Commentary on Shimoyama et al. (2012): three ontologies to define phenotype measurement data
- 1 Human and Molecular Genetics Center, Medical College of Wisconsin, Milwaukee, WI, USA
- 2 Pathology and Immunology, Washington University School of Medicine, St. Louis, MO, USA
Background: There is an increasing need to integrate phenotype measurement data across studies for both human studies and those involving model organisms. Current practices allow researchers to access only those data involved in a single experiment or multiple experiments utilizing the same protocol. Results: Three ontologies were created: Clinical Measurement Ontology, Measurement Method Ontology and Experimental Condition Ontology. These ontologies provided the framework for integration of rat phenotype data from multiple studies into a single resource as well as facilitated data integration from multiple human epidemiological studies into a centralized repository. Conclusion: An ontology based framework for phenotype measurement data affords the ability to successfully integrate vital phenotype data into critical resources, regardless of underlying technological structures allowing the user to easily query and retrieve data from multiple studies.
Background
The quest to link characteristics of an individual or organism to genetic structures dates to the mid-1800s and the work of Gregor Mendel (Sorsby, 1965). In the past 20 years, a great deal of progress has been made in identifying, naming, and standardizing the information about genetic structures. The International Nucleotide Sequence Database Collaboration1 was created to develop standard formats for genomic data to integrate data generated at multiple laboratories using a variety of technologies resulting in public databases housed at the National Center for Biotechnology Information (NCBI; Sayers et al., 2010), the DNA Databank of Japan (Kaminuma et al., 2010), and the European Bioinformatics Institute (EBI; Goujon et al., 2010). This integration of data has led to the development of numerous data mining, presentation, and analysis tools and provides a platform for comparisons of genetic and genomic structures across species. Unfortunately, a similar development in data standards and integration has not occurred for the characteristics of an individual or organism scientists wish to link to these structures. The potential value of integrating phenotype data from multiple sources (e.g., different laboratories or studies, varying techniques to measure similar phenotypes, multiple populations, or strains of a particular organism) is enormous. The power to identify novel genes associated with human disease and the role a gene plays in disease is greatly increased with clearly defined phenotype information and the inclusion of the environmental and experimental context (Butte and Kohane, 2006). However, most phenotype data is gathered or generated without thought to integrating the results with those from other studies even within the same laboratory or program, creating barriers to integrating and comparing results reported in publications. In both animal and human physiological and disease studies, there has been a long tradition of designing new protocols and adopting evolving best practices available at the time the study is launched. As a result, the same basic information gets collected differently across protocols. This leads to a common belief that each study is unique and cannot be compared to any other for anything more than the most general elements. Moreover, the data sets and study information are structured in such a way that, often, only those who are intimately familiar with the study understand the full depth of the data; this includes details such as the measurement methods used and the experimental conditions imposed. For most researchers, ferreting out this information from different studies requires extensive time and effort, as is generally experienced by post hoc collaborations among multiple studies. Even when these details are published, they are often described in widely different ways without full inclusion of details making comparisons across studies not only difficult but sometimes impossible.
Variations in experimental conditions, population, age, and study design all contribute to the difficulty in comparing phenotype data from multiple sources. For example, the comparison of blood pressure measured in different laboratories or programs can be impacted by the way in which blood pressure is measured (e.g., direct measurement via catheter in artery, telemetry, blood pressure cuff), the experimental conditions imposed as part of the study (e.g., low salt/high salt diet, exercise, oxygen levels), surgical manipulations (e.g., removal of a kidney), gender, and age. One approach to aggregating and integrating phenotype data would be to develop standard phenotyping protocols to be followed by all researchers. However, standardizing the methods used for phenotyping protocols has significant drawbacks. Many would see it as impractical since each researcher is testing fairly unique hypotheses which cannot be easily investigated by using a set protocol. Additionally, not all laboratories measure phenotypes using the same assays, nor do all investigators agree on one perfect method to measure each phenotype. Any movement toward this type of standardization would take years before results were evident, keeping existing data resources inaccessible. A more practical approach is to develop a method using ontologies and standardized data formats to integrate phenotype measurement data sets.
A number of groups have focused on standardizing biological information through standardized vocabularies and ontologies. Ontologies are hierarchically structured vocabularies of terms and relationships that are clearly defined and designed to represent and communicate information about a particular scientific domain (Figure 2). The entities and concepts represented by the terms in the lower nodes are assumed to inherit the properties and qualities of those of nodes higher up the branch. The National Institutes of Health, in recognition of the utility of ontologies and the need for more ontologies to represent biological concepts, provided funding for the creation of the National Center for Biomedical Ontology (NCBO)2 in 2006 (Rubin et al., 2006). There are currently 242 ontologies cataloged at NCBO including several which focus on phenotypes.
Mammalian Phenotype Ontology
The Mammalian Phenotype (MP) Ontology was initially created for annotating gene alleles at the Mouse Genome Informatics (MGI) database (Smith et al., 2005). For the MP ontology, “phenotype refers to the observable morphological, physiological, and behavioral characteristics of an individual in the context of the environment” (Smith and Eppig, 2009). Because MP was designed to be used with mouse knockouts, mutations, and other types of alleles, there is an underlying assumption of a comparison to the trait exhibited by a mouse with the genetic background from which the allele has been constructed or a comparison to a normal or “wild type” trait. Thus the terms often contain words such as “abnormal,” “increased,” or “decreased” with the implication of “relative to” an assumed observation. The actual measured values for observed traits are not connected to these annotations. MP follows the open-source Open Biological and Biomedical Ontology (OBO) file format and is organized on the Directed Acyclic Graph (DAG) structure with the highest nodes related to physiological systems such as cardiovascular, immune system as well as behavioral, life span, and cellular phenotypes. Each physiological system node is followed by a basic division into physiological and morphological phenotype branches. MGI currently has over 41,000 genotypes annotated with MP terms for a total of more than 193,000 annotations. MP is considered a pre-coordinated term ontology since both the entity (i.e., anatomical site or physiological process) and the quality of it (i.e., abnormal, increased, decreased) are included in the term. MP is also being used for the EuroPhenome project to annotate mutant mouse phenotype data generated using standard phenotyping platforms (Morgan et al., 2010). The advantages in terms of annotation are significant since curators only have to search a single ontology and has terms that more closely mimic those seen in literature and commonly used in laboratory settings.
Phenotype and Trait Ontology
Another approach to phenotype ontologies has been the Phentoype and Trait Ontology (PATO) project3 (Gkoutos et al., 2004). Unlike MP which is considered a pre-coordinated term phenotype ontology, PATO uses the EQ approach (entity + quality; Gkoutos et al., 2004; Smith and Eppig, 2009). Thus PATO presents terms related to qualities and attributes that are then linked to terms from other ontologies such as anatomy ontologies to describe phenotypic characteristics. Thus, “big ears” would be represented by the term “increased size” from PATO and the word “ear” from an anatomy ontology (Mungall et al., 2010). One of the advantages of this approach is the re-use of existing ontologies such as the anatomy ontology. Representing morphological traits through the use of anatomy ontologies and the qualities described in PATO is relatively straight forward. This is one reason that resources housing data for some organisms such as drosophila and zebrafish have found this approach useful (Mungall et al., 2010); the majority of their reported traits and phenotypes are morphological in nature. However, the representation of physiological traits and specific clinical measurement types is more problematic (Smith and Eppig, 2009). First, there is not necessarily a single ontology that adequately represents the physiological trait corresponding with the quality expressed by PATO; and second, a single EQ term may not adequately express the phenotype observed. For example while the morphological trait of “big ears” is relatively easy to represent by EQ, an MP term of “abnormal cochlear outer hair cell electromotility” provides a greater challenge. The disadvantages of the PATO approach for annotation are also significant. Curators would have to browse multiple ontologies to create a term on the fly and this approach creates terms and phrases that sometimes are stilted or not commonly used in the literature or laboratory settings.
Human Phenotype Ontology
The Human Phenotype Ontology (HPO) was developed in part to address the shortcomings of information presented in the Online Mendelian Inheritance in Man (OMIM) database4 (Amberger et al., 2009). OMIM has traditionally been the most commonly used resource for information on genetic diseases. Unfortunately, the information housed there is in free text format, making it difficult to mine computationally because of the non-standard way in which traits and abnormalities are described. For instance, OMIM uses the synonymous descriptions “generalized amyotrophy,” “generalized muscular atrophy,” and “muscular atrophy, generalized” so even simple searches may not return the results a user desires. While a human reader going through the free text of multiple entries will recognize similar meanings, computers will not. The initial version of HPO was created using the information at OMIM in an effort to merge synonyms and create links and relationships among the terms and concepts. This initial structure has been expanded and refined through manual curation of information from a variety of sources and consistent development of definitions and relationships (Robinson and Mundlos, 2010). As with MP, the emphasis has been on phenotypes which diverge from the normal or expected and disease states and terms are pre-constructed as with the MP.
Ontologies such as MP, PATO, and HPO were originally designed for use in simple annotations to a single data (e.g., gene product or allele) or an individual and the term was expected to appear alone in the annotation with the minimal accompanying information of an evidence code indicating level of experimental evidence to support the annotation and the reference from which the annotation was made. The existing phenotype ontologies were not developed to be attached to actual measurement values but to indicate a state or characteristic observed relative to that which has been determined to be “normal” or “wild type,” or relative to that exhibited by an individual with a known genotype. Information on experimental conditions and measurement assays used are vital parts of the phenotype record and the use of multiple ontologies to represent these has been advocated as a way to accomplish this (Shimoyama et al., 2005; Hancock et al., 2007). Clearly, developing separate ontologies for the elements of phenotype measurement, method of measurement, and conditions under which the measurement was made along with provisions for additional information on actual values, duration of conditions, and so on, will allow these aspects of the phenotype record to be linked. Database structures which allow re-use of information and multiple associations will facilitate data integration, data mining, and data presentation.
In this paper, we present three ontologies created to standardize phenotype measurement records for use in human studies and those using laboratory animals: Clinical Measurement Ontology (CMO), Measurement Method Ontology (MMO), Experimental Condition Ontology.
Materials and Methods
The standard elements of phenotype measurement records were identified as: (1) what was measured, (2) how it was measured, and (3) under what conditions it was measured. Ontologies were developed to standardize each of these elements (Figure 1) and include (1) CMO, (2) MMO, and (3) Experimental Conditions Ontology (XCO).
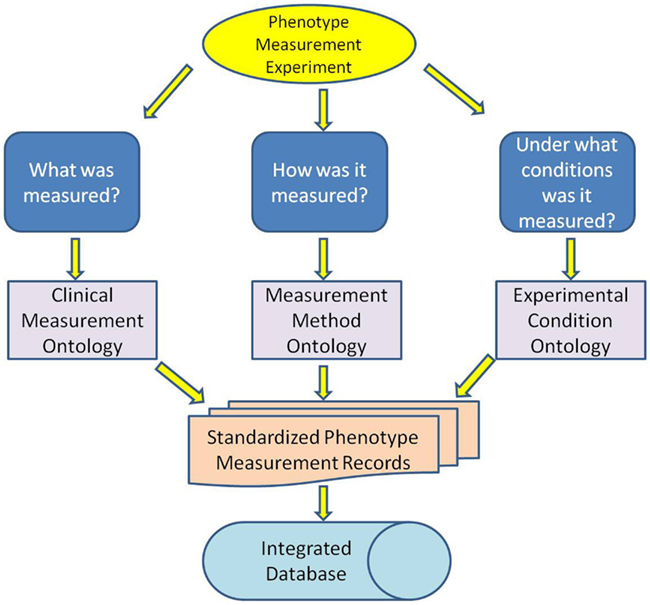
Figure 1. Three ontologies were developed to standardize the three elements of a measurement record: what was measured, how it was measured and under what conditions it was measured.
The ontologies are available through the NCBO Bioportal5, and at the Rat Genome Database in ftp files6. The ontologies undergo revisions and updates for both consistencies in format and to extend the breadth and depth of coverage as new measurement records are added. The ontologies were developed using the OBO format and the OBO Edit tool (Day-Richter et al., 2007) available at the NCBO, through its Foundry project (Smith et al., 2007). While developed in OBO, developments in OBO to Web Ontology Language (OWL) mapping tools should facilitate conversion to this other highly used ontology format. The major ontology development tools, OBO Edit for OBO and Protege for OWL now offer widgets that facilitate conversion from one file format to the other (see text footnote 2). The OBO Relation Ontology (RO) was used to create consistency in relationship representations (Smith et al., 2007). These ontologies follow the form of DAGs in which there is a set of nodes with edges forming the linkage between nodes (Robinson and Mundlos, 2010). The nodes are the terms in the ontology with the edges representing the relationships between nodes and the overall visualization of such ontologies resembles branches. In DAGs, the edges or relationships are one way, moving from one node to another, and they do not cycle back. The general relationship pattern in many of these ontologies is a movement from the more general (higher nodes) to the more specific (lower nodes). The entities and concepts represented by the terms in the lower nodes are assumed to inherit the properties and qualities of those of nodes higher up the branch.
The development of these ontologies has included cross references with other ontologies when an exact match of the entity exists. For example, relationships were created with ChEBI in the Experimental Condition Ontology and to the Electrocardiography Ontology (ECG) exist in the CMO. These relationships were created manually and are not used to create cross products. Cross referencing to other ontologies, such as the Cell Ontology, Evidence Code Ontology, and other ontologies used for the reporting of phenotypes, will continue with both manual and semi-automated methods as the ontologies are extended.
Clinical Measurement Ontology
The CMO provides the standardized vocabulary necessary to indicate the type of measurement made to assess a trait. For the purposes of this project and these ontologies, trait and clinical measurement are defined as follows:
Trait
A physiological or morphological state or property found in all members of a species. Traits can be described or assessed quantitatively (numerically) or qualitatively based on the results of an appropriate form of measurement. The assessment of the trait is not equivalent to the trait itself. Traits exist even when they are not assessed or measured. Often multiple forms of measurement are used to assess a single property or state.
Measurement
The act or result of the act of assessing a morphological or physiological state or property in a single individual or group of individuals and assigning a quantitative or qualitative value. A measurement does not exist until it is performed or taken. Often a single measurement can be used to assess multiple properties or states, sometimes in conjunction with other measurements.
For example, all humans have intelligence or mental capacity, but not all human individuals have an IQ because it has not yet been measured. Similarly, all humans have a body mass but they do not all have a body weight because it has not yet been measured.
Each term in the CMO describes a distinct type of measurement used to assess one or more traits. The terms are arranged in a hierarchical structure of classes so that lower classes are subclasses of higher classes in the branch (Figure 2).
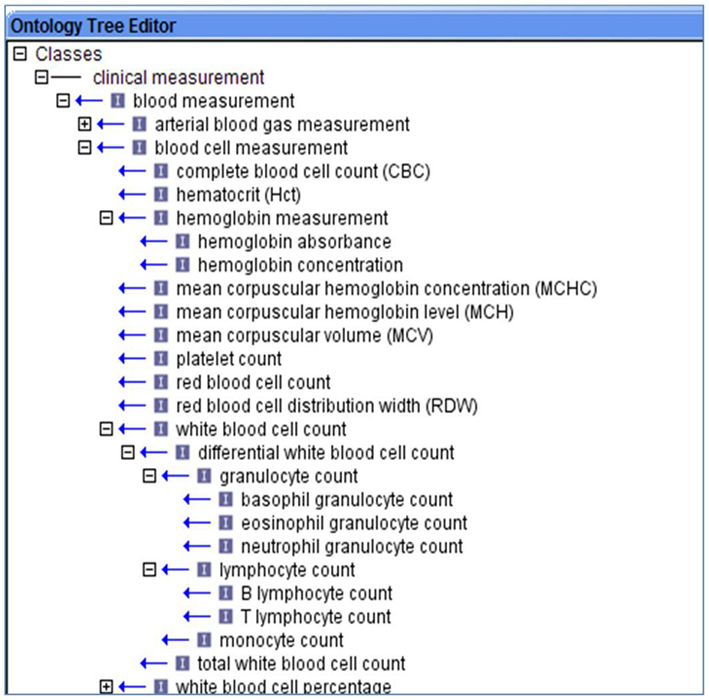
Figure 2. The Clinical Measurement Ontology is presented in a hierarchical structure with classes lower down a branch being subclasses of those above with an “is_a” relationship.
This represents an “is_a” type relationship so that a lower term “is_a” subclass of a higher term. Thus, blood cell measurement “is_a” blood measurement and complete blood cell count “is_a” blood cell measurement. The measurements in the ontology are primarily organized on the highest level according to the body system in which the measurement is made. Trait areas were targeted for ontology development based on the availability and extent of data in large scale rat phenotyping projects, published rat literature with phenotype measurements and targeted human epidemiological studies. Ontology development began with the identification of clinical measurements used to assess targeted traits, with terms and definitions being created and relationships among terms being set (Figure 3).
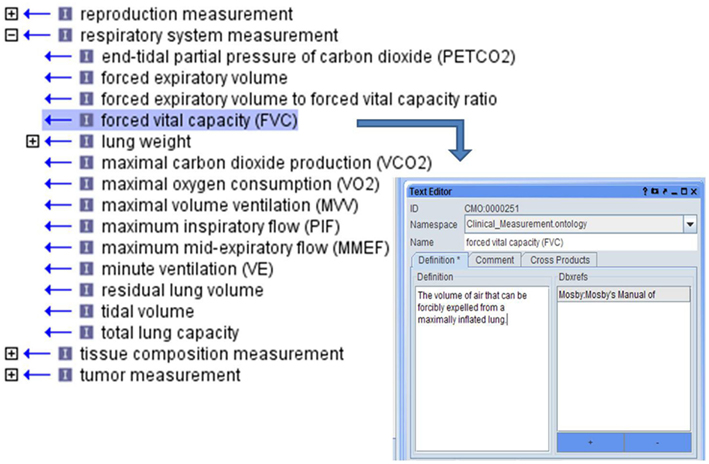
Figure 3. Each CMO term was created as phenotype domains addressed with appropriate definitions for each term.
Existing ontologies at NCBO were reviewed for associated terms and definitions. Because the clinical measurements in the targeted sources may be limited, additional literature including medical and physiological textbooks, laboratory manuals, and published research literature were reviewed to ensure completeness in the ontology. For example, to assess kidney mass the data source may only use right kidney weight. Further review of a variety of sources reveals that other typical measurements would include left kidney weight, weight of both kidneys, kidney weight expressed as a percentage of body weight which are also often used to assess the trait of kidney mass so these were also added to the ontology. For every clinical measurement term created, associated measurement method terms and experimental condition terms were created based on data in the originating sources as well as the review of additional literature and existing ontologies. There are currently 523 terms in the CMO for measurement types ranging from morphological to physiological for blood, cardiovascular, respiratory, renal, and other systems as well as for growth, reproduction, consumption, tumors, and tissue composition.
Measurement Method Ontology
A critical element in the description of a phenotype is the measurement method used. Several types of methods are commonly used to measure such things as blood pressure resulting in differing clinical measurement values so the inclusion of method as part of the measurement reading is necessary for the integration of data from multiple studies. The MMO is designed to provide this information. As described above, this ontology was developed in parallel with the CMO as trait areas are targeted. The MMO is organized by the underlying principle or mechanism of the method (Figure 4) with two major branches, “ex vivo method” and “in vivo method.” Methods were identified from protocol descriptions and data labels from the targeted data sources. As with the CMO, for completeness in the ontology, additional sources of method information such as vendors’ catalogs, laboratory manuals, and published literature were reviewed for associated methods. Thus, if one of the protocols for one of the originating data sources indicated that a balloon tipped catheter was used to measure blood pressure, a quick review of a variety of publications revealed that the basic category is vascular indwelling catheter with a variety of types including fluid filled catheter, intravascular electromagnetic flow sensor, and transducer tipped catheter. There are currently 195 terms in the MMO.
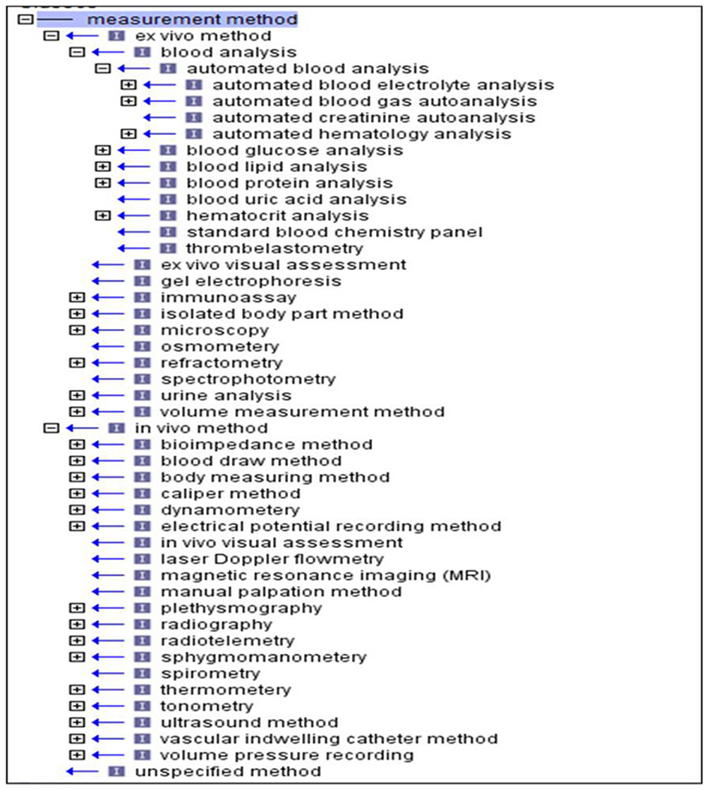
Figure 4. The Measurement Method Ontology structure is based on two major branches, “ex vivo” and “in vivo” and the underlying mechanism or technique used in the method.
Experimental Condition Ontology
While many phenotype measurements are made under baseline conditions, changes to diet, atmosphere, activity level, and other conditions are common aspects of phenotype experiments. Often this information is added as part of the phenotype label in the individual laboratory’s database or only included as part of a lengthy text protocol. Creation of standardized terminology and format for presenting this information with phenotype measurements is crucial to the integration of phenotype data from multiple datasets. An XCO was created to provide standardization and structure for this important information (Figure 5).
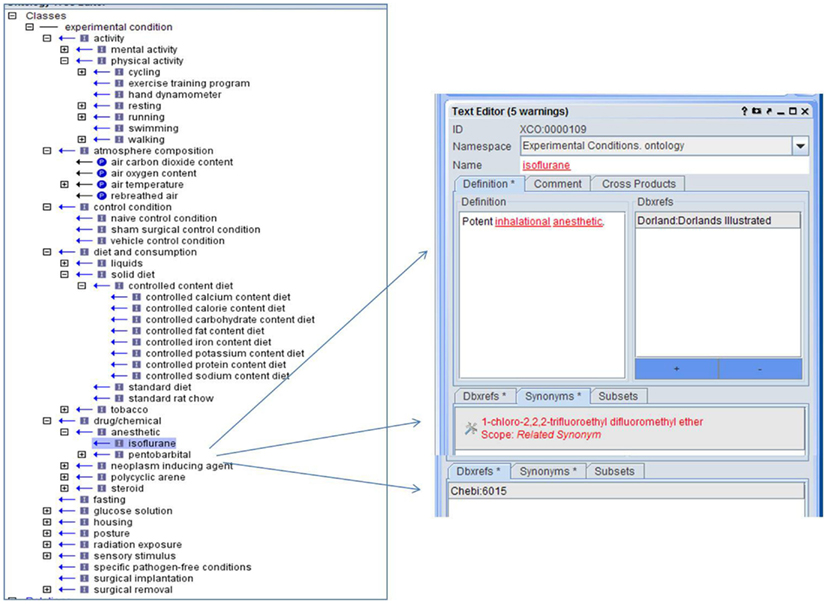
Figure 5. The Experiment Condition Ontology is structured by type of condition with both “is_a” and “part_of” relationships with links to identifiers found in other ontologies.
In addition, to the “is_a” relationship, in certain areas a “part_of” relationship is utilized so that parts of a whole can be described as in “air oxygen content” is “part_of” “atmosphere composition.” The ontology was designed so that conditions related to existing ontologies such as those involving chemicals or drugs represented in ChEBI (Degtyarenko et al., 2009), follow the structure and terminology of these ontologies and provide appropriate linkages through identifiers (Figure 5). Initial emphasis was on the conditions used in the targeted data sets and expanded to those conditions most commonly used in experiments involving the targeted trait domains. Structural provisions in the database structures of projects using the ontology provide ordinality information to indicate whether multiple conditions were simultaneous or sequential. Use of this ontology in annotating phenotype data allows users to retrieve multiple, disparate phenotype information in which similar experimental conditions were imposed. There are currently 110 terms in the XCO.
Data Integration
The three ontologies have been used to integrate multiple data sets for two major projects, one involving human data and the other involving rat data. The Cardiovascular Ontologies and Vocabularies in Epidemiological Research project was designed to integrate demographic and phenotype measurement data from three family blood pressure studies, Hypertension Genetic Epidemiology Network (HyperGEN; Williams et al., 2000); Genetic Epidemiology Network of Salt Sensitivity (GenSalt; Gu et al., 2007); and HEalth, RIsk factors exercise Training And GEnetics (HERITAGE; Bouchard et al., 1995). While all three studies focused on cardiovascular disease and associated risk factors, they were disparate in the types of interventions used, variety of measurements taken and the methods used to make the measurements. Measurements related to blood pressure, blood chemistry and lipid levels, body weight and body fat were included as well as interventions ranging from sodium controlled diets to exercise. Invasive, non-invasive and imaging techniques were also used. The HyperGEN study was designed to characterize the genes influencing hypertension by recruiting hypertensive sibships (i.e., each participant with two or more hypertensive sibs) from across multiple field centers and ethnic groups. The GenSalt study is an intervention study of the genetic and environmental factors related to dietary sodium and potassium effects on blood pressure in rural Chinese families. The HERITAGE study is an intervention study designed to assess genetic and environmental factors underlying the effects of endurance exercise training on several cardiorespiratory and cardiovascular disease risk factors. The CMO, MMO, and Experimental Condition Ontology were used to map data elements from each of the studies to a common format for integration into a single resource7. To date, 16 phenotype classes with records for 8,778 subjects have been integrated for the three studies and made available at the website. Additionally, all variables across the studies are being mapped and modeled with their associated ontology terms to facilitate querying and access to raw data fields of interest; to date 11 classes have been created representing over 100 phenotype measurements.
The rat PhenoMiner project8 (Figure 6) also has used the three ontologies to define data formats and standards for integrating rat phenotype measurement data from a variety of sources including two large scale phenotyping projects and published literature. PhysGen Program for Genomic Applications9 (Kwitek et al., 2006), one of the large scale phenotyping projects was designed to conduct high throughput phenotype screening for a targeted set of inbred strains, as well as consomic and mutant strains. The screens involved hundreds of different types of phenotype measurements for heart, lung, renal, vascular, and blood function under baseline conditions as well as varying diet, atmosphere, and activity conditions. Data was organized, stored, and presented by protocol so even though some similar measurements such as weight or blood pressure were measured in multiple protocols, the data was not integrated across protocols. The National BioResource Project for the Rat in Japan (Serikawa et al., 2009) was the second large scale rat phenotyping project. Phenotype screens for body weight, activity, behavior, blood pressure, blood chemistry, urine analysis, and organ weights have been conducted under baseline conditions for inbred and mutant strains.
Because the rat is an ideal model organism for pharmacology, biochemistry, and physiology research, the published literature is a rich resource of data on phenotype measurements for particular strains. Papers reporting cardiovascular, respiratory, renal, morphological, and blood chemistry measurement data as well as those with measurements related to cancer were targeted for the initial phase of the PhenoMiner resource. Over 13,000 measurement records from these three sources have been mapped to the three ontologies and integrated into PhenoMiner (Figure 7).
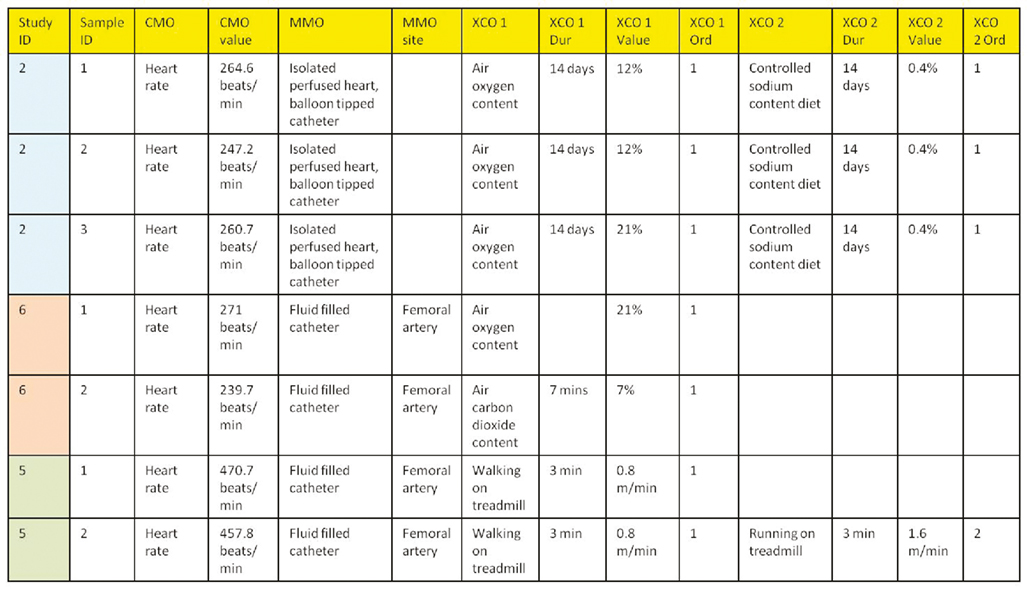
Figure 7. Example of phenotype measurement data from multiple studies mapped to the three ontologies for clinical measurement, measurement method, and experimental condition.
Discussion and Conclusion
The three ontologies created have proven to be excellent tools for standardizing phenotype measurement data for projects involving a wide variety of data types and data sources. Targeting the three basic elements of: (1) what was measured; (2) how it was measured; and (3) under what conditions it was measured, facilitated standardization while allowing for flexibility in providing associated information such as units of measurement or duration of condition to be formatted in ways particular to the integrating resource. These ontologies allowed phenotype measurement data from disparate studies to be integrated without compromising study-specific aspects related to methodology. Multiple datasets of human epidemiological data and rat phenotype data were successfully integrated into resources designed to meet the needs of diverse research communities even though the underlying technological framework for the databases and associated tools differed. While integrating varied phenotype datasets was the primary motivation for the development of these ontologies, they can be deployed in a variety of other projects as well. Because of their availability at NCBO, they can be utilized with the NCBO Annotator, a Web service that annotates journal abstracts10 which facilitates curation efforts and queries for appropriate literature for specific projects. Because of their focus on experimental data, the use of these ontologies in text mining tools would also help investigators identify and prioritize literature.
Creating structures to integrate phenotype measurement data from multiple sources is an important task as investigators draw on the strength of the genomic and sequence variation resources to identify underlying genotype factors related to phenotypes and diseases. In order to make these connections, researchers need to easily access and analyze phenotype measurement data related to individuals and various model strains, and information on experimental conditions and methodologies that may affect the measurement values. Employing multiple ontologies to standardize data formats facilitates the integration of these vital datasets and provides the structure on which innovative data mining, analysis, and presentation tools can be built. These types of resources can provide researchers with a more accurate picture of phenotype variations among populations and as well as the impact of measurement methods may have on measurement results. The influence of experimental and environmental conditions on phenotypes and disease will also be easier to elucidate when researchers have access to large numbers of measurements from a wide variety of studies. This is an important step in helping investigators link genotypes to phenotypes. Finally, the use of multiple ontologies to standardize data elements into single quantifiable records can be used in many paradigms to integrate datasets. Convergence among phenotyping efforts can be fostered using this methodology through the use of existing ontologies, such as MP, PATO, and HPO, in conjunction with ontologies that further refine the phenotype data. Engaging other communities will provide the platform for data mining across species or across phenotyping programs using a variety of phenotyping protocols.
Authors’ Contributions
Mary Shimoyama created the ontologies, organized the data mapping, and integration at the Medical College of Wisconsin and participated in data mapping for the COVER project. Rajni Nigam assisted in ontology term creation and participated in data mapping. Leslie Sanders McIntosh organized data mapping and informatics component creation for the COVER project. Rakesh Nagarajan participated in the informatics infrastructure design for COVER project. Treva Rice coordinated data mapping and integration for the COVER project. D. C. Rao participated in design and coordinated data mapping and integration for the COVER project. Melinda R. Dwinell participated in the design of the PhenoMiner tool and data integration at the Medical College of Wisconsin. All authors have read and approved the manuscript.
Conflict of Interest Statement
The authors declare that the research was conducted in the absence of any commercial or financial relationships that could be construed as a potential conflict of interest.
Acknowledgments
The authors would like to acknowledge the contributions of the RGD curation team at the Medical College of Wisconsin and the staff and informatics team at Washington University. This project is funded in part by R01HL094271, R01HL094286, and R01HL064541.
Footnotes
- ^http://www.insdc.org/
- ^http://bioontology.org/
- ^http://www.bioontology.org/wiki/index.php/PATO:Main_Page
- ^http://www.ncbi.nlm.nih.gov/omim
- ^http://bioportal.bioontology.org/
- ^http://rgd.mcw.edu/pub/ontology
- ^http://cover.wustl.edu/Cover/
- ^http://rgd.mcw.edu/phenotypes/
- ^http://pga.mcw.edu/
- ^http://bioportal.bioontology.org/annotator#
References
Amberger, J., Bocchini, C. A., Scott, A. F., and Hamosh, A. (2009). McKusick’s online Mendelian inheritance in man (OMIM). Nucleic Acids Res. 37, D793–D796.
Bouchard, C., Leon, A. S., Rao, D. C., Skinner, J. S., Wilmore, J. H., and Gagnon, J. (1995). The HERITAGE family study. Aims, design, and measurement protocol. Med. Sci. Sports Exerc. 27, 721–729.
Butte, A. J., and Kohane, I. S. (2006). Creation and implications of a phenome-genome network. Nat. Biotechnol. 24, 55–62.
Day-Richter, J., Harris, M. A., Haendel, M., and Lewis, S. (2007). OBO-Edit – an ontology editor for biologists. Bioinformatics 23, 2198–2200.
Degtyarenko, K., Hastings, J., De Matos, P., and Ennis, M. (2009). ChEBI: an open bioinformatics and cheminformatics resource. Curr. Protoc. Bioinformatics Chapter 14, Unit 14.19.
Gkoutos, G. V., Green, E. C., Mallon, A. M., Hancock, J. M., and Davidson, D. (2004). Building mouse phenotype ontologies. Pac. Symp. Biocomput. 178–189.
Goujon, M., Mcwilliam, H., Li, W., Valentin, F., Squizzato, S., Paern, J., and Lopez, R. (2010). A new bioinformatics analysis tools framework at EMBL-EBI. Nucleic Acids Res. 38(Suppl.), W695–W699.
Gu, D., He, J., Hixson, J. E., Jaquish, C. E., Liu, D., Rao, D. C., Whelton, P. K., Yao, Z., He, J., Bazzano, L. A., Chen, C.-S., Chen, J., Hamm, L., Muntner, P., Reynolds, K., Reuben, J. R., Whelton, P. K., Yang, W., Rao, D. C., Brown, M., Gu, C., Rice, T., Schwander, K., Wang, S., Gu, D., Cao, J., Chen, J., Duan, X., Huang, J., Huang, J., Li, J., Liu, D., Liu, D., Pan, E., Wei, Y., Wu, X., Lu, F., Jin, S., Meng, Q., Wu, F., Zhao, Y., Ma, J., Li, W., Zhang, J., Hu, D., Ding, Y., Wen, H., Zhang, M., Zhang, W., Ji, X., Li, R., Zu, H., Yao, C., Li, Y., Shen, C., Zhou, J., Mu, J., Chen, E., Huang, Q., Wang, M., Yao, Z.-J., Chen, S., Gu, D., Li, H., Wang, L., Zhang, P., Zhao, Q., Hixson, J. E., Shimmin, L. C., and Jaquish, C. E. (2007). GenSalt: rationale, design, methods and baseline characteristics of study participants. J. Hum. Hypertens. 21, 639–646.
Hancock, J. M., Adams, N. C., Aidinis, V., Blake, A., Bogue, M., Brown, S. D., Chesler, E. J., Davidson, D., Duran, C., Eppig, J. T., Gailus-Durner, V., Gates, H., Gkoutos, G. V., Greenaway, S., Hrabe De Angelis, M., Kollias, G., Leblanc, S., Lee, K., Lengger, C., Maier, H., Mallon, A. M., Masuya, H., Melvin, D. G., Muller, W., Parkinson, H., Proctor, G., Reuveni, E., Schofield, P., Shukla, A., Smith, C., Toyoda, T., Vasseur, L., Wakana, S., Walling, A., White, J., Wood, J., and Zouberakis, M. (2007). Mouse Phenotype Database Integration Consortium: integration [corrected] of mouse phenome data resources. Mamm. Genome 18, 157–163.
Kaminuma, E., Mashima, J., Kodama, Y., Gojobori, T., Ogasawara, O., Okubo, K., Takagi, T., and Nakamura, Y. (2010). DDBJ launches a new archive database with analytical tools for next-generation sequence data. Nucleic Acids Res. 38, D33–D38.
Kwitek, A. E., Jacob, H. J., Baker, J. E., Dwinell, M. R., Forster, H. V., Greene, A. S., Kunert, M. P., Lombard, J. H., Mattson, D. L., Pritchard, K. A. Jr., Roman, R. J., Tonellato, P. J., and Cowley, A. W. Jr. (2006). BN phenome: detailed characterization of the cardiovascular, renal, and pulmonary systems of the sequenced rat. Physiol. Genomics 25, 303–313.
Morgan, H., Beck, T., Blake, A., Gates, H., Adams, N., Debouzy, G., Leblanc, S., Lengger, C., Maier, H., Melvin, D., Meziane, H., Richardson, D., Wells, S., White, J., Wood, J., De Angelis, M. H., Brown, S. D., Hancock, J. M., and Mallon, A. M. (2010). EuroPhenome: a repository for high-throughput mouse phenotyping data. Nucleic Acids Res. 38, D577–D585.
Mungall, C. J., Gkoutos, G. V., Smith, C. L., Haendel, M. A., Lewis, S. E., and Ashburner, M. (2010). Integrating phenotype ontologies across multiple species. Genome Biol. 11, R2.
Rubin, D. L., Lewis, S. E., Mungall, C. J., Misra, S., Westerfield, M., Ashburner, M., Sim, I., Chute, C. G., Solbrig, H., Storey, M. A., Smith, B., Day-Richter, J., Noy, N. F., and Musen, M. A. (2006). National Center for Biomedical Ontology: advancing biomedicine through structured organization of scientific knowledge. OMICS 10, 185–198.
Sayers, E. W., Barrett, T., Benson, D. A., Bolton, E., Bryant, S. H., Canese, K., Chetvernin, V., Church, D. M., Dicuccio, M., Federhen, S., Feolo, M., Geer, L. Y., Helmberg, W., Kapustin, Y., Landsman, D., Lipman, D. J., Lu, Z., Madden, T. L., Madej, T., Maglott, D. R., Marchler-Bauer, A., Miller, V., Mizrachi, I., Ostell, J., Panchenko, A., Pruitt, K. D., Schuler, G. D., Sequeira, E., Sherry, S. T., Shumway, M., Sirotkin, K., Slotta, D., Souvorov, A., Starchenko, G., Tatusova, T. A., Wagner, L., Wang, Y., John Wilbur, W., Yaschenko, E., and Ye, J. (2010). Database resources of the National Center for Biotechnology Information. Nucleic Acids Res. 38, D5–D16.
Serikawa, T., Mashimo, T., Takizawa, A., Okajima, R., Maedomari, N., Kumafuji, K., Tagami, F., Neoda, Y., Otsuki, M., Nakanishi, S., Yamasaki, K., Voigt, B., and Kuramoto, T. (2009). National BioResource Project-Rat and related activities. Exp. Anim. 58, 333–341.
Shimoyama, M., Petri, V., Pasko, D., Bromberg, S., Wu, W., Chen, J., Nenasheva, N., Kwitek, A., Twigger, S., and Jacob, H. (2005). Using multiple ontologies to integrate complex biological data. Comp. Funct. Genomics 6, 373–378.
Smith, B., Ashburner, M., Rosse, C., Bard, J., Bug, W., Ceusters, W., Goldberg, L. J., Eilbeck, K., Ireland, A., Mungall, C. J., Leontis, N., Rocca-Serra, P., Ruttenberg, A., Sansone, S. A., Scheuermann, R. H., Shah, N., Whetzel, P. L., and Lewis, S. (2007). The OBO Foundry: coordinated evolution of ontologies to support biomedical data integration. Nat. Biotechnol. 25, 1251–1255.
Smith, C. L., and Eppig, J. T. (2009). The mammalian phenotype ontology: enabling robust annotation and comparative analysis. Wiley Interdiscip. Rev. Syst. Biol. Med. 1, 390–399.
Smith, C. L., Goldsmith, C. A., and Eppig, J. T. (2005). The mammalian phenotype ontology as a tool for annotating, analyzing and comparing phenotypic information. Genome Biol. 6, R7.
Williams, R. R., Rao, D. C., Ellison, R. C., Arnett, D. K., Heiss, G., Oberman, A., Eckfeldt, J. H., Leppert, M. F., Province, M. A., Mockrin, S. C., and Hunt, S. C. (2000). NHLBI family blood pressure program: methodology and recruitment in the HyperGEN network. Hypertension genetic epidemiology network. Ann. Epidemiol. 10, 389–400.
Keywords: ontology, phenotype
Citation: Shimoyama M, Nigam R, McIntosh LS, Nagarajan R, Rice T, Rao DC and Dwinell MR (2012) Three ontologies to define phenotype measurement data. Front. Gene. 3:87. doi: 10.3389/fgene.2012.00087
Received: 14 February 2012; Paper pending published: 05 March 2012;
Accepted: 30 April 2012; Published online: 28 May 2012.
Edited by:
John Hancock, Medical Research Council, UKCopyright: © 2012 Shimoyama, Nigam, McIntosh, Nagarajan, Rice, Rao and Dwinell. This is an open-access article distributed under the terms of the Creative Commons Attribution Non Commercial License, which permits non-commercial use, distribution, and reproduction in other forums, provided the original authors and source are credited.
*Correspondence: Mary Shimoyama, Department of Surgery, Medical College of Wisconsin, 8701 Watertown Plank Road, Milwaukee, WI 53226, USA. e-mail: shimoyama@mcw.edu