A Large-Scale Study of Surrogate Physicality and Gesturing on Human–Surrogate Interactions in a Public Space
- 1Synthetic Reality Lab, University of Central Florida, Orlando, FL, United States
- 2Mursion, San Francisco, CA, United States
- 3Department of Communication, Stanford University, Stanford, CA, United States
- 4Universal Creative, Orlando, FL, United States
- 5Department of Statistics, University of Central Florida, Orlando, FL, United States
Technological human surrogates, including robotic and virtual humans, have been popularly used in various scenarios, including training, education, and entertainment. Prior research has investigated the effects of the surrogate’s physicality and gesturing in human perceptions and social influence of the surrogate. However, those studies have been carried out in research laboratories, where the participants were aware that it was an experiment, and the participant demographics are typically relatively narrow—e.g., college students. In this paper, we describe and share results from a large-scale exploratory user study involving 7,685 people in a public space, where they were unaware of the experimental nature of the setting, to investigate the effects of surrogate physicality and gesturing on their behavior during human–surrogate interactions. We evaluate human behaviors using several variables, such as proactivity and reactivity, and proximity. We have identified several interesting phenomena that could lead to hypotheses developed as part of future hypothesis-based studies. Based on the measurements of the variables, we believe people are more likely to be engaged in a human–surrogate interaction when the surrogate is physically present, but movements and gesturing with its body parts have not shown the expected benefits for the interaction engagement. Regarding the demographics of the people in the study, we found higher overall engagement for females than males, and higher reactivity for younger than older people. We discuss implications for practitioners aiming to design a technological surrogate that will directly interact with real humans.
1. Introduction
Technological human surrogates, including robotic humans and virtual humans (e.g., avatars and agents), continue to be used in a variety of applications, including training, education, and entertainment. For example, healthcare training often involves the use of robotic human-like “manikins” (mannequins) or virtual humans as stand-ins for patients when training basic skills (Kenny and Parsons, 2011; Riek, 2015). In entertainment and education (Kanda et al., 2004; Mutlu et al., 2006), robotic human surrogates have been used to simulate real people who are not accessible, such as a celebrity or a historically significant politician, e.g., at the Hall of Presidents at Walt Disney World.
Human surrogates can be realized in different forms, each offering distinct advantages and disadvantages. For example, robotic humans have physicality, meaning that they take up space in the environment. If a robotic human has articulated body parts and/or a motion platform, it might be able to approach an individual, shake her hand, and demonstrate other physical aspects of social interaction. However, the physical realization could limit one’s ability to easily or dynamically change a robot’s appearance. Virtual humans (human surrogates created via computer graphics) typically have a low level of physicality, especially if they are presented in two dimensions (2D) on a flat screen although one can provide the illusion of 3D physicality of a virtual human through a stereoscopic immersive or see-through head-worn display. In either case, if a virtual human needs to shake hands with a user, it is challenging to make the user actually feel the handshake without haptic feedback devices because of the lack of a physical manifestation of the virtual human in the real world. Unlike a robotic human, however, a virtual human’s appearance and behavior can be easily and dynamically changed.
A large number of studies have explored the effects of physicality and gesturing during surrogate interactions with real humans. Preliminary data from the prior studies imply that people perceive and react to surrogates differently, depending on the degree of physicality and gesturing. However, most of the previous studies have relied on entirely subjective measures and were performed in a controlled lab environment where participants knew they were involved in an experiment, compared to a more natural real-world social setting (Sabanovic et al., 2006). If people are aware that they are part of an experiment, the experimental results can be affected. For example, participants might feel as if the experimenters are observing them, and/or anticipating a particular experimental outcome. Landsberger (1958) named this the Hawthorne effect, referring to the original related research carried out at the Hawthorne Works in Cicero, IL, USA. Furthermore, as pointed out by Oh et al. (2016), most studies are carried out using “small samples of college students with little demographic variance. Hence, the generalizability of the results is limited.” While such non-random convenience samples have certain limitations, they can provide useful information (Ferber, 1977; Banerjee and Chaudhury, 2010). To address some of these limitations, we wanted to examine human behavior while interacting with a human surrogate outside of the lab, in a more public setting where the individuals were not aware they were participating in an experiment (more natural circumstances), and their collective demographics were more diverse. After considering several options, including a shopping mall (Satake et al., 2009), we decided on a large-scale human behavior experiment in a public space in one of our university buildings, where we could expect a relatively wide variety of people coming and going over several months. Specifically, we decided to carry out the experiment in the entrance lobby of our building (UCF Partnership III), where we could observe the behavior of every individual who entered the building during designated periods of time.
Given the relatively uncontrolled settings, the uncontrolled circumstances of the people observed, and the expected range of demographics, we decided to take an exploratory approach to the study. Our hope was that we would observe some behavioral distinctions that would inform the design of subsequent more focused hypothesis-driven studies. Specifically, we decided to explore the social behavior of the people in the study as we manipulated the physicality and gesturing of the human surrogate. With respect to physicality, we used a RoboThespian™ robotic human surrogate as described below, which was either physically present with the people in the lobby (“RoboThespian”) or virtually present via a real-time video stream of a remotely situated RoboThespian displayed on three abutted fixed display screens present with the people in the lobby (“screen”). With respect to gesturing, we varied the RoboThespian’s upper-torso gestures such that the gestures were either active and clearly visible (“with gesturing”) or inactive (“without gesturing”). Physicality and gesturing details are shown in Figure 1. For control of the RoboThespian, we employed a “Wizard of Oz” paradigm (Roussos et al., 1996)—a remote real human controlled and spoke for the RoboThespian.
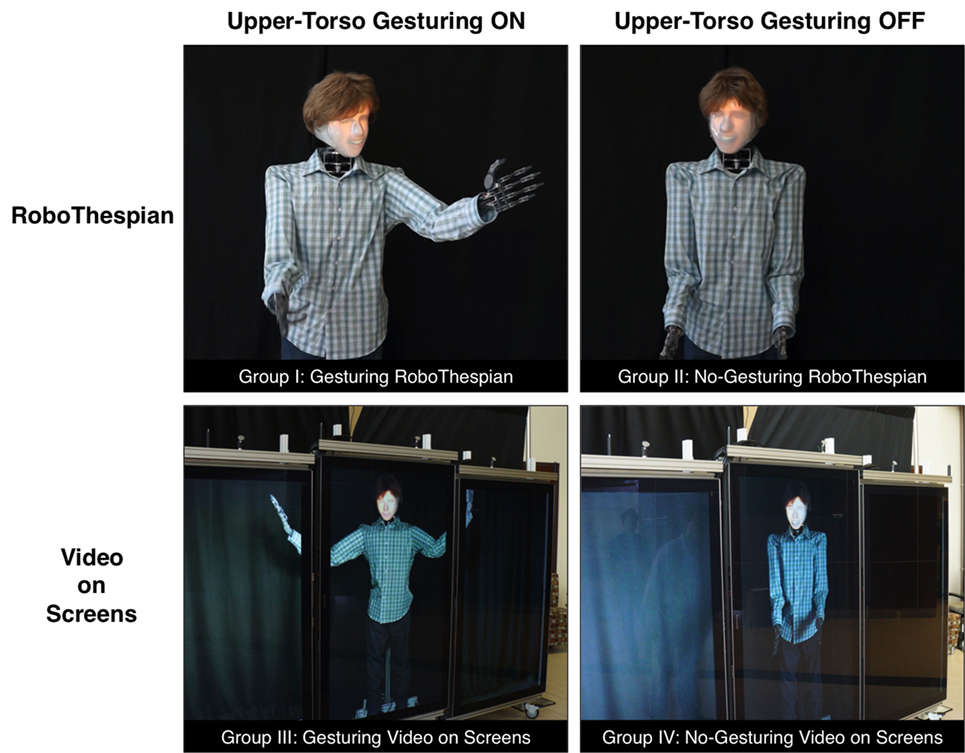
Figure 1. The 2 × 2 experimental conditions across physicality and upper-torso gesturing: RoboThespian vs. video screen × gesturing ON vs. gesturing OFF.
In the end, we observed 7,685 people in the experimental site (i.e., lobby) during 40 2-h sessions carried out during January–May of 2015. Here, we share some findings related to the behavioral differences of the observed people, such as proactivity and reactivity in conversations, under the four conditions of the surrogate corresponding to the four permutations of physicality (RoboThespian vs. screen) and gesturing (with gesturing vs. without gesturing). Ultimately, we hope that our results here, along with future targeted experiments, will lead to guidelines for selecting the right modalities for particular social scenarios when creating human surrogate systems.
2. Related Work
In this section, we position our study on physicality and gesturing in technological human surrogates from two perspectives. First, we examine the ability of technological human surrogates to influence or change human user’s attitudes, emotions, and behaviors (i.e., social influence) while also reviewing human participant’s demographical aspects, such as age and gender, in the influences/changes. Second, we focus more closely on the role of physicality and gesturing in previous studies.
2.1. Social Influence
Social influence refers to the change of one’s attitudes, perceptions, emotions, or behaviors by others (Blascovich, 2002). Within the more specific context of human surrogates, social influence indicates how interacting with a human surrogate can affect or change real humans’ attitudes, emotions, or behaviors.
Previous studies have indicated that virtual human surrogates can have social influence over real humans under different circumstances. For example, some used a virtual human to test social facilitation theory—that one performs simple tasks better and complex tasks worse when in the presence of others. Zanbaka et al. (2007) observed decreases in performance in complex tasks when the virtual human was present, but did not see improvements in simple tasks, while Park and Catrambone demonstrated the effects for both simple and complex tasks with virtual humans (Park and Catrambone, 2007). Guadagno et al. (2007) investigated the role of virtual human gender and behavioral realism in persuasion, and found that the virtual human was more persuasive when it had the same gender as the participant, and exhibited greater behavioral realism. They also found in-group favoritism for female participants, i.e., women liked a female virtual character, while male participants did not show in-group favoritism.
Rosenberg et al. (2013) found that people who helped a virtual human in distress by using a virtual “superpower”—the ability to fly in a virtual environment—were more likely to help people in the real world, even when they were no longer experiencing the virtual environment. Fox et al. investigated the relationship between the perceived agency of virtual surrogates and measures of social influence (e.g., presence, physiological measures, or interpersonal distance). When participants perceived that the virtual surrogate was controlled by a real human (i.e., an avatar), the surrogate was more influential than if it was perceived to be controlled by a computer algorithm (i.e., an agent) (Fox et al., 2015).
Similarly, physical robots can also have a positive effect on social influence. Kiesler et al. (2008) presented preliminary results, indicating that eating habits could be influenced by the presence of robotic or virtual agents. Fiore et al. (2013) studied how the gaze and proxemic behavior of a mobile robot could be perceived as social signals in human–robot interactions and affect one’s sense of social presence with the robot. Siegel et al. (2009) studied the effect of a robotic human’s gender in persuading individuals to make a monetary donation. They found that men were more likely to donate money to the female robot. Kanda et al. (2004) studied the behaviors of elementary students with an interactive humanoid, and found that the younger the participants were, the more time they spent with the robot. Ogawa et al. (2011) developed a humanoid robot and found that people changed their negative feelings toward the robot to positive feelings once they hugged it. In the study, they revealed the aged group tended to have a good impression of the robot from the beginning of the interaction and talk rather than listening to the robot. Walters et al. (2005) researched proxemics with a robot in terms of participant’s age and found that children tended to stand further away from the robot, compared to adults.
The findings above show that people perceive a surrogate they interact with differently according to the type or modality of the surrogate and suggest that some specific modalities might cause people to change their thoughts or behaviors. Also, they suggest that people’s age and gender affect their behaviors with human surrogates. In our experiment, we are particularly interested in exploring how people vary their behaviors and reactions to a surrogate with respect to its physicality and gesturing capabilities while also examining their demographical aspects.
2.2. Physicality and Gesturing
Researchers in robotics and virtual reality have studied the effects of physicality and gesturing in human surrogate systems across a range of surrogate modalities and capabilities. In this context, physicality generally refers to the degree to which a surrogate appears to the user with physical form and shape. Li points out that physicality has two different dimensions in many previous studies: physical embodiment—whether the surrogate has a real/physical body or not (e.g., a telepresent robot vs. a simulated virtual agent with a similar appearance); and physical presence—the fact that it is physically present in front of the user (e.g., robots that are co-present vs. telepresent) (Li, 2015). On the other hand, gesturing refers to actively using the body to convey meaning and emotion, provide instructions, perform an action, or exhibit a personality trait. Intuitively, physicality and gesturing have an inherent co-dependency on each other—a gesturing surrogate can extend or grow its physical presence further into the environment around it. Li also states the relationship between physicality and gesturing by addressing “gesturing may moderate the effect of physical vs. virtual agency” based on his summary of previous studies. Therefore, it is important to consider the effects of physicality and gesturing both individually and in tandem.
To investigate the physicality effect in social influence/human perceptions of the surrogate, many studies have employed both robots and virtual humans. Kiesler et al. (2008) found that people appeared more engaged in a conversation with a robot (compared to a virtual agent), yet were more likely to remember the conversation with the virtual agent. Fischer et al. investigated human responses to different surrogates: a virtual agent with eye-movement only, and robots with varying degrees of freedom in its body movement (e.g., the eyes or head). Participants’ verbal behavior during interactions with each surrogate indicated that the robot’s physicality engendered a greater feeling of interpersonal closeness. Also, higher degrees of freedom in the robot’s movement led participants to report the robot was more indicative during the interaction, in which the participants explained the use of household objects to the robot (Fischer et al., 2012). Segura et al. (2012) found that people preferred to interact with a robotic agent than a virtual agent because of the robot’s physicality, but their overall perceptions of the surrogates were not different—they concluded that the users focused more on the surrogate’s behaviors in the task-based context. Rodriguez-Lizundia et al. used an interactive bellboy robot to evaluate engagement and comfort with different robot designs and behaviors. The physicality of the robot helped people maintain human-like personal distances with the robot and also led to longer interactions with the robot (Rodriguez-Lizundia et al., 2015). Pan and Steed studied the level of trust people have in a surrogate advisor. They tested three surrogate forms: a video of a real human, a virtual human, and a robotic human (Pan and Steed, 2016). Participants had a higher tendency to seek advice from the video or robotic human when making a choice with risk involved. In a medical training context, Chuah et al. (2013) found that even partially increasing the physicality of virtual humans—here by adding a physical lower body (e.g., mannequin legs) to a display showing the virtual human’s upper body—could increase the sense of social presence with the virtual human. In the aspect of tele-communication, Tanaka et al. (2014) conducted an experiment using various communication media, including a virtual avatar and a robot, to check the effect of physicality on distant communication. In the context, the sense of social presence was higher with the surrogate having physicality.
However, it is still controversial which dimension of physicality (i.e., physical embodiment or physical presence) is a more effective factor in affecting human perceptions. Kidd and Breazeal (2004) compared a robot to an animated virtual character and found the surrogate’s physical embodiment—the fact that the robot was real and physical but not its physical presence in front of the users—encouraged higher engagement and positive perceptions of the robot. Conversely, Lee et al. (2006) concluded that the physical presence of the agent elicited higher social presence when they compared a virtual pet to a robotic doppelgänger pet to examine physicality in social interactions between human users and the pet agent. Recently, Li (2015) summarized the results of 33 previous publications related to physicality and suggested that a positive perception was attributed to the physical presence of surrogates, not the physical embodiment based on the summary.
Overall, gesturing appears to have positive effects on human–surrogate interactions. Kilner et al. (2003) found that a human’s gestural behavior was not influenced by the gestures of an industrial robot. However, Oztop et al. (2005) found that a robot with a human-like form could influence a person’s gestures during interactions, and suggested the form of the robot mattered in human perceptions. Kose-Bagci et al. (2009) also investigated the effect of embodiment and gesturing with a humanoid robot and a virtual character, which could play a drum with child participants, and revealed that children enjoyed playing with the physical robot more, especially when the robot could also gesture. In addition, the children’s drumming performance improved with the physically embodied robot. Adalgeirsson and Breazeal (2010) showed that a video-telepresence system with robotic body parts (e.g., arms), which could convey a remote user’s social expressions via physical gestures, could help users be more engaged in the telepresence interaction.
Some researchers were particularly interested in the effect of attentive gestures, such as pointing and gaze, in human–surrogate interactions. Wang and Gratch emphasized that a virtual agent exhibiting only mutual gaze (i.e., eye contact) could reduce the sense of rapport with the agent. They suggested that virtual agents should exhibit some other gestures such as head movements and body postures along with attentive gaze to improve the quality of real-virtual human interaction (Wang and Gratch, 2010). Häring et al. (2012) found that people performed better in solving a jigsaw puzzle when the robot instructor used both gaze and pointing gestures. In a human–human interaction experiment, Boucher et al. characterized the importance of a partner’s gaze behavior for collaborative tasks. Based on the experiment results, they implemented a heuristic method for a robotic agent’s gaze behavior, and showed that the gaze of the robot helped people recognize where the robot’s attention was focused (Boucher et al., 2012). Similarly, Knight and Simmons (2013) presented greater attentional coherence between a robot and humans when the robot exhibited physical indication (head direction) while explaining the surrounding where the robot and the humans were present together. Nagendran et al. (2015) employed two identical robots for a robot-mediated communication of two interlocutors in distant places (transcontinental sites), and observed that people used the robot gestures to convey their thoughts efficiently.
Overall, previous work supports the notion that a human surrogate’s physicality and gesturing, individually and in tandem, are important factors in influencing human perceptions of and behaviors with the surrogate. Our study takes an important next step in studying physicality and gesturing with technological human surrogates by focusing on how these two factors affect objective behavioral measures, while also moving the study of these factors outside of the lab, where we can observe more ecologically valid behavior among a more diverse group of people.
3. Experiment
Here, we describe our exploratory experiment, including the physical arrangements, the study design, the people observed, data collection procedures, and the corresponding dependent variables.
3.1. Surrogate
As described below, we utilized a physical robotic human surrogate called the RoboThespian™ (Figure 2)—a life-size robotic human manufactured by Engineered Arts Limited. For our experimental setting, the RoboThespian was controlled (inhabited) dynamically by a human.
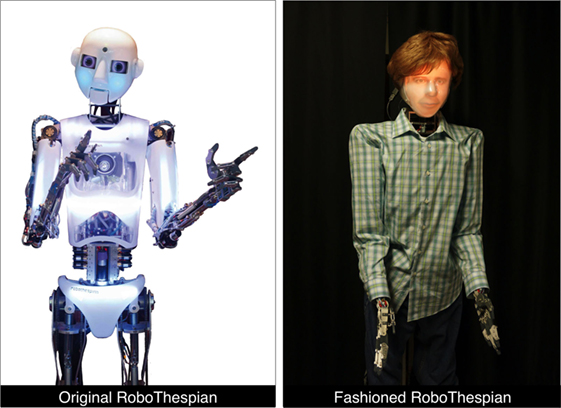
Figure 2. The RoboThespian™ used in the study. To provide a more plausible human-like appearance compared to the system as shipped, we fashioned custom clothing to fit over the metal and plastic frame, and fastened a wig to the head (right photo).
3.1.1. RoboThespian (Robotic Human Surrogate)
The RoboThespian includes a projector and short-throw optics in its head, allowing us to change its facial appearance and expressions dynamically via computer-rendered graphics and animations. To support gesturing, the RoboThespian uses a combination of electric and pneumatic actuation (fluidic muscles). The RoboThespian is fitted with ten fluidic muscles that control the following joints on each arm: shoulders (roll, pitch, and yaw), elbow (pitch), and wrist (pitch). Six independent servo motors control the head (roll, pitch, and yaw) and the torso (roll, pitch, and yaw). On each hand, the thumb is fixed while each of the four remaining fingers is actuated in a binary manner (extended or curled) using directional control valves. The finger actuation is intended to be purely for gesturing, pointing, or other types of non-verbal communication—the lack of thumb actuation and low force exerted when the fingers are closed makes the hands unsuitable for gripping or interacting with objects. As described, the upper-torso of the RoboThespian has a total of 24 independently controllable degrees of freedom. While the legs of the RoboThespian can be actuated (allowing the RoboThespian to squat down), they were fixed in a rigid standing configuration for this study. The natural low impedance characteristics of the pneumatic actuators make the RoboThespian relatively safe for use in an environment where other humans will be nearby during an interaction. We fashioned custom clothing to fit over the metal and plastic frame of the RoboThespian to provide a more plausible human-like appearance while not overly encumbering the motion. In addition to shoes, pants, and a long-sleeve shirt, we also fastened a wig to the head of the RoboThespian to give it hair and to hide the parts of the plastic head shell that did not have projected imagery (Figure 2).
3.1.2. Human in the Loop
We say that a surrogate has agency when a computer algorithm is used to generate autonomous responses during interpersonal communication (including both verbal and non-verbal behaviors). While still having made significant strides, enabling complete agency in technological human surrogates is not yet possible. The current state-of-the-art research in Artificial Intelligence (AI) cannot yet replicate the intelligence level and natural behavior of humans in social interaction. Thus, many previous studies involving social interactions with technically sophisticated surrogate systems have used a human-in-the-loop to control the surrogates (i.e., a Wizard of Oz paradigm with a human controller operating the surrogate behind the scenes).
In this study, a male professional actor was hired and trained to control the surrogate, the RoboThespian, from a remote location. The actor used an interface with an infra-red camera (TrackIR) and a magnetic tracking device (Razer Hydra) to affect the surrogate’s facial animations, head movements, and upper-torso gesturing. The actor was also able to view the environment around the surrogate’s location via a commercial video-chat program (Skype) and a camera set up in the interaction space. The camera was positioned to provide the actor with an approximation of the surrogate’s viewpoint. In addition, the actor wore a microphone headset, which allowed verbal communication with remote people. In this way, the actor could speak naturally while controlling the surrogate and exhibit appropriate verbal and non-verbal responses in context. The details of the control mechanism (AMITIES) are described in Nagendran et al. (2013). For the study we conducted, two different modalities of the surrogate (i.e., surrogate’s physicality and gesturing) were varied to see their effects in human–surrogate interactions (described in the section below); however, the human controller (human-in-the-loop) was not informed of the surrogate condition of the day and could not see the surrogate through the video-chat camera view to the people in the remote place considering the confounding effect of the controller’s surrogate condition awareness.
3.2. Study Design
We prepared a 2 × 2 factorial design to explore the effects of surrogate physicality and gesturing (Figure 1). The independent variable physicality had two levels: (i) the RoboThespian was physically present in the local environment or (ii) three large (65″) screens displayed a real-time video stream of the RoboThespian. The real-time video stream was used to minimize differences between the two levels in physical/behavioral authenticity and the visual fidelity of the human surrogate. The independent variable gesturing also had two possible states: (i) the RoboThespian exhibited gestures with the upper torso (including arms and hands) or (ii) the RoboThespian exhibited no upper torso gestures. Note that, independent of the physicality and gesturing level, the RoboThespian could move its head freely (under the control of the remote actor). This allowed the RoboThespian to show attention and interest by turning its head toward interlocutors while speaking to them. Figure 1 depicts the 2 × 2 factorial design visually, and the four corresponding experimental groups are described below.
• Group I (RoboThespian with Gesturing): people encounter the RoboThespian, which is physically present in the local environment, and the RoboThespian can perform the upper-torso gesturing. The upper-torso gesturing mostly includes arm movement, such as opening arms, hand shaking, and pointing.
• Group II (RoboThespian without Gesturing): people encounter the RoboThespian, which is physically present in the local environment, and the RoboThespian only moves its head but cannot move the upper torso (including arms and hands) at all.
• Group III (Screen with Gesturing): people encounter the video stream of the remotely located RoboThespian through the wide Screen consisting of three aligned large TV displays, and the RoboThespian on the screen can perform the upper-torso gesturing.
• Group IV (Screen without Gesturing): people encounter the video stream of the remotely located RoboThespian through the wide Screen consisting of three aligned large TV displays, and the RoboThespian on the display can only move its head but cannot move the upper torso (including arms and hands) at all.
The surrogate (in a form of the RoboThespian or the Screen) was placed in the lobby of an office building at a university research park for 2 h per day, and—via the controlling actor—greeted and interacted with people as they entered and left the lobby. To maximize the number of human–surrogate interactions in the daily 2 h session, the surrogate was placed in the lobby either from 11:00 a.m. to 1:00 p.m. or from 1:00 p.m. to 3:00 p.m. These session times roughly correspond to the start and end of lunch breaks and, thus, tend to be high traffic periods when people frequently moved in and out of the lobby. There were ten 2-h sessions per experimental group, for a total of 40 sessions in the study. The experimental groups described above were randomly assigned to each of the session time slots.
Although we established explicit independent variables and groups, this study was exploratory in nature, so we did not establish and test specific hypotheses.
3.3. Observed People and Procedure
No selection criteria were applied to limit who could be involved in the experiment. Anyone who entered the lobby was considered as part of the study. Interactions with the surrogate were entirely voluntary, so there was no compensation for the people who interacted with the surrogate in any way. The building where the study took place is home to companies, non-profit trade organizations, military research offices, and academic research labs. The observed people usually included employees/members or guests of these organizations. Most people were adults, although in some cases children passed through the building (e.g., on “Take your Child to Work” Day).
Figure 3 summarizes the procedure the actor followed when controlling the surrogate. When the lobby was empty, the surrogate (the physical RoboThespian or via video stream) stood still and was silent. If people entered the lobby and appeared to be staring at or looking around the surrogate, the actor controlling the surrogate would initiate a conversation with the people. In practice, the actor observed the people for approximately 5 s before initiating the conversation. In some cases, people were more proactive, in that they would initiate the conversation with the surrogate instead. The conversation between the people and the surrogate was not limited to any particular topic, and was mostly casual “small talk.” The actor usually began the conversation by asking whether they (the people and the surrogate) had met before. If the people answered yes, the surrogate would then ask them about his/her general perceptions of the previous interaction. Next, the surrogate would initiate a compliance test, a request of the people that aimed to probe the extent to which they felt socially connected to the surrogate. The compliance test used here was a photo-taking request. The surrogate asked the people to take a photo of itself using a camera located nearby. If they complied, it could imply they felt socially comfortable enough with the surrogate to provide help. After the photo-taking request, the surrogate briefly explained the purpose of the study and asked their permission to use the data collected during the conversation before ending the conversation. Note that the people did not have to continue through this entire process. They could terminate the interaction at any time. Given the public setting and experiment goals, we did not use a written form of informed consent prior to the study. However, people were verbally informed about the details and purpose of the study after the interaction with the surrogate, and they received the phone number of the Principal Investigator as a contact point. This experiment protocol was carried out with the approval of the Institutional Review Board at the University of Central Florida (IRB Number: SBE-16-12347).
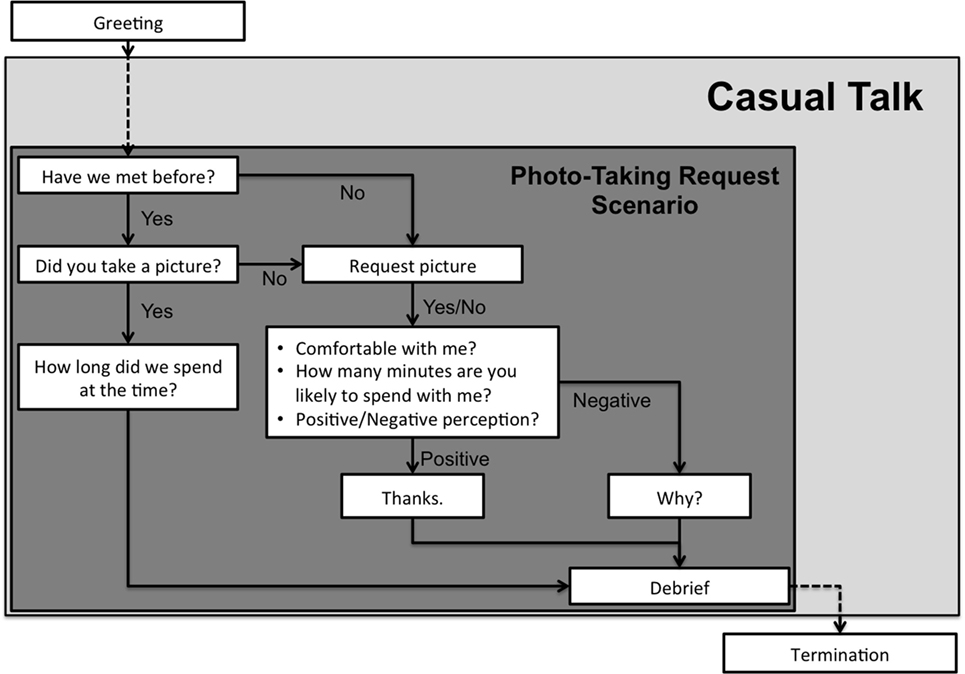
Figure 3. Possible interaction flow between the surrogate and the people. The flow can jump to the termination at any time during the interaction because of the unpredictable interaction pattern—the people could leave any time they wanted.
3.4. Experimental Setup
3.4.1. Surrogate and Environment Settings
Since we had two different states of physicality (the physical RoboThespian, or the video stream on wide-screens), we had to move either the RoboThespian or the screens in/out of the lobby according to the physicality condition of the day. For the Group I and II (RoboThespian) conditions, the RoboThespian and its peripheral devices (e.g., an air compressor and a PC to control the RoboThespian) were placed in the lobby. For the Group III and IV (screen) conditions, the RoboThespian and its peripheral devices remained in our lab space, and three live HD video streams of the RoboThespian from three HD camcorders were run through the building and fed to the three screens in the lobby. Because of the long distance between the lab space and the lobby, we used HDMI-to-Ethernet converters and transmitted the signal through the Ethernet ports in the lab/lobby, as opposed to direct HDMI connections. We needed to make the physical settings practical to set up because we had to move some form of equipment in/out of the lobby for each session. To ease the transition processes, we put most of the equipment on rolling tables and moved them together. Two speakers for conveying the surrogate’s voice (the controlling actor’s voice) were placed on the table behind/near the surrogate (RoboThespian or screens) so that people would perceive the voice as coming from the surrogate. For collecting their behavioral data during the interaction, we installed a Kinect sensor on top of the black curtain rod above the surrogate and placed a microphone on the floor next to the surrogate’s feet. We placed a webcam (for the controlling actor’s view) near the Kinect sensor on the curtain rod. All other devices on the tables were hidden behind black curtains. A camera for the photo-taking task was placed on a chair about 5 m away from the surrogate setup so that the surrogate could point to it during the gesturing conditions. The details of the surrogate and environment settings are shown in Figure 4.
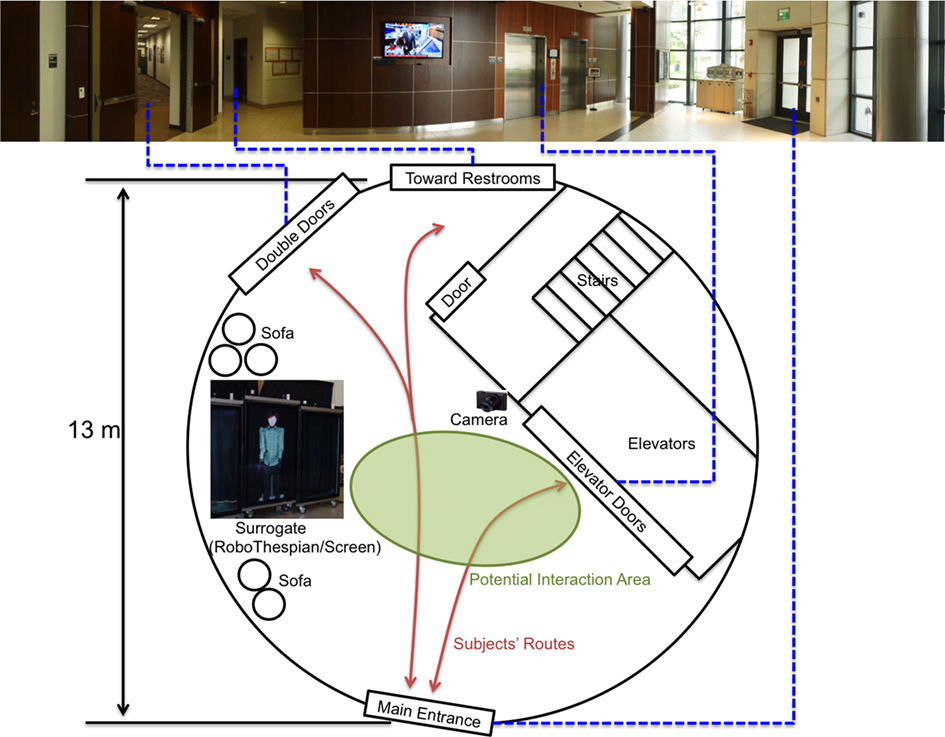
Figure 4. The physical experimental layout. (Top) A panoramic image of the lobby from the surrogate’s perspective. (Bottom) The floor plan of the lobby, with the potential interaction area and typical routes indicated.
3.4.2. Surrogate Control and Communication Connections
The human controller (a trained professional actor) controlled the surrogate from a remote room separated from both the lobby and the lab space while viewing the surrogate environment (lobby) through a commercial video-chat program (Skype). We configured the webcam for the Skype call in the lobby not to see the surrogate so that the controller was not aware of the physicality and gesturing conditions. We also had a human observer for tagging the interesting moments of the interaction next to the controller. The details about the human observer will be explained in the next section. We used multiple client–server software connection frameworks among the human controller, human observer, and the RoboThespian. The framework allowed the controller to manipulate the RoboThespian’s behaviors through controlling devices (refer to Section 3.1.2) and the observer to create pre-defined/custom tags in-situ while the interaction was happening. The controller wore a headset to communicate with people in the lobby through the video call. As the human observer also needed to see and hear the surrogate environment for appropriate tagging, we used a mirroring monitor and audio splitter for duplicating the human controller’s feeds. Overall diagrams for the settings are shown in Figure 5.
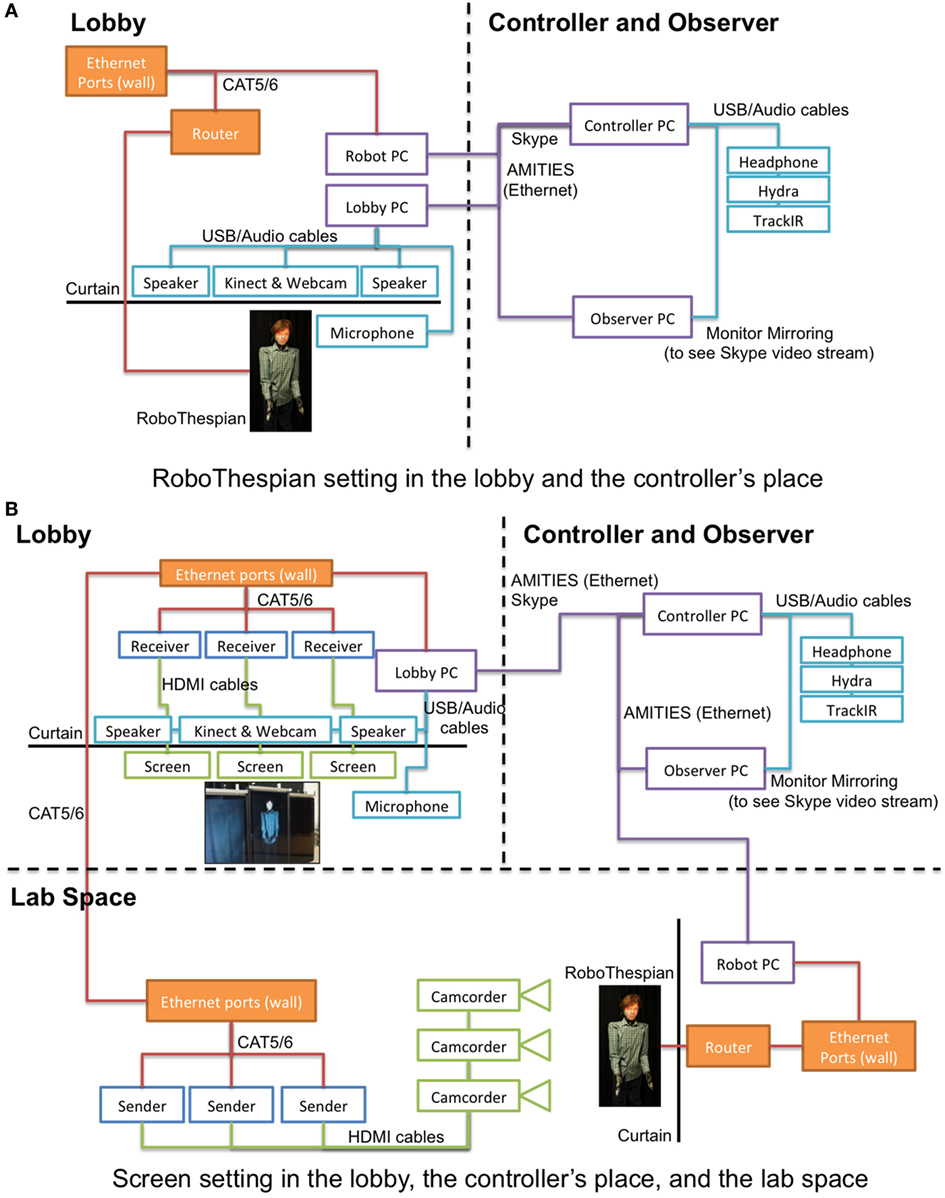
Figure 5. Diagrams indicating the components and connections corresponding to the two physicality conditions. (A) The condition where the RoboThespian is physically present in the lobby. (B) The condition where the RoboThespian is physically present in the laboratory, and viewed by the people in the lobby via HD video feeds displayed on the wide-screen display setup.
3.5. Data Collection and Human Observer
Audio and video data were collected during the interaction between the surrogate and the people in the lobby. For the video, we used a Microsoft Kinect (Kinect for Windows and SDK v1) to capture all the RGB color, depth, and skeletal images. The audio was recorded using the microphone on the floor near the surrogate’s feet. We expected a huge volume of data with a large number of people, and the data collected during this experimental study were unique since it was designed to understand open-ended natural interactions between the surrogate manifestations and real humans, not a controlled study. Thus, to facilitate easier classification of the data, an active observer-based tagging system that allows a person to tag events in real time was implemented. The human observer was seated next to the human controller and could see and hear the same as the controller. While there was anybody in the lobby, the observer created tags using an interface with pre-defined/custom tags (see Figure 6A). The pre-defined tags could describe the increase/decrease of the people in the lobby, whether the people initiated a conversation to the surrogate or vice versa (“Surrogate to Human” or “Human to Surrogate”), and whether the people responded to the surrogate or not (“Response”). These tags helped us to extract the information of interest regarding the dependent variables described in the next section. The distribution of the people based on the tags is shown in Figure 6B. The observer could see the surrogate’s gestures through a virtual character displayed on the interface. Whenever anyone was in the lobby, data recording started by the observer’s call. While collecting all the data (audio, video, and observer’s tags), they have time stamps synchronized to associate with each other after the study.
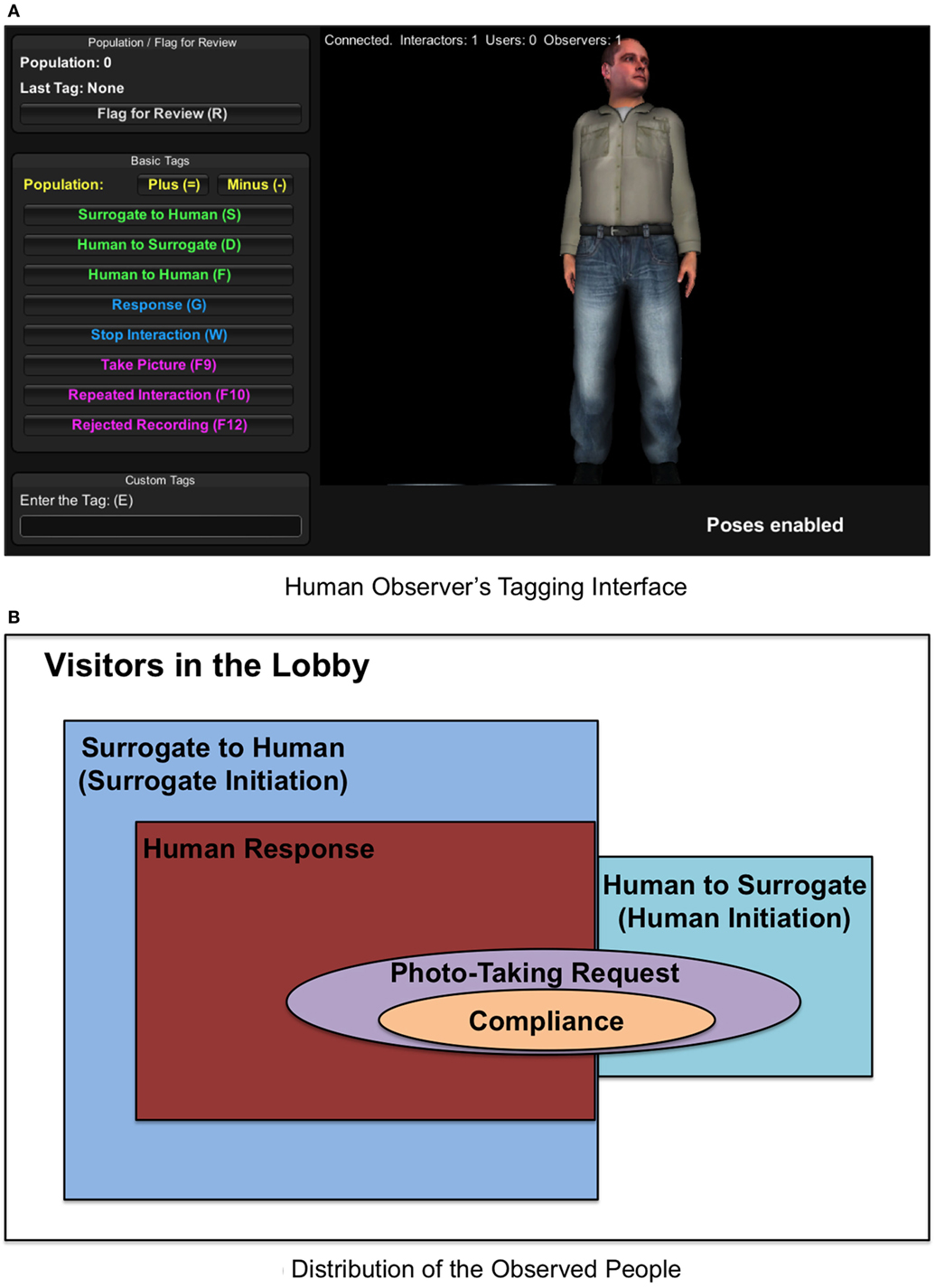
Figure 6. (A) The human observer interface for monitoring the lobby visitors and tagging interesting moments during the interaction. The observer could use pre-defined tags such as “Surrogate to Human” or “Human to Surrogate” (conversation initiations) and could also enter raw notes via a Custom Tag (lower left of the display). The fact that the virtual surrogate’s clothing does not match the clothing we used on the RoboThespian is OK—the only characteristics transferred to the RoboThespian were the face (dynamic appearance) and the body posture (dynamic upper body). (B) A diagram for the distribution of the people in the scope of this study.
3.6. Dependent Variables
The independent variables for the study were the surrogate’s physicality (RoboThespian and wide-screen video stream) and gesturing (upper-torso gesturing ON and OFF). After the study and while we were refining the collected data, we established several interesting aspects as the dependent variables—described below. We generally expected there would be positive associations between human behaviors and the surrogate’s physicality/gesturing on those dependent variables.
• Proactivity: The ratio of the number of people who initiated a conversation with the surrogate before the surrogate said anything to the total number of people who entered the lobby.
• Reactivity: The ratio of the number of people who responded to the surrogate after the surrogate initiated a conversation to the number of people addressed by the surrogate in an attempt to initiate a conversation.
• Commitment: The ratio of the number of people who conversed long enough to receive a photo-taking request from the surrogate to the total number of people who entered the lobby.
• Compliance: The ratio of the number of people who received and complied with the photo-taking request from the surrogate to the number of people who received the request.
• Photo Proximity: How close people stood to the surrogate when they took a photo of the surrogate (complied with the photo-taking request), as indicated by the size of the surrogate’s face in the photo.
4. Analysis and Results
We address the descriptives for four experimental groups (Group I–IV in Section 3.2) in Tables 1–6, but we focus on the comparisons for physicality and gesturing modalities by accumulating the associated groups, e.g., RoboThespian vs. Screen or Gesturing-ON vs. Gesturing-OFF (see Table 7 and Figure 7). In addition, we examine the effects of the observed person’s gender and age in the dependent variables. Here are the groups of interest for the four comparisons: physicality, gesturing, gender, and age.
• (Physicality) Group RoboThespian: Group I (RoboThespian with Gesturing) + Group II (RoboThespian without Gesturing).
• (Physicality) Group Screen: Group III (Screen with Gesturing) + Group IV (Screen without Gesturing).
• (Gesturing) Group Gesturing-ON: Group I (RoboThespian with Gesturing) + Group III (Screen with Gesturing).
• (Gesturing) Group Gesturing-OFF: Group II (RoboThespian without Gesturing) + Group IV (Screen without Gesturing).
• (Gender) Group Male: A group of people who are evaluated as males in video recordings.
• (Gender) Group Female: A group of people who are evaluated as females in video recordings.
• (Age) Group Young: A group of people whose ages are evaluated under 40, including the groups of Children (< 18), Young adults (18–25), and Adulthood (25–40), in video recordings.
• (Age) Group Old: A group of people whose ages are evaluated over 40, including the groups of Middle age (40–60) and Older people (> 60), in video recordings.
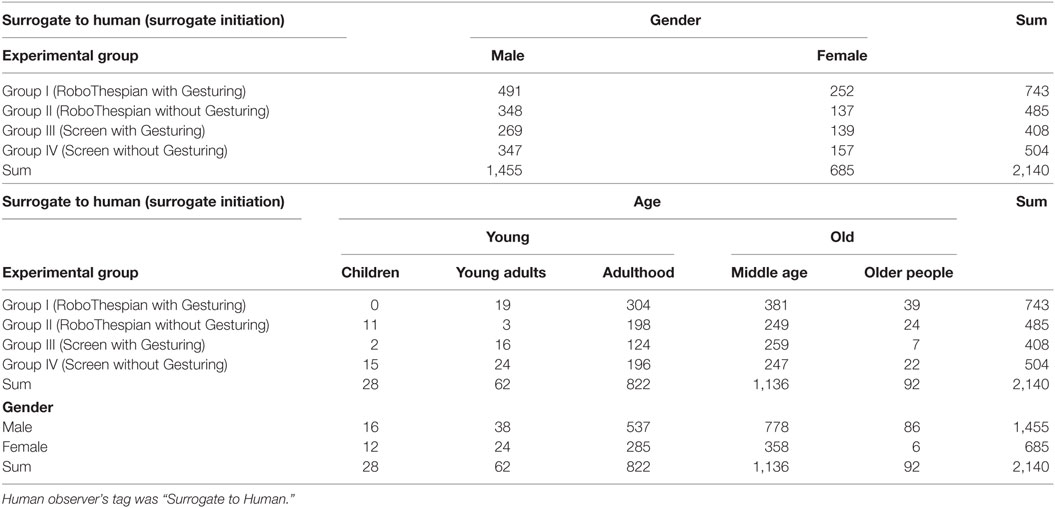
Table 3. The numbers of surrogate initiations—surrogate’s verbal initiations toward the people in the lobby.
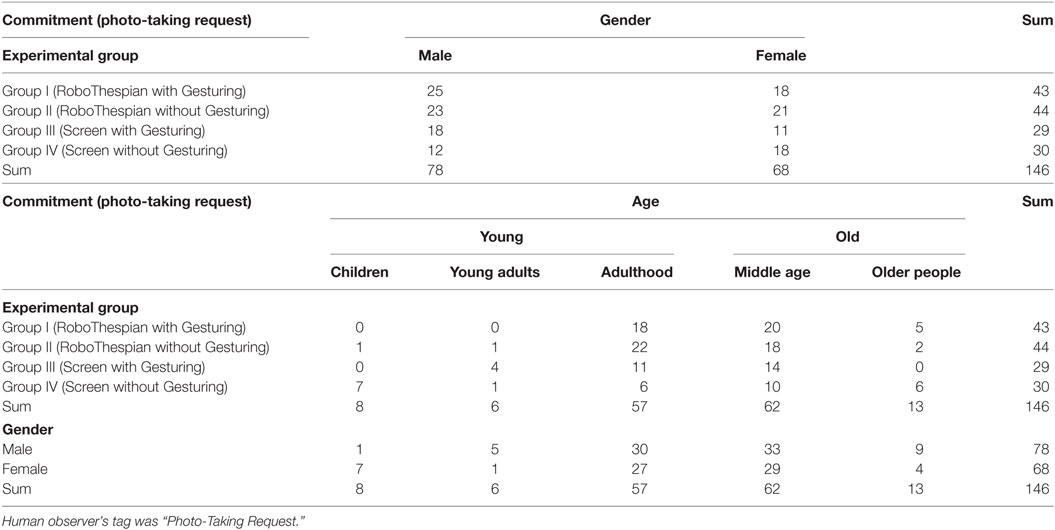
Table 5. The numbers of commitments—people who maintained the verbal interaction with the surrogate until they reached the photo-taking request.
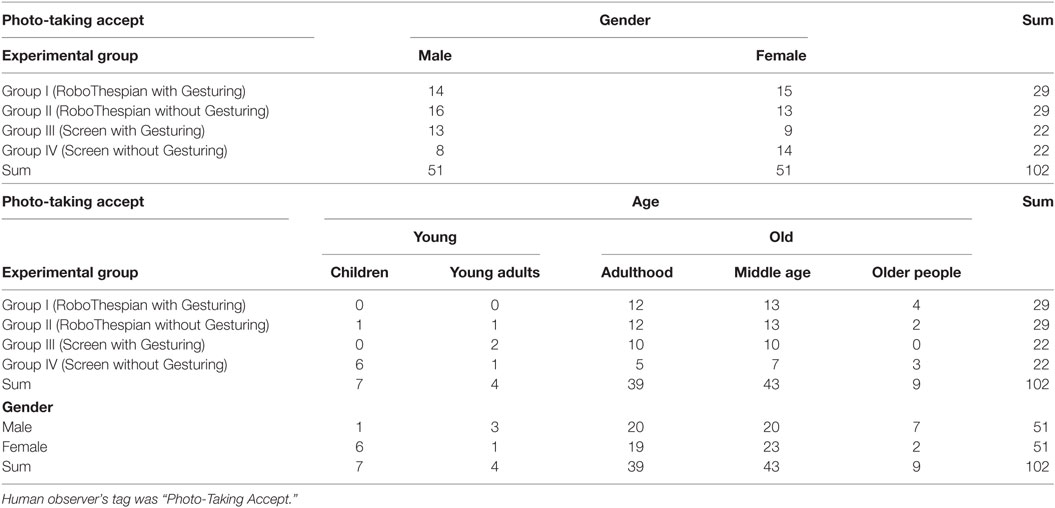
Table 6. The numbers of compliances—people who complied the photo-taking request and took a photo for the surrogate.
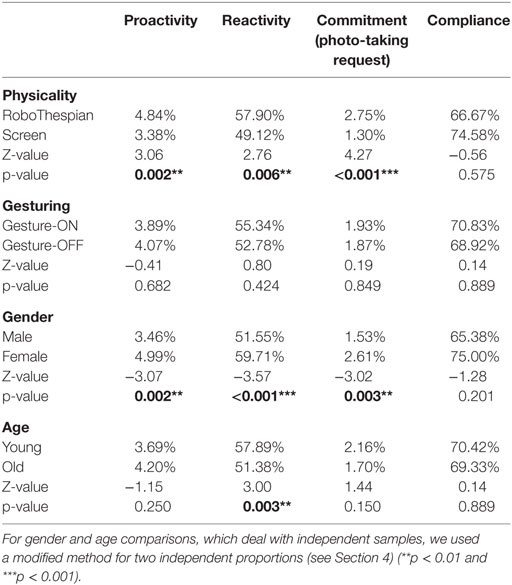
Table 7. Analysis results for physicality and gesturing comparisons by a large sample approximation of two correlated proportions.
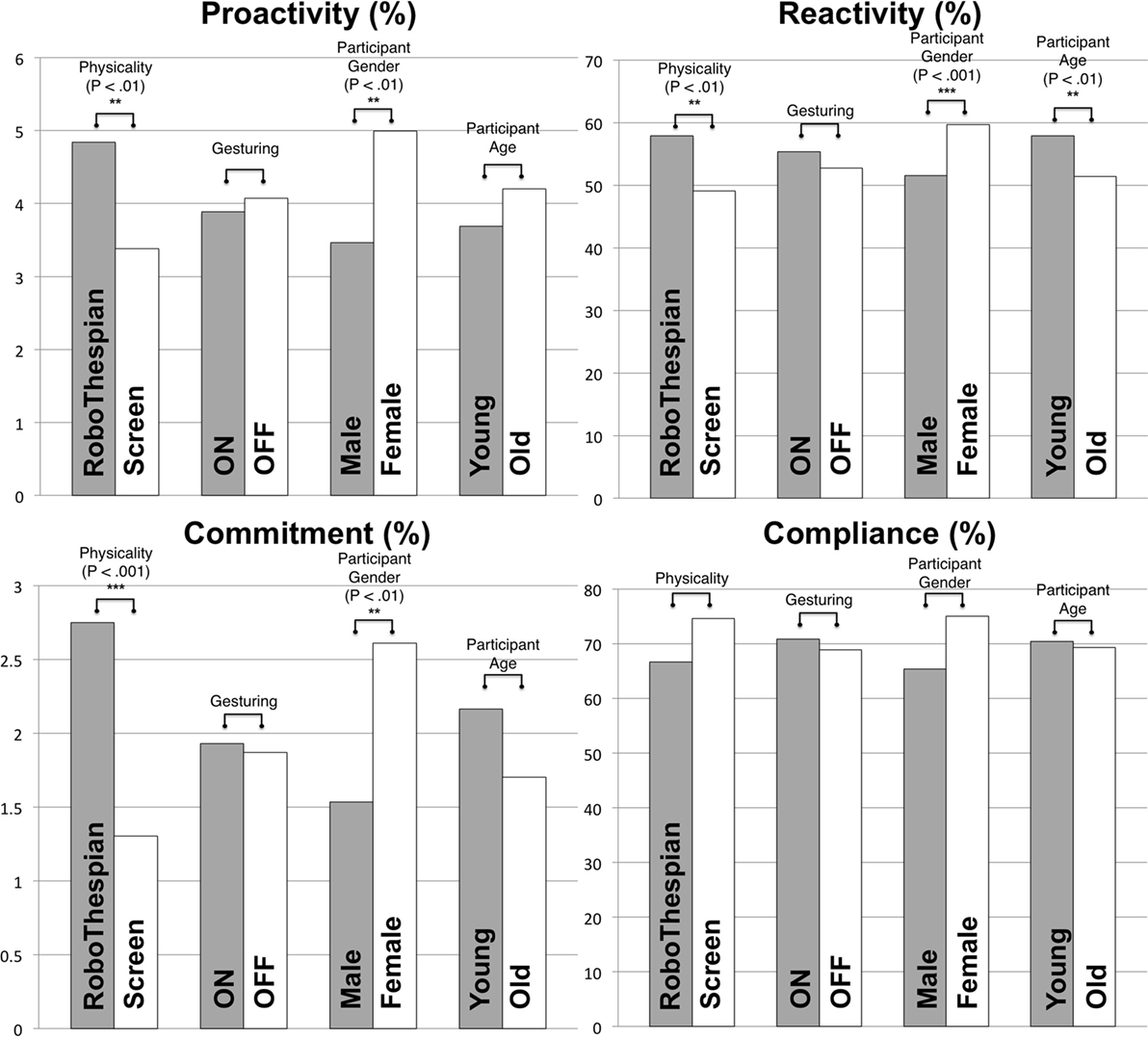
Figure 7. Analysis results. Two proportions comparisons via a large sample approximation in the dependent variables: proactivity, reactivity, commitment, and compliance. There are statistically significant differences in proactivity, reactivity, and commitment for physicality variations and for gender difference. Interestingly, the younger people tended to respond to the surrogate more easily than the older people with statistical significance.
It is important to note that because the study was not in a controlled setting, there were various situations that made the analysis difficult. People in the lobby were coming and going, sometimes talking to the surrogate, sometimes ignoring it, sometimes talking to each other, sometimes interrupting each other, etc.; thus, it was difficult to arrive at an exact number of interactions and people. It helps to understand how we recorded and analyzed the data. We did not record data during the entire 2-h sessions. Instead, we would only start recording when one or more people entered the empty lobby from any direction—door, hallways, or elevator; and stop recording when the lobby was again empty. As such, during any given 2-h session, we had many such “recordings”—a segment of data that begins when one person enters the lobby and ends when it becomes empty again. Over the course of the entire study, a total of 3,942 recordings were collected. The recordings contain all the people during the 40 study sessions even including those who did not have any verbal interactions with the surrogate and who were just walking through the lobby. Also, we had a real human observer who was creating timestamped tags for interesting moments while the lobby was not empty (see Section 3.5). Due to the large number of the recordings and the complexity, we mainly relied on the tags that the human observer created during the study to examine the dependent variables that we are interested in (refer to Section 3.6). Nevertheless, we needed to review all the images/videos containing the people in the lobby to confirm the tags, count the number of people, and code their demographic information. Five human coders, including the first author of this paper, reviewed the images/videos and manually approximated the people’s gender and age. From this intensive reviewing process, we counted a total of 7,685 people (see Table 1) and built the demographical data of their gender and age. These gender and age were used as comparison criteria for the analysis of the dependent variables along with the surrogate’s physicality and gesturing. To limit potential coding biases, we developed the coding criteria together with the coders, and then each practiced and tested their individual coding against the reference coder, which is the first author. For example, the coders only evaluated people who were visually present in the video clips and considered separate people if they were in different video clips (i.e., different interaction segments). The coders cross-checked their initial codings of one day of video clips with the reference coder’s and confirmed that more than ninety percent and eighty percent of their evaluations were consistent with the reference in the codings of the gender and the age, respectively. Also, we tried to distribute the tasks evenly between the five coders in terms of the surrogate’s physicality and gesturing conditions, so that the coders’ variation could be minimized.
For the aforementioned complexity of the uncontrolled experiment, it is also difficult to arrive at an exact number of unique people who interacted with the surrogate, and which interactions might have been repeated interactions by the same people, potentially over different sessions or conditions. Because of this, the people observed in the experiment could be partially dependent among the experimental groups. Due to this unique characteristic of the data, we use a large sample approximation of two correlated proportions for comparison that can be employed for partially dependent samples in most variables. For the method, we use a modified formula for an estimate of the variance as in Equation (2)—the last covariance term reflects the dependency of the samples—and evaluate it with a two-tailed z table (significance level α = 0.05).
where ni and mi are the numbers of observed people in the group of interest according to the dependent variables (refer to Section 3.6). The last term of Equation (2)—the covariance part—reflects the sample dependency. If the samples are independent, this term would be eliminated. Also, if the samples are fully dependent (i.e., repeated measure), the n1 and n2 would be the same, but in our case the numbers of people that we evaluate are different without a way to cover this partially dependent situation. Hence, we use the formula for dependent samples with different sample sizes, n1 and n2. Note that we ignored the covariance part for comparison of two independent proportions, for example, for gender/age comparisons. For the photo proximity that involves only independent samples, we do Chi-squared tests.
We also refined and analyzed additional data from the Kinect sensor, including skeleton data and depth images, for the observed people’s motion dynamics such as two-handed movements in the lobby. However, we think the data acquired with the Kinect sensor was not accurate enough to reliably conclude any results on the dynamics of human–surrogate interactions in our experiment, and we could not find any significant difference in the motion dynamics data. Thus, we do not include the analysis in this paper.
4.1. Proactivity and Reactivity
First, we evaluate the people’s proactivity and reactivity. For these variables, we count the number of verbal initiations and responses. The proactivity is defined as the number of people who initiate a verbal conversation divided by the total number of people (Tables 1 and 2; Figure 7). The reactivity is calculated as the number of people who respond to the surrogate’s verbal initiation, divided by the total number of the surrogate’s verbal initiations (Tables 3 and 4; Figure 7).
As analysis results, the proactivity shows statistically significant differences in the physicality comparison between the Group RoboThespian and the Group Screen (Z = 3.06, p = 0.002), and also in the observed people’s gender comparison between the Male group and the Female group (Z = −3.07, p = 0.002) (see Table 7 and Figure 7). However, it does not present any significant differences with respect to the surrogate’s gesturing variations and the observed people’s age variations. Based on this result, it appears the people in the Group RoboThespian tended to initiate a verbal interaction more voluntarily/proactively before the surrogate started the conversation than the Group Screen, and females seemed to be more proactive than males.
Similarly for the reactivity, although there does not appear to be a statistically significant difference between the gesturing variations, there do appear to be statistically significant differences in the physicality variations, the observed people’s gender and age. With respect to the physicality variations: the Group RoboThespian and the Group Screen, there are significant differences in the reactivity (Z = 2.76, p = 0.006), in the age comparison (Z = 3.00, p = 0.003), and in the gender comparison (Z = −3.57, p < 0.001). Given the result, it appears that the people in the Group RoboThespian tended to be more likely to respond to the surrogate’s verbal initiation compared to the Group Screen while the Group Young tended to be more reactive than the Group Old. Besides, the Group Female seemed to be more reactive than the Group Male.
4.2. Commitment
It would be ideal if we could measure the exact time that the observed people spent with the surrogate as a numerical measure of their commitment; however, it was difficult to evaluate the actual interaction time from the recordings and associated data because we could not reliably identify the exact moment when an interaction finished due to aforementioned intractable dynamics of interactions (see Section 4). People sometimes had multiple pauses in the middle of a conversation for various reasons (another person interrupted, ignoring the surrogate, etc.) and multiple people could jump into another person’s interaction with the surrogate. Thus, we had to develop heuristics to decide when the interaction ended. Also, the large number of interactions made it difficult to review all the videos for evaluating the interaction durations. One reliable metric that we did have was if a conversation progressed long enough to reach the photo-taking request moment. Thus, here we assume that it was a sufficiently long conversation in those cases and analyze the ratio of the number of people who reached the photo-taking request over the total number of people who entered the lobby. The number of photo-taking requests for each group is shown in Table 5, and the ratios for the groups are in Table 7 and Figure 7. A large sample approximation analysis showed that there is a statistically significant difference in the ratio of photo-taking request between the Group RoboThespian and the Group Screen (Z = 4.27, p < 0.001), and there is also a significant difference between the Group Male and the Group Female (Z = −3.02, p < 0.003). However, no significant differences are found in the different gesturing groups and the age groups. The result suggests that the people for the Group RoboThespian more likely spent sufficient time interacting with the surrogate until they reached the photo-taking request moment, compared to the people for the Group Screen. Also, females seemed to reach the photo-taking request more easily than males.
4.3. Compliance
We wanted to check the people’s compliance rate for the task of photo-taking among the surrogate conditions, and expected to see a higher compliance rate in the Group RoboThespian and the Group Gesturing-ON than the counterpart groups. We had surmised that we might observe a difference, but the results do not bear that out; there does not appear to be any statistically significant difference among the experimental groups. The numbers of task compliance for the groups and the test results are shown in Tables 6 and 7 and Figure 7.
4.4. Photo Proximity
We also evaluated the photos taken by the people who interacted with the surrogate. By analyzing the size of the surrogate’s face on the photos, we found that the Group RoboThespian tended to take the photos more closely and to have a larger surrogate face on the photos, compared with the Group Screen. For a statistical analysis (Chi-squared tests), we calculated the average surrogate face size (1,988 pixels out of 640 × 480) from the entire set of photos and separated the photos into two groups: “Large” group having the surrogate face larger than the average size and “Small” group having the smaller surrogate face than the average size. The results from the Chi-Squared tests show that there is a significant difference in the surrogate’s face size on the photos between the Group RoboThespian and the Group Screen, χ2(1, N = 99) = 4.632, p = 0.031, but not any significant difference between the gesturing variations (Figure 8).
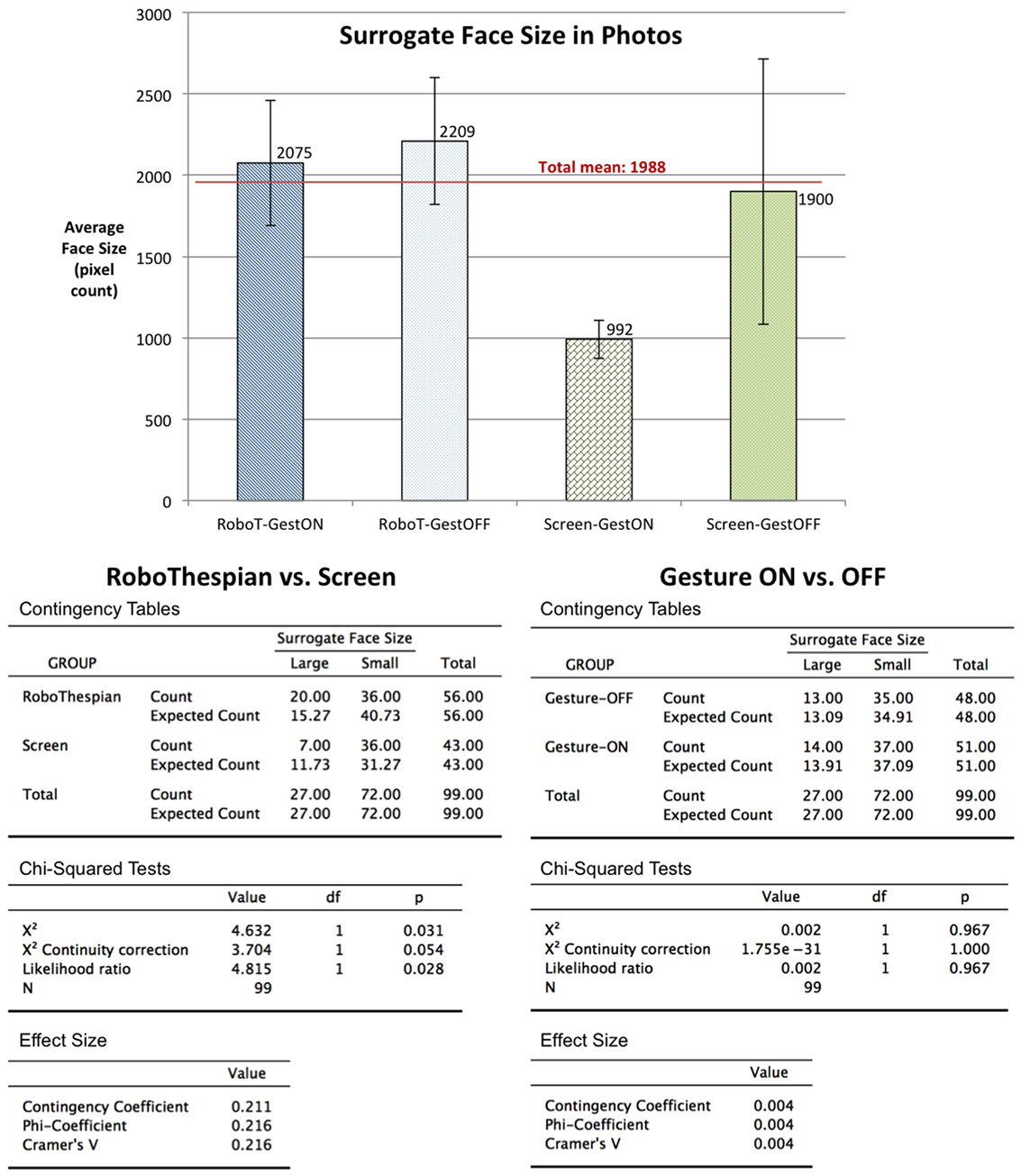
Figure 8. Top: a comparison of the surrogate’s face size in the photographs (640 × 480) that people took (in pixels) among the experimental groups. The graph illustrates the means of surrogate’s face size with standard error bars for each group. Bottom-left: a Chi-square test result in terms of the physicality. There is a statistically significant difference in the face size between the Group RoboThespian and the Group Screen, and the Group RoboThespian more likely had larger surrogate face in the photos, which means that they were close to the surrogate. Bottom-right: a Chi-square test result in terms of the gesturing. There is no statistically significant difference.
5. Discussion
Given the analysis above, there are several results that seem to support the importance of surrogate’s physicality and gesturing in social influence as previous research suggested. Here, we briefly summarize and discuss the findings from the study.
5.1. Proactivity and Reactivity
Physicality Effect: The people with the RoboThespian were more proactive to initiate a conversation with the surrogate and more reactive to the surrogate than the people with the video screen. If these proactivity and reactivity are correlated with engagement, our result for the higher proactivity and reactivity with the RoboThespian would support the idea that physical presence of the surrogate increases the user’s engagement in the interaction, as other previous research suggested (Kidd and Breazeal, 2004; Kiesler et al., 2008). Moreover, humans communicate with each other or another intelligent entity by (verbally) initiating or responding to them; thus, the higher proactivity and reactivity in the experiment could mean that the people treat the surrogate more as a human-like entity, which has enough intelligence to make a conversation. The novelty of the RoboThespian might also interest people in the lobby such that they want to interact with the surrogate.
Gesturing Effect: Based on the statistical analysis, we could not see the gesturing effect in the proactivity and reactivity variables. The people who encountered the surrogate stood static at the beginning, so there might not be enough gesturing stimuli to attract/encourage the people to interact with the surrogate. That might be a reason why we could not see any statistically significant difference among the gesturing variations. However, we found that the RoboThespian with gesturing had the highest rate in both proactivity and reactivity variables. It could be considered that gesturing might be more important/influential when the surrogate has a physical manifestation. Gesturing is intuitively considered a cue for increasing attentiveness (e.g., waving arms to get attention from others) (Wang and Gratch, 2010; Knight and Simmons, 2013); thus, people might pay more attention to the surrogate when the surrogate has a physical gesturing body compared to the body movements on a flat screen.
5.2. Commitment
Physicality Effect: The people with the RoboThespian were more likely to maintain the interaction until the moment for the photo-taking request compared to the people with the video screen. Although we assumed and evaluated the number of photo-taking requests over the total number of the people entering the lobby as a measure of commitment instead of the actual interaction duration, we believe it is a reasonable assumption because reaching the photo-taking request indicates that the people spent sufficient time with the surrogate. Based on the observation that there is a physicality effect in this commitment, but no significant effect of the gesturing, we suggest that the physical manifestation, which can give one the physical sense of co-presence with something/someone, might be more effective for attracting people to stay longer. The novelty of the RoboThespian might also play a role to encourage the longer interaction as well. This result agrees with the finding of longer interactions with an embodied robot from Rodriguez-Lizundia et al. (2015).
Only 146 people maintained the interaction until the photo-taking request out of 7,685 people. Even if we only consider the number of people who had conversations with the surrogate, i.e., the sum of human initiations (N = 306) and human responses (N = 1,159), 146 might seem like a relatively small number; however, it is not clear that people would have spent more time with an actual human being in the lobby compared to the surrogate.
5.3. Compliance
The variations of the surrogate’s physicality and gesturing did not appear to cause any changes in the people’s compliance for the photo-taking task. Based on video reviews of the interactions between the people and the surrogate, there were several cases where the people rejected the photo-taking request. Some people said that they had to leave for their next meeting but seemed to take the request seriously. Some others seemed to not take the request seriously. To increase our confidence regarding the lack of seriousness on the photo request with the surrogate, we could carry out an experiment to measure compliance when a real human asks; however, we could not answer this question with our current data.
5.4. Photo Proximity
Physicality Effect: The people with the RoboThespian took the photos closer to the surrogate, resulting in larger faces in the photos of the RoboThespian compared to photos taken by the people with the video screen. The representations of the surrogate’s body and head in both the RoboThespian Group and the Screen Group had the same size in the real space. We see a couple of possible reasons for this closer photo-shoot with the RoboThespian. First, the people might have felt more comfortable with the human-like physical body of the RoboThespian; so, they came closer. Second, observing the photos, the people in the Screen Group tended to include the entire three wide displays on the photos, which resulted in a smaller surrogate face in the photo. If the physical display had the same size as the RoboThespian’s physical body, people might have taken photos with a similar size of the surrogate’s face both for the Screen and the RoboThespian settings. This could suggest that they perceived the physical manifestations of the surrogate (i.e., the body of the RoboThespian or the displays) as a target object for the photo-shoot, compared to the visual imagery of the surrogate on the display. This might reinforce the importance of the physicality in human perception indirectly.
5.5. Misc. Observations
There are several situations that interest us related to the interaction between the surrogate and the people other than the variables addressed above. For example, there were several people trying to identify the surrogate’s agency—whether it was controlled by a computer algorithm or a real human. One of them kept asking a lot of math questions, such as “what is one plus one?”—apparently to check the intelligence of the surrogate. This seems to support the notion that the agency of the surrogate could influence a people’s perception of the surrogate, as Fox et al. (2015) presented. Also, some people exhibited impolite verbal behaviors with the surrogate. Both the agency-checking and impolite behaviors could imply that they were not aware of the existence of the human controller behind the scene (human-in-the-loop) and treated the surrogate as an autonomous agent during the interaction. This suggests that our intention to allow the surrogate to exhibit adaptability and intelligent interaction with the people by adapting the Wizard of Oz paradigm not only helped the smooth verbal interactions but also kept the surrogate perceived as actual contemporary surrogate systems; thus, our findings could be potentially generalizable to actual human–surrogate interactions.
In summary, considering the objective human behavior data from the study, we believe the surrogate’s physicality generally plays a more significant role in increasing engagement in the interaction with the surrogate, compared to any gesturing feature. On the other hand, gesturing may play an important role in attracting one’s attention to the surrogate or a nearby object when it has physical manifestations.
6. Conclusion
Our work here is motivated by broad interests in the effects of a human surrogate’s physicality and gesturing during human–surrogate interactions, and an interest in “breaking free” from the confines of the typical laboratory-based controlled experiment. We were interested in a setting that reduced individual awareness of the experiment while simultaneously increasing the quantity of individuals and the diversity of their demographics. We were also interested in experimental measures that were both unobtrusive and objective. Given these motivations, and some practical considerations, we decided that the lobby of one of our university buildings would provide an ideal setting. We were able to instrument the space with a variety of unnoticeable behavioral measures, and to collect data over a relatively long period of time (several months), for all conditions of interest.
Considering the large number of 7,685 people and the natural setting, we decided against a hypothesis-based experiment, but instead approached this as an exploratory study. While measuring behaviors “in the wild” has advantages, e.g., the ability to observe natural interactions without experimental biases, the lack of control over the people, and absence of explicit written questionnaires meant that it was challenging to tease out some of the interesting aspects. We defined several variables of interest related to human–surrogate interactions and extracted measures from the (substantial) data collected during the interactions. Our measures included the people’s conversational proactivity with and reactivity to the surrogate, their commitment to the interaction (based on the duration of the interaction), and task compliance. The results provided statistically significant support for positive effects of the surrogate’s physicality related to the human social behavior, but we found no benefits of movements or gesturing of its body parts. This aligns with findings from previous research where people exhibited more favorable responses with physical surrogates than virtual surrogates, and were more engaged in the interaction with the physical surrogates, and supports the idea that the surrogate’s physical presence with the human is the influential factor. Along these lines, we intend to evaluate the illusion of physicality via augmented reality displays in future work to see if the effects can be replicated. Regarding the demographics of the people, we found higher overall engagement for females than males and higher reactivity for younger than older people.
While our exploratory study was not aimed at supporting any explicit hypotheses, we hope that the results—given the sheer size of the study—will inform practitioners and researchers in future studies looking at related effects in “wild” or controlled settings. More generally, while not every use of a surrogate fits our “lobby greeting” scenario, we hope the results will help guide the use of surrogates in similar applications in this growing field.
Ethics Statement
This study was carried out with the approval of the Institutional Review Board at the University of Central Florida (IRB Number: SBE-16-12347). Given the public setting and experiment goals, we did not use a written form of informed consent prior to the study; however, participants were verbally informed the details and purpose of the study after the interaction with the surrogate, and they received the phone number of the Principal Investigator as a contact point.
Author Contributions
JB, AN, and GW initially developed the idea to undertake a large-scale user study in a public space and then designed the study. With the exception of AR, GB, and XY (who joined later), all of the authors participated in the study preparation process, including managing the experiment logistics (e.g., the physical settings, the control, and data collection programs) and deciding what we would measure. KK, ML, and RS led on the implementation of the measures, etc. KK managed the study throughout—he scheduled the sessions, managed the equipment movement/setup, managed the control and collection aspects during the sessions, analyzed the data, and led development of the initial draft of the manuscript. ML and RS helped to run the study with KK. GW contributed to the data analysis by developing ideas and advising KK. KK, AR, RS, and GW wrote most of the manuscript, proof-read the paragraphs, and structured the narrative of the paper overall. JB initiated and directed the demographic analysis. GB helped uncover new insights related to demographics and prior analysis results. XY directed the study design with view on the statistical analysis. JB, GB, and XY further contributed to the interpretation of the results, narrative and presentation aspects of the paper. Finally, all the authors reviewed and edited the paper in the end.
Conflict of Interest Statement
The authors declare that the research was conducted in the absence of any commercial or financial relationships that could be construed as a potential conflict of interest.
Acknowledgments
The authors would like to acknowledge members of SREAL at the University of Central Florida, especially thanking Eric Imperiale (3D Artist) and Brian Kelly (Software Developer). We would also like to thank Max Hilend (Human Controller for the surrogate) and Andrew Carnero (Human Observer for tagging) for their efforts in running the study and Manuel Gonzalez who helped us to refine and analyze the data.
Funding
The work presented in this publication is supported primarily by the Office of Naval Research (ONR) Code 30 under Dr. Peter Squire, Program Officer (ONR awards N00014-14-1-0248 and N00014-12-1-1003). We also acknowledge Florida Hospital for their support of GW via their Endowed Chair in Healthcare Simulation.
References
Adalgeirsson, S. O., and Breazeal, C. (2010). “MeBot: a robotic platform for socially embodied telepresence,” in ACM/IEEE International Conference on Human-Robot Interaction, Osaka, 15–22.
Banerjee, A., and Chaudhury, S. (2010). Statistics without tears: populations and samples. Ind. Psychiatry J. 19, 60–65. doi: 10.4103/0972-6748.77642
Blascovich, J. (2002). “Social influence within immersive virtual environments,” in The Social Life of Avatars, Computer Supported Cooperative Work, ed. R. Schroeder (London: Springer), 127–145.
Boucher, J.-D., Pattacini, U., Lelong, A., Bailly, G., Elisei, F., Fagel, S., et al. (2012). I reach faster when i see you look: gaze effects in human-human and human-robot face-to-face cooperation. Front. Neurorobot. 6:3. doi:10.3389/fnbot.2012.00003
Chuah, J. H., Robb, A., White, C., Wendling, A., Lampotang, S., Kopper, R., et al. (2013). Exploring agent physicality and social presence for medical team training. Presence Teleop. Virt. Environ. 22, 141–170. doi:10.1162/PRES_a_00145
Fiore, S. M., Wiltshire, T. J., Lobato, E. J. C., Jentsch, F. G., Huang, W. H., and Axelrod, B. (2013). Toward understanding social cues and signals in human-robot interaction: effects of robot gaze and proxemic behavior. Front. Psychol. 4:859. doi:10.3389/fpsyg.2013.00859
Fischer, K., Lohan, K., and Foth, K. (2012). “Levels of embodiment: linguistic analyses of factors influencing HRI,” in International Conference on Human-Robot Interaction, Boston, MA, 463–470.
Fox, J., Ahn, S. J. G., Janssen, J. H., Yeykelis, L., Segovia, K. Y., and Bailenson, J. N. (2015). Avatars versus agents: a meta-analysis quantifying the effect of agency on social influence. Hum.Comput. Interact. 30, 401–432. doi:10.1080/07370024.2014.921494
Guadagno, R. E., Blascovich, J., Bailenson, J. N., and Mccall, C. (2007). Virtual Humans and persuasion: the effects of agency and behavioral realism. Media Psychol. 10, 1–22. doi:10.1080/15213260701300865
Häring, M., Eichberg, J., and André, E. (2012). “Studies on grounding with gaze and pointing gestures in human-robot-interaction,” in Lecture Notes in Artificial Intelligence (International Conference on Social Robotics), Vol. 7621, Chengdu, 378–387.
Kanda, T., Hirano, T., Eaton, D., and Ishiguro, H. (2004). Interactive robots as social partners and peer tutors for children: a field trial. Hum.Comput. Interact. 19, 61–84. doi:10.1207/s15327051hci1901&2_4
Kenny, P. G., and Parsons, T. D. (2011). “Embodied conversational virtual patients,” in Conversational Agents and Natural Language Interaction: Techniques and Effective Practices, eds D. Perez-Marin and I. Pascual-Nieto (IGI Publishing Hershey), 254–281.
Kidd, C. D., and Breazeal, C. (2004). “Effect of a robot on user perceptions,” in IEEE/RSJ International Conference on Intelligent Robots and Systems, Sendai 3559–3564.
Kiesler, S., Powers, A., Fussell, S. R., and Torrey, C. (2008). Anthropomorphic interactions with a robot and robot-like agent. Soc. Cogn. 26, 169–181. doi:10.1521/soco.2008.26.2.169
Kilner, J., Paulignan, Y., and Blakemore, S. (2003). An interference effect of observed biological movement on action. Curr. Biol. 13, 522–525. doi:10.1016/S0960-9822(03)00165-9
Knight, H., and Simmons, R. (2013). “Estimating human interest and attention via gaze analysis,” in IEEE International Conference on Robotics and Automation, Karlsruhe, 4350–4355.
Kose-Bagci, H., Ferrari, E., Dautenhahn, K., Syrdal, D. S., and Nehaniv, C. L. (2009). Effects of embodiment and gestures on social interaction in drumming games with a humanoid robot. Adv. Rob. 23, 1951–1996. doi:10.1163/016918609X12518783330360
Landsberger, H. A. (1958). Hawthorne Revisited: Management and the Worker, Its Critics, and Developments in Human Relations in Industry, 1st Edn. Ithaca, NY: Cornell University Press.
Lee, K. M., Jung, Y., Kim, J., and Kim, S. R. (2006). Are physically embodied social agents better than disembodied social agents? The effects of physical embodiment, tactile interaction, and people’s loneliness in human–robot interaction. Int. J. Hum. Comput. Stud. 64, 962–973. doi:10.1016/j.ijhcs.2006.05.002
Li, J. (2015). The benefit of being physically present: a survey of experimental works comparing copresent robots, telepresent robots and virtual agents. Int. J. Hum. Comput. Stud. 77, 23–37. doi:10.1016/j.ijhcs.2015.01.001
Mutlu, B., Forlizzi, J., and Hodgins, J. (2006). “A storytelling robot: modeling and evaluation of human-like gaze behavior,” in IEEE-RAS International Conference on Humanoid Robots, Genova, 518–523.
Nagendran, A., Pillat, R., Adam, K., Welch, G., and Hughes, C. (2013). A unified framework for individualized avatar-based interactions. Presence Teleop. Virt. Environ. 23, 109–132. doi:10.1162/PRES_a_00177
Nagendran, A., Steed, A., Kelly, B., and Pan, Y. (2015). Symmetric telepresence using robotic humanoid surrogates. Comput. Anim. Virt. Worlds 26, 271–280. doi:10.1002/cav.1638
Ogawa, K., Nishio, S., Koda, K., Balistreri, G., Watanabe, T., and Ishiguro, H. (2011). Exploring the natural reaction of young and aged person with Telenoid in a real world. J. Adv. Comput. Intell. Intell. Inf. 15, 592–597. doi:10.20965/jaciii.2011.p0592
Oh, S. Y., Shriram, K., Laha, B., Baughman, S., Ogle, E., and Bailenson, J. (2016). “Immersion at scale: researcher’s guide to ecologically valid mobile experiments,” in IEEE Virtual Reality Conference, Greenville, SC, 249–250.
Oztop, E., Franklin, D. W., Chaminade, T., and Cheng, G. (2005). Human-humanoid interaction: is a humanoid robot perceived as a human? Int. J. Hum. Rob. 2, 537–559. doi:10.1142/S0219843605000582
Pan, Y., and Steed, A. (2016). A comparison of avatar, video, and robot-mediated interaction on users’ trust in expertise. Front. Rob. AI 3:12. doi:10.3389/frobt.2016.00012
Park, S., and Catrambone, R. (2007). Social facilitation effects of virtual humans. Hum. Factors 49, 1054–1060. doi:10.1518/001872007X249910
Riek, L. D. (2015). “Robotics technology in mental health care,” in Artificial Intelligence in Behavioral Health and Mental Health Care, ed. D. Luxton (Elsevier), 185–203.
Rodriguez-Lizundia, E., Marcos, S., Zalama, E., Gómez-García-Bermejo, J., and Gordaliza, A. (2015). A bellboy robot: study of the effects of robot behaviour on user engagement and comfort. Int. J. Hum. Comput. Stud. 82, 83–95. doi:10.1016/j.ijhcs.2015.06.001
Rosenberg, R. S., Baughman, S. L., and Bailenson, J. N. (2013). Virtual superheroes: using superpowers in virtual reality to encourage prosocial behavior. PLoS ONE 8:e55003. doi:10.1371/journal.pone.0055003
Roussos, M., Johnson, A., Leigh, J., Vasilakis, C., and Moher, T. (1996). “Constructing collaborative stories within virtual learning landscapes,” in European Conference on AI in Education, Lisbon, 129–135.
Sabanovic, S., Michalowski, M. P., and Simmons, R. (2006). “Robots in the wild: observing human-robot social interaction outside the lab,” in International Workshop on Advanced Motion Control, Istanbul, 596–601.
Satake, S., Kanda, T., Glas, D. F., Imai, M., Ishiguro, H., and Hagita, N. (2009). “How to approach humans? Strategies for social robots to initiate interaction,” in ACM/IEEE International Conference on Human-Robot Interaction, La Jolla, CA:109–116.
Segura, E. M., Kriegel, M., Aylett, R., Deshmukh, A., and Cramer, H. (2012). “How do you like me in this: user embodiment preferences for companion agents,” in Lecture Notes in Artificial Intelligence (International Conference on Intelligent Virtual Agents), Vol. 7502, Santa Cruz, CA, 112–125.
Siegel, M., Breazeal, C., and Norton, M. I. (2009). “Persuasive robotics: the influence of robot gender on human behavior,” in IEEE/RSJ International Conference on Intelligent Robots and Systems, St. Louis, MO, 2563–2568.
Tanaka, K., Nakanishi, H., and Ishiguro, H. (2014). “Comparing video, avatar, and robot mediated communication: pros and cons of embodiment,” in Communications in Computer and Information Science, Vol. 460, eds T. Yuizono, G. Zurita, N. Baloian, T. Inoue, and H. Ogata (Berlin Heidelberg: Springer-Verlag), 96–110.
Walters, M. L., Dautenhahn, K., Koay, K. L., Kaouri, C., Boekhorst, R. T., Nehaniv, C., et al. (2005). “Close encounters: spatial distances between people and a robot of mechanistic appearance,” in IEEE-RAS International Conference on Humanoid Robots, Tsukuba, 450–455.
Wang, N., and Gratch, J. (2010). “Don’t just stare at me!,” in ACM SIGCHI Conference on Human Factors in Computing Systems, Atlanta, 1241–1250.
Keywords: technological human surrogates, physicality, gesturing, demographics, social interaction, social influence, behavioral analysis, in-the-wild experiment
Citation: Kim K, Nagendran A, Bailenson JN, Raij A, Bruder G, Lee M, Schubert R, Yan X and Welch GF (2017) A Large-Scale Study of Surrogate Physicality and Gesturing on Human–Surrogate Interactions in a Public Space. Front. Robot. AI 4:32. doi: 10.3389/frobt.2017.00032
Received: 11 January 2017; Accepted: 19 June 2017;
Published: 07 July 2017
Edited by:
Rob Lindeman, University of Canterbury, New ZealandReviewed by:
Andreas Duenser, Commonwealth Scientific and Industrial Research Organisation (CSIRO), AustraliaEmanuele Ruffaldi, Sant’Anna School of Advanced Studies, Italy
Copyright: © 2017 Kim, Nagendran, Bailenson, Raij, Bruder, Lee, Schubert, Yan and Welch. This is an open-access article distributed under the terms of the Creative Commons Attribution License (CC BY). The use, distribution or reproduction in other forums is permitted, provided the original author(s) or licensor are credited and that the original publication in this journal is cited, in accordance with accepted academic practice. No use, distribution or reproduction is permitted which does not comply with these terms.
*Correspondence: Kangsoo Kim, kskim@knights.ucf.edu