- 1Health Management Center, Xiangya Hospital, Central South University, Changsha, China
- 2Department of Radiology, Xiangya Hospital, Central South University, Changsha, China
- 3School of Humanities and Social Sciences, National University of Defense Technology, Changsha, China
- 4Department of Oral and Maxillofacial Surgery, Xiangya Hospital, Central South University, Changsha, China
Betel quid (BQ) is one of the most commonly consumed psychoactive substances. It has been suggested to be associated with various health issues, especially oral cancer. Evidence also points to possible decreased cognitive functions after long-term BQ chewing, such as attention and inhibition control. The present study aims to investigate the brain structure basis of BQ chewing in Hunan province of China. Twenty-five BQ chewers and 25 controls were recruited to participate in this study. Voxel-based morphormetry analysis revealed that there were three key regions showing structural differences between BQ chewers and controls, including bilateral dorsolateral prefrontal cortex (DLPFC)/insula, ventral medial prefrontal cortex, and left orbitofrontal cortex. Moreover, the GMV in the DLPFC could potentially predict BQ dependence scores, level of daily BQ chewing, and history of BQ chewing. These results suggested that participants who showed BQ chewing dependence may have deficit in inhibition control and affective decision-making, and the level of deficit was dependent on the level of daily BQ chewing, and history of BQ chewing. Understanding the neurobiology features of BQ chewing would help us develop novel ways to diagnose and prevent BQ dependence.
Introduction
Betel quid (BQ, also called betel nut or areca nut) is one of most commonly consumed psychoactive substances (1). Consequently, the International Agency for Research on Cancer (2) has categorized BQ as a Group 1 carcinogen. Long-term BQ chewing was associated with various health issues, especially oral cancer and precancerous conditions (3–9). Studies also suggested that there were decreased cognitive functions after long-term BQ chewing, such as attention and inhibition control (10). Recently, neuroimaging studies compared frequent BQ chewers and controls and found that frequent chewers have altered brain structure (11, 12) and resting-state functional connectivity (13–17). Specifically, BQ chewers have decreased functional connectivity in the DMN including ventral medial prefrontal cortex (VMPFC), and orbitofrontal cortex (OFC)/anterior cingulate cortex (ACC) (14).
However, there is little evidence showing there are structural difference between BQ chewers and controls. One pioneer studies examined 33 BQ chewers and 32 controls and found that BQ chewers have less gray-matter volumes (GMV) in the midbrain, right ACC, bilateral dorsolateral prefrontal cortex (DLPFC) and right superior temporal gyrus, and more GMV in the right hippocampal and right precuneus (11). They also found that the GMV in the left DLPFC and right ACC was correlated with the history of BQ chewing (11). Consequently, researchers suggested that BQ chewing should be treated as substance dependence and they can meet the DSM criteria of substance dependence (14, 15). Volkow and colleagues (18–20) argued that addiction is a brain disease, and different addictions showed similar brain alterations, especially for drug-non-dependent frontal regions. Thus, we could also learn from the structural alterations of other addictive behaviors. For example, Ersche and colleagues (21) found that cocaine-dependent individuals had decreased GMV in orbitofrontal, cingulate, insular, temporoparietal, and cerebellar cortex, increased GMV in the basal ganglia. Similarly, Romero and colleagues (22) found that cocaine-dependent subjects presented higher fractional anisotropy values in the anterior cingulate and lower fractional anisotropy values in the anterior-posterior commissure plane.
The present study aimed to investigate the brain structure basis of BQ chewing in Hunan province of China. In this area, people chew dried areca nut while people in other parts of China (especially Hainan and Taiwan) chew fresh areca nuts (11). We also tried to explore if the GMV in certain brain regions could potentially predict the history of BQ chewing. Understanding the neurobiology feature of BQ chewing would help us develop novel ways to diagnose and prevent BQ dependence.
Materials and Methods
Participants
Fifty participants in Hunan area were recruited to participate in this study. All research protocols were explained to the participants and approved by the local IRB (Xiangya Hospital of Central South University of Hunan Province, Changsha, China). All participants signed the written consent form before any examinations. BQ chewers (N = 25) were recruited from the outpatient department in Xiangya Hospital of Central South University in Changsha, Hunan, China. As reported before (14), these participants meet the DSM-IV criteria for substance use disorders as determined by the Structured Clinical Interview. Controls (N = 25) with matched age and education level were recruited from the same area. Participants were excluded if they (i) met criteria for other substance dependence; (ii) have a medical history of any neurological or psychiatric disorder; and (iii) claustrophobia or other disease prevent them from MRI scanning, e.g., any metal implants. All participants have normal or corrected to normal vision. The demographic and clinical characteristics of the participants can be found in Table 1.
Procedures
Participants were asked to come to the Xiangya Hospital to finish the behavior interview and MRI scan. They were asked to read and sign the consent form first and then complete the behavioral interview. The MRI scan took about 20 min to finish. During the scan, images for one high-resolution structural scan were acquired.
Behavior Interviews
The severity of BQ dependence was assessed by the Betel Quid Dependence Scale (BQDS), which follows DSM-IV criteria (23). It is a 16-item self-report instrument with three factors: physical and psychological urge, increasing dosage, and maladaptive use.
MRI Protocol
All MRI images were acquired using a Siemens Skyra 3T scanner at Xiangya Hospital. Participants lay in the supine position on the scanner bed. They were instructed to have a rest but keep their head very still during the structural scan. The scan was performed using T1-weighted 3D-Magnetization Prepared Rapid Gradient Echo sequence, covering the whole brain with the following scanning parameters: TR/TE = 1900/2.01 ms, flip angel = 9°, matrix = 256 × 256, number of slices = 176, FOV = 256 mm × 256 mm, and slice thickness = 1 mm.
VBM Analysis
We used FSL-VBM implemented in FSL (24) to analyze the structural MRI data (25–27). Brains were extracted using BET (28) and segmented into gray matter, white matter and CSF using FAST4 (29). Two steps of registration (linear and non-linear) were performed to register the gray-matter partial volume images to the standard space (MNI152). A study specific template was created by averaging all normalized images. Last, the resulting GMV images were smoothed with an isotropic Gaussian kernel (3 mm). Statistics were performed with FSL non-non-parametric permutation methods (Randomise v2.1) (30). Voxel-wise GLM models were used to test the group difference and correlation between GMV data and BQ chewing. The null distribution at each voxel was constructed using 10,000 random permutations. Multiple comparisons were corrected across the whole brain using the threshold-free cluster enhancement. The mean GMV in each cluster was then extracted for each individual following region of interests analysis standard.
Results
Demographics and Clinical Characteristics
The demographic and clinical characteristics for BQ chewers and controls are given in Table 1. The BQ chewers exhibited a mean of 10.87 ± 1.71 BQDS score, a mean duration of BQ chewing of 12.96 ± 5.05 years, and average daily dosage of BQ of 48.48 ± 17.54 g. The two groups did not differ in terms of age (t(48) = 1.06, p = 0.29), but the control group was educated for longer time (t(48) = −2.47, p = 0.017). In later analysis, years of education was used as a co-variate in the model.
Statistical Comparison of VBM Analysis
VBM results showed that, after controlling for education, several brain regions had reduced GMV in BQ chewers compared to controls, including bilateral VMPFC, bilateral DLPFC extending to IFG and insula, and left OFC (Table 2; Figure 1). No brain regions showed higher GMV for BQ chewers than controls.
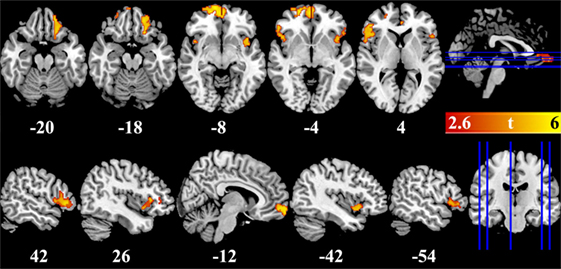
Figure 1. Brain regions showed gray-matter volume difference between betel quid chewers and controls. Figures are displayed in canonical (upper panel) and sagittal (lower panel) view. Numbers below each brain slices were the corresponding z (upper panel) or x (lower panel) value in the MNI space. Color bar represented the significance of the difference (t values).
Region of interest analysis showed that, in the BQ chewers group, the GMV in left and right DLPFC/insula could predict the BQDS score [left: r(25) = 0.536, p = 0.006, right: r(25) = 0.587, p = 0.002], years of BQ chewing [left: r(25) = 0.492, p = 0.01, right: r(25) = 0.615, p = 0.001], and daily BQ use [left: r(25) = 0.394, p = 0.05, right: r(25) = 0.413, p = 0.04]. It should be noted that, the correlations between DLPFC and daily BQ use were not significant after Bonferroni correction for multiple comparison.
Discussion
The present study recruited 25 BQ chewers and 25 controls to examine the altered brain structures associated with BQ chewing. Results suggested that there are three key regions presenting structural difference between BQ chewers and controls, including the DLPFC/IFG/insula, VMPFC, and OFC. Especially, the GMV in the DLPFC could potentially predict the BQ dependence score, level of daily BQ chewing, and years of chewing BQ.
These results emphasize that BQ chewers showed similar brain structural alternations as observed in other addictive behaviors. The gray-matter morphology deficit in these regions have been shown to be related to different types of substance and/or behavioral addiction, including cocaine (31–37), heroin (38–41), opiates (42), cannabis (43), nicotine (44, 45), alcohol (46, 47), ketamine (48), MDMA (49), methamphetamine (50), internet (51), and online games (52, 53). For example, Tanabe et al. (54) investigated participants who showed dependence on two or more substances, and results suggested lower GMV in bilateral medial OFC in patients and the neural marker was correlated with decision-making performance in a modified gambling task. It should be noted that, our results were also consistent with a previous reports who suggested similar brain systems’ GMV alternation in BQ chewers (11), especially the DLPFC. Moreover, we also suggested that the GMV reduction in DLPFC was correlated with history of BQ chewing as well as BQ dependence score, which is also consistent with previous reports (11).
These results also implied deficits of two neural systems in BQ chewing, namely the inhibition control system (DLPFC/insula) and affective decision-making system (VMPFC and OFC) (55–59). This idea is also consistent with other types of drug addiction showing that these two systems were altered. For example, drug addiction literature suggested that DLPFC activity reduction in heavy smokers, cocaine users, etc. (20, 60–62). These two systems typically showed hypo-activity in drug addicts, suggesting their impaired ability to resist stimuli that are rewarding in the short term, but lead to negative consequences in the long term (55–58). These two systems, which depend primarily on the functions of the prefrontal cortex, are necessary to control the basic impulses and allow more flexible pursuit of long-term goals (55–58).
Several studies suggest that the cognitive or regulatory control of tempting choices is in part dependent on brain regions that we have hypothesized to be components of the so-called “reflective system” (55–58, 63–67). For example, one study shows that word-level cognitive labels can change the subjective ratings of the affective value of the taste and flavor of a food when the taste or flavor stimulus is identical; this cognitive modulation is expressed in the OFC and ACC (68). This is consistent with the idea that BQ chewers have higher GMV in these two systems, as demonstrated in a previous study (11). In fMRI studies of food consumption, obese men and women had less activation in the left DLPFC in response to a meal than did their lean counterparts (69). While many researchers still clump all mechanisms of decision-making and inhibitory control under one umbrella, the rubric of “Executive Functions,” Bechara et al. (55, 70) have argued that the two are separable neuropsychological mechanisms. More specifically, there is a distinction in functionality between (1) simple inhibitory and impulse control processes mediated by the lateral orbitofrontal and inferior frontal gyrus regions and (2) affective decision-making mediated by VMPFC and OFC, including the frontal pole, which are highly relevant to behavioral control ability and to the decisions individuals make frequently on a daily basis (55). Both inhibitory/impulse control function and affective decision-making are important, specific aspects of higher order executive control functioning (55, 71). Good inhibitory functioning reflects the ability to actively stop a pre-potent behavioral response after it has been triggered. Individuals with deficits or failures in these systems have a tendency to act more impulsively. Adequate affective decision-making reflects an integration of cognitive and affective systems (hence, considered “hot”—emotionally linked—cognition, and the ability to more optimally weigh short-term gains against long-term losses or probable outcomes of an action). Excessive BQ chewing has been known to have short-term “reinforcing effects” but long-term negative consequences should be less likely or problematic for individuals with higher affective decision-making ability.
There are three noteworthy limitations of this study. First, the sample size is relatively small and the participants are all males. Second, the two groups showed significant difference in years of education which could contribute to the GMV difference even though we tried to minimize this effect by adding education as a co-variate. Third, this is a cross-sectional and correlational study, which limits our inference on the causality. Further longitudinal study should be done to replicate and extend the conclusion from this study.
Ethics Statement
All research protocols were explained to the participants and approved by the local IRB (Xiangya Hospital of Central South University of Hunan Province, Changsha, China.). All participants signed the written consent form before any examinations.
Author Contributions
FY and WL conceived and designed the experiments. XZ, CJ, and CF conducted the experiments and collected data. XZ and LK analyzed the results. FY, CJ, and CF wrote the main manuscript text. All authors reviewed the manuscript.
Conflict of Interest Statement
The authors declare that the research was conducted in the absence of any commercial or financial relationships that could be construed as a potential conflict of interest.
Funding
This work was supported by research grants from the National Natural Science Foundation of China (grant number 81301211 to XZ), Foundation for the Author of National Excellent Doctoral Dissertation of PR China (grant number 201411 to XZ), Development and Reform Commission of Hunan Province (grant number 2016-No.65 to FY), and Introduction of Overseas Expertise and Intellectual Resources of Hunan Province (grant number 2016 to FY).
References
1. Boucher BJ, Mannan N. Metabolic effects of the consumption of Areca catechu. Addict Biol (2002) 7(1):103–10. doi:10.1080/13556210120091464
2. IARC Working Group on the Evaluation of Carcinogenic Risks to Humans. Betel-quid and areca-nut chewing and some areca-nut derived nitrosamines. IARC Monogr Eval Carcinog Risks Hum (2004) 85:1–334.
3. Chu NS. Neurological aspects of areca and betel chewing. Addict Biol (2002) 7(1):111–4. doi:10.1080/13556210120091473
4. Chen YJ, Chang JTC, Liao CT, Wang HM, Yen TC, Chiu CC, et al. Head and neck cancer in the betel quid chewing area: recent advances in molecular carcinogenesis. Cancer Sci (2008) 99(8):1507–14. doi:10.1111/j.1349-7006.2008.00863.x
5. Lee C, Ko Y, Huang H, Chao Y, Tsai C, Shieh T, et al. The precancer risk of betel quid chewing, tobacco use and alcohol consumption in oral leukoplakia and oral submucous fibrosis in southern Taiwan. Br J Cancer (2003) 88(3):366–72. doi:10.1038/sj.bjc.6600727
6. Zain RB, Ikeda N, Gupta PC, Warnakulasuriya S, Wyk CW, Shrestha P, et al. Oral mucosal lesions associated with betel quid, areca nut and tobacco chewing habits: consensus from a workshop held in Kuala Lumpur, Malaysia, November 25-27, 1996. J Oral Pathol Med (1999) 28(1):1–4. doi:10.1111/j.1600-0714.1999.tb01985.x
8. Ko YC, Chiang TA, Chang SJ, Hsieh SF. Prevalence of betel quid chewing habit in Taiwan and related sociodemographic factors. J Oral Pathol Med (1992) 21(6):261–4. doi:10.1111/j.1600-0714.1992.tb01007.x
9. Ko YC, Huang YL, Lee CH, Chen MJ, Lin LM, Tsai CC. Betel quid chewing, cigarette smoking and alcohol consumption related to oral cancer in Taiwan. J Oral Pathol Med (1995) 24(10):450–3. doi:10.1111/j.1600-0714.1995.tb01132.x
10. Ho MC, Li RH, Tang TC. Betel nut chewing effects on sustained attention and inhibitory control after sleep deprivation. Aust J Psychol (2015) 67(4):222–30. doi:10.1111/ajpy.12081
11. Chen F, Zhong Y, Zhang Z, Xu Q, Liu T, Pan M, et al. Gray matter abnormalities associated with betel quid dependence: a voxel-based morphometry study. Am J Transl Res (2015) 7(2):364–74.
12. Weng J-C, Kao T-W, Huang G-J, Tyan Y-S, Tseng H-C, Ho M-C. Evaluation of structural connectivity changes in betel-quid chewers using generalized q-sampling MRI. Psychopharmacology (Berl) (2017) 234(13):1945–55. doi:10.1007/s00213-017-4602-0
13. Liu T, Li J, Zhang Z, Xu Q, Lu G, Huang S, et al. Altered long-and short-range functional connectivity in patients with betel quid dependence: a resting-state functional MRI study. Cell Physiol Biochem (2016) 40(6):1626–36. doi:10.1159/000453212
14. Zhu X, Zhu Q, Jiang C, Shen H, Wang F, Liao W, et al. Disrupted resting-state default mode network in betel quid-dependent individuals. Front Psychol (2017) 8:84. doi:10.3389/fpsyg.2017.00084
15. Liu T, Li J, Zhao Z, Zhong Y, Zhang Z, Xu Q, et al. Betel quid dependence is associated with functional connectivity changes of the anterior cingulate cortex: a resting-state fMRI study. J Transl Med (2016) 14(1):33. doi:10.1186/s12967-016-0784-1
16. Huang X, Liu Z, Mwansisya TE, Pu W, Zhou L, Liu C, et al. Betel quid chewing alters functional connectivity in frontal and default networks: a resting-state fMRI study. J Magn Reson Imaging (2017) 45(1):157–66. doi:10.1002/jmri.25322
17. Liu T, Li J-J, Zhao Z-Y, Yang G-S, Pan M-J, Li C-Q, et al. Altered spontaneous brain activity in betel quid dependence: a resting-state functional magnetic resonance imaging study. Medicine (2016) 95(5):e2638. doi:10.1097/MD.0000000000002638
18. Volkow ND, Fowler JS. Addiction, a disease of compulsion and drive: involvement of the orbitofrontal cortex. Cereb Cortex (2000) 10(3):318–25. doi:10.1093/cercor/10.3.318
19. Volkow ND, Baler RD. NOW vs LATER brain circuits: implications for obesity and addiction. Trends Neurosci (2015) 38(6):345–52. doi:10.1016/j.tins.2015.04.002
20. Goldstein RZ, Volkow ND. Dysfunction of the prefrontal cortex in addiction: neuroimaging findings and clinical implications. Nat Rev Neurosci (2011) 12(11):652–69. doi:10.1038/nrn3119
21. Ersche KD, Barnes A, Jones PS, Morein-Zamir S, Robbins TW, Bullmore ET. Abnormal structure of frontostriatal brain systems is associated with aspects of impulsivity and compulsivity in cocaine dependence. Brain (2011) 134(7):2013–24. doi:10.1093/brain/awr138
22. Romero MJ, Asensio S, Palau C, Sanchez A, Romero FJ. Cocaine addiction: diffusion tensor imaging study of the inferior frontal and anterior cingulate white matter. Psychiatry Res (2010) 181(1):57–63. doi:10.1016/j.pscychresns.2009.07.004
23. Lee C-Y, Chang C-S, Shieh T-Y, Chang Y-Y. Development and validation of a self-rating scale for betel quid chewers based on a male-prisoner population in Taiwan: the Betel Quid Dependence Scale. Drug Alcohol Depend (2012) 121(1):18–22. doi:10.1016/j.drugalcdep.2011.07.027
24. Smith S, Jenkinson M, Woolrich M, Beckmann C, Behrens T, Johansen-Berg H, et al. Advances in functional and structural MR image analysis and implementation as FSL. Neuroimage (2004) 23:S208–19. doi:10.1016/j.neuroimage.2004.07.051
25. Ashburner J, Friston K. Voxel-based morphometry – the methods. Neuroimage (2000) 11(6):805–21. doi:10.1006/nimg.2000.0582
26. Good C, Johnsrude I, Ashburner J, Henson R, Friston K, Frackowiak R. A voxel-based morphometric study of ageing in 465 normal adult human brains. Neuroimage (2001) 14(1):21–36. doi:10.1006/nimg.2001.0857
27. He Q, Xue G, Chen C, Chen C, Lu Z-L, Dong Q. Decoding the neuroanatomical basis of reading ability: a multivoxel morphometric study. J Neurosci (2013) 33(31):12835–43. doi:10.1523/JNEUROSCI.0449-13.2013
28. Smith S. Fast robust automated brain extraction. Hum Brain Mapp (2002) 17(3):143–55. doi:10.1002/hbm.10062
29. Zhang Y, Brady M, Smith S. Segmentation of brain MR images through a hidden Markov random field model and the expectation-maximization algorithm. IEEE Trans Med Imaging (2001) 20(1):45–57. doi:10.1109/42.906424
30. Nichols T, Holmes A. Nonparametric permutation tests for functional neuroimaging: a primer with examples. Hum Brain Mapp (2002) 15(1):1–25. doi:10.1002/hbm.1058
31. Franklin TR, Acton PD, Maldjian JA, Gray JD, Croft JR, Dackis CA, et al. Decreased gray matter concentration in the insular, orbitofrontal, cingulate, and temporal cortices of cocaine patients. Biol Psychiatry (2002) 51(2):134–42. doi:10.1016/S0006-3223(01)01269-0
32. Moreno-López L, Catena A, Fernández-Serrano MJ, Delgado-Rico E, Stamatakis EA, Pérez-García M, et al. Trait impulsivity and prefrontal gray matter reductions in cocaine dependent individuals. Drug Alcohol Depend (2012) 125(3):208–14. doi:10.1016/j.drugalcdep.2012.02.012
33. Sim ME, Lyoo IK, Streeter CC, Covell J, Sarid-Segal O, Ciraulo DA, et al. Cerebellar gray matter volume correlates with duration of cocaine use in cocaine-dependent subjects. Neuropsychopharmacology (2007) 32(10):2229–37. doi:10.1038/sj.npp.1301346
34. Konova AB, Moeller SJ, Tomasi D, Parvaz MA, Alia-Klein N, Volkow ND, et al. Structural and behavioral correlates of abnormal encoding of money value in the sensorimotor striatum in cocaine addiction. Eur J Neurosci (2012) 36(7):2979–88. doi:10.1111/j.1460-9568.2012.08211.x
35. Kaag AM, Crunelle CL, van Wingen G, Homberg J, van den Brink W, Reneman L. Relationship between trait impulsivity and cortical volume, thickness and surface area in male cocaine users and non-drug using controls. Drug Alcohol Depend (2014) 144:210–7. doi:10.1016/j.drugalcdep.2014.09.016
36. Barrós-Loscertales A, Garavan H, Bustamante JC, Ventura-Campos N, Llopis JJ, Belloch V, et al. Reduced striatal volume in cocaine-dependent patients. Neuroimage (2011) 56(3):1021–6. doi:10.1016/j.neuroimage.2011.02.035
37. Ansell EB, Rando K, Tuit K, Guarnaccia J, Sinha R. Cumulative adversity and smaller gray matter volume in medial prefrontal, anterior cingulate, and insula regions. Biol Psychiatry (2012) 72(1):57–64. doi:10.1016/j.biopsych.2011.11.022
38. Yuan Y, Zhu Z, Shi J, Zou Z, Yuan F, Liu Y, et al. Gray matter density negatively correlates with duration of heroin use in young lifetime heroin-dependent individuals. Brain Cogn (2009) 71(3):223–8. doi:10.1016/j.bandc.2009.08.014
39. Wang X, Li B, Zhou X, Liao Y, Tang J, Liu T, et al. Changes in brain gray matter in abstinent heroin addicts. Drug Alcohol Depend (2012) 126(3):304–8. doi:10.1016/j.drugalcdep.2012.05.030
40. Liu H, Hao Y, Kaneko Y, Ouyang X, Zhang Y, Xu L, et al. Frontal and cingulate gray matter volume reduction in heroin dependence: optimized voxel-based morphometry. Psychiatry Clin Neurosci (2009) 63(4):563–8. doi:10.1111/j.1440-1819.2009.01989.x
41. Yuan K, Qin W, Dong M, Liu J, Sun J, Liu P, et al. Gray matter deficits and resting-state abnormalities in abstinent heroin-dependent individuals. Neurosci Lett (2010) 482(2):101–5. doi:10.1016/j.neulet.2010.07.005
42. Lyoo IK, Pollack MH, Silveri MM, Ahn KH, Diaz CI, Hwang J, et al. Prefrontal and temporal gray matter density decreases in opiate dependence. Psychopharmacology (2006) 184(2):139–44. doi:10.1007/s00213-005-0198-x
43. Cousijn J, Wiers RW, Ridderinkhof KR, van den Brink W, Veltman DJ, Goudriaan AE. Grey matter alterations associated with cannabis use: results of a VBM study in heavy cannabis users and healthy controls. Neuroimage (2012) 59(4):3845–51. doi:10.1016/j.neuroimage.2011.09.046
44. Brody AL, Mandelkern MA, Jarvik ME, Lee GS, Smith EC, Huang JC, et al. Differences between smokers and nonsmokers in regional gray matter volumes and densities. Biol Psychiatry (2004) 55(1):77–84. doi:10.1016/S0006-3223(03)00610-3
45. Liao Y, Tang J, Liu T, Chen X, Hao W. Differences between smokers and non-smokers in regional gray matter volumes: a voxel-based morphometry study. Addict Biol (2012) 17(6):977–80. doi:10.1111/j.1369-1600.2010.00250.x
46. Benegal V, Antony G, Venkatasubramanian G, Jayakumar PN. Imaging study: gray matter volume abnormalities and externalizing symptoms in subjects at high risk for alcohol dependence. Addict Biol (2007) 12(1):122–32. doi:10.1111/j.1369-1600.2006.00043.x
47. Mechtcheriakov S, Brenneis C, Egger K, Koppelstaetter F, Schocke M, Marksteiner J. A widespread distinct pattern of cerebral atrophy in patients with alcohol addiction revealed by voxel-based morphometry. J Neurol Neurosurg Psychiatry (2007) 78(6):610–4. doi:10.1136/jnnp.2006.095869
48. Liao Y, Tang J, Corlett PR, Wang X, Yang M, Chen H, et al. Reduced dorsal prefrontal gray matter after chronic ketamine use. Biol Psychiatry (2011) 69(1):42–8. doi:10.1016/j.biopsych.2010.08.030
49. Cowan RL, Lyoo IK, Sung SM, Ahn KH, Kim MJ, Hwang J, et al. Reduced cortical gray matter density in human MDMA (Ecstasy) users: a voxel-based morphometry study. Drug Alcohol Depend (2003) 72(3):225–35. doi:10.1016/j.drugalcdep.2003.07.001
50. Kim SJ, Lyoo IK, Hwang J, Chung A, Sung YH, Kim J, et al. Prefrontal grey-matter changes in short-term and long-term abstinent methamphetamine abusers. Int J Neuropsychopharmacol (2006) 9(02):221–8. doi:10.1017/S1461145705005699
51. Zhou Y, Lin F-C, Du Y-S, Qin LD, Zhao Z-M, Xu J-R, et al. Gray matter abnormalities in Internet addiction: a voxel-based morphometry study. Eur J Radiol (2011) 79(1):92–5. doi:10.1016/j.ejrad.2009.10.025
52. Weng C-B, Qian R-B, Fu X-M, Lin B, Han X-P, Niu C-S, et al. Gray matter and white matter abnormalities in online game addiction. Eur J Radiol (2013) 82(8):1308–12. doi:10.1016/j.ejrad.2013.01.031
53. Han DH, Lyoo IK, Renshaw PF. Differential regional gray matter volumes in patients with on-line game addiction and professional gamers. J Psychiatr Res (2012) 46(4):507–15. doi:10.1016/j.jpsychires.2012.01.004
54. Tanabe J, Tregellas JR, Dalwani M, Thompson L, Owens E, Crowley T, et al. Medial orbitofrontal cortex gray matter is reduced in abstinent substance-dependent individuals. Biol Psychiatry (2009) 65(2):160–4. doi:10.1016/j.biopsych.2008.07.030
55. Noël X, Brevers D, Bechara A. A neurocognitive approach to understanding the neurobiology of addiction. Curr Opin Neurobiol (2013) 23(4):632–8. doi:10.1016/j.conb.2013.01.018
56. He Q, Xiao L, Xue G, Wong S, Ames SL, Xie B, et al. Altered dynamics between neural systems sub-serving decisions for unhealthy food. Front Neurosci (2014) 8:350. doi:10.3389/fnins.2014.00350
57. He Q, Chen C, Dong Q, Xue G, Chen C, Lu Z, et al. Gray and white matter structures in the midcingulate cortex region contribute to body mass index in Chinese young adults. Brain Struct Funct (2015) 220(1):319–29. doi:10.1007/s00429-013-0657-9
58. He Q, Xiao L, Xue G, Wong S, Ames SL, Schembre SM, et al. Poor ability to resist tempting calorie rich food is linked to altered balance between neural systems involved in urge and self-control. Nutr J (2014) 13(1):92. doi:10.1186/1475-2891-13-92
59. He Q, Chen M, Chen C, Xue G, Feng T, Bechara A. Anodal stimulation of the left DLPFC increases IGT scores and decreases delay discounting rate in healthy males. Front Psychol (2016) 7:1421. doi:10.3389/fpsyg.2016.01421
60. Feil J, Sheppard D, Fitzgerald PB, Yücel M, Lubman DI, Bradshaw JL. Addiction, compulsive drug seeking, and the role of frontostriatal mechanisms in regulating inhibitory control. Neurosci Biobehav Rev (2010) 35(2):248–75. doi:10.1016/j.neubiorev.2010.03.001
61. Volkow ND, Wang G-J, Fowler JS, Tomasi D, Telang F. Addiction: beyond dopamine reward circuitry. Proc Natl Acad Sci U S A (2011) 108(37):15037–42. doi:10.1073/pnas.1010654108
62. Zhu X, Zhu Q, Shen H, Liao W, Yuan F. Rumination and default mode network subsystems connectivity in first-episode, drug-naive young patients with major depressive disorder. Sci Rep (2017) 7:43105. doi:10.1038/srep43105
63. He Q, Turel O, Bechara A. Brain anatomy alterations associated with Social Networking Site (SNS) addiction. Sci Rep (2017) 7:45064. doi:10.1038/srep45064
64. Turel O, Bechara A. A triadic reflective-impulsive-interoceptive awareness model of general and impulsive information system use: behavioral tests of neuro-cognitive theory. Front Psychol (2016) 7:601. doi:10.3389/fpsyg.2016.00601
65. Turel O, Bechara A. Effects of motor impulsivity and sleep quality on swearing, interpersonally deviant and disadvantageous behaviors on online social networking sites. Pers Individ Dif (2017) 108:91–7. doi:10.1016/j.paid.2016.12.005
66. Turel O, He Q, Xue G, Xiao L, Bechara A. Examination of neural systems sub-serving Facebook “addiction”. Psychol Rep (2014) 115(3):675–95. doi:10.2466/18.PR0.115c31z8
67. Turel O, Qahri-Saremi H. Problematic use of social networking sites: antecedents and consequence from a dual-system theory perspective. J Manage Inf Syst (2016) 33(4):1087–116. doi:10.1080/07421222.2016.1267529
68. Hare TA, Camerer CF, Rangel A. Self-control in decision-making involves modulation of the vmPFC valuation system. Science (2009) 324(5927):646–8. doi:10.1126/science.1168450
69. Le DSN, Pannacciulli N, Chen K, Del Parigi A, Salbe AD, Reiman EM, et al. Less activation of the left dorsolateral prefrontal cortex in response to a meal: a feature of obesity. Am J Clin Nutr (2006) 84(4):725–31.
70. Bechara A, Tranel D, Damasio H. Characterization of the decision-making deficit of patients with ventromedial prefrontal cortex lesions. Brain (2000) 123(Pt 11):2189–202. doi:10.1093/brain/123.11.2189
Keywords: betel quid dependence, MRI, VBM, dorsolateral prefrontal cortex, ventral medial prefrontal cortex, orbitofrontal cortex
Citation: Yuan F, Kong L, Zhu X, Jiang C, Fang C and Liao W (2017) Altered Gray-Matter Volumes Associated With Betel Quid Dependence. Front. Psychiatry 8:139. doi: 10.3389/fpsyt.2017.00139
Received: 23 June 2017; Accepted: 17 July 2017;
Published: 03 August 2017
Edited by:
Qinghua He, Southwest University, ChinaCopyright: © 2017 Yuan, Kong, Zhu, Jiang, Fang and Liao. This is an open-access article distributed under the terms of the Creative Commons Attribution License (CC BY). The use, distribution or reproduction in other forums is permitted, provided the original author(s) or licensor are credited and that the original publication in this journal is cited, in accordance with accepted academic practice. No use, distribution or reproduction is permitted which does not comply with these terms.
*Correspondence: Weihua Liao, 369535642@qq.com