Cognitive impairments in schizophrenia as assessed through activation and connectivity measures of magnetoencephalography (MEG) data
- 1 Department of Radiology and Biomedical Imaging, University of California, San Francisco, CA, USA
- 2 Veterans Affairs Medical Center, San Francisco, CA, USA
- 3 Department of Psychiatry, University of California, San Francisco, CA, USA
The cognitive dysfunction present in patients with schizophrenia is thought to be driven in part by disorganized connections between higher-order cortical fields. Although studies utilizing electroencephalography (EEG), PET and fMRI have contributed significantly to our understanding of these mechanisms, magnetoencephalography (MEG) possesses great potential to answer long-standing questions linking brain interactions to cognitive operations in the disorder. Many experimental paradigms employed in EEG and fMRI are readily extendible to MEG and have expanded our understanding of the neurophysiological architecture present in schizophrenia. Source reconstruction techniques, such as adaptive spatial filtering, take advantage of the spatial localization abilities of MEG, allowing us to evaluate which specific structures contribute to atypical cognition in schizophrenia. Finally, both bivariate and multivariate functional connectivity metrics of MEG data are useful for understanding how these interactions in the brain are impaired in schizophrenia, and how cognitive and clinical outcomes are affected as a result. We also present here data from our own laboratory that illustrates how some of these novel functional connectivity measures, specifically imaginary coherence (IC), are quite powerful in relating disconnectivity in the brain to characteristic behavioral findings in the disorder.
Introduction
The clinical and cognitive features of schizophrenia are believed to be due, at least in part, to impairments in the activity of – and the interactions in activity between – functionally critical brain regions (Friston and Frith, 1995; Gold and Weinberger, 1995). The hypothesis that schizophrenia is a “disconnection syndrome” dates back to Bleuler, with the implication that disordered thought processing, social function and cognitive abilities are the result of a “splitting” of mental faculties. The general interpretation of this disconnection hypothesis has been that, in schizophrenia, reduced activity or undercoupling within key brain networks leads to an inability to efficiently modulate incoming neural processes. Only recently, with the advent of non-invasive imaging techniques, has direct evidence for this failure of brain function in schizophrenia been available. Changes in activity and connectivity identified through these methods support a consensus that schizophrenia is the net result of an inability to effectively integrate and process information in the central nervous system, contributing to aberrant patterns of cognition and behavior. However, our understanding of how this failure of integration relates to behavioral performance and illness symptoms in schizophrenia is far from complete. The goal of this article is to overview the role of magnetoencephalography (MEG), an imaging technique with exquisite spatial and temporal resolution, in linking brain function to cognition and disease states in schizophrenia.
Evidence for Functional Disconnectivity in DTI, fMRI, PET and Electroencephalography
The disconnection hypothesis has been recently supported by imaging studies which have shown altered patterns of anatomical brain connectivity in patients with schizophrenia using techniques such as diffusion tensor imaging (DTI; see Kubicki et al., 2005; Kyriakopoulos et al., 2008 for a review). DTI, which measures the diffusion of water molecules along white-matter fiber tracts in the brain, has been a successful approach in demonstrating abnormalities in neural circuitry within this population. These differences in connectivity are often between brain regions associated with higher-order cognitive processing and executive function (Dwork et al., 2007). One consistent finding is that the fractional anisotropy (a DTI index representing the fraction of the tensor that contributes to the anisotropic diffusion) is reduced in patients with schizophrenia (Ardekani et al., 2003; Burns et al., 2003; Kumra et al., 2004). While these studies are highly informative, it is unclear how these deficits in neuroanatomical connectivity directly relate to cognitive impairments identified in patients with schizophrenia.
Consistent with data from DTI, investigations of functional connectivity in PET and functional MRI data both during cognitive tasks (see Ragland et al., 2007; Potkin and Ford, 2009 for a review) and at rest (see Grecius, 2008 for a review) support the hypothesis of neural disconnectivity in schizophrenia. Although related to anatomical connectivity, studies of functional connectivity instead focus on how processing between multiple brain regions are correlated and how information is parsed between multiple nodes of a network (Friston, 1994; Ramnani et al., 2004). Although the notion of “disconnection” in schizophrenia tacitly assumes that an overall pattern of underconnectivity will be identified in this patient population, both increases and decreases in functional connectivity have been identified in this group.
While these investigations of functional connectivity in fMRI and PET are extremely informative, especially at a spatial level, there are significant areas of research that simply cannot be accessed by these imaging methodologies. The sampling resolution of either technique generally occurs, at best, on the order of seconds, which makes it extremely difficult to evaluate how information flows between brain regions. In executive function, information is not only transferred between cortical regions at a rapid rate (on the order of milliseconds) but is also thought to be dependent on processing in different oscillatory domains (alpha, beta, gamma, etc.). Therefore, fMRI and PET are ideal at reconstructing brain activity at very low frequencies (<0.1 Hz) and, presently, are unable to parse out activity in higher frequency bands known to exist in mammalian cortex.
In the human brain, oscillatory activity at high frequencies has traditionally been studied non-invasively using electroencephalography (EEG). Functional connectivity can be examined in EEG by looking at coupling between specific sensors through techniques such as coherence, and a number of studies have demonstrated deviations in sensor coherence in patients with schizophrenia (Leocani and Comi, 1999). There is a lack of agreement in the literature with respect to changes in EEG coherence in schizophrenia, with some studies illustrating increased functional connections in patients (Ford et al., 1986; Merrin et al., 1989) while others have reported reduced sensor coherence (Merrin and Floyd, 1992; Tauscher et al., 1998) or no differences at all (Wada et al., 1998). While it has been proposed from these EEG studies that compromised interactions between the frontal and temporal lobe are a primary feature of schizophrenia (Ford et al., 2002; Strelets et al., 2002; Winterer et al., 2003) the spatial limitations of EEG prevent us from knowing exactly which regions are affected in the disorder.
Magnetoencephalography has begun to receive attention in imaging research as having direct applications to the study of impaired cognitive processes in clinical populations, including schizophrenia (Reite et al., 1999; Näätänen and Kähkönen, 2009). There are significant advantages to using MEG to study neural processes, especially when modeling how activity within a cortical field can influence and interact with activity in other parts of the brain. First, like EEG, MEG is able to reconstruct neural activity on the order of milliseconds. Secondly, recording from MEG sensors allows investigators to access oscillatory neural activity in higher frequency ranges (e.g. alpha, beta, gamma) than those attainable in both fMRI/PET. In addition, the sampling frequency of data acquisition in MEG (generally greater than 1 kHz) is not limited by electrode impedance (as in EEG), permitting the examination of ultra-high frequency (>100 Hz) brain activity in this modality. Thirdly, volume conduction artifacts commonly found in other imaging modalities are significantly reduced in MEG, as structures such as the skull and CSF do not interfere with the propagation of the magnetic fields (Leahy et al., 1998). Finally, novel source localization algorithms allow high-fidelity reconstruction of whole brain activity, also referred to as electromagnetic brain imaging (EBI). EBI provides information about where changes in observed neural activity in MEG data arise in the brain (Robinson and Vrba, 1999; Sekihara et al., 2001; Dalal et al., 2008). The application of EBI is particularly useful for examining changes in timing between disconnected regions identified in DTI and fMRI studies, because of its superior time-scale.
In this article, we will review how MEG, and especially EBI, has been applied to examining impaired social, emotional and cognitive processing in patients with schizophrenia. Special attention will be paid to how studies use MEG to investigate functional connectivity. Finally, we will review some novel metrics for estimating neural interactions in MEG and how these techniques can be applied to further our understanding of the neural mechanisms affected in schizophrenia.
Cognitive Impairments in Schizophrenia as Assessed by MEG Sensor Data
Many of the studies that examine MEG sensor data in schizophrenia have been extensions of EEG studies, where a change in the averaged amplitude at a particular sensor, during a cognitive paradigm, is interpreted to represent aberrations of cortical processing. With the improved spatial resolution of MEG, these studies both independently confirm findings from EEG studies, as well as expand our knowledge of the specific brain regions that contribute to cognitive dysfunction in the disorder.
Information Filtering and Sensory Gating
A reduction in the positive deflection of the EEG waveform around 50 ms following the onset of a repeated stimulus (P50) is thought to represent the attenuation of irrelevant information. This level of filtering of an incoming sensory stimulus, referred to as “sensory gating”, is known to be compromised in patients with schizophrenia (Adler et al., 1982). An inability to separate relevant from irrelevant sensory stimuli is putatively related to sensory overload and distractibility, thereby impacting higher-order cognitive function (attention, maintenance of working memory, self-regulation). Examination of the magnetic component of the P50 (M50) has provided detail about the spatial and temporal characteristics of this mechanism in auditory processing. Standard examinations of sensory gating are generally computed from EEG sensor recordings at a midline location (electrode Cz) providing no information about the origin of the source or hemispheric lateralization effects.
Initial MEG studies have been able to localize the source of the M50 in an auditory stimulus train to the bilateral superior temporal gyrus (STG; Reite et al., 1988). In schizophrenia, impaired sensory gating of the M50 is lateralized, with insufficient sensory gating of an auditory stimulus within the M50 response located in the left hemisphere (Thoma et al., 2003). This lateralized effect in left auditory cortex was also found to be significantly and positively correlated with poor working memory in patients with schizophrenia (Thoma et al., 2003). It has been suggested that such a failure of sensory gating in schizophrenia is due to faulty functional connectivity between the STG with deep brain structures involved in sensory filtering (see Huang et al., 2003). Concurrent EEG/MEG recordings during a paired-click paradigm have shown that while MEG sources in bilateral STG reliably account for most of the P50 EEG component at Cz in healthy control subjects, residual high-gamma activity (∼40 Hz) persists in patients with schizophrenia once the STG sources are accounted for (Huang et al., 2003).
In the frequency domain, a reduced M50 in schizophrenia is thought to be due to faulty habituation of oscillations in the gamma range, possibly due to noisy cortical sources contributing to this signal (Clementz et al., 1997). When sensory gating in the auditory system becomes deficient, it potentially influences downstream processes that are dependent upon efficient filtering represented in the M50, including the component that occurs 100 ms following the stimulus (the M100). In schizophrenia, both the M50 and the M100 have been independently shown to be impaired (Clementz et al., 1997; Thoma et al., 2003). This lack of sensory gating can make it difficult for the patient to discriminate between environmentally relevant sources of auditory information and may have profound effects on higher-order cognition. For example, recent work by Dale et al. (2009) has demonstrated that when successive speech syllables are presented within auditory noise, the M100 response to the second stimulus is significantly and abnormally “unattenuated” in patients with schizophrenia. This lack of M100 attenuation was correlated with poor task accuracy and low global cognition scores (Dale et al., 2009).
Functional interactions between the M50 and the M100 in schizophrenia have also been examined using MEG. In a study by Hanlon et al. (2005), auditory sensory gating was examined using a dual-click experiment during MEG recordings, and hierarchical linear regression was used to model coupling between sensory gating in the M50 and M100 sources. In the patient group, a left lateralized reduction in M50 sensory gating and bilateral reduction in M100 sensory gating suggested that unrestricted, robust signal at 100 ms was the net result of failure in gating information from an early response (Figure 1). Impaired sensory gating represented in the M100 response was associated with a gating deficit in the M50 from source localizations over left auditory cortex, indicating that a failure to properly filter incoming signals through the M50 affected downstream processing in the M100. This relationship between M50 and M100 sensory gating for right hemisphere sources was not significant (Hanlon et al., 2005). Developed connections between non-essential cortical fields can interfere with sensory gating and auditory processing. In concurrent EEG/MEG recordings, while no significant relationship has been identified between a EEG P50 component and symptom ratings, a failure of sensory gating of the right M50 component (but not the left) has been shown to be positively correlated with negative symptom scores (Thoma et al., 2005). This is in contrast to a relationship between impaired sensory gating in the left hemisphere, which is correlated with attention and working memory (Thoma et al., 2003). Although these lateralization effects are still under investigation, they are consistent with models of compromised fronto-temporal function in schizophrenia.
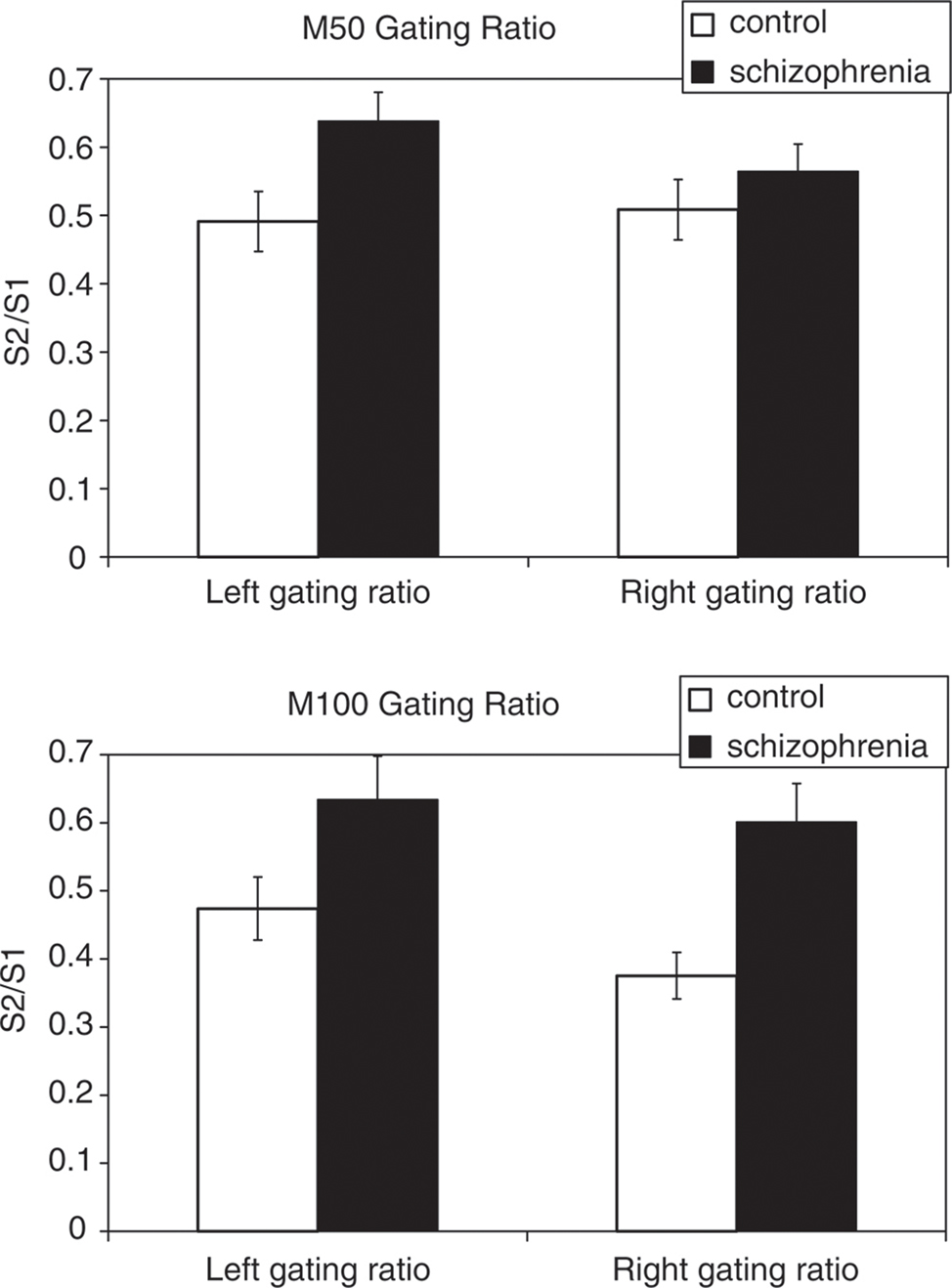
Figure 1. Auditory sensory gating responses (indexed as a ratio in sensor power between the response to the second (S2) and first (S1) auditory click) in both patients with schizophrenia and controls. Auditory gating ratios at both 50 ms (M50) and 100 ms (M100) following stimulus presentation are significantly greater in the patient group in the left hemisphere. In the right hemisphere, reduced sensory gating in the patient group was only significant for a M100 response. Error bars = SE mean. Reproduced, with permission, from Hanlon et al. (2005).
The extent to which this impairment in sensory gating generalizes across sensory modalities in schizophrenia is presently unclear. Responses to tactile stimuli in primary somatosensory cortex (S1) and secondary somatosensory cortex (S2) have also been examined in these patients using MEG. These studies have demonstrated that gating ratios for an early somatosensory evoked response (M20) in S1 are relatively intact in schizophrenia (Edgar et al., 2005; Thoma et al., 2007), while sensory gating of the late response (M80) in S2 is profoundly affected in these patients (Thoma et al., 2007), Although this argues against a broad deficit in cross-model processing in schizophrenia, it is consistent with a general notion of filtering impairments across sensory systems in this condition.
Detection of Salient Events in Schizophrenia: Mismatch Negativity (MMN)
One cortical process extensively studied through MEG is the automatic response that is generated following a deviant stimulus in a train of auditory standards, or the mismatch negativity effect (MMN, or the magnetic equivalent, MMNm). This evoked response, which occurs 150–200 ms following a deviant stimulus, is thought to reflect, in healthy individuals, a pre-attentive process whose purpose is to reorient the individual towards a novel stimulus that has entered the environment (for a review, see Pulvermüller and Shtyrov, 2006). In patients with schizophrenia, the amplitude of the MMNm is generally reduced, across experimental designs and stimulus types (Figure 2, see Kasai et al., 2002; Kircher et al., 2004; Yamasue et al., 2004; Thönnessen et al., 2008). In schizophrenia, this deviation in MMNm is best produced in an optimum design paradigm (Näätänen et al., 2004), where the deviant occurs much more frequently in the stimulus train and is modified along one feature throughout stimulus presentation (either its frequency, intensity, duration, sound source or gap in tone, see Thönnessen et al., 2008). The MMNm is thought to be bilateral for categorical speech sound deviants (Kasai et al., 2002) and low MMNm amplitude may be due to, in part, reduced gray matter volume in the cortex along the planum temporale found in patients with schizophrenia (Yamasue et al., 2004). The demonstration of compromised gray matter density (and thus cortical function) in the temporal lobes of these patients are congruent with work in combined fMRI/MEG studies that examine MMNm in the patient and control group using both neuroimaging modalities (Kircher et al., 2004). In this study, reduced MMNm in patients with schizophrenia correlated with a decrease in BOLD signal in STG, along the planum temporale (secondary auditory cortex) but not in primary auditory cortex, along Heschl’s gyrus (Kircher et al., 2004). Although a difference in MMN amplitude between patient and control groups is readily identifiable in averaged EEG sensor data, the sources that contribute to this deviation are likely to be far more complex than just reduced activity within secondary auditory areas. MMN represents a powerful and well-developed experimental paradigm used to assess auditory processing dysfunction in schizophrenia.
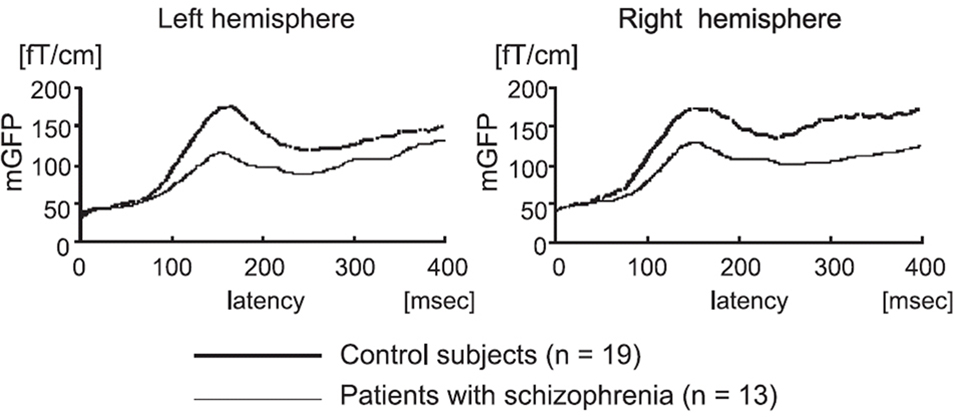
Figure 2. Mismatch negativity (MMN) in healthy control participants and in patients with schizophrenia. Changes in grand magnetic field power (mGFP) over auditory cortex in response to a deviant stimulus (phoneme change) are significantly reduced in patients with schizophrenia bilaterally. Reproduced, with permission, from Yamasue et al. (2004).
A reduced MMN component measured through MEG may eventually prove to be a useful biomarker in determining susceptibility to schizophrenia, as in the case of ultra-high risk (UHR) individuals in the prodromal stage of the disorder. A recent study by Shin et al. (2009) demonstrated that this impairment in MMNm is persistent even before the onset of symptoms in schizophrenia. When compared to control participants, smaller magnetic field changes over auditory cortex in the left hemisphere were found in ultra high-risk subjects. Furthermore, MMNm amplitude was negatively correlated with measures of at-risk mental states (CAARMS) in the UHR group (Figure 3).
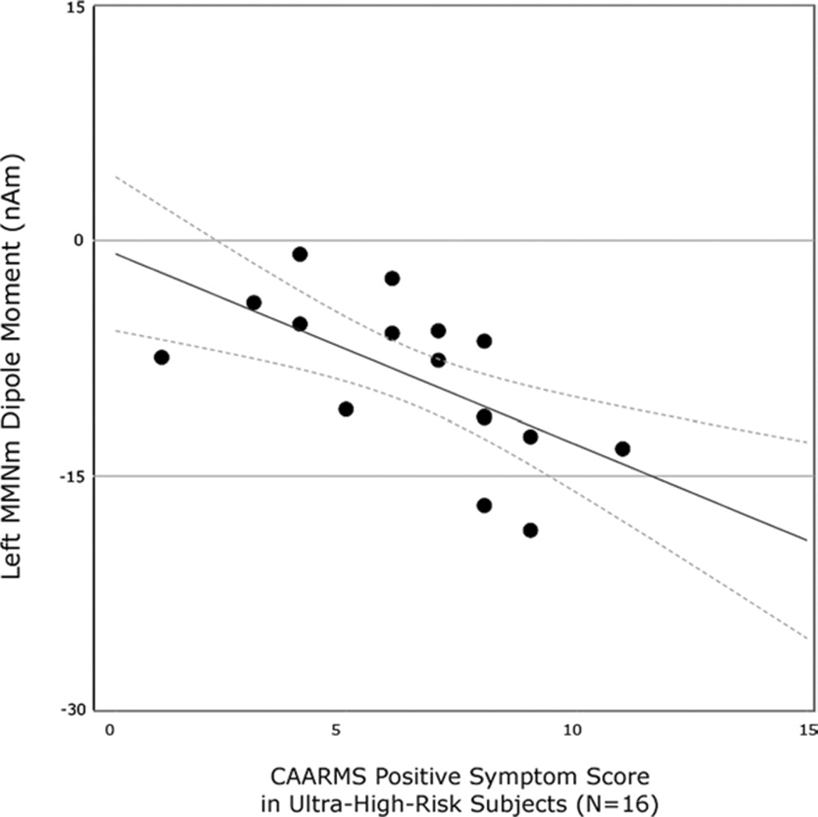
Figure 3. Correlations between MMN measures in patients during the prodromal phase of the disorder with an assessment of ultra-high risk state (CAARMS). A negative relationship is seen between MMN strength and ranking on the CAARMS metric. Reproduced, with permission, from Shin et al. (2009).
Emotional Processing
An inability to extract relevant emotional content from a facial expression in order to guide behavior is thought to be a fundamental component of the broad social cognitive dysfunction found in patients with schizophrenia (Krong and Moran, 2008; Kohler et al., 2009). MEG studies of facial emotion processing have been able to examine the stages of cortical processing that are impaired in these patients (Rockstroh et al., 2006). Healthy individuals were able to discriminate between stimuli that are either emotionally charged or neutral, even when the stimuli were presented at a very high rate of visual stimulation (∼4 Hz; Junghöfer et al., 2003; Peyk et al., 2008). Although patients with schizophrenia are able to make similar judgments of emotional valence, data from MEG sensors during this task suggest that this information is incorporated in an atypical fashion. Following an emotionally arousing visual stimulus, changes in amplitude normally seen in the posterior MEG sensors are markedly reduced in patients (Rockstroh et al., 2006). The timing of this deficient response fell over the range of 90–300 ms following the visual stimulus. While this deviant response did not compromise the patients’ accuracy in detecting emotion, it is possible that it contributes in some as yet unidentified manner to the impaired social abilities seen in schizophrenia.
Spontaneous Cortical Activity
Similar to what has been done in fMRI, EEG and PET, MEG has been used to examine deviations in resting-state cortical activity in patients with schizophrenia. Early investigations of low-frequency oscillations have shown that, in this group, stronger delta and theta (2–6 Hz) power at rest occurred along with a reduction in alpha power in the same sample (Cañive et al., 1998; Sperling et al., 2002). This increase in slow-frequency (<6 Hz) oscillations measured in MEG is likely due to a non-specific increase in the dipole moment amplitude across sensors for delta power, while an increase in theta power might originate from an increase in parietal, temporal and occipital sources (Fehr et al., 2001, 2003). Fluctuations in delta and theta power in MEG have also been shown to be related to positive and negative symptoms assessed in these patients (Fehr et al., 2001, 2003). Sperling et al. (2002) were also able to relate this increase in the 2–6 Hz range at rest with positive symptoms, with a positive correlation between power over temporal and parietal sources and PANSS ratings in patients with paranoid hallucinatory schizophrenia. A tight relationship between symptom measures and neural oscillations, which persist in the absence of behavior, demonstrates the pervasiveness of the neural dysfunction in schizophrenia. These changes in spontaneous cortical activity may or may not be driven by changes in connectivity between brain regions.
Other Cognitive Functions
A number of other classical experimental designs in cognitive psychology have been adapted for use with MEG. For tasks such as mental arithmetic, MEG has been used to demonstrate both reductions in high-gamma sensor power (Kissler et al., 2000) and increases in delta and theta band activity (Fehr et al., 2003) in patients with schizophrenia. Disorganized brain activity (source clustering) recorded in MEG data during a categorical picture-naming task has also been shown to be present in patients with schizophrenia, and this impaired neural activity has been shown to be related to both positive and negative symptoms (Löw et al., 2006). In addition, examination of high-frequency cortical activity in MEG in response to speech stimuli has also been demonstrated to be related to clinical symptom scores. In patients with schizophrenia, the processing of speech sounds in the gamma (25–40 Hz) range over left auditory cortex is delayed, and negatively correlated with the severity of auditory hallucinations (Hirano et al., 2008; Figure 4).
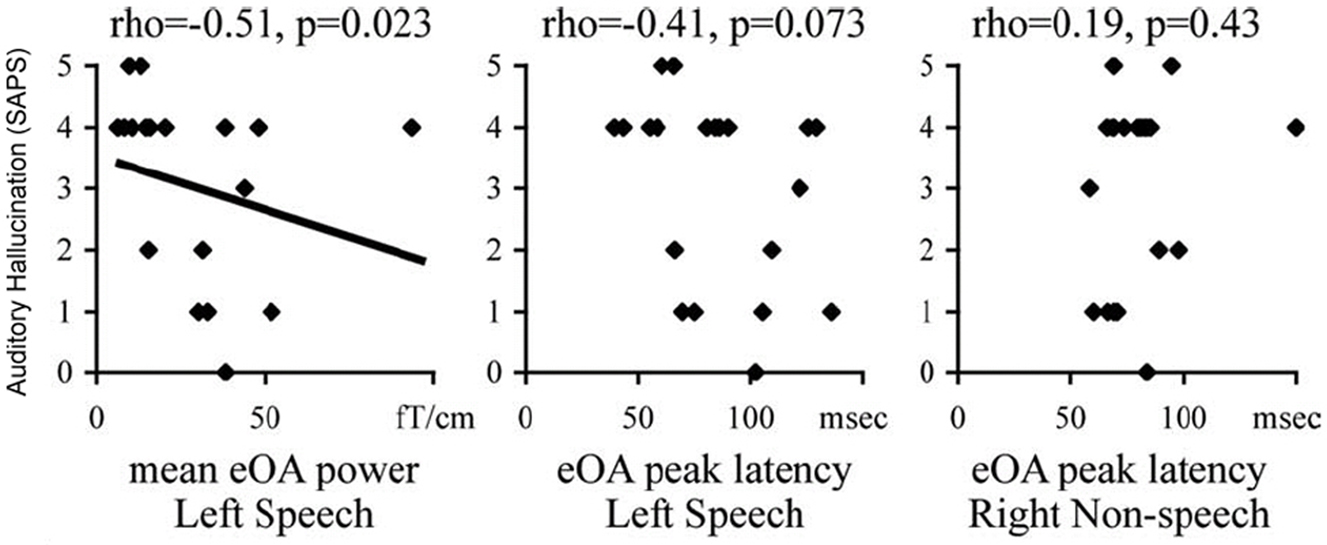
Figure 4. Relationship between evoked oscillatory activity (eOA) in response to speech sounds in patients with schizophrenia to auditory hallucination scores as measured through SAPS. During the period of 100–150 ms following a speech sound, the magnitude of the eOA was negatively correlated with auditory hallucinations in the left hemisphere only. No significant relationship was seen between eOA latency and SAPS scores in either the left or right hemispheres. Reproduced, with permission, from Hirano et al. (2008).
Localizing Segments of Networks Affected in Schizophrenia: Source-Space Reconstructions of MEG Data
Advances in EBI enable reconstruction of underlying brain activity from MEG sensor data. From the spatio-temporal patterns in the sensor data, it is now possible to reconstruct brain activity, also referred to as sources, using sophisticated and powerful algorithms that are also called “source reconstruction algorithms”. An obvious advantage to examining data in source space is that the signals interpreted correspond to specific brain regions. However, the drawback to analyzing data in source space is that these algorithms attempt to solve an ill-posed inverse problem, wherein the number of unknown source locations is greater than the number of sensors. There have been significant advances in source localization algorithms in recent times that overcome several problems in EBI; and correspondingly, MEG studies have become synonymous with EBI.
There are currently a wide variety of source localization algorithms available for estimating source activity. Parametric dipole fitting is a common technique, where a small number of point dipole sources are assumed to generate the MEG data, and the problem reduces to determining parameters such as the location, orientation, and amplitude of these point dipolar sources. However this approach has issues with local minima and can be sensitive to the initialization of the number and location of dipoles (Mosher et al., 1992; Uutela et al., 1998). This initialization bias can be circumvented by automated dipole fit procedures, such as a multistart spatial–temporal downhill simplex algorithm (MSST) (Huang et al., 1998). Also, there are spatial scanning techniques that estimate the time course at every candidate location while suppressing the interference from activity at the other candidate source locations. Candidate locations for the source reconstructions are often constructed from an individual’s MRI and can either occupy the entire volume, be constrained to the gray matter, or only lie on the cortical surface. Some examples of scanning techniques are minimum-variance adaptive beamforming and other variants of beamformers (Sekihara and Nagarajan, 2008), multiple signal classification (Mosher and Leahy, 1998), synthetic aperture morphometry (SAM) (Vrba and Robinson, 2001), dynamic imaging of coherent sources (DICS) (Gross et al., 2001), and source activity using knowledge of event timing for independence from noise and interference (SAKETINI; Zumer et al., 2007). An alternative approach to the scanning methods is to solve for the activity in all candidate source locations simultaneously, also referred to as a tomographic approach. There are a variety of tomographic methods such as minimum-norm estimate (Hämäläinen and Ilmoniemi, 1994), dynamic statistical parametric mapping (Dale et al., 2000), and standardized low resolution brain electromagnetic tomography (Pascual-Marqui, 2002). Some methods promote sparseness in the solution, where the majority of the candidate locations do not have significant activity; empirical evidence shows that a sparse source model can improve the accuracy of the localization in a noisy environment. Some sparse methods include focal underdetermined system solution (Gorodnitsky and Rao, 1997), minimum-current estimate (Uutela et al., 1999), and Champagne (Wipf and Nagarajan, 2009). One way to promote sparsity in the source activity is to use a L-1 norm instead of a L-2 norm in the minimized cost function (Uutela et al., 1999; Wipf et al., 2009), or alternatively use many recently proposed machine learning algorithms for reconstructing sparse sources (Wipf and Nagarajan, 2009; Wipf et al., 2009). While to date a small number of MEG studies in schizophrenia have used EBI techniques, as improvements in EBI continue to advance, it is expected that this number should significantly increase in the coming years.
Cognitive Control
MEG sources have been modeled using adaptive spatial filters in order to reconstruct patterns of brain activity during a cognitive interference task (the Stroop task), in both healthy controls and patients with schizophrenia (Kawaguchi et al., 2005). This approach both localizes changes in the signal and provides information about the changes that occur over time. In healthy individuals, oscillatory activity in the gamma range (25–60 Hz) progressed from parieto-occipital to pre-frontal and primary motor cortex, over the time scale of 150–400 ms post-stimulus and pre-response (Ukai et al., 2002; Kawaguchi et al., 2005). In patients with schizophrenia that suffer from auditory hallucinations, additional activity was seen over right dorsolateral pre-frontal cortex (DLPFC) early on (∼400 ms post-stimulus), a pattern not identified in the patient group without these symptoms (Kawaguchi et al., 2005). This data suggests that dysfunctional Stroop-like inhibition in patients with auditory hallucinations is not the result of aberrant information flow between brain areas in a cognitive control network. Instead, it appears that functionally irrelevant brain regions (such as right DLPFC) interfere with communication between relevant cortical fields.
Working Memory
During auditory working memory (e.g., Sternberg) tasks, a decrease in beta power within the sensors over auditory cortex occurs during the memory retention phase, a neural process known to be impaired (e.g., weak beta decrease) in patients with schizophrenia (Gruzelier et al., 1990; Reite and Rojas, 1997). However, a recent study by Ince et al. (2009) demonstrated that this impairment may extend beyond activity in the beta range. When patients were instructed to memorize a string of letters forming a word, separate patterns of activity in the delta, alpha, beta bands were readily identifiable from a classification of the MEG sensor data. Source-space projections derived from the classifications of the MEG data revealed that these changes in oscillatory power during the task specific to patients localized to superior frontal regions in the delta band, occipital cortex in the alpha range and frontal-temporal areas in the beta band (Ince et al., 2009). This type of categorization was not identified when subjects maintained a non-word in memory, consistent with the notion of language impairments in schizophrenia.
Spontaneous Cortical Activity
Few studies have also reconstructed spontaneous cortical activity in source space from MEG sensor data. One particular focus has been on high-gamma activity during rest. In patients with schizophrenia, power at the MEG sensor level in the high gamma range (60–71 Hz) was found to be reduced at rest when compared to healthy controls (Kissler et al., 2000). A more recent study by Rutter et al. (2009) found that reconstructing data in source space using SAM provides some information about the origin of these changes in gamma power. The greatest reduction in power in the patients was in the 30–80 Hz range, and this reduction in gamma power was localized to medial parietal cortex (Rutter et al., 2009). Although this study did not use connectivity measures per se, the decrease in gamma power overlapped a region of the pre-cuneus known in functional MRI studies to be involved in introspective thought and also known to be functionally underconnected in patients with schizophrenia (Bluhm et al., 2007).
Exploring Aberrant Functional Connectivity in Schizophrenia Across Multiple Sources Through MEG
Ultimately, our understanding of neural activity and behavioral performance in schizophrenia is dependent not only on activity within an area during an active or inactive state, but also on how the brain integrates information across multiple sources. Although a common approach is to examine functional connectivity by using hemodynamic measures of brain activity (such as fMRI), MEG directly measures changes in the magnetic field induced by underlying neuronal currents, and is better suited for modeling these types of interactions. Decomposition of information across, space, time, and oscillatory domains yields complex information about how sources in the brain interact across many levels.
Ioannides et al. (2004) used MEG to examine functional connectivity in schizophrenia during emotional valence judgments in order to explore which segments of the cortical network are dysfunctional in the disorder. Patients were instructed to make decisions regarding the emotional content of the face (happy or sad) presented to the participant during MEG recordings. Interactions between brain sources were assessed using mutual information, a functional connectivity metric that evaluates the extent to which two cortical sources share a common time-series. Robust interactions between inferior frontal cortex, the fusiform gyrus (FG), primary and secondary visual cortex (V1/V2), and the amygdala were identified through functional connectivity analysis in healthy control subjects (Figure 5A). A markedly different pattern is seen in patients with schizophrenia, with reduced interactions between functionally critical brain regions during this task (Figure 5B).
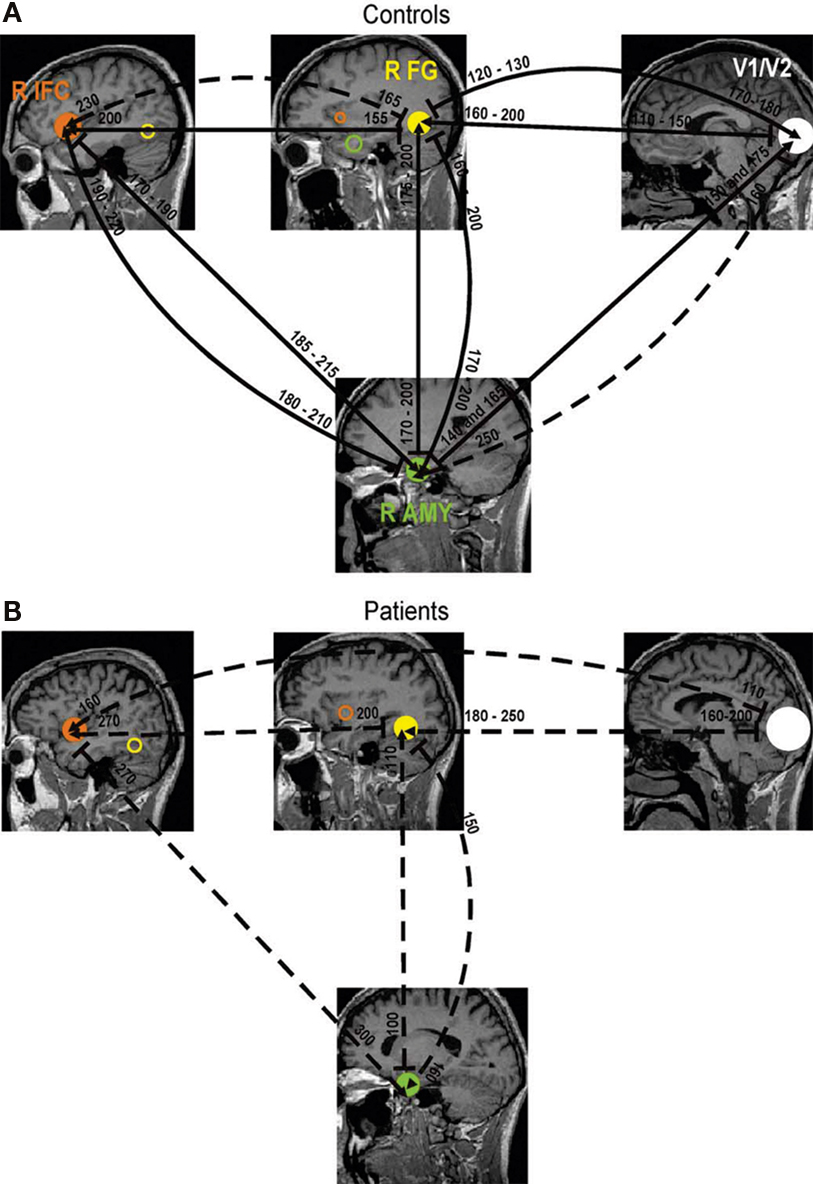
Figure 5. Functional connections between brain regions active during an emotional processing task estimated through MEG. Robust influence amongst time courses (mutual information) can be derived between the right inferior frontal gyrus (IFG), FG, visual cortex (V1/V2) and amygdala (AMY) in healthy controls (A). Coupling between these regions become more isolated in patients with schizophrenia, with reductions in the relatedness between two sources (dashed lines) as well as deviations in activation onset and latency (B). Reproduced, with permission, from Ioannides et al. (2004).
Using MEG resting-state recordings, Georgopoulos et al. (2007) were able to discriminate not only between healthy controls and patients with schizophrenia, but also between patients and subjects with other clinical diagnoses. Data was acquired with the eyes open for a short period of time (between 45 and 60 s) and functional connectivity was estimated by entering residuals (derived from an autoregressive moving average model) into a partial cross-correlation (PCC) analysis between all sensor pairs. In order to characterize a resting-state connectivity pattern distinct for patients with schizophrenia, PCC values were entered into a linear classifier, which separated connectivity patterns between subject groups. The network of connections measured by MEG at rest in the patient group was found to be non-overlapping with categorized activity of healthy controls (Figure 6). Similarly, these resting-state networks were distinct from patterns of connectivity in the other patient groups, including patients with Alzheimer’s disease and Multiple sclerosis (Georgopoulos et al., 2007). Since no a priori network of connections were defined in this model, it illustrates that coherent, spontaneous activity recorded in MEG, even at the sensor level, can be used to separate clinical conditions like schizophrenia from unaffected populations.
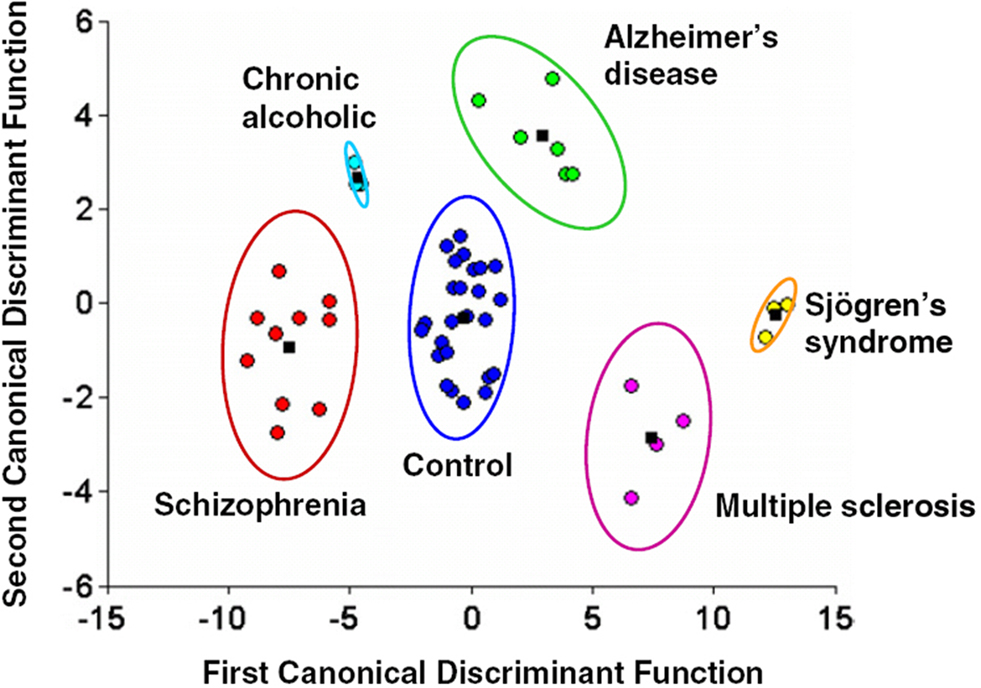
Figure 6. Results from a linear discriminant classification analysis applied to MEG resting-state functional connectivity (partial cross-correlation) data across multiple groups, including both controls and patients with schizophrenia. Even from MEG recordings when no task is being performed, the classifier was able to discriminate between the different populations, with no overlap seen between the control and schizophrenia groups. These differences suggest that resting-state functional connectivity derived from MEG data can be a robust indicator of clinical diagnosis. Reproduced, with permission, from Georgopoulos et al. (2007).
Novel Metrics for Estimating Functional Connectivity in MEG
Despite the advantage of MEG (and EEG) in the temporal domain over fMRI, there have been relatively few publications that assess event-related or resting-state functional connectivity using MEG or EEG as compared to fMRI. There are two genres of metrics used in MEG functional connectivity: bivariate quantities are calculated in a pair-wise fashion between pairs of voxels and multivariate techniques model the interactions between several regions of interest. Likewise, functional connectivity metrics in MEG data analyses can be applied either in sensor-space or in source-space. Although many metrics have been proposed for functional connectivity in MEG, no careful comparisons have been made for the same dataset across bivariate and multivariate metrics. Here we provide a brief review of both bivariate and multivariate metrics applied to EEG and MEG data in source space and sensor space, as these techniques have the potential to reveal neural interactions compromised in schizophrenia.
Bivariate Metrics of Functional Connectivity in MEG
Bivariate metrics can be applied to MEG/EEG data in two ways. Since these metrics are computed between two time courses, they can either be computed between target sensors/voxels or they can be computed between all sensors/voxels and then an average connectivity value can be calculated for every sensor/voxel. The first of these methods is used when there is knowledge about the areas involved and can be considered a “hypothesis-driven” approach. The second, in contrast, can be described as a “data-driven” approach and is applicable when there is not a priori knowledge about which areas should exhibit high or changed connectivity. Correlation and its frequency domain analog, coherence, are the two most commonly used bivariate metrics in the literature (see Srinivasan et al., 2007). An extension of using coherence on sensor time-courses, a source localization algorithm called DICS, is particularly designed to construct coherent activity by estimating time-courses and calculating magnitude coherence (Gross et al., 2001). There are also phase difference-based bivariate metrics that can be applied in similar fashion to the metrics described above. The difference in instantaneous phase between two time courses can be calculated using the Hilbert transform. There are different subsequent calculations that can be performed with the phase difference, e.g. phase coherence (PC), phase synchronization, index of synchronization (for examples, see Nikouline et al., 2001; Schoonhoven et al., 2003; Hadjipapas et al., 2005).
All types of bivariate metrics are susceptible to spurious interactions that arise from volume conduction artifacts in MEG and EEG recordings. The magnetic field or electric potential generated by a single neuronal source is picked up by not only the nearest sensor to the source, but the neighboring sensors also pick up the signal with a zero-time lag. This creates instantaneous blurring across the sensors. As such, the time-courses of many sensors can contain overlapping information due to this electromagnetic phenomenon, which can produce spurious interactions. Some bivariate metrics used for MEG and EEG functional connectivity analyses have been designed to overcome this blurring by isolating the non-zero-time-lag interactions from the zero-time-lag interactions, namely imaginary coherence (IC) and phase lag index (PLI). Both metrics are designed to assess only non-zero time lagged interactions in source or sensor data in order to cancel out the effects of cross-talk across the detection sensors.
Imaginary coherence is calculated by only considering the imaginary component of the complex-valued coherence. The imaginary part of the coherence is produced by non-instantaneous interactions between waveforms. It was found to be a better measure of coupling than the magnitude of coherence in an EEG experiment of voluntary finger movement (Nolte et al., 2004). PLI is similar to IC in that it includes only information that is transmitted at a non-zero time lag; any two signals that are instantaneously coupled and therefore have a phase difference of zero, are not included in the calculation of PLI. In a study by Stam et al. (2007), PLI and PC of EEG and MEG data were more sensitive than IC to increasing levels of true synchronization in the simulated data, but IC and PLI were less susceptible to spurious correlations in the data due to common sources. In addition, PLI and IC were better able to detect beta band connectivity and uncovered a different spatial pattern of connectivity in the MEG data. IC has also revealed significant changes in the over all resting-state connectivity induced by brain lesions (Marzetti et al., 2007; Guggisberg et al., 2008).
Multivariate Connectivity Metrics in MEG
In contrast to bivariate metrics, which compute relationships between elements in a pair-wise fashion, multivariate metrics are able to model interactions between multiple areas in a single model (see Astolfi et al., 2005). While powerful, computational complexity is an issue when performing a multivariate analysis. While all areas can be modeled simultaneously, the limitation of these methods lies in maintaining the necessary condition that the number of parameters fit in the model does not exceed the number of time points. This is done by considering fewer areas or voxels or by limiting the number of lags the model will analyze. Multivariate autoregressive models (MVAR) can be applied in the time domain, or in the frequency domain, as is the case with partial directed coherence and direct transfer function methods (for examples, see Schelter et al., 2006; Porcaro et al., 2009). Although some of these methods have been demonstrated to be powerful in determining neural networks associated with basic sensory processing (Porcaro et al., 2009) future studies will determine how these metrics can be extended to examinations of impairments in cognitive function in schizophrenia.
Demonstration: Using IC to Evaluate Functional Connectivity and Cognition in Schizophrenia
Bivariate metrics, such as IC, are powerful enough to detect functionally connected networks from MEG data (Nolte et al., 2004; Guggisberg et al., 2008). We have used IC to examine resting-state functional connectivity in 30 clinically-stable, chronically-ill patients with schizophrenia (as diagnosed through the Structured Clinical Interview for DSM-IV) as well as 15 age, gender, and education matched healthy comparison subjects. All participants were assessed with a standard neurocognitive battery of MATRICS-recommended measures (Nuechterlein and Green, 2006) during a separate visit within a 2-week period prior to MEG scanning. Resting state data (eyes closed) was collected over a 4-min period from patients and controls using a 275-channel CTF Omega 2000 whole-head biomagnetometer (VSM MedTech, Coquitlam, BC, Canada) with a sampling rate of 600 Hz. A single epoch (60 s) of artifact-free data was selected specifically for each subject based on the baseline of the MEG sensor data. Sources of oscillating neural activity in the alpha range (∼8–12 Hz) were estimated using an adaptive spatial filtering technique (Robinson and Vrba, 1999). IC was computed between each pair of voxels in the MEG timeseries, and then all IC values were Fisher’s z-transformed, averaged across all voxels, and spatially normalized to an MNI template (as in Guggisberg et al., 2008). As mentioned in the previous section, this metric (global IC) provides an estimate of functional connectivity at each voxel by averaging across all the connections of that single voxel with the rest of the brain. Given that the characteristics of resting-state MEG data have been shown to be robust indicators of disease state (Georgopoulos et al., 2007), our goal was to evaluate how functional connectivity in both patients with schizophrenia and healthy controls was related to measures of neurocognitive function collected outside of the MEG scan session. Resting-state connectivity maps were correlated with two measures of cognitive function known to be impaired in schizophrenia: a verbal memory task (the Hopkins Verbal Learning Test, HVLT, delayed recall) and a category fluency task. These tasks are known to recruit regions of frontal association cortex involved in language and executive function (Smith et al., 1998; Wager and Smith, 2003; Baldo et al., 2006).
Although performance on HVLT-delayed recall was poorer in the patient group, this difference was not statistically significantly when compared against education-matched controls (p = 0.07). However, HVLT-delayed performance was positively and significantly correlated with IC measures of right dorsolateral pre-frontal cortex (DLPFC; Figure 7) only for patients with schizophrenia (r = 0.51, p < 0.05, false-discovery rate (FDR) corrected for multiple comparisons) and not healthy comparison subjects (r = 0.34, p = 0.21). Such an effect found only in the patient group even when HVLT-delayed scores were not significantly different between the two groups suggests that this relationship between DLPFC connectivity and verbal memory is specific to schizophrenia. Active-state fMRI studies have shown that the functional connections of this region in the right hemisphere, in Brodmann’s Area 9 of the middle frontal gyrus, are reduced in schizophrenia during various cognitive tasks such as movement sequencing and continuous performance (Salgado-Pineda et al., 2007; Woodward et al., 2009).
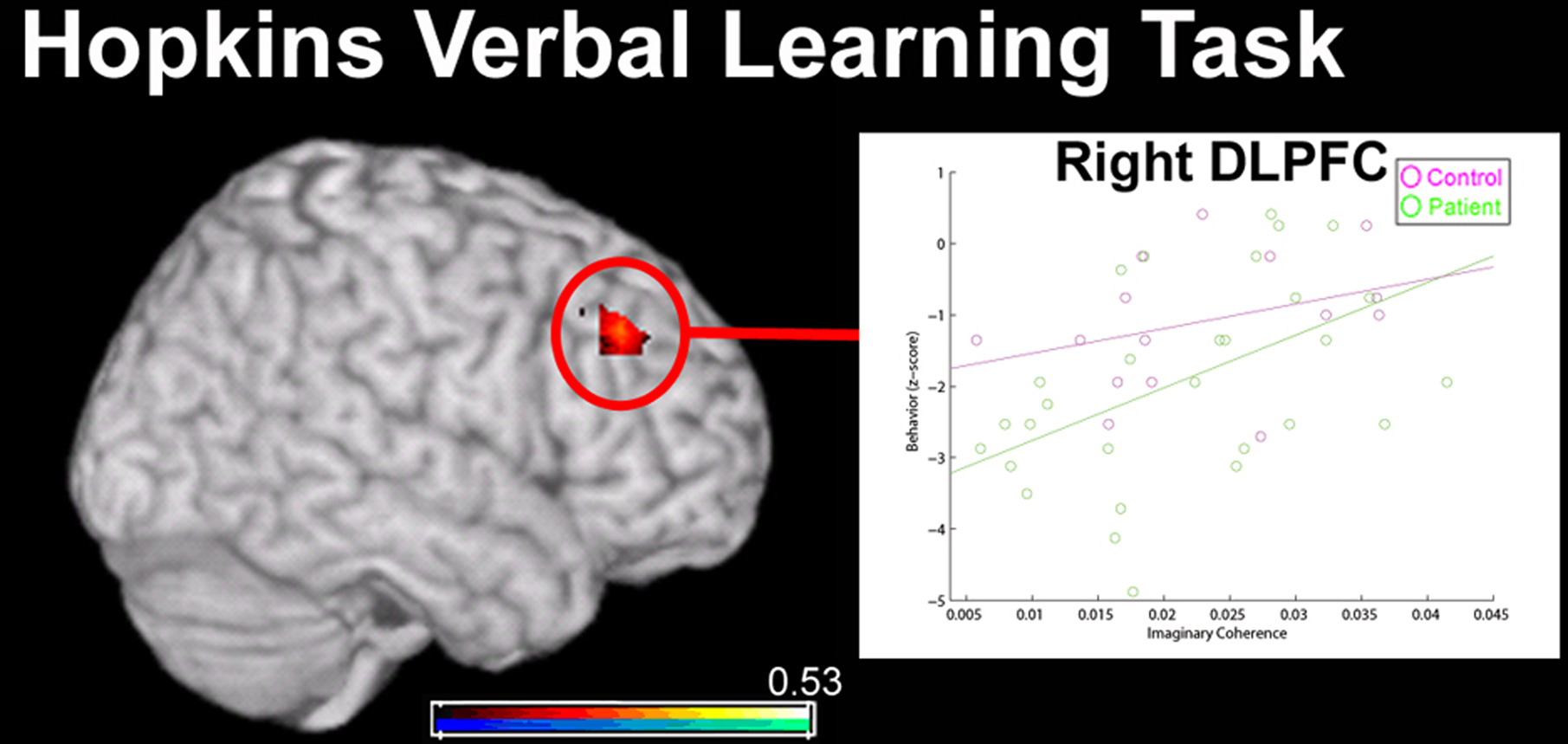
Figure 7. Relationship between MEG resting-state functional connectivity (imaginary coherence) data and performance on the Hopkins Verbal Learning Task (HVLT; delay component) in 15 healthy controls (in pink) and 30 patients with schizophrenia (in green). Global connectivity of a region in right dorsolateral pre-frontal cortex (DLPFC; over the middle frontal gyrus) was positively correlated with HVLT performance (overlay, in red). However, this correlation was only significant for the patient group, indicating that this association between reduced connectivity of right DLPFC and verbal working memory is specific to the disorder. No significant relationship between functional connectivity of any region and performance during immediate recall in the HVLT was identified in either group.
Resting-state functional connectivity scores were also related to performance during a category fluency test, where subjects are required to verbally produce words in a specified category. As in the case of HVLT-delay scores, patient performance was not significantly different from controls (p = 0.19). A region of the medial parietal lobe and left DLPFC were positively correlated with performance on this task (Figure 8). Category fluency performance was positively correlated with global connectivity of the left medial frontal gyrus, in Brodmann’s Area 8 (BA8), across both groups (p < 0.1, FDR corrected); a similar trend was seen for the connectivity of the precuneus (Prec; Figure 8) only for control subjects (r2 = 0.64, p < 0.1 FDR corrected). These data suggest that while BA8 connectivity is related to verbal fluency across groups, an interaction between precuneus connectivity and performance present in controls is absent in patients with schizophrenia. In a recent fMRI study by Schlösser et al. (2009), where patients with schizophrenia are trained to overlearn verbal material, an increase in functional connectivity between left DLPFC and other regions (including right DLPFC, parietal cortex and the cerebellum) were associated with verbal item acquisition. These areas are also known to be underactive in patients during fMRI studies when the task is cognitively demanding (such as the n-back task; Pomarol-Clotet et al., 2008).
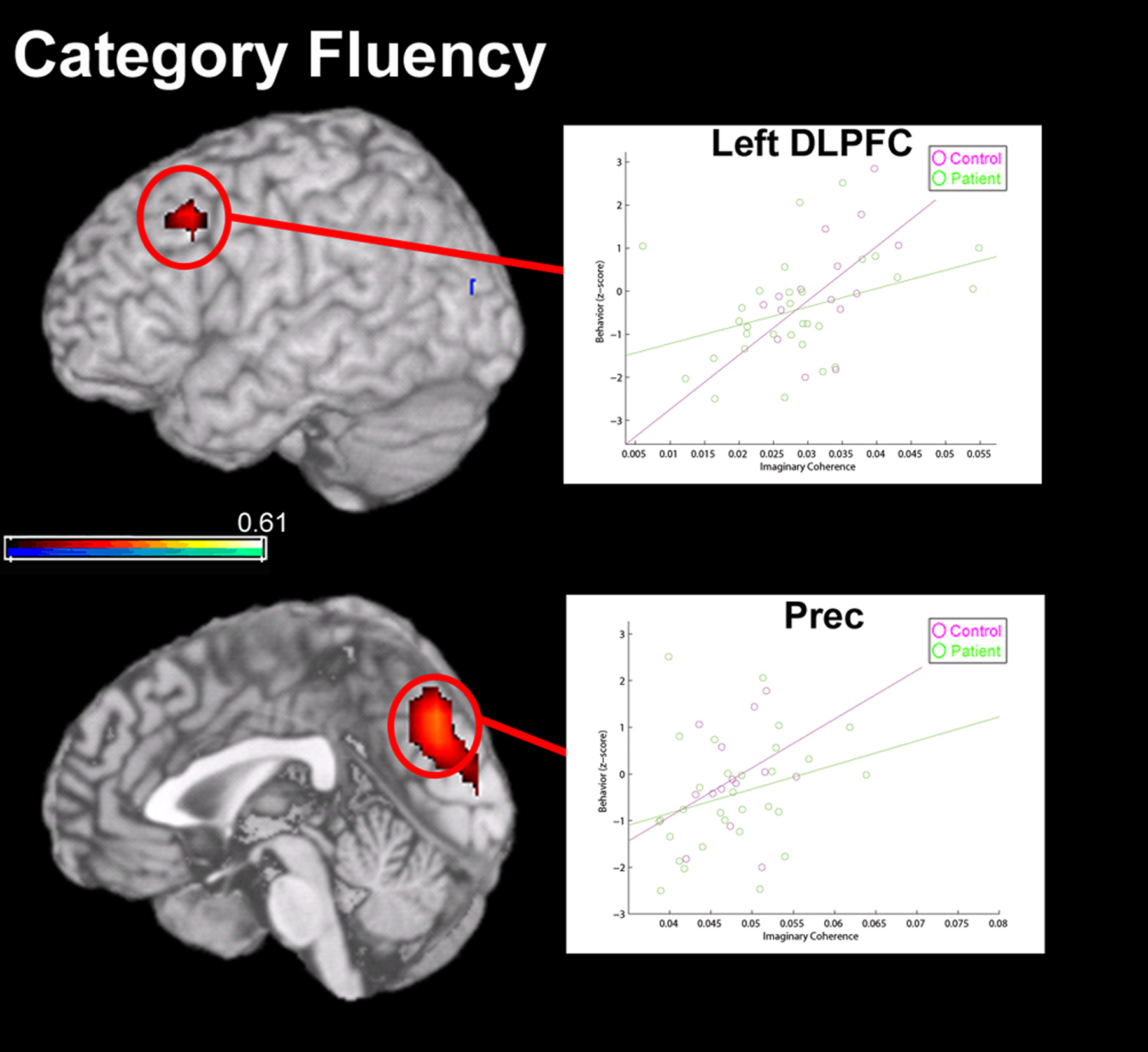
Figure 8. Correlation between global functional connectivity (imaginary coherence) of MEG data at rest with performance on a measure of verbal (Category) fluency. Functional connectivity of two regions were positively correlated (overlay, in red) with performance on this task, including left dorsolateral pre-frontal cortex (DLPFC) and a region of medial parietal cortex, in the precuneus (PreC). In left DLPFC, strong global connectivity was correlated with good performance in both patients and controls. However, in the precuneus, a relationship between global connectivity and Category fluency performance was only significant for the healthy control group.
Conclusion and Future Directions
There is a developing effort in the literature to relate the complexity of early processing in sensory regions to cognitive dysfunction in schizophrenia (Gilbert and Sigman, 2007; Javitt, 2009). Given that early-level sensory processing deficits in schizophrenia (for example, auditory pitch perception or function within the magnocellular pathway in vision) affect “top-down” functions such as emotional discrimination (Leitman et al., 2005; Butler et al., 2009) it is likely that these sensory responses impact higher-level cognitive processing in the disorder. Neuroplasticity-based cognitive training approaches that target auditory processing efficiency have been shown to improve higher-order verbal cognition function and to remediate neurophysiological deficits recorded in MEG (such as M100 attenuation) in patients with schizophrenia (Adcock et al., 2009). Future work using MEG to examine interactions between brain regions – as well as neuroplastic responses in such interactions as a function of cognitive-enhancing interventions – will yield highly useful information for the design of innovative treatments.
There is a considerable degree of cognitive, behavioral and interpersonal complexity to a condition like schizophrenia; therefore, it is reasonable to assume that the neurophysiological framework of the disorder is comprised of functional alterations at multiple levels. MEG is able to interrogate a time scale of neural processing inaccessible to other imaging modalities, and thus serves as a complementary tool for understanding function and connectivity within neural networks in schizophrenia. Promising applications of MEG connectivity in the treatment of the illness are twofold: (1) as a means of characterizing an endophenotype that may predict clinical outcome or response to treatment in prodromal and genetically high-risk patients; and (2) as a means of monitoring changes in brain activity over the course of treatment (see Scherk and Falkai, 2006; Whalley, et al., 2009). It is also possible to capitalize on the spatial and temporal resolution of MEG by combining this data with connectivity information derived from fMRI and DTI (Dale and Halgren, 2001; Stufflebeam et al., 2008; Freeman et al., 2009; Zumer et al., 2009). Ultimately, the goal of this work will be to deepen our understanding of pathophysiology, of effective treatment, and of prevention in this devastating illness.
Conflict of Interest Statement
The authors declare that the research was conducted in the absence of any commercial or financial relationships that could be construed as a potential conflict of interest.
Acknowledgments
We wish to thank Christine Holland, Alex Genevsky, Mary Vertinski, Susanne Honma and Colleen Cook. Grants: This work was supported by the San Francisco VA Medical Center and by NIMH RO1 Grant MH068725-01A1 awarded to SV, NIH R01 Grants R01 DC004855 and DC006435 awarded to SSN and a Dystonia Medical Research Foundation fellowship awarded to LBNH. Its contents are solely the responsibility of the authors and do not necessarily represent the official views of the National Institute of Health.
References
Adcock, R. A., Dale, C., Fisher, M., Aldebot, S., Genevsky, A., Simpson, G. V., Nagarajan, S., and Vinogradov, S. (2009). When top-down meets bottom-up: auditory training enhances verbal memory in schizophrenia. Schizophr. Bull. 35, 1132–1141.
Adler, L., Pachtman, E., Franks, R., Pecevich, M., Waldo, M., and Freedman, R. (1982). Neurophysiological evidence for a defect in neuronal mechanisms involved in sensory gating in schizophrenia. Biol. Psychiatry 17, 639–654.
Ardekani, B. A., Nierenberg, J., Hoptman, M. J., Javitt, D. C., and Lim, K. O. (2003). MRI study of white matter diffusion anisotrophy in schizophrenia. Neuroreport 14, 2025–2029.
Astolfi, L., Cincotti, F., Mattia, D., de Vico Falliani, F., Lai, M., Baccala, L., Salinari, S., Ursino, M., Zavaglia, M., and Bablioni, F. (2005). Comparison of different multivariate methods for the estimation of cortical connectivity: simulations and applications to EEG data. Conf. Proc. IEEE Eng. Med. Biol. Soc. 5, 4484–4487.
Baldo, J. V., Schwartz, S., Wilkins, D., and Dronkers, N. F. (2006). Role of frontal versus temporal cortex in verbal fluency as revealed by voxel-based lesion symptom mapping. J. Int. Neuropsychol. Soc. 12, 896–900.
Bluhm, R. L., Miller, J., Lanius, R. A., Osuch, E. A., Boksman, K. Neufeld, R.W.J., Theberge, J., Schaefer, B., and Williamson, P. (2007). Spontaneous low-frequency fluctuations in the BOLD signal in schizophrenic patients: anamolies in the default network. Schizophr. Bull. 33, 1004–1012.
Burns, J., Job, D., Bastin, M. E., Whalley, H., Macgillivray, T., Johnstone, E. C., and Lawrie, S. M. (2003). Structural disconnectivity in schizophrenia: a diffusion tensor magnetic resonance imaging study. Br. J. Psychiatry 182, 439–443.
Butler, P. D., Abeles, I. Y., Weiskopf, N. G., Tambini, A., Jalbrzikowski, M., Legatt, M. E., Zemon, V., Loughead, J., Gur, R. C., and Javitt, D. C. (2009). Sensory contributions to impaired emotion processing in schizophrenia. Schizophr. Bull. 35, 1095–1107.
Cañive, J. M., Lewine, J. D., Edgar, J. C., Davis, J. T., Miller, G. A., Torres, F., and Tuason, V. B. (1998). Spontaneous brain magnetic activity in schizophrenic patients treated with aripiprazole. Psychopharmacol. Bull. 34, 101–105.
Clementz, B. A., Blumenfeld, L. D., and Cobb, S. (1997). The gamma band response may account for poor P50 suppression in schizophrenia. Neuroreport 8, 3889–3893.
Dalal, S. S., Guggisberg, A. G., Edwards, E., Sekihara, K., Findlay, A. M., Canolty, R. T., Berger, M. S., Knight, R. T., Barbaro, N. M., Kirsch, H. E., and Nagarajan, S. S. (2008). Five-dimensional neuroimaging: localization of the time-frequency dynamics of cortical activity. Neuroimage 40, 1686–1700.
Dale, C. L., Findlay, A. M., Adcock, R. A., Vertinsky, M., Fisher, M., Genevsky, A., Aldebot, S., Subramaniam, K., Luks, T. L., Simpson, G. V., Nagarajan, S. S., and Vinogradov, S. (2009). Timing is everything: neural response dynamics during syllable processing and its relation to higher-order cognition in schizophrenia and healthy comparison subjects. Int. J. Psychophysiol. 28 [Epub ahead of print].
Dale, A. M., and Halgren, E. (2001). Spatiotemporal mapping of brain activity by integration of multiple imaging modalities. Curr. Opin. Neurobiol. 11, 202–208.
Dale, A. M., Liu, A. K., Fischl, B. R., Buckner, R. L., Belliveau, J. W., Lewine, J. D., and Halgren, E. (2000). Dynamic statistical parametric mapping: combining fMRI and MEG for high-resolution imaging of cortical activity. Neuron 26, 55–67.
Dwork, A. J., Mancevski, B., and Rosoklija, G. (2007). White matter and cognitive function in schizophrenia. Int. J. Neuropsychopharmacol. 10, 513–536.
Edgar, J. C., Miller, G. A., Moses, S. N., Thoma, R. J., Huang, M. X., Hanlon, F. M., Weisen, M. P., Sherwood, A., Bustillo, J., Adler, L. E., and Cañive, J. M. (2005). Cross-modal generality of the gating deficit. Psychophysiology 42, 318–327.
Fehr, T., Kissler, J., Elbert, T., Wienbruch, C., Moratti, S., and Rockstroh, B. (2001). Source distribution of neuromagnetic focal slow waves and MEG-delta activity in schizophrenic patients. Biol. Psychiatry 50, 108–116.
Fehr, T., Kissler, J., Wienbruch, C., Moratti, S., Elbert, T., Watzl, H., and Rockstroh, B. (2003). Source distribution of neuromagnetic slow-wave activity in schizophrenic patients – effects of activation. Schizophr. Res. 63, 63–71.
Ford, M. R., Goethe, J. W., and Dekker, D. K. (1986). EEG coherence and power in the discrimination of psychiatric disorders and medication effects. Biol. Psychiatry 21, 1175–1188.
Ford, J. M., Mathalon, D. H., Whitfield, S., Faustman, W. O., and Roth, W. T. (2002). Reduced communication between frontal and temporal lobes during talking in schizophrenia. Biol. Psychiatry 51, 484–492.
Freeman, W. J., Ahlfors, S. P., and Menon, V. (2009). Combining fMRI with EEG and MEG in order to relate patterns of brain activity to cognition. Int. J. Psychophysiol. 73, 43–52.
Friston, K. J. (1994). Functional and effective connectivity in neuroimaging: a synthesis. Hum. Brain Mapp. 2, 56–78.
Friston, K. J., and Frith, C. D. (1995). Schizophrenia: a disconnection syndrome? Clin. Neurosci. 3, 89–97.
Georgopoulos, A. P., Karageorgiou, E., Leuthold, A. C., Lewis, S. M., Lynch, J. K., Alonso, A. A., Aslam, Z., Carpenter, A. F., Georgopoulos, A., Hemmy, L. S., Koutlas, I. G., Langheim, F. J. P., McCarten, J. R., McPherson, S. E., Pardo, J. V., Pardo, P. J., Parry, G. J., Rottunda, S. J., Segal, B. M., Sponheim, S. R., Stanwyck, J. J., Stephane, M., and Westermeyer, J. J. (2007). Synchronous neural interactions assessed by magnetoencephalography: a functional biomarker for brain disorders. J. Neural Eng. 4, 349–355.
Gilbert, C. D., and Sigman, M. (2007). Brain states: top-down influences in sensory processing. Neuron 45, 677–696.
Gold, J. M., and Weinberger, D. R. (1995). Cognitive deficits and the neurobiology of schizophrenia. Curr. Opin. Neurobiol. 5, 225–230.
Gorodnitsky, I., and Rao, B. D. (1997). Sparse signal reconstruction from limited data using FOCUSS: a re-weighted minimum norm algorithm. IEEE Trans Signal Processing 45, 600–616.
Grecius, M. (2008). Resting-state functional connectivity in neuropsychiatric disorders. Curr. Opin. Neurol. 21, 424–430.
Gross, J., Kujala, J., Hamalainen, M., Timmermann, L., Schnitzler, A., and Salmelin, R. (2001). Dynamic imaging of coherent sources: studying neural interactions in the human brain. Proc. Natl. Acad. Sci. U.S.A. 98, 694–699.
Gruzelier, J., Liddiard, D., Davis, L., and Wilson, L. (1990). Topographical EEG differences between schizophrenic patients and controls during neuropsychological functional activation. Int. J. Psychophysiol. 8, 275–282.
Guggisberg, A. G., Honma, S. M., Findlay, A. M., Dalal, S. S., Kirsch, H. E., Berger, M. S., and Nagarajan, S. S. (2008). Mapping functional connectivity in patients with brain lesions. Ann. Neurol. 63, 193–203.
Hadjipapas, A., Hillebrand, A., Hilliday, I. E., Singh, K. D., and Barnes, G. R. (2005). Assessing interactions of linear and nonlinear neuronal sources using MEG beamformers: a proof of concept. Clin. Neurophysiol. 116, 1300–1313.
Hämäläinen, M. S., and Ilmoniemi, R. J. (1994). Interpreting magnetic fields of the brain: minimum norm estimates. Med. Biol. Eng. Comput. 32, 35–42.
Hanlon, F. M., Miller, G. A., Thoma, R. J., Irwin, J., Jones, A., Moses, S. N., Huang, M., Weisend, M. P., Paulson, K. M., Edgar, J. C., Adler, L. E., and Cañive, J. M. (2005). Distinct M50 and M100 auditory gating deficits in schizophrenia. Psychophysiology 42, 417–427.
Hirano, S., Hirano, Y., Maekawa, T., Obayashi, C., Oribe, N., Kuroki, T., Kanba, S., and Onitsuka, T. (2008). Abnormal neural oscillatory activity to speech sounds in schizophrenia: a magnetoencephalography study. J. Neurosci. 28, 4987–4903.
Huang, M., Aine, C. J., Supek, S., Best, E., Ranken, D., and Flynn, E. R. (1998). Multi-start downhill simplex method for spatio-temporal source localization in magnetoencephalography. Electroencephalogr. Clin. Neurophysiol. 108, 32–44.
Huang, M. X., Edgar, J. C., Thoma, R. J., Hanlon, F. M., Moses, S. N., Lee, R. R., Paulson, K. M., Weisend, M. P., Irwin, J. G., Bustillo, J. R., Adler, L. E., Miller, G. A., and Cañive, J. M. (2003). Predicting EEG responses using Meg sources in superior temporal gyrus reveals source asynchrony in patients with schizophrenia. Clin. Neurophysiol. 114, 835–850.
Ince, N. F., Pellizzer, G., Tewfik, A. H., Nelson, K., Leuthold, A., McClannahan, K., and Stephane, M. (2009). Classification of schizophrenia with spectro-temporo-spatial MEG patterns in working memory. Clin. Neurophysiol. 120, 1123–1134.
Ioannides, A. A., Poghosyan, V., Dammers, J., and Streit, M. (2004). Real-time neural activity and connectivity in healthy individuals and schizophrenia patients. Neuroimage 23, 473–482.
Javitt, D. C. (2009). Sensory processing in schizophrenia: neither simple nor intact. Schizophr. Bull. 35, 1059–1064.
Junghöfer, M., Schauer, M., Neuner, F., Odenwald, M., Rockstroh, B., and Elbert, T. (2003). Enhanced fear-network in torture survivors activated by RSVP of aversive material can be monitored by magnetic source imaging. Psychophysiology 40, S51.
Kasai, K., Yamada, H., Kamio, S., Nakagome, K., Iwanami, A., Fukuda, M., Yumoto, M., Itoh, K., Koshida, I., Abe, O., and Kato, N. (2002). Neuromagnetic correlates of impaired automatic categorical perception of speech sounds in schizophrenia. Schizophr. Res. 59, 159–172.
Kawaguchi, S., Ukai, S., Shinosaki, K., Ishii, R., Yamamoto, M., Ogawa, A., Mizuno-Matsumoto, Y., Fujita, N., Yoshimine, T., and Takeda, M. (2005). Information processing flow and neural activations in the dorsolateral prefrontal cortex in the Stroop task in schizophrenic patients. Neuropsychobiology 51, 191–203.
Kircher, T. T. J., Rapp, A., Grodd, W., Buchkremer, G., Weiskopf, N., Lutzenberger, W., Ackermann, H., and Mathiak, K. (2004). Mismatch negativity responses in schizophrenia: a combined fMRI and whole-head MEG study. Am. J. Psychiatry 161, 294–304.
Kissler, J., Müller, M. M., Fehr, T., Rockstroh, B., and Elbert, T. (2000). MEG gamma band activity in schizophrenia patients and healthy subjects in a mental arithmetic task and at rest. Clin. Neurophysiol. 111, 2079–2087.
Kohler, C. G., Walker, J. B., Martin, E. A., Healey, K. M., and Modberg, P. J. (2009). Facial emotion perception in schizophrenia: a meta-analytic review. Schizophr. Bull. 27 [Epub ahead of print].
Krong, A. M., and Moran, E. K. (2008). Emotional response deficits in schizophrenia: insights from affective science. Schizophr. Bull. 34, 819–834.
Kubicki, M., Westin, C. F., McCarley, R. W., and Shenton, M. E. (2005). The application of DTI to investigate white matter abnormalities in schizophrenia. Ann. N. Y. Acad. Sci. 1064, 134–148.
Kumra, S., Ashtari, M., McMenimam, M., Vogel, J., Augustin, R., Becker, D. E., Nakayama, E., Gyato, K., Kane, J. M., Lim, K., and Szeszko, P. (2004). Reduced frontal white matter integrity in early-onset schizophrenia: a preliminary study. Biol. Psychiatry 55, 1138–1145.
Kyriakopoulos, M., Bargiotas, T., Barker, G. J., and Frangou, S. (2008). Diffusion tensor imaging in schizophrenia. Eur. Psychiatry 23, 255–273.
Leahy, R. M., Mosher, J. C., Spencer, M. E., Huang, M. X., and Lewine, J. D. (1998). A study of dipole localization accuracy for MEG and EEG using a human skull phantom. Electroencephalogr. Clin. Neurophysiol. 107, 159–173.
Leitman, D. I., Foxe, J. J., Butler, P. D., Saperstein, A., Revheim, N., and Javitt, D. C. (2005). Sensory contributions to impaired prosodic processing in schizophrenia. Biol. Psychiatry 58, 56–61.
Leocani, L., and Comi, G. (1999). EEG coherence in pathological conditions. J. Clin. Neurophysiol. 16, 548–555.
Löw, A., Rockstroh, B., Elbert, T., Silberman, Y., and Bentin, S. (2006). Disordered semantic representation in schizophrenic temporal cortex revealed by neuromagnetic response patterns. BMC Psychiatry 6, 23–30.
Marzetti, L., Della Penna, S., Nolte, G., Franciotti, R., Stefanics, G., and Romani, G. L. (2007). A cartesian time-frequency approach to reveal brain interaction dynamics. Brain Topogr. 19, 147–154.
Merrin, E. L., and Floyd, T. C. (1992). Negative symptoms and EEG alpha activity in schizophrenic patients. Schizophr. Res. 8, 11–20.
Merrin, E. L., Floyd, T. C., and Fein, G. (1989). EEG coherence in unmedicated schizophrenic patients. Biol. Psychiatry 25, 60–66.
Mosher, J. C., and Leahy, R. M. (1998). Recursive MUSIC: a framework for EEG and MEG source localization. IEEE Trans. Biomed. Eng. 45, 1342–1354.
Mosher, J. C., Leahy, R. M., and Lewis, P. (1992). Multiple dipole modeling and localization from spatiotemporal MEG data. IEEE Trans. Biomed. Eng. 39, 541–557.
Näätänen, R., and Kähkönen, S. (2009). Central auditory dysfunction in schizophrenia as revealed by the mismatch negativity (MMN) and its magnetic equivalent MMNm: a review. Int. J. Neuropsychopharmacol. 12, 125–135.
Näätänen, R., Pakarinen, S., Rinne, T., and Takegata, R. (2004). The mismatch negativity (MMN) – towards the optimal paradigm. Clin. Neurophsyiol. 115, 140–144.
Nikouline, V. V., Linkenkaer-Hansen, K., Huttunen, J., and Ilmoniemi, R. J. (2001). Interhemispheric phase synchrony and amplitude correlation of spontaneous beta oscillations in human subjects: a magnetoencephalographic study. Neuroreport 12, 2487–2491.
Nolte, G., Bai, O., Wheaton, L., Mari, Z., Vorbach, S., and Hallett, M. (2004). Identifying true brain interaction from EEG data using the imaginary part of coherency. Clin. Neurophysiol. 115, 2292–2307.
Nuechterlein, K. H., and Green, M. F. (2006). MATRICS Consensus Cognitive Battery Manual. Los Angeles, MATRICS Assessment.
Pascual-Marqui, R. D. (2002). Standardized low resolution brain electromagnetic tomography (sLORETA): technical details. Methods Find. Exp. Clin. Pharmacol. 24, 5–12.
Peyk, P., Schupp, H. T., Elbert, T., and Junghöfer, M. (2008). Emotion processing in the visual brain: a MEG analysis. Brain Topogr. 20, 205–215.
Pomarol-Clotet, E., Salvador, R., Sarró, S., Gomar, J., Vila, F., Martínez, A., Guerrero, A., Ortiz-Gil, J., Sans-Sansa, B., Capdevila, A., Cebamanos, J. M., and McKenna, P. J. (2008). Failure to deactivate in the prefrontal cortex in schizophrenia: a dysfunction of the default mode network? Psychol. Med. 38, 1185–1193.
Porcaro, C., Zappasodi, F., Rossini, P. M., and Tecchio, F. (2009). Choice of multivariate autoregressive model order affecting real network functional connectivity estimate. Clin. Neurophysiol. 120, 436–448.
Potkin, S. G., and Ford, J. M. (2009). Widespread cortical dysfunction in schizophrenia: the FBIRN imaging consortium. Schizophr. Bull. 35, 15–18.
Pulvermüller, F., and Shtyrov, Y. (2006). Language outside the focus of attention: the mismatch negativity as a tool for studying higher cognitive processes. Prog. Neurobiol. 79, 49–71.
Ragland, J. D., Yoon, J., Minzenberg, M. J., and Carter, C. S. (2007). Neuroimaging of cognitive disability in schizophrenia: search for pathophysiological mechanism. Int. Rev. Psychiatry 19, 417–427.
Ramnani, N., Behrens, T. E., Penny, W., and Matthews, P. M. (2004). New approaches for exploring anatomical and functional connectivity in the human brain. Biol. Psychiatry 56, 613–619.
Reite, M., and Rojas, D. (1997). MEG correlates of psychoses: anomalous lateralization and abnormal memory function. In Quantative and Topological EEG and MEG Analysis, H. Witte, U. Zwiener, B. Schack and A. Doering, eds (Druckhaus Mayer Verlag, Jena), pp. 150–154.
Reite, M., Teale, P., and Rojas, D. C. (1999). Magnetoencephalography: applications in psychiatry. Biol. Psychiatry 45, 1553–1563.
Reite, M., Teale, P., Zimmerman, J. E., Davis, K., and Whalen, J. (1988). Source location of a 50 msec latency auditory evoked field component. Electroencephalogr. Clin. Neurophysiol. 70, 490–498.
Robinson, S. E. and Vrba, J. (1999). Functional neuroimaging by synthetic aperture magnetometry (SAM). In Recent Advances in Biomagnetism, T. Yoshimoto, M. Kotani, S. Kuriki, H. Karibe, and N. Nakasato, eds (Sendai, Tohoku University Press), pp. 302–305.
Rockstroh, B., Junghöfer, M., Elbert, T., Buodo, G., and Miller, G. A. (2006). Electromagnetic brain activity evoked by affective stimuli in schizophrenia. Psychophysiology 43, 431–439.
Rutter, L., Carter, F. W., Holroyd, T., Nadar, S. R., Mitchell-Francis, J., Apud, J., Weinberger, D. R., and Coppola, R. (2009). Magnetoencephalographic gamma power reduction in patients with schizophrenia during resting condition. Hum. Brain Mapp. 30, 3254–3264.
Salgado-Pineda, P., Caclin, A., Baeza, I., Junque, C., Bernardo, M., Blin, O., and Fonlupt, P. (2007). Schizophrenia and frontal cortex: where does it fail? Schizophr. Res. 91, 73–81.
Schelter, B., Winterhaler, M., Eichler, M., Peifer, M., Hellwig, B., Guschlbauer, B., Lücking, C. H., Dahlhaus, R., and Timmer, J. (2006). Testing for directed influences among neural signals using partial directed coherence. J. Neurosci. Methods 152, 210–219.
Scherk, H., and Falkai, P. (2006). Effects of antipsychotics on brain structure. Curr. Opin. Psychiatry 19, 145–150.
Schlösser, R., Koch, K., Wagner, G., Schultz, C., Robel, M., Schachtzabel, C., Reichenbach, J. R., and Sauer, H. (2009). Intensive practice of a cognitive task is associated with enhanced functional integration in schizophrenia. Psychol. Med. 20, 1–11.
Schoonhoven, R., Boden, C. J., Verbunt, J. P., and de Munck, J. C. (2003). A whole head MEG study of the amplitude-modulation-following response: phase coherence, group delay and dipole source analysis. Clin. Neurophysiol. 114, 2096–2106.
Sekihara, K., and Nagarajan, S. S. (2008). Adaptive Spatial Filtering for Electromagnetic Brain Imaging. Springer Verlag. Berlin, Germany.
Sekihara, K., Nagarajan, S. S., Poeppel, D., Marantz, A., and Miyashita, Y. (2001). Reconstructing spatio-temporal activities of neural sources using an MEG vector beamformer technique. IEEE Trans. Biomed. Eng. 48, 760–771.
Shin, K. S., Kim, J. S., Kang, D. H., Koh, Y., Choi, J. S., O’Donnell, B. F., Chung, C. K., and Kwon, J. S. (2009). Pre-attentive auditory processing in ultra-high-risk for schizophrenia with magnetoencephalography. Biol. Psychiatry 65, 1071–1078.
Smith, E. E., Jonides, J., Marshuetz, C., and Koeppe, R. A. (1998). Components of verbal working memory: evidence from neuroimaging. Proc. Natl. Acad. Sci. U.S.A. 95, 876–882.
Sperling, W., Martus, P., Kober, H., Bleich, S., and Kornhuber, J. (2002). Spontaneous, slow and fast magnetoencephalographic activity in patients with schizophrenia. Schizophr. Res. 58, 189–199.
Srinivasan, R., Winter, W. R., Ding, J., and Nunez, P. L. (2007). EEG and MEG coherence: measures of functional connectivity at distinct spatial scales of neocortical dynamics. J. Neurosci. Methods. 166, 41–52.
Stam, C. J., Nolte, G., and Daffertshofer, A. (2007). Phase lag index: assessment of functional connectivity from multi-channel EEG and MEG with diminished bias from common sources. Hum. Brain Mapp. 28, 1178–1193.
Strelets, V. B., Novototsky-Vlasov, V. Y., and Golikova, J. V. (2002). Cortical connectivity in high-frequency beta-rhythm in schizophrenics with positive and negative symptoms. Int. J. Psychophysiol. 44, 101–115.
Stufflebeam, S. M., Witzel, T., Mikulski, S., Hämäläinen, M. S., Temereanca, S., Barton, J. J., Tuch, D. S., and Manoach, D. S. (2008). A non-invasive method to relate the timing of neural activity to white matter microstructural integrity. Neuroimage 42, 710–716.
Tauscher, J., Fischer, P., Neumeister, A., Rappelsberger, P., and Kasper, S. (1998). Low frontal electroencephalographic coherence in neuroleptic-free schizophrenic patients. Biol. Psychiatry 15, 438–447.
Thoma, R. J., Hanlon, F. M., Huang, M., Miller, G. A., Moses, S. N., Weisend, M. P., Jones, A., Paulson, K. M., Irwin, J., and Cañive, J. M. (2007). Impaired secondary somatosensory gating in patients with schizophrenia. Psychiatry Res. 151, 189–199.
Thoma, R. J., Hanlon, F. M., Moses, S. N., Edgar, J. C., Huang, M., Weisend, M. P., Irwin, J., Sherwood, A., Paulson, K., Bustillo, J., Adler, L. E., Miller, G. A., and Cañive, J. M. (2003). Lateralization of auditory sensory gating and neuropsychological dysfunction in schizophrenia. Am. J. Psychiatry 160, 1595–1605.
Thoma, R. J., Hanlon, F. M., Moses, S. N., Ricker, D., Huang, M., Edgar, C., Irwin, J., Torres, F., Weisend, M. P., Adler, L. E., Miller, G. A., and Cañive, J. M. (2005). M50 sensory gating predicts negative symptoms in schizophrenia. Schizophr. Res. 73, 311–318.
Thönnessen, H., Zvyagintsev, M., Harke, K. C., Boers, F., Dammers, J., Norra, Ch., and Mathiak, K. (2008). Optimized mismatch negativity paradigm reflects deficits in schizophrenia patients. A combined EEG and MEG study. Biol. Psychol. 77, 205–216.
Ukai, S., Shinosaki, K., Ishii, R., Ogama, A., Mizuno-Matsumo, Y., Inouye, T., Hirabuki, N., Yoshimine, T., Robinson, S. E., and Takeda, M. (2002). Parallel distributed processing neuroimaging in the Stroop task using spatially filtered magnetoencephalography analysis. Neurosci. Lett. 334, 9–12.
Uutela, K., Hämäläinen, M., and Salmelin, R. (1998). Global optimization in the localization of neuromagnetic sources. IEEE Trans. Biomed. Eng. 45, 716–723.
Uutela, K., Hämäläinen, M., and Somersalo, E. (1999). Visualization of magnetoencephalographic data using minimum current estimates. Neuroimage 10, 173–180.
Vrba, J., and Robinson, S. E. (2001). Signal processing in magnetoencephalography. Methods 25, 249–271.
Wada, Y., Nanbu, Y., Jiang, Z. Y., Koshino, Y., and Hasimoto, T. (1998). Interhemispheric EEG coherence in never-medicated patients with paranoid schizophrenia: analysis at rest and during photic stimulation. Clin. Electroencephalogr. 29, 170–176.
Wager, T. D., and Smith, E. E. (2003). Neuroimaging studies of working memory: a meta-analysis. Cogn. Affect. Behav. Neurosci. 3, 255–274.
Whalley, H. C., Steele, J. D., Mukherjee, P., Romaniuk, L., McIntosh, A. M., Hall, J., and Lawrie, S. M. (2009). Connecting the brain and new drug targets for schizophrenia. Curr. Pharm. Des. 15, 2615–2631.
Winterer, G., Coppola, R., Egan, M. F., Goldberg, T. E., and Weinberger, D. R. (2003). Functional and effective frontotemporal connectivity and genetic risk for schizophrenia. Biol. Psychiatry 54, 1181–1192.
Wipf, D. P., and Nagarajan, S. (2009). A unified Bayesian framework for MEG/EEG source imaging. Neuroimage 44, 947–966.
Wipf, D., Owen, J. P., Attias, H., Sekihara, K., and Nagarajan, S. S. (2009). Robust Bayesian estimation of the location, orientation, and time course of multiple correlated neural sources using MEG. Neuroimage. 49, 641–655.
Woodward, N. D., Waldie, B., Rogers, B., Tibbo, P., Seres, P., and Purdon, S. E. (2009). Abnormal prefrontal cortical activity and connectivity during response selection in first episode psychosis, chronic schizophrenia, and unaffected siblings of individuals with schizophrenia. Schizophr. Res. 109, 182–190.
Yamasue, H., Yamada, H., Yumoto, M., Kamio, S., Kudo, N., Uetsuki, M., Abe, O., Fukuda, R., Aoki, S., Ohtomo, K., Iwanami, A., Kato, N., and Kasai, K. (2004). Abnormal association between reduced magnetic mismatch field to speech sounds and smaller left planum temporale volume in schizophrenia. Neuroimage 22, 720–727.
Zumer, J, Attias, H., Sekihara, K., and Nagarajan, S. S. (2007). A probabilistic algorithm integrating source localization and noise suppression for MEG and EEG data. Neuroimage 37, 102–115
Keywords: magnetoencephalography, functional connectivity, resting-state, schizophrenia, executive function
Citation: Hinkley LBN, Owen JP, Fisher M, Findlay AM, Vinogradov S and Nagarajan SS (2010) Cognitive impairments in schizophrenia as assessed through activation and connectivity measures of magnetoencephalography (MEG) data. Front. Hum. Neurosci. 3:73. doi: 10.3389/neuro.09.073.2009
Received: 02 June 2009;
Paper pending published: 21 August 2009;
Accepted: 16 December 2009;
Published online: 11 November 2010.
Edited by:
Vince D. Calhoun, University of New Mexico, USAReviewed by:
Jose Canive, University of New Mexico, USAFaith Hanlon, The Mind Research Network, USA
Copyright: © 2010 Hinkley, Owen, Fisher, Findlay, Vinogradov and Nagarajan. This is an open-access article subject to an exclusive license agreement between the authors and the Frontiers Research Foundation, which permits unrestricted use, distribution, and reproduction in any medium, provided the original authors and source are credited.
*Correspondence: Srikantan S. Nagarajan, Biomagnetic Imaging Laboratory, Department of Radiology and Biomedical Imaging, University of California, San Francisco, 513 Parnassus Avenue, S362, San Francisco, CA 94143, USA. e-mail: sri@radiology.ucsf.edu