Repetitive tactile stimulation changes resting-state functional connectivity—implications for treatment of sensorimotor decline
- 1Bernstein Focus State Dependencies of Learning and Bernstein Center for Computational Neuroscience, Berlin, Germany
- 2Department of Neurology, Charité University Medicine, Berlin, Germany
- 3Department of Neurophysiology and Pathophysiology, University Medical Center Hamburg-Eppendorf, Hamburg, Germany
- 4Institute for Neuroinformatics, Neural Plasticity Lab, Ruhr-University Bochum, Germany
- 5Max Planck Institute for Human Cognitive and Brain Sciences, Leipzig, Germany
- 6Berlin School of Mind and Brain and Mind and Brain Institute, Humboldt University, Berlin, Germany
Neurological disorders and physiological aging can lead to a decline of perceptual abilities. In contrast to the conventional therapeutic approach that comprises intensive training and practicing, passive repetitive sensory stimulation (RSS) has recently gained increasing attention as an alternative to countervail the sensory decline by improving perceptual abilities without the need of active participation. A particularly effective type of high-frequency RSS, utilizing Hebbian learning principles, improves perceptual acuity as well as sensorimotor functions and has been successfully applied to treat chronic stroke patients and elderly subjects. High-frequency RSS has been shown to induce plastic changes of somatosensory cortex such as representational map reorganization, but its impact on the brain's ongoing network activity and resting-state functional connectivity has not been investigated so far. Here, we applied high-frequency RSS in healthy human subjects and analyzed resting state Electroencephalography (EEG) functional connectivity patterns before and after RSS by means of imaginary coherency (ImCoh), a frequency-specific connectivity measure which is known to reduce over-estimation biases due to volume conduction and common reference. Thirty minutes of passive high-frequency RSS lead to significant ImCoh-changes of the resting state mu-rhythm in the individual upper alpha frequency band within distributed sensory and motor cortical areas. These stimulation induced distributed functional connectivity changes likely underlie the previously observed improvement in sensorimotor integration.
Introduction
Pathological changes in neuronal functioning lead to a decline of perceptual and sensory abilities. The most obvious cause is a damage of sensory brain areas due to trauma or stroke (Carey et al., 1993; Feys et al., 1998; Rosamond et al., 2007), but also a variety of neurological disorders such as Parkinson's disease (Koller, 1984; Sathian et al., 1997) or dystonia (Tinazzi et al., 2003; Stamelou et al., 2011) can affect perceptual and sensory function, possibly by a dysfunction of the sensorimotor network (Silberstein et al., 2005; Tamura et al., 2009; Litvak et al., 2011). In addition, a more natural but progressive decline of perceptual and sensory capacity develops with increasing age, not only in the visual and auditory, but also in the somatosensory system (Kalisch et al., 2009). Therapeutic strategies to treat the decline of perceptual abilities, which often impacts also motor function, usually comprise intensive and repeated mass training of the respective sensory modality (Sawaki et al., 2003; Kornatz et al., 2005).
A highly efficient alternative approach consists of passive repetitive sensory stimulation (RSS), which has been shown to enhance sensory abilities in chronic stroke patients (Powell et al., 1999; Conforto et al., 2002, 2007; Sawaki et al., 2006; Smith et al., 2009). RSS is a form of repetitive stimulation, following the idea of Hebbian learning: synchronous neural activity that is instrumental to drive plastic changes, is evoked by tactile “co”-activation of the skin, or electrical co-activation of the peripheral nerves of the fingers. Several studies have shown that after such type of stimulation, tactile discrimination abilities were improved and cortical representation of the respective skin area was enlarged (Godde et al., 2000; Pleger et al., 2001, 2003; Dinse et al., 2003). Evidence for the Hebbian nature of coactivation-related learning comes in addition from the fact that, when using a modified version of the coactivation protocol consisting of a single, small stimulation site instead of one large area, no changes in perception or cortical maps occur (Pleger et al., 2003; Ragert et al., 2008). This implies that spatial summation requirements indicative of cooperative processes need to be fulfilled to drive behavioral changes.
High-frequency RSS enhances sensory and motor abilities in post-stroke patients suffering from sensory loss (Smith et al., 2009), and counteracts age-related declines of perceptual discrimination abilities (Dinse et al., 2006) and sensorimotor performance (Kalisch et al., 2008, 2010). Several studies have related the effectiveness of RSS to an induction of neural plasticity in the somatosensory system (Pleger et al., 2001, 2003; Dinse et al., 2003). However, these findings focus predominantly on cortical representations of evoked sensory neuronal activity (e.g., an altered representation of the stimulated hand area in primary somatosensory cortex). Less is known about the impact of RSS on global connectivity features of the involved neuronal network during the resting state. Illuminating this relationship would be particularly helpful in order to account for the improvement of sensorimotor integration observed in chronic stroke patients (Smith et al., 2009) and elderly subjects (Kalisch et al., 2008, 2010), since changes in sensorimotor integration most likely correspond to changes of long-range interactions between sensory, motor, and association areas (Diamond et al., 2008; Aronoff et al., 2010; Mao et al., 2011).
One way to assess the functional interactions between these distant but coordinated brain areas is to analyze resting state functional connectivity, which reflects a measure of correlations between spatially distant ongoing neuronal dynamics. To investigate the impact of RSS on resting state functional connectivity was the aim of this study. We applied high-frequency RSS in healthy subjects and recorded resting state activity by means of non-invasive Electroencephalography (EEG) before and after the RSS procedure. As a measure of functional connectivity we analyzed changes in EEG imaginary coherency (ImCoh), which can be used to assess frequency-specific interactions between distinct brain areas and unlike the conventional measure of (magnitude squared) coherence has the advantage to reduce overestimation errors due to common references, volume conduction, and cross-talk (Nolte et al., 2004; Guggisberg et al., 2008). The sensorimotor system is known to exhibit dominant resting state rhythms (Salmelin and Hari, 1994b) that peak in the alpha (8–12 Hz) and beta (13–29 Hz) band, the former known as mu-rhythm or rolandic alpha rhythm (Gastaut, 1952; Kuhlman, 1978), the latter as rolandic beta rhythm (Pfurtscheller, 1981, 1992; Salmelin and Hari, 1994a). While the rolandic beta rhythm has a stronger link to the precentral motor cortex, the mu-rhythm is more tightly related to the somatosensory postcentral cortex (Salmelin et al., 1995; Ritter et al., 2009). Given the previously reported positive impact of high-frequency RSS on the sensorimotor abilities in stroke patients (Smith et al., 2009) and elderly subjects (Kalisch et al., 2008, 2010), we hypothesized that RSS might induce changes of functional connectivity within the respective sensorimotor cortical network, and therefore, focused analysis on the alpha and beta frequency bands.
Materials and Methods
Subjects and Experimental Schedule
Thirty-three healthy, right-handed subjects (three male, 26.1 ± 4.0 years) participated in the study. The study was performed in compliance with the relevant laws and institutional guidelines and approved by the ethics committee of the Charité University Medicine Berlin. In the pre-session, 15 min of resting state EEG was recorded using a 64-channel EEG system (BrainAmp, Brain Products, 0.1–250 Hz hardware bandpass filter, 59 scalp channels arranged according to the International 10–20 System, two ECG channel, and one vertical EOG channel, all referenced against FCz, impedances <5 kΩ, sampling rate 5 kHz). During the whole session subjects were sitting in a quiet and dimly lit room and were instructed to stay awake and watch a silent animal documentary on a distant computer screen, which allowed maintaining a high state of vigilance while still minimizing eye movements. During the RSS-session high-frequency somatosensory stimuli were delivered for 30 min to the right index finger (IF) of the subject (see section “High-frequency RSS” for details of the stimulation protocol). EEG was continuously recorded during RSS and was used to identify EEG somatosensory signal components (see section “EEG pre-processing”). In the post-session, 30 min after RSS terminated, another 15 min of resting state EEG was recorded, with all settings identical to the pre-session.
High-Frequency RSS
Two types of previously reported somatosensory RSS stimuli were used (electrical or vibrotactile). In 21 subjects, two disposable surface electrodes with an area of 15 × 20 mm were attached to the palmar skin of the right IF, with the positive electrode applied to the distal and the negative electrode applied to the proximal phalanxes (Smith et al., 2009; Kalisch et al., 2010). In 12 subjects, a small loudspeaker diaphragm with a diameter of ∼8 mm was mounted to the tip of the right IF and was used to transmit the tactile stimuli to the skin (Godde et al., 2000; Pleger et al., 2001; Dinse et al., 2003). Stimulation trains consisted of 20 single pulses within 1 s (i.e., a repetition rate of 20 Hz) with an inter-train interval of 5 s. Duration of the RSS protocol was 30 min, resulting in a total number of 6000 pulses. RSS stimuli were applied at 50% above perception threshold.
Psychophysical Measurements
Behavioral impact of RSS was assessed by testing tactile discrimination performance via a two-alternative forced-choice simultaneous spatial two-point-discrimination (2PD) paradigm. This data was in fact acquired in the context of another study and not used for any further analysis, but is reported here for the sake of completeness. In every subject, 2PD was performed before and after RSS, for the right stimulated and left control IF. For details of the 2PD procedure please refer to previous studies using the identical protocol (Godde et al., 2000; Pleger et al., 2001; Dinse et al., 2003).
EEG—Pre-Processing
All EEG data analysis was carried out using MATLAB v7.6.0 and the EEGLAB toolbox (Delorme and Makeig, 2004). EEG was down-sampled to 100 Hz and filtered (1–40 Hz) to remove slow drifts and line-noise and to improve the frequency specificity of subsequent post-processing techniques. EEG data were visually inspected and segments containing gross artifacts (due to movements or bad electrode impedances) were excluded. In all datasets such segments constituted less than 2% of the data, indicating an overall sufficient data quality. For each subject, EEG datasets of all three sessions (Pre, RSS, Post) were merged and submitted to an independent component analysis (ICA). ICA linearly unmixes the original EEG channel data into a sum of maximally temporally independent and spatially fixed components (Bell and Sejnowski, 1995; Makeig et al., 1996). Characteristics of the resulting independent components (ICs) were obtained by the following analyses: for pre- and post-sessions, we calculated resting state power spectra using Welch's methods, where the time series is divided into overlapping, Hamming-windowed segments, the squared magnitude of the discrete Fourier transform is computed for all segments and then averaged. Segments had 50% overlap and a window length of two seconds (i.e., twice the number of data points sampled every second), resulting in a frequency resolution of 0.5 Hz. For the RSS session, we calculated two types of activity: stimulus-locked activity, i.e., the somatosensory-evoked potentials (SEP), reflecting evoked activity, and the event-related spectral perturbation (ERSP), reflecting frequency-resolved induced oscillatory activity [please refer to (Delorme and Makeig, 2004) for a detailed description of ERSP calculation]. ICA was used for further identification of artifacts. Time-courses, spectra, and topographic distributions of all ICs were inspected to identify components that reflected eye movement, scalp muscle artifacts and movement artifacts. These ICs were removed from the data. Furthermore, in each subject an IC was identified that represents activity related to sensorimotor activity and in particular to the somatosensory processing of the high-frequency RSS stimulus (further termed somato-IC). The somato-IC was identified by four criteria: (1) a somatosensory topography of the component map, (2) an alpha (8–12 Hz) frequency peak in the component spectrum (a beta peak is usually expected, but was not obligatory), (3) a significant SEP, and (4) a significant event-related desynchronization in the alpha frequency band of the ERSP images. If more than one somato-IC was identified in one subject, the first in order (explaining the most variance) was chosen.
For each subject, the individual alpha peak frequency of the rolandic rhythm was determined (further termed Iαf) as the frequency in the power spectrum of the somato-IC showing the maximum value within the broad alpha (8–12 Hz) band (Klimesch, 1996; Klimesch et al., 2006). We defined three sub-bands with reference to Iαf: peak (Iαf – 0.5 Hz–Iαf + 0.5 Hz), lower (Iαf – 1.5 Hz–Iαf), and upper (Iαf–Iαf + 1.5 Hz) alpha frequency band. This subdivision was motivated by previous studies showing that the broad alpha band can be divided into a lower and an upper sub-band, which exhibit different functional properties (Klimesch et al., 1994, 1997, 2006; Rohm et al., 2001; Doppelmayr et al., 2002). Additionally the beta band was defined as 13–29 Hz (since a beta peak was not identifiable in every subject, no individual beta peak frequency was calculated). Our approach to identify components extracted by the ICA related to somatosensory processing had the primary goal of ensuring that the RSS was conducted correctly. However, we performed all subsequent analyses in channel space and not in component space because we did not want to bias our analysis a priori only to ongoing activity related to the somatosensory system. A considerable fraction of rhythmic brain activity relevant to our hypothesis might be hidden in other components that were not categorized as somato-ICs.
EEG—Imaginary Coherency
Functional connectivity was assessed by means of ImCoh. Introduced by Nolte et al., this connectivity estimate is known to reduce overestimation biases inherent in many other measures of EEG functional connectivity, such as absolute coherence, phase locking, or synchronization likelihood (Nolte et al., 2004; Guggisberg et al., 2008). In these measures, spurious interactions with zero time lag arise due to common references, cross-talk, and volume conduction. In contrast, ImCoh only captures “true” interactions that occur with a certain time lag, omitting all spurious zero time lag interactions. ImCoh is defined as the imaginary part of coherency Cij(f), which is a complex measure of the linear relationship between two time series and at a certain frequency f. Cij(f) is defined as the normalized cross-spectrum between the two signals (which in our case are the time series of two EEG channels i and j):
where Sij(f) ≡ 〈xi(f)x*j(f)〉 is the cross-spectrum, * is the complex conjugate, 〈 〉 is the expectation value, and xi(f), xj(f) are the complex Fourier transforms of , .
With n = 59 scalp channels available, there are possible ImCoh values, since C is antisymmetric with vanishing diagonal. ImCoh was calculated separately for each subject, recording session, and channel pair combination. The 15 min EEG time series was partitioned into overlapping (50%) segments with a window length of 2 s, which resulted in a frequency resolution of 0.5 Hz. ImCoh values for each of the four frequency bands (three alpha sub-bands and beta band) were calculated by averaging the ImCoh of the respective frequencies. For each channel pair, changes of ImCoh between pre- and post-session were tested for significance using Student's t-tests. In order to allow appropriate use of a parametric statistical test, Fisher's Z-transformation was used to normalize the distribution of ImCoh data (see Nolte et al., 2004 for details on the transformation of complex coherency). After transformation normality of the distribution was confirmed by an Anderson–Darling test.
Correction for Multiple Comparisons
The large number of tested channel pairs increases the probability that one or more null hypotheses are mistakenly rejected, also known as the family-wise error rate (FWER). Thus, a correction for multiple comparisons is needed before a statement with respect to statistical significance can be made. Due to the possible redundancy between neighboring channels, a classical Bonferroni correction is a too conservative approach. An established alternative is the false discovery rate (FDR), which is a less conservative and more powerful method for controlling the FWER (Benjamini and Hochberg, 1995).
FDR calculates the expected proportion of false positives (type I error) among all significant hypotheses H1 … Hm. Their corresponding P-values P1 … Pm are sorted in increasing order and for a given threshold α the largest P(i) such that , is identified. Then all hypotheses H1 … Hi are rejected. Here, we used the generalized Benjamini–Hochberg–Yekutieli procedure which controls the FDR under arbitrary dependence assumptions by using and thus is guaranteed to be accurate for any test dependency structure, i.e., even if the tests are positively or negatively correlated (Benjamini and Yekutieli, 2001).
Results
EEG—Identification of Sensorimotor Components
We aimed to investigate the impact of high-frequency RSS on resting state functional connectivity in the sensorimotor system. As a first step we used ICA to identify EEG components in each subject that reflect sensorimotor activity (somato-ICs), including the response to the RSS (for definition of criteria see Materials and Methods section “EEG—pre-processing”). Figure 1 shows the properties of a somato-IC in a representative subject. The distribution of the component's weights shows a clear sensorimotor topography (Figure 1A). Its resting state frequency spectrum exhibits distinct peaks in the alpha and beta frequency bands, which reflect the ongoing rolandic rhythms (Figure 1C). The component shows both an evoked and an induced response to the RSS (Figures 1B and D).
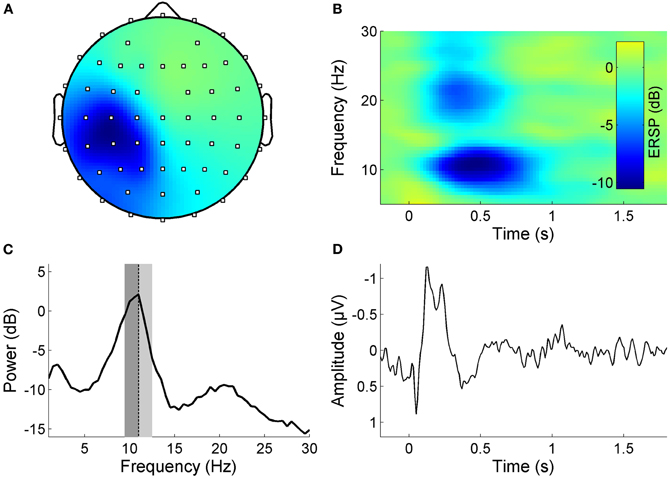
Figure 1. Properties of a somato-IC reflecting sensorimotor activity in a representative subject. (A) Scalp map projection of IC weights (dots display channel locations, color-coding as in panel B, but unitless). The projection shows a lateralized somatosensory topography with a peak contralateral to the stimulation site. (B) Time-frequency image showing ERSP, which is the relative change in event-related power at different frequencies, time-locked to the high-frequency RSS which starts at time point zero and is sustained for one second. Note the pronounced ERD in the alpha and beta frequency band during stimulation. (C) Mean log power spectrum with distinct peaks in the alpha and beta frequency band. Individual alpha frequency Iα f indicated as dotted lines, lower/upper alpha bands relative to Iαf are indicated as dark/light gray areas. (D) Corresponding time-locked average SEP.
In seven subjects we could not identify any somato-IC. Neither did they show any somatosensory features (SEP, ERD, spectral alpha peak) in channel space. In fact all subjects that showed an EEG response to the RSS in channel space yielded at least one somato-IC. There might be several reasons why these subjects did not respond to stimulation, at least in terms of a measurable EEG response. The stimulation amplitude was set to 50% above perception threshold, which was determined in an iterative manner via the subjects' feedback. It is conceivable that in some cases, the amplitude of the stimulation might have been too low to induce any EEG response (and also any perceptual learning). Another possibility is a malfunction of the stimulation device. Since we could not exclude technical failure or a bad setting of the stimulation amplitude we excluded these subjects. Importantly the exclusion was based on the absence of their EEG response, not on the basis of their behavioral results. Nevertheless, these subjects did not show any behavioral improvement (mean ± SD two-point-discrimination threshold changes of the right stimulated IF were −1.03 ± 7.27%, p < 0.8125, Wilcoxon-Signed Rank test).
The final subject group was still large enough to perform proper group analyses and statistics (26 subjects, 3 male, 26.1 ± 4.0 years). All remaining subjects exhibited a clear alpha peak in the resting state spectrum of their primary somato-IC. The group mean (±SD) peak frequency in the alpha band was 9.9 ± 1.35 Hz.
Prior to further analysis, ICs representing eye movement, scalp muscle artifacts, and movement artifacts were removed from the data. The mean ± SD number of ICs removed from data was 2.19 ± 0.89, which corresponds to 3.7 ± 1.5% of the total 59 ICs per subject. Although the total number of discarded ICs limits the maximum number of independent coherencies, the removal of these non-physiological and non-neuronal ICs increases the overall sensitivity for coherencies based on neuronal processes.
EEG—Functional Connectivity Changes
To assess the impact of RSS on resting state functional connectivity, we analyzed ImCoh before and after the RSS procedure. Figure 2 shows an overview of the frequency-resolved (1–40 Hz, resolution 0.5 Hz) statistical dispersion of ImCoh-spectra. Depicted are the standard deviations (SD) across all subjects and all channel pairs for pre- and post-sessions and for the difference spectra post-pre indicating the change between the two sessions. Before calculating the SD, spectra of all subjects were shifted so that their alpha peak frequencies coincide at 10 Hz (e.g., if a subject exhibits an alpha peak frequency of 11.5 Hz, the spectrum is shifted 1.5 Hz to the left). This visualization corresponds to the band-specific analyses, which we performed based on individual peak frequencies (Klimesch, 1996; Klimesch et al., 2006). The original alpha peak frequencies of all subjects are indicated in Figure 2A as black dots. The most pronounced change of ImCoh is clearly visible in the alpha frequency band (8–12 Hz). A first hint to the location of the alpha ImCoh change is given when looking at the SD of ImCoh spectra of channel pairs in specific regions-of-interest (ROIs). A separate display for frontal, central, and occipital channels reveals that the main effect originates from central channels, with maximal changes in the alpha and beta frequency bands (Figure 2D). This fits to the sensory modality of the RSS stimulation, since the sensorimotor system is known to exhibit oscillatory activity in these bands (Kuhlman, 1978; Pfurtscheller, 1981, 2006).
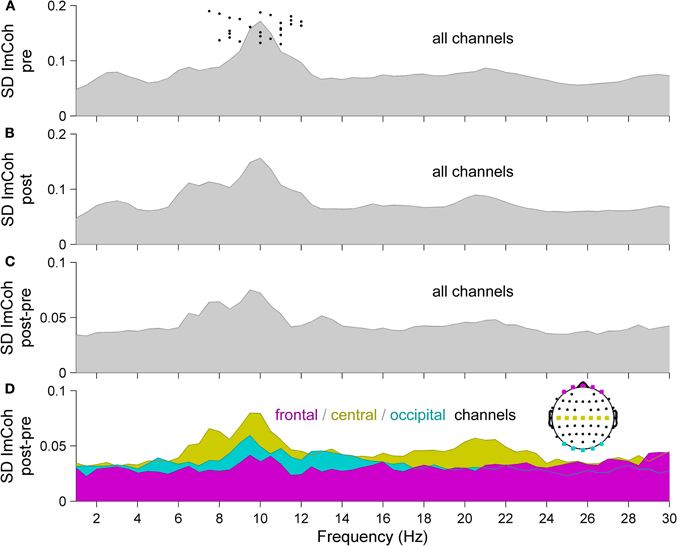
Figure 2. Statistical dispersion of ImCoh-spectra for (A) pre- and (B) post-sessions and for (C, D) difference spectra post-pre, indicating the change between the two sessions. (A–C) Standard deviations (SD) across all subjects and all scalp channels. Before calculating the SD, spectra of all subjects were shifted so that their alpha peak frequencies coincide at 10 Hz. Original alpha peak frequencies of all subjects are indicated as dots in panel A. (D) SD of ImCoh difference spectra from panel C, but separately for three sub-groups of channels, indicated in the head plot (magenta: frontal, yellow: central, cyan: occipital). Since ImCoh is antisymmectric (im(Cij(f)) = −im(Cji(f))) only the upper off-diagonal elements of the imaginary part of the coherency matrix are shown. Normality of distributions was confirmed by Anderson–Darling tests, allowing the use of SD as a measure of statistical dispersion.
Please note that Figure 2 is purely illustrative and does not allow for any statistical interpretation. Therefore next, we analyzed which of the changes of ImCoh between pre- and post-sessions were statistically significant. Given the sensory modality of the applied RSS, we focused on the ongoing oscillatory activity in the alpha (8–12 Hz) and beta (13–29 Hz) frequency bands. Also, given the known distinct functional roles of different alpha sub-bands (Klimesch and Doppelmayr, 1997; Pfurtscheller et al., 2000; Klimesch et al., 2003; Sadaghiani et al., 2010), we additionally defined three alpha sub-bands (peak, lower and upper band), which were determined in each subject according to their individual peak frequencies (see Materials and Methods section for details). Of all analyzed frequency bands, we found the maximum connectivity change in the upper alpha frequency band. Figure 3 shows the group results for this band. A pronounced change of ImCoh can be seen over central cortical areas contralateral to the somatosensory stimulation. This change is highly significant (Figures 3B and C). Figure 3D shows significant channel pairs after FDR-correction for multiple comparisons, which were: C1–C3, C1–C5, C1–CP5, CP1–CP3, CP1–CP5, CP3–C1, FC1–FT7. In the other analyzed alpha and beta frequency bands, we also found significant changes of ImCoh, but they were only marginal and after FDR-correction for multiple comparisons confined to isolated single channel pairs (lower alpha band: no significant changes, peak alpha band: C1–C3, beta band: FC3-TP7).
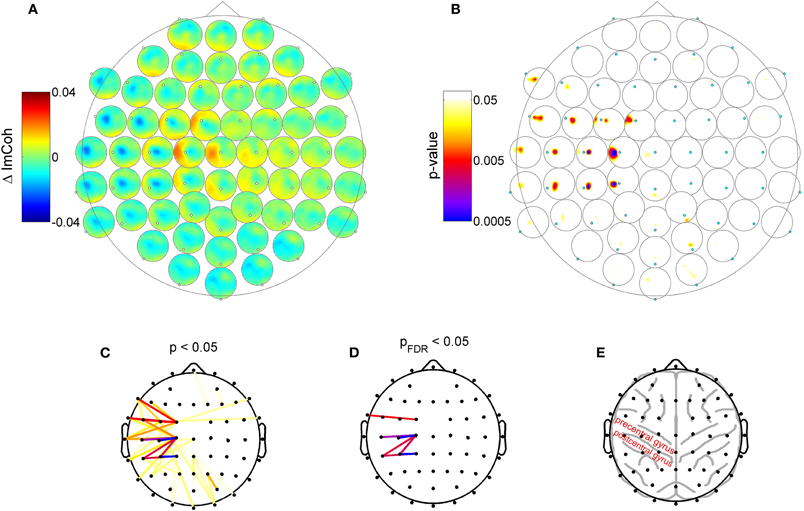
Figure 3. Change of resting state functional connectivity after high-frequency RSS. (A) ImCoh difference between pre- and post-session (before and after RSS). Dots: Channel relative to which ImCoh is shown. (B) Corresponding p-values indicating statistically significant changes of ImCoh. A cluster of significant connectivity changes is located over contralateral central areas. (C) Different visualization of data shown in panel B. Dots indicate channel locations, lines indicate channel pairs with statistically significant ImCoh changes (color coding as in panel B). (D) Same as panel C, but only significant ImCoh changes after FDR-correction for multiple comparisons. (E) Outline of typical locations of cortex gyri and sulci, indicating that the main change of ImCoh is located over pre- and post-central cortical areas.
Although our data allows only limited claims regarding the exact cortical source of the change in resting state functional connectivity, they indicate that the main change is possibly related to activity in the sensorimotor cortex. First, the peak of change is located over central electrodes contralateral to the somatosensory stimulation site, indicating that the change in functional connectivity is produced by the repetitive somatosensory stimulation. Secondly, the main change in functional connectivity is reflected in a change of ImCoh in the alpha band, which, due to the location, most certainly corresponds to mu-rhythm activity, which is the dominant resting state rhythm of the sensorimotor system. The observed connectivity changes exhibited a predominant medio-lateral orientation and are distributed over frontoparietal sensorimotor cortex (Figure 3E). According to Koessler et al. (2009), who estimated the cranio-cerebral correlations between surface EEG channel positions and the underlying cortical fissures, our main effect reflects connectivity changes between precentral gyrus and postcentral/inferior parietal gyrus (channel C1 to channels C3, C5, Cp1), and within postcentral/inferior parietal gyrus (channel CP1 to CP3, CP5).
Increased Tactile Acuity after RSS
RSS led to a significant reduction of the 2PD threshold (post-hoc difference post-pre = −0.24 mm, p < 4.67 × 10−6, paired Student's t-test) for the stimulated right IF, while threshold of the left control IF remained unchanged (difference post-pre = −0.0058 mm, p < 0.906). These results are closely in line with earlier studies using the identical stimulation protocol as used in our current study (Godde et al., 2000; Pleger et al., 2001; Dinse et al., 2003). While 2PD data was measured here to ensure stimulation efficacy and for further analysis in the context of another study, we do not believe it is an effective test for changes in functional connectivity between distant brain regions, and hence did not further analyze behavioral data in the scope of this study. We plan to follow up on the link between functional connectivity changes and behavior by testing e.g., sensorimotor integration by means of motor tasks (Smith et al., 2009).
Discussion
We investigated the impact of a specific form of intermittent high-frequency RSS on the network characteristics of the brain. In particular, we analyzed resting-state functional connectivity and found effects within sensorimotor areas which are known to be relevant for sensorimotor processing and integration (Diamond et al., 2008; Aronoff et al., 2010; Mao et al., 2011). The here-used RSS paradigm is known to evoke Hebbian-like learning processes and as a consequence causes both an improvement in tactile acuity (Godde et al., 2000) and a cortical reorganization of the somatosensory representational maps (Pleger et al., 2001, 2003; Dinse et al., 2003).
Similar improvements in tactile discrimination as well as in cortical representation can be induced by repetitive transcranial magnetic stimulation (rTMS). The approaches of RSS and of rTMS share a common conceptual idea: to adapt stimulation protocols typically used in studies of synaptic plasticity at a cellular level in order to alter perception and behavior in human individuals. Crucial to this approach is the observation that changes induced in this way are bidirectional depending on stimulation frequency. Both low frequency repetitive stimulation as well as low frequency rTMS have been shown to reduce cortical excitability in parallel to impairment of behavior. In contrast, high-frequency, intermittent stimulation enhances excitability, which is associated with improvement of performance (Siebner and Rothwell, 2003; Ragert et al., 2004; Tegenthoff et al., 2005; Reis et al., 2008; Dinse, 2011). What makes exposure-based learning different to rTMS is the fact that in contrast to rTMS, the use of sensory stimuli employs the natural sensory pathway to reach a particular cortical area, and that this allows application of highly specific stimulus features such as orientation or luminance, which has been shown to induce specific learning effects for those features (Beste et al., 2011), an option not possible for rTMS.
Please note that we referred to the effects of passive stimulation as “learning processes.” The rationale for this is based on previous studies showing that the effects of repetitive stimulation depend on NMDA-receptor activation (Dinse et al., 2003), thus demonstrating that the effects of passive stimulation are mediated by very basic mechanisms underlying synaptic plasticity, and on empirical findings showing facilitation of intracortical excitability (Hoffken et al., 2007). We therefore argue that the effects of passive stimulation are based on modifications of synaptic efficacy and transmission, both fundamental principles underlying “learning”. In a more general view, learning is defined as the acquisition of new knowledge, behaviors, skills, values, preferences or understanding, and may involve synthesizing different types of information. Apparently, the term “learning” is rather broadly defined. Given such a broad definition, in our view the outcome of persistent improvement observed following passive stimulation qualifies as learning.
High-frequency RSS was previously proposed as therapeutic approach to treat the decline and loss of sensory abilities (Kalisch et al., 2008, 2010; Smith et al., 2009). Additionally, high frequency RSS has been shown to improve sensorimotor abilities such as haptic and fine motor performance in elderly subjects (Kalisch et al., 2008, 2010). It has, therefore, successfully been applied in stroke patients to improve motor test results and to stabilize motor training effects over a longer time course (Smith et al., 2009). These data implicate that a purely passive sensory repetitive stimulation protocol has a pronounced impact on the network characteristics of the sensorimotor system, and point to the importance of sensory input and sensorimotor integration for the outcome of motor-training rehabilitation paradigms. Here, we provide neurophysiological evidence supporting these recent behavioral results. We show that high-frequency RSS not only changes sensory function and evoked activity in somatosensory areas (Pleger et al., 2001, 2003; Dinse et al., 2003), but also significantly affects resting-state functional connectivity between brain areas engaged in sensorimotor integration processes.
As a means of assessing functional connectivity, we examined coherency changes of ongoing oscillatory activity in human EEG. Although the approach of using ImCoh as a measure of functional connectivity is fairly new (Nolte et al., 2004), it has been applied in a wide variety of studies of different clinical and neuroscientific context (Guggisberg et al., 2008; Cole et al., 2010; Hinkley et al., 2010, 2011; Gonzalez et al., 2011; Martino et al., 2011; Nicolas et al., 2011; Sander et al., 2011).
Our results show that a brief application of RSS alters functional connectivity of the sensorimotor system. Connectivity changes had a mainly medio-lateral orientation, which principally is in line with earlier findings indicating a change of the center of gravity of somatosensory-evoked activity in medio-lateral direction in EEG and fMRI data (Pleger et al., 2001, 2003). In contrast to those previous results, however, the here-reported changes of functional connectivity were present during the resting state, i.e., in the absence of any explicit sensorimotor stimuli and are distributed over EEG channels which are located over pre- and post-central cortex (Koessler et al., 2009)—suggesting an involvement of not only sensory but also motor areas.
Hence, the here reported connectivity changes might be related to the findings by Smith et al. (2009) and Kalisch et al. (2008, 2010), who observed enhanced sensorimotor integration, enhanced information transfer, and enhanced learning after RSS. Furthermore, our findings might not only be related to these studies on stroke rehabilitation and physiological aging, but possibly apply to a variety of pathologies associated with sensory loss and motor function impairment.
The observed effect is based on changes in oscillatory coupling of ongoing EEG rhythms, with the main finding being in the upper alpha band (∼10–12 Hz). The alpha rhythm is one of the most prominent oscillatory hallmarks of the resting human brain. The amplitude of alpha-band oscillations exhibits temporal correlations and spatial coherency, over long-range distances (Nunez et al., 2001; Freyer et al., 2009a, 2011), which makes them eligible for the investigation of the brain's network characteristics. The most prominent alpha rhythm is related to the visual system and plays a strong functional role in the processing of visual stimuli (Makeig et al., 2002; Kirschfeld, 2005; Hanslmayr et al., 2007; Becker et al., 2008, 2011; Ritter and Becker, 2009; Scheeringa et al., 2011). However, the changes in functional connectivity in the present study relates to alpha activity that is distinct from the “classic” occipital alpha rhythm, which dominates the EEG when the subject is at rest with eyes closed. In the present case, the subjects had their eyes open, which leads to a strong attenuation of the occipital alpha rhythm. As can be seen in Figure 2D, alpha band ImCoh changes in occipital electrodes were much less pronounced than in central electrodes. In fact, the change in the alpha band is not stronger than in any other of the investigated frequencies indicating that it does not exceed noise level. Given the clear peak in central channels and the modality of the stimulus, the present finding most likely reflects the alpha band activity of the sensorimotor system, i.e., the mu-rhythm (Gastaut, 1952; Kuhlman, 1978).
Evidence for a functional role of the mu-rhythm is plenty. For example, intermediate amplitudes of the rhythm facilitate somatosensory stimulus perception in near-threshold stimulation conditions (Linkenkaer-Hansen et al., 2004). Pre-stimulus mu-rhythm amplitude predicts detection of a target stimulus against stronger masking stimuli (Schubert et al., 2009). The rhythm is also involved in higher cognitive processes, as indicated by studies showing a modulation of the mu-rhythm by attention (Jones et al., 2010; Anderson and Ding, 2011) and by the numerous application of mu-rhythm-based brain computer interfaces (Blankertz et al., 2007; Muller et al., 2008; Yuan and He, 2009; Pfurtscheller et al., 2010; Boulay et al., 2011). Combined EEG-fMRI studies have shown the mu-rhythm to be negatively correlated to the BOLD signal in primary somatosensory and motor cortex (Ritter et al., 2009), indicating less net neuronal activity, which is well in line with the recently proposed theory that alpha band rhythms serve a “gating-by-inhibition” (Klimesch et al., 2007; Jensen and Mazaheri, 2010). Finally, mu-rhythm has been shown to exhibit a functional connection to stimulus-induced brain signals in remote brain areas, thus providing evidence for long-range connectivity (Reinacher et al., 2009). But what might be the role of this rhythm in sensorimotor integration? Here, we speculate that the observed RSS-induced change in oscillatory coupling between distant sensorimotor areas reflects enhanced effectiveness of neuronal information transfer and sensorimotor integration, possibly via a modified feedback loop between motor and sensory areas, which in turn lead to the observed improvement of behavioral sensorimotor abilities. In order to provide a more detailed account of the mechanisms that link changes in oscillatory resting-state dynamics, altered connectivity, and improved somatosensory capabilities, comprehensive approaches combining advanced multimodal empirical data acquisition capable to also monitor ultrahigh frequency activity (Ritter et al., 2008; Freyer et al., 2009b), spatiotemporal nesting analysis (Schultze-Kraft et al., 2011), multivariate approaches (McIntosh et al., 1996; Boonstra et al., 2007; Krishnan et al., 2011), and computational modeling will be employed in the future.
Conclusions
Here we provide evidence for the impact of passive sensory stimulation on the resting state functional connectivity in the human brain. We show that 30 min of RSS leads to significant coherency changes of the ongoing mu-rhythm, the dominant resting state rhythm of the sensorimotor system. These changes might constitute a neurophysiological substrate for the previously observed improvements in sensorimotor training in response to RSS (Kalisch et al., 2008, 2010; Smith et al., 2009). Functional connectivity measures provide tools for assessing network interactions in the human brain, and may thus be used to study efficacy of different sensory and motor training paradigms.
Conflict of Interest Statement
The authors declare that the research was conducted in the absence of any commercial or financial relationships that could be construed as a potential conflict of interest.
Acknowledgments
The authors acknowledge the support of the German Ministry of Education and Research (Bernstein Focus State Dependencies of Learning 01GQ0971: Frank Freyer, Matthias Reinacher, Hubert R. Dinse, Petra Ritter), the James S. McDonnell Foundation (Brain Network Recovery Group JSMF22002082: Petra Ritter), the Max-Planck Society (Minerva Program: Petra Ritter).
References
Anderson, K. L., and Ding, M. (2011). Attentional modulation of the somatosensory mu rhythm. Neuroscience 180, 165–180.
Aronoff, R., Matyas, F., Mateo, C., Ciron, C., Schneider, B., and Petersen, C. C. (2010). Long-range connectivity of mouse primary somatosensory barrel cortex. Eur. J. Neurosci. 31, 2221–2233.
Becker, R., Reinacher, M., Freyer, F., Villringer, A., and Ritter, P. (2011). How ongoing neuronal oscillations account for evoked fMRI variability. J. Neurosci. 31, 11016–11027.
Becker, R., Ritter, P., and Villringer, A. (2008). Influence of ongoing alpha rhythm on the visual evoked potential. Neuroimage 39, 707–716.
Bell, A. J., and Sejnowski, T. J. (1995). An information maximization approach to blind separation and blind deconvolution. Neural Comput. 7, 1129–1159.
Benjamini, Y., and Hochberg, Y. (1995). Controlling the false discovery rate – a practical and powerful approach to multiple testing. J. R. Stat. Soc. B 57, 289–300.
Benjamini, Y., and Yekutieli, D. (2001). The control of the false discovery rate in multiple testing under dependency. Ann. Stat. 29, 1165–1188.
Beste, C., Wascher, E., Gunturkun, O., and Dinse, H. R. (2011). Improvement and impairment of visually guided behavior through LTP- and LTD-like exposure-based visual learning. Curr. Biol. 21, 876–882.
Blankertz, B., Dornhege, G., Krauledat, M., Muller, K. R., and Curio, G. (2007). The non-invasive berlin brain-computer interface: fast acquisition of effective performance in untrained subjects. Neuroimage 37, 539–550.
Boonstra, T. W., Daffertshofer, A., Breakspear, M., and Beek, P. J. (2007). Multivariate time-frequency analysis of electromagnetic brain activity during bimanual motor learning. Neuroimage 36, 370–377.
Boulay, C. B., Sarnacki, W. A., Wolpaw, J. R., and McFarland, D. J. (2011). Trained modulation of sensorimotor rhythms can affect reaction time. Clin. Neurophysiol. 122, 1820–1826.
Carey, L. M., Matyas, T. A., and Oke, L. E. (1993). Sensory loss in stroke patients – effective training of tactile and proprioceptive discrimination. Arch. Phys. Med. Rehabil. 74, 602–611.
Cole, M. W., Bagic, A., Kass, R., and Schneider, W. (2010). Prefrontal dynamics underlying rapid instructed task learning reverse with practice. J. Neurosci. 30, 14245–14254.
Conforto, A. B., Cohen, L. G., Dos Santos, R. L., Scaff, M., and Marie, S. K. (2007). Effects of somatosensory stimulation on motor function in chronic cortico-subcortical strokes. J. Neurol. 254, 333–339.
Conforto, A. B., Kaelin-Lang, A., and Cohen, L. G. (2002). Increase in hand muscle strength of stroke patients after somatosensory stimulation. Ann. Neurol. 51, 122–125.
Delorme, A., and Makeig, S. (2004). EEGLAB: an open source toolbox for analysis of single-trial EEG dynamics including independent component analysis. J. Neurosci. Methods 134, 9–21.
Diamond, M. E., Von Heimendahl, M., Knutsen, P. M., Kleinfeld, D., and Ahissar, E. (2008). ‘Where’ and ‘what’ in the whisker sensorimotor system. Nat. Rev. Neurosci. 9, 601–612.
Dinse, H. R. (2011). Choosing to improve or to impair. Clin. Neurophysiol. doi: 10.1016/j.clinph.2011.10.011. [Epub ahead of print].
Dinse, H. R., Kleibel, N., Kalisch, T., Ragert, P., Wilimzig, C., and Tegenthoff, M. (2006). Tactile coactivation resets age-related decline of human tactile discrimination. Ann. Neurol. 60, 88–94.
Dinse, H. R., Ragert, P., Pleger, B., Schwenkreis, P., and Tegenthoff, M. (2003). Pharmacological modulation of perceptual learning and associated cortical reorganization. Science 301, 91–94.
Doppelmayr, M., Klimesch, W., Stadler, W., Pollhuber, D., and Heine, C. (2002). EEG alpha power and intelligence. Intelligence 30, 289–302.
Feys, H. M., De Weerdt, W. J., Selz, B. E., Steck, G. A. C., Spichiger, R., Vereeck, L. E., Putman, K. D., and van Hoydonck, G. A. (1998). Effect of a therapeutic intervention for the hemiplegic upper limb in the acute phase after stroke – A single-blind, randomized, controlled multicenter trial. Stroke 29, 785–792.
Freyer, F., Aquino, K., Robinson, P. A., Ritter, P., and Breakspear, M. (2009a). Bistability and non-Gaussian fluctuations in spontaneous cortical activity. J. Neurosci. 29, 8512–8524.
Freyer, F., Becker, R., Anami, K., Curio, G., Villringer, A., and Ritter, P. (2009b). Ultrahigh-frequency EEG during fMRI: pushing the limits of imaging-artifact correction. Neuroimage 48, 94–108.
Freyer, F., Roberts, J. A., Becker, R., Robinson, P. A., Ritter, P., and Breakspear, M. (2011). Biophysical mechanisms of multistability in resting-state cortical rhythms. J. Neurosci. 31, 6353–6361.
Gastaut, H. (1952). Electrocorticographic study of the reactivity of rolandic rhythm. Rev. Neurol. (Paris) 87, 176–182.
Godde, B., Stauffenberg, B., Spengler, F., and Dinse, H. R. (2000). Tactile coactivation-induced changes in spatial discrimination performance. J. Neurosci. 20, 1597–1604.
Gonzalez, J. J., Manas, S., De Vera, L., Mendez, L. D., Lopez, S., Garrido, J. M., and Pereda, E. (2011). Assessment of electroencephalographic functional connectivity in term and preterm neonates. Clin. Neurophysiol. 122, 696–702.
Guggisberg, A. G., Honma, S. M., Findlay, A. M., Dalal, S. S., Kirsch, H. E., Berger, M. S., and Nagarajan, S. S. (2008). Mapping functional connectivity in patients with brain lesions. Ann. Neurol. 63, 193–203.
Hanslmayr, S., Aslan, A., Staudigl, T., Klimesch, W., Herrmann, C. S., and Bauml, K. H. (2007). Prestimulus oscillations predict visual perception performance between and within subjects. Neuroimage 37, 1465–1473.
Hinkley, L. B., Owen, J. P., Fisher, M., Findlay, A. M., Vinogradov, S., and Nagarajan, S. S. (2010). Cognitive impairments in schizophrenia as assessed through activation and connectivity measures of magnetoencephalography (MEG) data. Front. Hum. Neurosci. 3:73. doi: 10.3389/neuro.09.073.2009
Hinkley, L. B., Vinogradov, S., Guggisberg, A. G., Fisher, M., Findlay, A. M., and Nagarajan, S. S. (2011). Clinical symptoms and alpha band resting-state functional connectivity imaging in patients with schizophrenia: implications for novel approaches to treatment. Biol. Psychiatry 70, 1134–1142.
Hoffken, O., Veit, M., Knossalla, F., Lissek, S., Bliem, B., Ragert, P., Dinse, H. R., and Tegenthoff, M. (2007). Sustained increase of somatosensory cortex excitability by tactile coactivation studied by paired median nerve stimulation in humans correlates with perceptual gain. J. Physiol. 584, 463–471.
Jensen, O., and Mazaheri, A. (2010). Shaping functional architecture by oscillatory alpha activity: gating by inhibition. Front. Hum. Neurosci. 4:186. doi: 10.3389/fnhum.2010.00186
Jones, S. R., Kerr, C. E., Wan, Q., Pritchett, D. L., Hamalainen, M., and Moore, C. I. (2010). Cued spatial attention drives functionally relevant modulation of the mu rhythm in primary somatosensory cortex. J. Neurosci. 30, 13760–13765.
Kalisch, T., Ragert, P., Schwenkreis, P., Dinse, H. R., and Tegenthoff, M. (2009). Impaired tactile acuity in old age is accompanied by enlarged hand representations in somatosensory cortex. Cereb. Cortex 19, 1530–1538.
Kalisch, T., Tegenthoff, M., and Dinse, H. R. (2008). Improvement of sensorimotor functions in old age by passive sensory stimulation. Clin. Interv. Aging 3, 673–690.
Kalisch, T., Tegenthoff, M., and Dinse, H. R. (2010). Repetitive electric stimulation elicits enduring improvement of sensorimotor performance in seniors. Neural Plast. 2010, 690531.
Kirschfeld, K. (2005). The physical basis of alpha waves in the electroencephalogram and the origin of the “berger effect”. Biol. Cybern. 92, 177–185.
Klimesch, W. (1996). Memory processes, brain oscillations and EEG synchronization. Int. J. Psychophysiol. 24, 61–100.
Klimesch, W., and Doppelmayr, M. (1997). Upper alpha desynchronization, semantic memory, and task difficulty. J. Psychophysiol. 11, 373–374.
Klimesch, W., Doppelmayr, M., and Hanslmayr, S. (2006). Upper alpha ERD and absolute power: their meaning for memory performance. Prog. Brain Res. 159, 151–165.
Klimesch, W., Doppelmayr, M., Pachinger, T., and Ripper, B. (1997). Brain oscillations and human memory: EEG correlates in the upper alpha and theta band. Neurosci. Lett. 238, 9–12.
Klimesch, W., Sauseng, P., and Gerloff, C. (2003). Enhancing cognitive performance with repetitive transcranial magnetic stimulation at human individual alpha frequency. Eur. J. Neurosci. 17, 1129–1133.
Klimesch, W., Sauseng, P., and Hanslmayr, S. (2007). EEG alpha oscillations: the inhibition-timing hypothesis. Brain Res. Rev. 53, 63–88.
Klimesch, W., Schimke, H., and Schwaiger, J. (1994). Episodic and semantic memory – an analysis in the eeg theta-band and alpha-band. Electroencephalogr. Clin. Neurophysiol. 91, 428–441.
Koessler, L., Maillard, L., Benhadid, A., Vignal, J. P., Felblinger, J., Vespignani, H., and Braun, M. (2009). Automated cortical projection of EEG sensors: anatomical correlation via the international 10-10 system. Neuroimage 46, 64–72.
Kornatz, K. W., Christou, E. A., and Enoka, R. M. (2005). Practice reduces motor unit discharge variability in a hand muscle and improves manual dexterity in old adults. J. Appl. Physiol. 98, 2072–2080.
Krishnan, A., Williams, L. J., McIntosh, A. R., and Abdi, H. (2011). Partial least squares (PLS) methods for neuroimaging: a tutorial and review. Neuroimage 56, 455–475.
Kuhlman, W. N. (1978). Functional topography of the human mu rhythm. Electroencephalogr. Clin. Neurophysiol. 44, 83–93.
Linkenkaer-Hansen, K., Nikulin, V. V., Palva, S., Ilmoniemi, R. J., and Palva, J. M. (2004). Prestimulus oscillations enhance psychophysical performance in humans. J. Neurosci. 24, 10186–10190.
Litvak, V., Jha, A., Eusebio, A., Oostenveld, R., Foltynie, T., Limousin, P., Zrinzo, L., Hariz, M. I., Friston, K., and Brown, P. (2011). Resting oscillatory cortico-subthalamic connectivity in patients with parkinson's disease. Brain 134, 359–374.
Makeig, S., Jung, T. P., Bell, A. J., Ghahremani, D., and Sejnowski, T. J. (1996). Blind separation of event-related brain response components. Psychophysiology 33, S58.
Makeig, S., Westerfield, M., Jung, T. P., Enghoff, S., Townsend, J., Courchesne, E., and Sejnowski, T. J. (2002). Dynamic brain sources of visual evoked responses. Science 295, 690–694.
Mao, T. Y., Kusefoglu, D., Hooks, B. M., Huber, D., Petreanu, L., and Svoboda, K. (2011). Long-range neuronal circuits underlying the interaction between sensory and motor cortex. Neuron 72, 111–123.
Martino, J., Honma, S. M., Findlay, A. M., Guggisberg, A. G., Owen, J. P., Kirsch, H. E., Berger, M. S., and Nagarajan, S. S. (2011). Resting functional connectivity in patients with brain tumors in eloquent areas. Ann. Neurol. 69, 521–532.
McIntosh, A. R., Bookstein, F. L., Haxby, J. V., and Grady, C. L. (1996). Spatial pattern analysis of functional brain images using partial least squares. Neuroimage 3, 143–157.
Muller, K. R., Tangermann, M., Dornhege, G., Krauledat, M., Curio, G., and Blankertz, B. (2008). Machine learning for real-time single-trial EEG-analysis: from brain-computer interfacing to mental state monitoring. J. Neurosci. Methods 167, 82–90.
Nicolas, M. J., Lopez-Azcarate, J., Valencia, M., Alegre, M., Perez-Alcazar, M., Iriarte, J., and Artieda, J. (2011). Ketamine-induced oscillations in the motor circuit of the rat basal ganglia. PLoS ONE 6:e21814. doi: 10.1371/journal.pone.0021814
Nolte, G., Bai, O., Wheaton, L., Mari, Z., Vorbach, S., and Hallett, M. (2004). Identifying true brain interaction from EEG data using the imaginary part of coherency. Clin. Neurophysiol. 115, 2292–2307.
Nunez, P. L., Wingeier, B. M., and Silberstein, R. B. (2001). Spatial-temporal structures of human alpha rhythms: theory, microcurrent sources, multiscale measurements, and global binding of local networks. Hum. Brain Mapp. 13, 125–164.
Pfurtscheller, G. (1981). Central beta rhythm during sensorimotor activities in man. Electroencephalogr. Clin. Neurophysiol. 51, 253–264.
Pfurtscheller, G. (1992). Event-related synchronization (ERS): an electrophysiological correlate of cortical areas at rest. Electroencephalogr. Clin. Neurophysiol. 83, 62–69.
Pfurtscheller, G., Allison, B. Z., Brunner, C., Bauernfeind, G., Solis-Escalante, T., Scherer, R., Zander, T. O., Mueller-Putz, G., Neuper, C., and Birbaumer, N. (2010). The hybrid BCI. Front. Neurosci. 4:30. doi: 10.3389/fnpro.2010.00003
Pfurtscheller, G., Neuper, C., and Krausz, G. (2000). Functional dissociation of lower and upper frequency mu rhythms in relation to voluntary limb movement. Clin. Neurophysiol. 111, 1873–1879.
Pleger, B., Dinse, H. R., Ragert, P., Schwenkreis, P., Malin, J. P., and Tegenthoff, M. (2001). Shifts in cortical representations predict human discrimination improvement. Proc. Natl. Acad. Sci. U.S.A. 98, 12255–12260.
Pleger, B., Foerster, A. F., Ragert, P., Dinse, H. R., Schwenkreis, P., Malin, J. P., Nicolas, V., and Tegenthoff, M. (2003). Functional imaging of perceptual learning in human primary and secondary somatosensory cortex. Neuron 40, 643–653.
Powell, J., Pandyan, A. D., Granat, M., Cameron, M., and Stott, D. J. (1999). Electrical stimulation of wrist extensors in poststroke hemiplegia. Stroke 30, 1384–1389.
Ragert, P., Becker, M., Tegenthoff, M., Pleger, B., and Dinse, H. R. (2004). Sustained increase of somatosensory cortex excitability by 5 Hz repetitive transcranial magnetic stimulation studied by paired median nerve stimulation in humans. Neurosci. Lett. 356, 91–94.
Ragert, P., Kalisch, T., Bliem, B., Franzkowiak, S., and Dinse, H. R. (2008). Differential effects of tactile high- and low-frequency stimulation on tactile discrimination in human subjects. BMC Neurosci. 9,9.
Reinacher, M., Becker, R., Villringer, A., and Ritter, P. (2009). Oscillatory brain states interact with late cognitive components of the somatosensory evoked potential. J. Neurosci. Methods 183, 49–56.
Reis, J., Swayne, O. B., Vandermeeren, Y., Camus, M., Dimyan, M. A., Harris-Love, M., Perez, M. A., Ragert, P., Rothwell, J. C., and Cohen, L. G. (2008). Contribution of transcranial magnetic stimulation to the understanding of cortical mechanisms involved in motor control. J. Physiol. 586, 325–351.
Ritter, P., and Becker, R. (2009). Detecting alpha rhythm phase reset by phase sorting: caveats to consider. Neuroimage 47, 1–4.
Ritter, P., Freyer, F., Curio, G., and Villringer, A. (2008). High-frequency (600 Hz) population spikes in human EEG delineate thalamic and cortical fMRI activation sites. Neuroimage 42, 483–490.
Ritter, P., Moosmann, M., and Villringer, A. (2009). Rolandic alpha and beta EEG rhythms' strengths are inversely related to fMRI-BOLD signal in primary somatosensory and motor cortex. Hum. Brain Mapp. 30, 1168–1187.
Rohm, D., Klimesch, W., Haider, H., and Doppelmayr, M. (2001). The role of theta and alpha oscillations for language comprehension in the human electroencephalogram. Neurosci. Lett. 310, 137–140.
Rosamond, W., Flegal, K., Friday, G., Furie, K., Go, A., Greenlund, K., Haase, N., Ho, M., Howard, V., Kissela, B., Kittner, S., Lloyd-Jones, D., McDermott, M., Meigs, J., Moy, C., Nichol, G., O'Donnell, C. J., Roger, V., Rumsfeld, J., Sorlie, P., Steinberger, J., Thom, T., Wasserthiel-Smoller, S., Hong, Y. L., and Assoc, A. H. (2007). Heart disease and stroke statistics – 2007 update – a report from the american heart association statistics committee and stroke statistics subcommittee. Circulation 115, E69–E171.
Sadaghiani, S., Scheeringa, R., Lehongre, K., Morillon, B., Giraud, A. L., and Kleinschmidt, A. (2010). Intrinsic connectivity networks, alpha oscillations, and tonic alertness: a simultaneous electroencephalography/functional magne-tic resonance imaging study. J. Neurosci. 30, 10243–10250.
Salmelin, R., Hamalainen, M., Kajola, M., and Hari, R. (1995). Functional segregation of movement-related rhythmic activity in the human brain. Neuroimage 2, 237–243.
Salmelin, R., and Hari, R. (1994a). Characterization of spontaneous MEG rhythms in healthy adults. Electroencephalogr. Clin. Neurophysiol. 91, 237–248.
Salmelin, R., and Hari, R. (1994b). Spatiotemporal characteristics of sensorimotor neuromagnetic rhythms related to thumb movement. Neuroscience 60, 537–550.
Sander, T. H., Leistner, S., Geisler, F., Mackert, B. M., and Trahms, L. (2011). Characterization of motor and somatosensory function for stroke patients. Physiol. Meas. 32, 1737–1746.
Sathian, K., Zangaladze, A., Green, J., Vitek, J. L., and Delong, M. R. (1997). Tactile spatial acuity and roughness discrimination: impairments due to aging and parkinson's disease. Neurology 49, 168–177.
Sawaki, L., Wu, C. W., Kaelin-Lang, A., and Cohen, L. G. (2006). Effects of somatosensory stimulation on use-dependent plasticity in chronic stroke. Stroke 37, 246–247.
Sawaki, L., Yaseen, Z., Kopylev, L., and Cohen, L. G. (2003). Age-dependent changes in the ability to encode a novel elementary motor memory. Ann. Neurol. 53, 521–524.
Scheeringa, R., Mazaheri, A., Bojak, I., Norris, D. G., and Kleinschmidt, A. (2011). Modulation of visually evoked cortical fMRI responses by phase of ongoing occipital alpha oscillations. J. Neurosci. 31, 3813–3820.
Schubert, R., Haufe, S., Blankenburg, F., Villringer, A., and Curio, G. (2009). Now you'll feel it, now you won't: EEG rhythms predict the effectiveness of perceptual masking. J. Cogn. Neurosci. 21, 2407–2419.
Schultze-Kraft, M., Becker, R., Breakspear, M., and Ritter, P. (2011). Exploiting the potential of three dimensional spatial wavelet analysis to explore nesting of temporal oscillations and spatial variance in simultaneous EEG-fMRI data. Prog. Biophys. Mol. Biol. 105, 67–79.
Siebner, H. R., and Rothwell, J. (2003). Transcranial magnetic stimulation: new insights into representational cortical plasticity. Exp. Brain Res. 148, 1–16.
Silberstein, P., Pogosyan, A., Kuhn, A. A., Hotton, G., Tisch, S., Kupsch, A., Dowsey-Limousin, P., Hariz, M. I., and Brown, P. (2005). Cortico-cortical coupling in parkinson's disease and its modulation by therapy. Brain 128, 1277–1291.
Smith, P. S., Dinse, H. R., Kalisch, T., Johnson, M., and Walker-Batson, D. (2009). Effects of repetitive electrical stimulation to treat sensory loss in persons poststroke. Arch. Phys. Med. Rehabil. 90, 2108–2111.
Stamelou, M., Edwards, M. J., Hallett, M., and Bhatia, K. P. (2011). The non-motor syndrome of primary dystonia: clinical and pathophysiological implications. Brain doi: 10.1093/brain/awr224. [Epub ahead of print].
Tamura, Y., Ueki, Y., Lin, P., Vorbach, S., Mima, T., Kakigi, R., and Hallett, M. (2009). Disordered plasticity in the primary somatosensory cortex in focal hand dystonia. Brain 132, 749–755.
Tegenthoff, M., Ragert, P., Pleger, B., Schwenkreis, P., Forster, A. F., Nicolas, V., and Dinse, H. R. (2005). Improvement of tactile discrimination performance and enlargement of cortical somatosensory maps after 5 Hz rTMS. PLoS Biol. 3:e362. doi: 10.1371/journal.pbio.0030362
Tinazzi, M., Rosso, T., and Fiaschi, A. (2003). Role of the somatosensory system in primary dystonia. Mov. Disord. 18, 605–622.
Keywords: EEG, resting state, functional connectivity, sensory stimulation, plasticity, sensorimotor, mu-rhythm, ongoing activity
Citation: Freyer F, Reinacher M, Nolte G, Dinse HR and Ritter P (2012) Repetitive tactile stimulation changes resting-state functional connectivity—implications for treatment of sensorimotor decline. Front. Hum. Neurosci. 6:144. doi: 10.3389/fnhum.2012.00144
Received: 15 December 2011; Accepted: 08 May 2012;
Published online: 23 May 2012.
Edited by:
Burkhard Pleger, Max Planck Institute for Human Cognitive and Brain Sciences, GermanyReviewed by:
Sarang S. Dalal, INSERM, FranceAndreas Daffertshofer, VU University Amsterdam, Netherlands
Copyright: © 2012 Freyer, Reinacher, Nolte, Dinse and Ritter. This is an open-access article distributed under the terms of the Creative Commons Attribution Non Commercial License, which permits non-commercial use, distribution, and reproduction in other forums, provided the original authors and source are credited.
*Correspondence: Petra Ritter, Department of Neurology, Charité Universitaetsmedizin Berlin, Charitéplatz 1, 10117 Berlin, Germany. e-mail: petra.ritter@charite.de