Remember the Past to Face the Future. Nephron (2020) 144(10):463–78. doi: 10.1159/000508983
197. Provenzano M, Serra R, Garofalo C, Michael A, Crugliano G, Battaglia Y, et al. OMICS in Chronic Kidney Disease: Focus on Prognosis and Prediction. Int J Mol Sci (2021) 23(1):336. doi: 10.3390/ijms23010336
198. Odenkirk MT, Reif DM, Baker ES. Multiomic Big Data Analysis Challenges: Increasing Confidence in the Interpretation of Artificial Intelligence Assessments. Anal Chem (2021) 93(22):7763–73. doi: 10.1021/acs.analchem.0c04850
199. Nurk S, Koren S, Arang R, Rautianen M, Al E. The Complete Sequence of a Human Genome. Science (2022) 376(6588):44–53. doi: 10.1126/science.abj6987
200. Spielmann M, Mundlos S. Looking Beyond the Genes: The Role of non-Coding Variants in Human Disease. Hum Mol Genet (2016) 25(R2):R157–65. doi: 10.1093/hmg/ddw205
201. Wishart DS, Guo A, Oler E, Wang F, Anjum A, Peters H, et al. HMDB 5.0: The Human Metabolome Database for 2022. Nucleic Acids Res (2022) 50:622–31. doi: 10.1093/nar/gkab1062
202. The Gene Ontology Consortium. Gene Ontology: Tool for the Unification of Biology. Nat Genet (2000) 25:25–9. doi: 10.1038/75556
203. The Gene Ontology Consortium. Expansion of the Gene Ontology Knowledgebase and Resources. Nucleic Acids Res (2017) 45:331–8. doi: 10.1093/nar/gkw1108
204. García-Campos MA, Espinal-Enríquez J, Hernández-Lemus E. Pathway Analysis: State of the Art. Front Physiol (2015) 6:383. doi: 10.3389/fphys.2015.00383
205. Wishart DS, Feunang YD, Marcu A, Guo AC, Liang K, Rosa V, et al. HMDB 4.0: The Human Metabolome Database for 2018. Nucleic Acids Res (2018) 46(D1):D608–17. doi: 10.1093/nar/gkx1089
206. Natale DA, Arighi CN, Blake JA, Bona J, Chen C, Chen S, et al. Protein Ontology (PRO): Enhancing and Scaling Up the Representation of Protein Entities. Nucleic Acids Res (2017) 45(D1):D339–46. doi: 10.1093/nar/gkw1075
207. The Gene Ontology Consortium. The Gene Ontology Resource: Enriching a GOld Mine. Nucleic Acids Res (2021) 49(D1):D325–34. doi: 10.1093/nar/gkaa1113
208. Kafkas S, Hoehndorf R. Ontology Based Text Mining of Gene-Phenotype Associations: Application to Candidate Gene Prediction. Database (2019) 2019:baz019. doi: 10.1093/database/baz019
209. Barupal DK, Fiehn O. Generating the Blood Exposome Database Using a Comprehensive Text Mining and Database Fusion Approach. Environ Health Perspect (2019) 127(9):97008. doi: 10.1289/EHP4713
210. Sullivan KM, Susztak K. Unravelling the Complex Genetics of Common Kidney Diseases: From Variants to Mechanisms. Nat Rev Nephrol (2020) 16(11):628–40. doi: 10.1038/s41581-020-0298-1
211. Aguet F, Brown AA, Castel SE, Davis JR, He Y, Jo B, et al. GTEx Consortium. Genetic effects on gene expression across human tissues. Nature (2017) 550(7675):204–13. doi: 10.1038/nature24277
212. Xu X, Eales JM, Akbarov A, Guo H, Becker L, Talavera D, et al. Molecular Insights Into Genome-Wide Association Studies of Chronic Kidney Disease-Defining Traits. Nat Commun (2018) 9(1):4800. doi: 10.1038/s41467-018-07260-4
213. Wu C, Zhou F, Ren J, Li X, Jiang Y, Ma S. A Selective Review of Multi-Level Omics Data Integration Using Variable Selection. High-Throughput (2019) 8(1):4. doi: 10.3390/ht8010004
214. Richardson S, Tseng GC, Sun W. Statistical Methods in Integrative Genomics. Annu Rev Stat Appl (2016) 3:181–209. doi: 10.1146/annurev-statistics-041715-033506
215. Stern JL, Paucek RD, Huang FW, Ghandi M, Nwumeh R, Costello JC, et al. Allele-Specific DNA Methylation and Its Interplay With Repressive Histone Marks at Promoter-Mutant TERT Genes. Cell Rep (2017) 21(13):3700–7. doi: 10.1016/j.celrep.2017.12.001
216. Cavalli M, Baltzer N, Umer HM, Grau J, Lemnian I, Pan G, et al. Allele Specific Chromatin Signals, 3D Interactions, and Motif Predictions for Immune and B Cell Related Diseases. Sci Rep (2019) 9(1):2695. doi: 10.1038/s41598-019-39633-0
217. Wilson PC, Muto Y, Wu H, Karihaloo A, Waikar SS. Multimodal Single Cell Sequencing of Human Diabetic Kidney Disease Implicates Chromatin Accessibility and Genetic Background in Disease Progression. bioRxiv (2022), 1–40. doi: 10.1101/2022.01.28.478204
218. Huang S, Shen L. Long Noncoding RNA NEAT1 Accelerates the Proliferation and Fibrosis in Diabetic Nephropathy Through Activating Akt/mTOR Signaling Pathway. J Cell Physiol (2019) 234(7):11200–7. doi: 10.1002/jcp.27770
219. Picard M, Scott-Boyer M, Bodein A, Périn O, Droit A. Integration Strategies of Multi-Omics Data for Machine Learning Analysis. Comput Struct Biotechnol J (2021) 19:3735–46. doi: 10.1016/j.csbj.2021.06.030
220. Subramanian I, Verma S, Kumar S, Jere A, Anamika K. Multi-Omics Data Integration, Interpretation, and Its Application. Bioinform Biol Insights (2020) 14:1–24. doi: 10.1177/1177932219899051
221. Song M, Greenbaum J, Luttrell J4, Zhou W, Wu C. A Review of Integrative Imputation for Multi-Omics Datasets. Front Genet (2020) 11:570255. doi: 10.3389/fgene.2020.570255
222. Krassowski M, Das V, Sahu SK, Misra BB. State of the Field in Multi-Omics Research: From Computational Needs to Data Mining and Sharing. Front Genet (2020) 11:610798. doi: 10.3389/fgene.2020.610798
223. Duan R, Gao L, Gao Y, Hu Y, Xu H, Huang M, et al. Evaluation and Comparison of Multi-Omics Data Integration Methods for Cancer Subtyping. PloS Comput Biol (2021) 17(8):e1009224. doi: 10.1371/journal.pcbi.1009224
224. Liu S, Gui Y, Wang MS, Zhang L, Xu T, Pan Y, et al. Serum Integrative Omics Reveals the Landscape of Human Diabetic Kidney Disease. Mol Metab (2021) 54:101367. doi: 10.1016/j.molmet.2021.101367
225. Guan Y, Liang X, Ma Z, Hu H, Liu H, Miao Z, et al. A Single Genetic Locus Controls Both Expression of DPEP1/CHMP1A and Kidney Disease Development via Ferroptosis. Nat Commun (2021) 12(1):5078. doi: 10.1038/s41467-021-25377-x
226. Miao Z, Balzer MS, Ma Z, Liu H, Wu J, Shrestha R, et al. Single Cell Regulatory Landscape of the Mouse Kidney Highlights Cellular Differentiation Programs and Disease Targets. Nat Commun (2021) 12(1):2277. doi: 10.1038/s41467-021-22266-1
227. Li Y, Haug S, Schlosser P, Teumer A, Tin A, Pattaro C, et al. Integration of GWAS Summary Statistics and Gene Expression Reveals Target Cell Types Underlying Kidney Function Traits. J Am Soc Nephrol (2020) 31(10):2326–40. doi: 10.1681/ASN.2020010051
228. Teumer A, Li Y, Ghasemi S, Prins BP, Wuttke M, Hermle T, et al. Genome-Wide Association Meta-Analyses and Fine-Mapping Elucidate Pathways Influencing Albuminuria. Nat Commun (2019) 10(1):4130. doi: 10.1038/s41467-019-11576-0
229. Hellwege JN, Velez Edwards DR, Giri A, Qiu C, Park J, Torstenson ES, et al. Mapping eGFR Loci to the Renal Transcriptome and Phenome in the VA Million Veteran Program. Nat Commun (2019) 10(1):3842. doi: 10.1038/s41467-019-11704-w
230. Wang B, Ji G, Naeem H, Wang J, Kantharidis P, Powell D, et al. The Use of Targeted Next Generation Sequencing to Explore Candidate Regulators of TGF-β1’s Impact on Kidney Cells. Front Physiol (2018) 9:1755. doi: 10.3389/fphys.2018.01755
231. Wilkinson MD, Dumontier M, Aalbersberg IJ, Appleton G, Axton M, Baak A, et al. The FAIR Guiding Principles for Scientific Data Management and Stewardship. Sci Data (2016) 3:160018. doi: 10.1038/sdata.2016.18
232. de Maturana EL, Alonso L, Alarcón P, Martín-Antoniano IA, Pineda S, Piorno L, et al. Challenges in the Integration of Omics and non-Omics Data. Genes (Basel) (2019) 10(3):238. doi: 10.3390/genes10030238
233. Shang N, Khan A, Polubriaginof F, Zanoni F, Mehl K, Fasel D, et al. Medical Records-Based Chronic Kidney Disease Phenotype for Clinical Care and “Big Data” Observational and Genetic Studies. NPJ Digit Med (2021) 4(1):70. doi: 10.1038/s41746-021-00428-1
234. eMerge Consortium. Lessons Learned From the eMERGE Network: Balancing Genomics in Discovery and Practice. Hum Genet Genomics Adv (2021) 2(1):100018. doi: 10.1016/j.xhgg.2020.100018
235. Bond KM, Mccarthy MM, Rubin JB, Swanson KR. Molecular Omics Resources Should Require Sex Annotation: A Call for Action. Nat Methods (2021) 18(6):585–8. doi: 10.1038/s41592-021-01168-6
236. Si H, Banga RS, Kapitsinou P, Ramaiah M, Lawrence J, Gruenwald A, et al. Human and Murine Kidneys Show Gender- and Species- Specific Gene Expression Differences in Response to Injury. PloS One (2009) 4(3):e4802. doi: 10.1371/journal.pone.0004802
237. McEvoy ACM, Murphy JM, Zhang L, Clotet-freixas S. Single-Cell Profiling of Healthy Human Kidney Reveals Features of Sex-Based Transcriptional Programs and Tissue-Specific Immunity. bioRxiv (2021), 1–42. doi: 10.1101/2021.12.09.471943
238. Sandholm N, Mcknight AJ, Salem RM, Brennan EP, Forsblom C, Harjutsalo V, et al. Chromosome 2q31.1 Associates With ESRD in Women With Type 1 Diabetes. J Am Soc Nephrol (2013) 24(10):1537–43. doi: 10.1681/ASN.2012111122
239. Graham SE, Nielsen JB, Zawistowski M, Zhou W, Fritsche LG, Gabrielsen ME, et al. Sex-Specific and Pleiotropic Effects Underlying Kidney Function Identified From GWAS Meta-Analysis. Nat Commun (2019) 10(1):1847. doi: 10.1038/s41467-019-09861-z
240. He R, Ai L, Zhang D, Wan L, Zheng T, Yin J, et al. Different Effect of Testosterone and Oestrogen on Urinary Excretion of Metformin via Regulating OCTs and MATEs Expression in the Kidney of Mice. J Cell Mol Med (2016) 20(12):2309–17. doi: 10.1111/jcmm.12922
241. Lickteig AJ, Cheng X, Augustine LM, Klaassen CD, Nathan J. Tissue Distribution, Ontogeny and Induction of the Transporters Multidrug and Toxin Extrusion (MATE) 1 and MATE2 mRNA Expression Levels in Mice Andrew. Life Sci (2008) 83(520):59–64. doi: 10.1016/j.lfs.2008.05.004
242. Loftfield E, Zhou W, Graubard BI, Yeager M, Chanock SJ, Freedman ND, et al. Predictors of Mosaic Chromosome Y Loss and Associations With Mortality in the UK Biobank. Sci Rep (2018) 8(1):12316. doi: 10.1038/s41598-018-30759-1
243. Arseneault M, Monlong J, Vasudev NS, Laskar RS, Safisamghabadi M, Harnden P, et al. Loss of Chromosome Y Leads to Down Regulation of KDM5D and KDM6C Epigenetic Modifiers in Clear Cell Renal Cell Carcinoma. Sci Rep (2017) 44876:44876. doi: 10.1038/srep44876
Keywords: biomarkers, CKD, data integration, DKD, kidney, multi-omic, review, therapeutics
Citation: Hill C, Avila-Palencia I, Maxwell AP, Hunter RF and McKnight AJ (2022) Harnessing the Full Potential of Multi-Omic Analyses to Advance the Study and Treatment of Chronic Kidney Disease. Front. Nephrol. 2:923068. doi: 10.3389/fneph.2022.923068
Received: 18 April 2022; Accepted: 30 May 2022;
Published: 27 June 2022.
Edited by:
Markus Pirklbauer, Innsbruck Medical University, AustriaReviewed by:
Mei Liu, University of Kansas Medical Center, United StatesGiulia Capitoli, University of Milano Bicocca, Italy
Copyright © 2022 Hill, Avila-Palencia, Maxwell, Hunter and McKnight. This is an open-access article distributed under the terms of the Creative Commons Attribution License (CC BY). The use, distribution or reproduction in other forums is permitted, provided the original author(s) and the copyright owner(s) are credited and that the original publication in this journal is cited, in accordance with accepted academic practice. No use, distribution or reproduction is permitted which does not comply with these terms.
*Correspondence: Amy Jayne McKnight, a.j.mcknight@qub.ac.uk