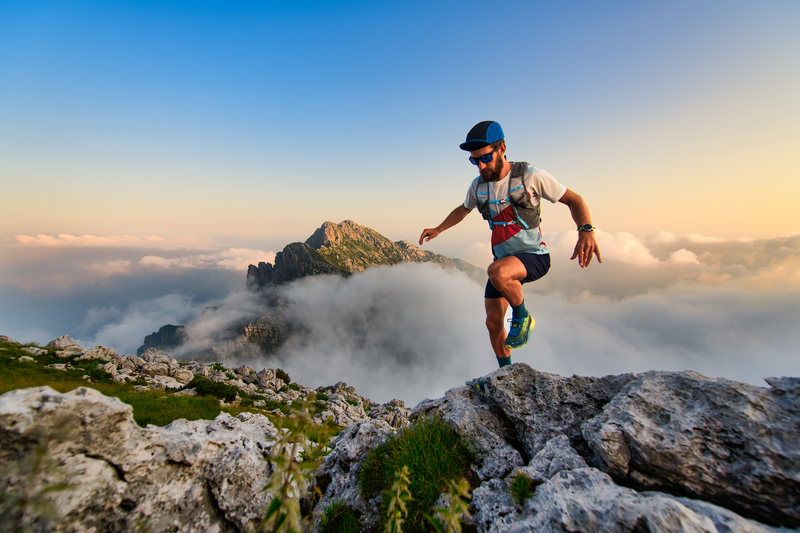
95% of researchers rate our articles as excellent or good
Learn more about the work of our research integrity team to safeguard the quality of each article we publish.
Find out more
REVIEW article
Front. Geochem. , 04 April 2025
Sec. Biogeochemistry
Volume 3 - 2025 | https://doi.org/10.3389/fgeoc.2025.1492386
Globally peatlands store 25% of global soil organic carbon but this large carbon store is at risk under climate change and from widespread anthropogenic disturbances. The impact of climate change on tropical peatlands, which represent 23%–30% of the global peatland area, is particularly poorly understood and Earth System Models do not yet include a suitable representation of the soil carbon cycle for tropical peatlands. Peat decomposition via soil heterotrophic respiration to CO2 (SHR-CO2) is a main component of the peatland carbon cycle. However, the lack of consensus on the importance of different drivers and the scarcity of empirical data hinders model development. Therefore, this study reviews the drivers of SHR-CO2 (moisture, temperature, decomposability and, nutrients and decomposers) for tropical peatlands. We compile available empirical data to inform model development; and highlight priorities for future experimental work that would enable further model refinement. We point out that the sharp decrease of SHR-CO2 under anoxic water-saturated conditions is a major parameter for tropical peat decomposition and the ratio of SHR-CO2 under anoxic conditions to the SHR-CO2 at the optimum moisture is 0.10 ± 0.08. Additionally, we highlight that, at present, the common assumption that SHR-CO2 doubles with an 10°C increase (Q10 of ca. 2) remains the most parsimonious option considering the lack of empirical data to establish a more process-based peatland SHR-CO2 temperature relationship. Finally, we identify three priorities to advance tropical peatland model improvement: (1) narrowing the constraint on the optimum moisture range for SHR-CO2 in tropical peatlands, (2) investigating the interaction between moisture and temperature sensitivity, and (3) identifying the most widely applicable metric to characterise peat decomposability that might enable quantitative comparison across the tropics.
Peat forms in waterlogged soils when plant production and the resulting litter addition rate exceeds the decomposition rate as anoxic conditions limit respiration and decomposition of the organic material (Chimner and Ewel, 2005; Blake et al., 2008; Vitt, 2013; Page and Baird, 2016). Water saturation controls oxygen availability, and oxygen limitation slows organic matter decomposition (Hirano et al., 2009; Loisel et al., 2021). Therefore, hydrology is one of the most important drivers of the carbon balance in peatlands (Hirano et al., 2009). Other controls on decomposition are temperature and decomposability. Higher temperatures, up to a point, increase peat decomposition rates (Gallego-Sala, 2008; Charman et al., 2013). Decomposition is also influenced by lability, i.e., how easily decomposable the peat is. Lability is in turn strongly controlled by the dominant parent peat-forming plant functional type (such as tree, shrub, graminoid, forb or moss) (Hodgkins et al., 2018; Uhelski et al., 2022).
Globally, peatlands cover ca. 3% of the global land area, yet store a disproportionate 25% of soil organic carbon (Parmesan et al., 2022). However, this large amount of carbon is at risk due to widespread anthropogenic disturbance and climate change, and this loss of peatland carbon globally already contributes to a positive climate feedback, accelerating climate change (Parmesan et al., 2022). Tropical peatlands are estimated to represent 23%–30% of the global peatland area but large uncertainties remain about their extent, carbon stock and about the main environmental drivers of tropical peat carbon cycling (Gallego-Sala et al., 2018; Ribeiro et al., 2021). In northern peatlands, an increase of the carbon sink is expected under warming conditions if sufficient moisture is maintained and there is no increase in cloudiness (Charman et al., 2013). However, observations suggest that in the tropics the peatland carbon sink will decrease under warmer conditions (Gallego-Sala et al., 2018). This is because it is expected that all the carbon loss mechanisms (higher temperatures, lower moisture, fires, land-use change and sea-level rise) will be amplified in the future (Loisel et al., 2021). However, the impact of climate change on tropical peatlands and their future remains poorly understood (Loisel et al., 2021; Ribeiro et al., 2021).
Most Earth System Models (ESMs) do not include peatlands and their dynamic feedbacks, and none in the latest Coupled Model Intercomparison Project phase (CMIP6) included a representation of peatlands (Arora et al., 2020; Chadburn et al., 2022). Since CMIP6, some of those ESM land surface components (JULES, ORCHIDEE and CLM5) were developed to include peatlands (Qiu et al., 2018; Qiu et al., 2019; Apers et al., 2022; Chadburn et al., 2022; Kwon et al., 2022). However, none include suitable representation of the soil carbon cycle for tropical peatlands as recent development focused on the northern peatlands’ soil carbon or hydrology. Further development is therefore needed to improve the representation of peatland carbon globally. Amongst the many specialised soil and peat models, the representation of tropical peatlands is also scarcer than northern or temperate peatlands (Table 1). Overall, few modelling studies focus on the tropical peatland carbon cycle because of the lack of available data for model parametrisation and validation (Hirata et al., 2016; Ribeiro et al., 2021).
Consequently, in this study, we review available data and the current state of knowledge on peat decomposition processes in the tropics. Peat decomposes to either CO2 and/or CH4 via soil heterotrophic respiration, and these gases are eventually lost to the atmosphere or to dissolved or particulate organic carbon that is lost laterally via streams or rivers (Wright et al., 2011; Lawson et al., 2015; Sihi et al., 2016; Ribeiro et al., 2021). This review specifically focuses on soil heterotrophic respiration to CO2 (SHR-CO2) and aims to (1) compile the existing knowledge on the drivers of SHR-CO2 in undisturbed tropical peatlands, (2) understand patterns and processes also using results from extra-tropical peatlands, and disturbed peatlands, (3) review how process-based models simulate peatland SHR-CO2 including a corresponding summary of existing observational data to inform parameter range choices for models, and finally (4) identify the gaps in the existing empirical data needed for future model improvements.
This review is composed of four sections, each focusing on a driver of peatland SHR-CO2 (moisture, temperature, substrate decomposability, nutrients), followed by one section on their interactions. Each section includes a summary of current knowledge and existing data, a summary of how these processes are accounted for in peat models and a discussion of future work needed.
The selection of models here does not aim to be an extensive review of peat process-based models as such a systematic review has already been conducted by Mozafari et al. (2023). Instead, the 16 models selected (Table 1) which simulate peatland soil organic carbon (SOC) decomposition, aim to be representative of the different types or families of models commonly used in the literature and include peat-specific models, highly organic or wetland soil models, soil-models and land surface models/dynamic global vegetation components of ESMs that include a peat module (Table 1). The models also have different spatial domains. LPX-Bern, Digibog, ecosys and Wetland-DNDC have been applied to boreal, temperate, and tropical or subtropical sites. However, most models do not simulate peatland soil carbon in all three bioclimatic regions. Models with a boreal-only published version are JULES-peat, LPJ-WHyMe, LPJ-GUESS, ORCHIDEE-peat, and Peatbog. ECOSSE, Millenia, MWM and peatland-VU have only been applied to temperate peatlands. CLASS-CTEM and CoupModel have been applied to boreal and temperate sites. Finally, there is a temperate and a tropical version of HPM.
Soil moisture and water table depth affect the soil microbial community structure and microbial activity, and in turn the organic matter decomposition (Jackson et al., 2009; Mäkiranta et al., 2009; Husen et al., 2014; Liu L. et al., 2016; Girkin et al., 2020a; Shabbir et al., 2024). In tropical peatlands, bacteria dominate the microbial community with the largest relative contribution to respiration (Husen and Agus, 2011; Dhandapani et al., 2019; Dhandapani et al., 2020; Girkin et al., 2020a). The bacterial community biomass and diversity peak at intermediate depths (Andersen et al., 2013; Dhandapani et al., 2020) while the fungi play a dominant role in decomposition in the upper oxic layers (Andersen et al., 2013; Girkin et al., 2020a). The presence of fungi is limited by the low redox potential at depth and in waterlogged conditions (Andersen et al., 2013; Girkin et al., 2020a). The fungi:bacteria ratio influences CO2 production as fungi produce less CO2 than bacteria per unit of cell mass therefore resulting in lower CO2 production rate per gram of microbial biomass (Li Q. et al., 2021). In permanently waterlogged anaerobic layers, the dominant pathway of peat decomposition is methanogenesis (Andersen et al., 2013).
Husen et al. (2014) observed that the number of microbial populations in moist and dry peat samples remained high, but their activity was inhibited with lower moisture. Dry conditions limit solute transport and therefore reduce microbial activity and microorganisms can become dormant under extremely dry conditions (Moyano et al., 2013; Yan et al., 2018). Conversely, high moisture leads to oxygen limitation and a reduction of microbial activity thus lowering rates of decomposition and CO2 production (Jackson et al., 2009; Mäkiranta et al., 2009; Moyano et al., 2013; Yan et al., 2018). A lower number of microbial populations has also been observed in saturated peat compared to non-saturated conditions as higher oxygen availability increases the quantity of microbes and enriches the structure of the microbial community (Husen et al., 2014; Liu L. et al., 2016).
Moisture is therefore one of the principal controls of SHR-CO2 and the relationship between the two has been studied in depth and modelled for other non-peat types of soils (Moyano et al., 2012; Moyano et al., 2013; Yan et al., 2018). Available publications and data on tropical peatlands have only evaluated SHR-CO2 under 2 or 3 moisture treatments (Brady, 1997; Inglett et al., 2012; Hoyos-Santillan et al., 2015; Hoyos-Santillan et al., 2016; Hoyos-Santillan et al., 2019; Sjögersten et al., 2018; Young et al., 2023). To the best of our knowledge, Van Lent et al. (2019) is the only paper to report a fitted function of SHR-CO2 across moisture conditions from 25% to 100% saturation and flooded conditions for an intact tropical peatland. They were able to fit a cubic polynomial to the data for their intact site in the Peruvian Amazon. They observed a significantly lower CO2 production for the intact site across the water filled pore space (WFPS) treatments compared to their two degraded sites. However, when scaled to their respective maximum, the SHR-CO2 curves with moisture are similar and overlap for all three levels of degradation. Some additional SHR-CO2 rates across the moisture range and fitted SHR-CO2-moisture functions are also available for four degraded/cultivated tropical peatlands (Husen et al., 2014; Van Lent et al., 2019), for some Canadian temperate and boreal sites (Hogg et al., 1992; Byun et al., 2021) and a mountain peatland (Wang X. et al., 2010). Although these studies fitted polynomial functions of various degrees (quadratic being the most common), the fitted functions are all bell-shaped with some flatter parabolas. These various polynomial functions show a steeper decline of CO2 flux in low and high moistures conditions compared to the cubic polynomial curve fitted for the intact tropical site (Van Lent et al., 2019). Nevertheless, this does not necessarily mean there is a significant difference in the processes behind the SHR-CO2 between those different sites as those studies used different experimental settings. The shape of the curve at high moisture conditions is influenced by whether the incubations were completely anoxic or not. Most incubations reported here did not include flushing the incubated samples and therefore some oxygen might have still been present at saturation leading to a higher CO2 flux. On the other end the lowest moisture treatment in each incubation experiment also influences the resulting moisture-SHR-CO2 function shape. The driest incubations treatment amongst the studies varies between near 0% WFPS to 25%WFPS. Notably Van Lent et al. (2019) considered moisture treatments above 25%WFPS, additional measurements in drier conditions might draw the function towards a in a steeper bell-shaped curve.
These bell-shaped curves are characterised by an optimum moisture level and are more or less concave depending on the relative values of SHR-CO2 at 0% and 100% saturation (Figure 1). The relative SHR-CO2 (unitless ratio) at 0% moisture is of relative unimportance for intact peatlands as they are generally characterised by wet soils and rarely approach completely dry conditions. This might become more important for degraded peatlands and/or those exposed to prolonged droughts. Conversely, the relative SHR-CO2 at saturation, also referred to as the ‘moisture resting point’ herein (unitless), is of key importance for carbon accumulation in peatlands. So, the impact of moisture on SHR-CO2 for peatlands can be characterised by two critical values: the optimum moisture level for maximum SHR-CO2 and the relative reduced respiration at saturation (or moisture resting point) (Figure 1).
Figure 1. Moisture - soil heterotrophic respiration to CO2 schematic curves. SHR-CO2 ratio (unitless) is the relative SHR-CO2 at each moisture content relative to the maximum respiration at the optimum moisture content. The optimum moisture is the moisture (% saturation) at the maximum SHR-CO2 ratio. The moisture resting point is the value of the SHR-CO2 ratio at saturation.
Since SHR-CO2 rates drop under both low and high moisture, there is, in between, an optimum moisture condition at which the SHR-CO2 rate reaches a maximum (Figure 1). The position of this optimal moisture level varies with soil type and with soil characteristics such as porosity and soil organic matter content.
According to Moyano et al. (2012), the optimum moisture in inorganic and organic soils ranges between 0.4 and 0.9, and the response of respiration to moisture is significantly correlated to soil properties for mineral soils unlike for organic soils. Yan et al. (2018) developed a moisture function of soil heterotrophic respiration for a range of soil types and with measurable parameters. The function was evaluated and calibrated on incubation data from a range of soil types (but not including peat) and provides an insight into the effects of soil properties on the relationship between SHR-CO2 and moisture. Analytical and simulated results show that the optimum water content increases with clay content and porosity but decreases with soil organic carbon. However, SHR-CO2 was not sensitive to the latter in highly organic soils (Yan et al., 2018). Considering that peat is carbon-rich, the optimum water content for peat soil should not be linked to carbon content but could remain significantly related to porosity.
Incubation of peat samples from boreal and temperate sites in Canada showed a CO2 flux maxima between 59% and 95% water filled pore space (WFPS) (Byun et al., 2021) (Figure 2A). Hogg et al. (1992) report optimum moisture between 44 and 61 mean % saturation for another Canadian boreal peatland site. Husen et al. (2014) and Husen and Agus (2011) report optimum moisture of 24% WFPS for subsurface peat (30–50 cm) and of 52% and 66% WFPS for surface (0–20 cm) drained peat palm oil plantations in Indonesia (Figure 2A). For shallow surface samples (0–6 cm) (Comeau et al., 2021) observed an optimal moisture falling within the 20–40%WFPS interval for their primary forest and oil palm plantation sites and 40–60%WFPS for their drained forest site. Van Lent et al. (2019) measured optimum moisture of 73, 71% and 62% WFPS along a gradient of peatland degradation from intact to high degradation in the Peruvian Amazon.
Figure 2. (A) Optimum moisture for SHR-CO2 from intact peatlands in Canada (n = 28) (Byun et al., 2021; Hogg et al., 1992), one intact and three disturbed peatlands in Indonesia (n = 5) (Husen et al., 2014; Comeau et al., 2021) and a gradient of peatland degradation in the Peruvian Amazon (n = 3) (van Lent et al., 2019) (ANOVA, F = 17.05, df = 4, p < 0.001, letters indicate significant differences Tukey HSD p < 0.05). (B) Moisture - SHR-CO2 curves and envelope from above mentioned studies (except from Comeau et al. (2021) as they did not fit a moisture curve in their study).
There is a significant latitudinal trend for optimum moisture (absolute value of latitude (decimal degrees), Pearson’s correlation coefficient = 0.53, p < 0.001) across the data from the five optimum moisture studies (Hogg et al., 1992; Husen et al., 2014; Van Lent et al., 2019; Byun et al., 2021; Comeau et al., 2021). However, considering the small sample size which also include various degrees of degradation, this relationship is not necessarily robust. Optimum moisture is significantly different between degradation levels (ANOVA, F = 6.507, df = 2, p < 0.005) (Figure 2B). The optimum for the four drained sites is significantly lower than for intact sites (n = 30), but there is no significant difference between harvested (n = 2) and drained and harvested and intact. This suggests that optimum moisture might be different between pristine and degraded sites but remains uncertain as the number of disturbed sites in the compilation is very low. This higher degradation associated with lower optimum moisture WFPS values is congruent with Yan et al. (2018), as peat porosity (inverse relationship with bulk density) decreases with drainage and degradation. However, there is no significant correlation between the bulk density and the optimum moisture across the compiled moisture data (p > 0.05, n = 36). More experimental data would be necessary to clarify if a general relationship for mineral, organic carbon-rich and peat exists.
For comparison purposes, the SHR-CO2 at saturation (unitless) is expressed here as a fraction of the maximum SHR-CO2 at the optimum moisture.
To the best of our knowledge, van Lent et al. (2019) is the only study reporting a fitted function of SHR-CO2 across the moisture range for one intact and two degraded tropical peatland sites, leading to MRP values between 0.56 and 0.85. Other studies did not fit a function across the moisture range and or only measured SHR or litter decomposition under two or three moisture conditions and oxygen treatments (defined as % saturation moisture, above/below the water table, anoxic, oxic or flooded oxic). This does not allow the calculation of the moisture resting point (MRP) as the maximum SHR-CO2 at the optimum moisture is unknown. However, an upper estimate of the moisture resting point can be obtained substituting the SHR-CO2 at the optimum moisture in this ratio with the available SHR-CO2 from the drier or oxic treatment (Figure 3; Supplementary Table S3).
Figure 3. Upper estimate of the moisture resting point (MRP) (unitless) (i.e., ratio of SHR-CO2 between treatments) for intact tropical peatlands [Brady, 1997 (n = 15); Inglett et al., 2012 (n = 15); Hoyos-Santillan et al., 2015 (n = 12), 2016 (n = 36), 2019 (n = 2); Sjögersten et al., 2018 (n = 8); van Lent et al., 2019 (n = 1); Young et al., 2023 (n = 6)] (ANOVA, F = 53.49, df = 7, p < 0.001, letters indicate significant differences Tukey HSD p < 0.05).
The upper estimate of the moisture resting point is highly variable, but this can be explained by the differing methodological approaches of the studies which limits their comparability (length, sample material, in situ or ex-situ, CO2 flux vs. decay) (Figure 4A; Supplementary Table S3). Common methods to study peat (or litter) decomposition are litter bag experiments (based on mass loss), surface or depth gas flux measurements in situ, or incubation experiments (gas fluxes) ex situ. Litter bag experiments can slightly overestimate the decomposition coefficient as there can be some losses during the experiment set up (Lam et al., 2021). Small fragments of leaf litter can easily be lost during collection and washing through the mesh when they are highly decomposed. A small mesh size relative to litter fragments can reduce this bias (Lam et al., 2021). In situ gas measurements at the peat surface do not give a total instantaneous gas production estimate for the whole peat profile but a flux that is dependent on transport also. This may be a relevant nuance to keep in mind depending on the temporal and geographic scale of the model.
Figure 4. Upper estimates of the MPR (unitless) (i.e., ratio of SHR-CO2 between treatments) for intact tropical peatlands (A) by measurement method (n = 75, 2 and 18, ANOVA F = 3.294, df = 2, p < 0.05, Tukey HSD pair-wise differences insignificant p > 0.05) and (B) by moisture-oxygen effect studied for the incubation group (n = 40, 16, and 19). The resting point for the water saturation effect group is significantly higher than for the two other treatments (ANOVA F = 160.7, df = 2, p < 0.001, and subsequent Tukey’s test p < 10−7, letters indicate significant differences). Anoxia group: ratio of SHR-CO2 between an anoxic sample and its corresponding oxic sample under saturated conditions. Water-saturation group: ratio of SHR-CO2 between water saturated sample and a corresponding unsaturated sample all under oxic conditions. Anoxia and water-saturation group: ratio of SHR-CO2 between an anoxic and water-saturated sample and a corresponding oxic and unsaturated sample.
The in situ gas measurements by Hoyos-Santillan et al. (2019) carried out with a fluctuating water table depth, give an estimate of the CO2 in the peat column when the water table is above (>5 cm) or below (<−5 cm) the peat surface. This study highlights that CO2 fluxes measured in situ using a chamber show a significant relationship with water table depth. However, even in conditions where the water table is 5 cm below the surface, some of the peat column remains saturated at depth and this method therefore underestimates the ratio between water-saturated and drier conditions (i.e., the moisture resting point).
The second methodological group of studies are the litter bag experiments (Hoyos-Santillan et al., 2015; Young et al., 2023) (Figure 4A). This experimental setup can overestimate the decomposition, but it also measures total carbon losses via total mass loss whereas the in situ and ex situ gas measurements differentiate the CO2 from CH4, DOC and POC. The two studies available for tropical peatlands both estimate the decomposition of leaf, stem and root litter at the surface and at 50 cm depth. The two depths are assumed to represent drier conditions above the water table and saturated conditions under the water table. However, the difference between oxic (surface litterbags) and anoxic (50 cm depth litterbags) can be underestimated as those conditions are not fully maintained because of seasonal flooding and drying (even at 50 cm depth) of the peatlands, therefore skewing further the resulting dry/saturated ratio towards high values. The decomposer community is also a confounding factor when comparing oxic and anoxic decomposition with this method as the microbial community composition and size changes with depth and litter type (Jackson et al., 2009; Andersen et al., 2013; Li et al., 2021). Therefore, the comparison of the decomposition in the surface and subsurface litter bags does not simply represents the decomposition difference between contrasting moisture conditions but also includes the effect of the microbial and macro decomposer or detritivore communities. Despite the limited validity of the dry/saturated ratios for comparison with the litter bag method, Hoyos-Santillan et al. (2015) concluded that the decline of oxygen with depth due to saturation is the main control of litter decomposition, in agreement with the in-situ gas measurements (Hoyos-Santillan et al., 2019).
Finally, the third methodological group are ex situ incubations studies (Brady, 1997; Inglett et al., 2012; Hoyos-Santillan et al., 2016; Sjögersten et al., 2018) (Figure 4A). These studies constitute the group with the lowest upper estimates of the moisture resting point with a median of 0.126. However, it still presents a large variability and with many outliers despite the controlled laboratory conditions which avoid the limitations highlighted for the litter bag and in situ measurements. The large variability and presence of outliers can be explained by the difference in treatments between the incubation experiments and the entangled effect of moisture and oxygen availability. The incubation studies had mainly three water-oxygen treatments: 1) saturated and anoxic (100%WFPS or flooded and N2 flushed microcosms), 2) saturated but not fully anoxic or oxic because the saturation threshold is sometimes defined as <100%WFPS and/or the microcosms were not flushed with N2 (therefore the water in the samples remain more or less oxygenated) and/or even actively maintained oxic using shaking with a headspace containing oxygen and 3) not saturated oxic (<100%WFPS).
The ratio of the first and second type of treatments isolates the impact of oxygen availability on heterotrophic respiration (Figure 4B, effect of anoxia). The ratio of the second and third types of experiments provides information on the impact of water saturation (and maybe associated decrease in oxygen availability in the essays where the oxic conditions were not maintained on purpose) (Figure 4B, effect of water saturation) and the ratio of the first and third groups of experiments shows the impact of water saturation and anoxia combined. SHR-CO2 decreases slightly with saturation in oxic conditions but significantly more with anoxia, whether in water saturated condition or not (Figure 4B) (ANOVA, F = 160.7, df = 2, p < 0.001, TukeyHSD p < 0.001). However, the impact of anoxia and the combined impact of anoxia and water saturation are not significantly different for the moisture resting point (p > 0.05). This suggests that the assumption that saturation of the peat is equivalent to anoxia in incubation experiments may not always be correct. However, assuming that high water tables eventually lead to anoxia is still a useful approximation for models as we can assume that over time, oxygen in the peat water is consumed in aerobic metabolic processes so after a time, saturation equates anoxia. The median upper estimates of the moisture resting point for anoxia (group 1) and anoxia and water saturated (group 3) is 0.105 ± 0.078, and the range is 0.005–0.290 with one outlier at 0.446 (Figure 4B). Including incubation data for undisturbed temperate and boreal peatlands (Supplementary Table S4) leads to similar overall values (median 0.120, range 0.005-0.302 with outliers up to 0.693 which are potentially due to imperfect anoxic incubation conditions).
The most common type of function used to account for the impact of moisture on SHR-CO2 is bell-shaped, in agreement with observed relationships. ORCHIDEE-peat, LPJ-GUESS and HPM use a bell-shaped curve that uses a single smooth function (Figure 5). Other models use a roughly bell-shaped composite function, with multiple individually defined sections for different moisture or water table depth intervals (JULES-peat, MWM, Peatbog, CoupModel, ECOSSE) (Figure 5). Some models account for moisture via the respiration base rates, with different parameter values for the oxic acrotelm and anoxic catotelm or use a constant anoxic factor (LPJ-WHyMe, CLASS-CTEM, MILLENNIA and Digibog). LPX-Bern uses a combination of the two approaches mentioned above depending on the position in the peat profile. Finally, some models rely on more complex formulations: PEATLAND-VU, ecosys, and WETLAND-DNDC isolate the influence of moisture and oxygen or redox potential availability into two distinct functions.
Figure 5. Moisture - SHR-CO2 curves (Hogg et al., 1992; Husen et al., 2014; van Lent et al., 2019; Byun et al., 2021) and moisture functions from the peat models when applicable. For JULES-Peat the displayed envelope indicates the range of values found in a typical boreal peat profile. The models not represented on the graph are relying on water table depth or consider only an oxic and anoxic zone (MILLENNIA, DigiBog, CLASS-CTEM, LPJ-WHyMe, LPX-Bern), soil water potential (ecosys, PEATLAND-VU), or require information on soil hydraulic properties (ECOSSE) or three locally fitted parameters (CoupModel).
The resulting optimum moisture conditions in models, where applicable, range between 45% (LPX-Bern for the acrotelm) and 75% (LPJ-GUESS) (Figure 5). Some consider there is a plateau of maximum SHR-CO2, defined between field capacity and −100 kPa of matrix potential (ECOSSE) or 80% saturation (PEATLAND-VU) or between 20% and 77% saturation (CoupModel). Finally, in JULES-peat the position of the maximum SHR-CO2 plateau is a function of the soil wilting point. The latter can either be set as a parameter or to vary with soil characteristics and a higher wilting point will lead to maximum decomposition at higher moisture levels.
The moisture resting point across models varies between 0 (PEATLAND-VU) and 0.35 (LPX-Bern acrotelm). In models with a more complex formulation, the resting point is also sometimes considered as a function of redox potential (0.8–0.2 with decreasing redox potential in WETLAND-DNDC) or depth below the water table (from 0.3 to 0.001 in HPM).
Our review of the moisture sensitivity of SHR-CO2 in tropical peatlands indicates that the optimal moisture varies within a large range and might be a function of soil properties, and that the respiration at saturation only drops in controlled anoxic conditions. The moisture impact on SHR-CO2 is usually modelled as a bell-shaped curve which is characterised by two key parameters: the optimum moisture and the moisture resting point (Figure 1).
The impact of moisture on SHR-CO2 is intertwined with the impact of oxygen availability. Saturation alone without oxygen limitation only slightly decreases SHR-CO2, as oxygen dissolved in the water is still available for aerobic decomposition. On the other hand, anoxia strongly limits SHR-CO2 whether the microcosms were water saturated or not. The bell-shaped curve function observed is a result of both moisture and oxygen availability. Moisture resting point estimates, assuming water saturation equals anoxia, ranged between 0.005-0.290 with a median of 0.105.
The optimum moisture of degraded peatlands with higher bulk densities was lower than for intact peatlands. This is in agreement with the relationship between porosity and optimum moisture observed by Yan et al. (2018) for a range of non-peat soils. The mean optimum moisture in intact global peatlands is 68% saturation (range 44–95 %saturation).
Despite this insight into moisture sensitivity, constraining the relationship of optimum moisture and SHR-CO2 in a more sophisticated way in models using soil properties and a distinct oxygen and moisture functions for tropical peatlands is still not possible due to the scarcity of incubation data for peat soils. Considering the current state of knowledge, the use of a simple assumption of anoxia with water saturation and a constant optimal moisture remains a more parsimonious solution for peat models. Moving forwards, further SHR-CO2 incubation measurements of peat soils across the moisture range (for instance at 20% WFPS intervals) are needed and including detailed soil sample properties, such as bulk density or porosity, and the oxygen level at which the measurements are made would also be valuable. This would provide the necessary evidence to refine models on the optimum moisture conditions for tropical peat soils and shed light on a potential maximum respiration plateau.
Temperature response and sensitivity of soil heterotrophic respiration is one of the main uncertainties in our understanding and predictions of the global carbon cycle (Carey et al., 2016; Booth et al., 2017; Intergovernmental Panel on Climate Change, 2023).
Amongst the various approaches to quantify the influence of temperature on soil carbon decomposition, Q10 is a widely used metric that allows comparison between studies (e.g., Hamdi et al., 2013; Kirschbaum, 1995; Lloyd and Taylor, 1994; Wu Q. et al., 2021). Q10 for SHR-CO2 is defined as the proportional change in respiration when the soil is exposed to a 10°C increase in temperature:
where
There are several methods to extract Q10 values of soil carbon decomposition from incubation experiments (Hamdi et al., 2013; Liang et al., 2015; Wang Q. et al., 2019; Byun et al., 2021) and in particular, the equal-time and the model-fit methods are the most common. The equal-time method relies on the comparison of fluxes from two samples incubated at different temperatures after an equal-time incubation or one sample incubated at different temperatures for a given time (Q10 = (Chigh ∕Clow) [10/(Thigh−Tlow)]) (Hamdi et al., 2013; Wang Q. et al., 2019; Byun et al., 2021). In the model-fit method, Q10 is a fitted model parameter. Again, with the data either coming from multiple samples each incubated a specific temperature or from one sample incubated under various temperatures over a given time. Several models are generally used (such as one-pool model, or two or three carbon pools) each with different assumptions (Hamdi et al., 2013; Liang et al., 2015). Although less common, Q10 can also be derived from the activation energy obtained with the Arrhenius function.
Liu et al. (2016) and Shabbir et al. (2024) both observed a positive impact of temperature on the structure and size of microbial communities in mountain peatlands. For a temperate peatland, Li et al. (2021) found an optimum temperature for CO2 production rate per gram of microbial biomass between 24°C and 28°C. However, the response of the microbial community to temperature also depends on the time-scale of warming as the microorganism can potentially acclimatise (Andersen et al., 2013; Sihi et al., 2018).
A general rule of thumb is that an increase of 10°C typically doubles the rate of reaction (Davidson and Janssens, 2006; Sadaca et al., 2012), and the evidence suggests this simple approximation may not be far from observed temperature sensitivity. Hamdi et al. (2013) compiled Q10 data across ecosystems for soil organic decomposition and after normalising for temperature and averaging across incubation time, found a mean Q10 value of 2.04 ± 1.09 (n = 317). In an another similar compilation, Wang et al. (2019) obtained a mean Q10 of 2.41 (n = 1728, range 0.50–9.70). Q10 is often set to a constant value of 2 for organic matter decomposition, however this generalization has been questioned by the results of field and laboratory measurements which show varying values regionally and with soil type (Hamdi et al., 2013; Laub et al., 2021; Wu Q. et al., 2021).
Estimates of Q10 values from undisturbed tropical peat samples available in the literature (n = 69) range between 0.85 and 3.10 with a few outliers between 7.40 and 11.93 (Figures 6A, B) (Brady, 1997; Inglett et al., 2012; Sihi et al., 2016; Sihi et al., 2018; Sjögersten et al., 2018; Maie et al., 2019; Flanagan et al., 2020; Girkin et al., 2020b). The median value is 1.84. The Q10 estimates were measured under various moisture and oxygen availability treatments as well as peat decomposability types which might explain part of the variability. Comparison is also restricted because of methodological differences between studies.
Figure 6. (A) Q10 estimates from incubation experiments of intact tropical peatland per region from 8 studies (Brady, 1997; Inglett et al., 2012; Sihi et al., 2016; Sihi et al., 2018; Sjögersten et al., 2018; Maie et al., 2019; Flanagan et al., 2020; Girkin et al., 2020b). There is no no significant difference between groups (ANOVA, F = 1.108, df = 4, p > 0.05). And outliers (n = 6, range : 7.40 - 11.93) are not shown on graph. And (B) resulting reaction rate ratio for the corresponding range of Q10 from these incubations (for a 20°C reference temperature).
Estimates of Q10 values from peatlands globally (n = 873, from 44 incubation studies, see Supplementary Table S5 for details) range between 0.24 and 12.10 with 90% of the estimates falling between 1.16 and 3.62 and a median value of 2.04. The number of available estimates for boreal and temperate peatlands (n = 463 and 329 respectively) is much higher than for tropical peatlands (n = 81 disturbed and undisturbed). Also, there are no estimates for Africa and very little data for the Southern hemisphere (Figure 7). When aggregated into biomes, only the boreal and temperate groups are significantly different (ANOVA, F = 9.843, df = 2, p < 0.001, Tukey HSD temperate-boreal p < 0.001). No geographic pattern is clearly visible (Figure 7), and even though there is a significant linear relationship between latitude and log10 (Q10) (df = 871, F = 8.986, p < 0.005), it is a very weak relationship (R2adjusted = 0.009).
Figure 7. Global peatland Q10 estimates from incubation of peatland soils classified by 20% quantiles. Background tile: Esri, DeLorme, NAVTEQ.
Liu H. et al. (2024) also observed a significant positive linear relationship at the global scale for peatlands between Q10 (log10) and latitude (R2adjusted = 0.29). However, the relationship observed in this study, beyond having a lower R2, also has a much lower slope (see Supplementary Figure S2.2). This could be due to differences between datasets. Despite the important overlap, Liu H. et al. (2024) included in situ measurements whereas only incubation studies were included in this study. This emphasizes the remaining uncertainty surrounding how much of the data variability is due to intrinsic temperature sensitivity differences and the processes of soil organic matter (SOM) decomposition versus how much is due to noise resulting from methodological differences including peat samples characteristics and incubation treatment differences between studies.
Despite being widely used, using a constant Q10 across a large temperature range is an oversimplified assumption to describe SOC decomposition and does not describe accurately its temperature sensitivity (Davidson and Janssens, 2006; Hamdi et al., 2013; Wu Q. et al., 2021). Q10 does not rely on any theoretical background or account for the underlying mechanisms of SOC decomposition (Kirschbaum, 1995; Wu Q. et al., 2021). The assumption that reaction rates increase exponentially with temperature may be a reasonable simplification applicable to elementary reactions over a small temperature range, but is of limited applicability for more complex processes (Wu Q. et al., 2021).
Soil carbon decomposition rates can vary with time and therefore different incubation durations can result in different Q10 values, even for the same reaction (Hamdi et al., 2013; Wu Q. et al., 2021). In our compilation of peatland Q10 estimates, the incubation length ranges from a few hours to 600 days which limits the comparability of these studies, as longer incubations results are more likely to be affected by substrate limitation than short incubations. Q10 values will initially decrease with time due to differing rates of decomposition between labile and recalcitrant pools as their relative size varies with the duration of incubation. Therefore, comparing incubations of different duration may not be informative, as the average substrate quality is likely to be different for incubations of different length, which will lead to different apparent temperature sensitivities (Hamdi et al., 2013; Liang et al., 2015). However, in Hamdi et al. (2013) dataset, Q10 values derived from the equal-time method did not differ significantly when compared to values derived from other methods. Our Q10 data compilation includes estimates derived from various calculation methods, but the equal-time methodology is the most common. There is a significant linear relationship between Q10 and the incubation duration (df = 860, F = 10.1, p < 0.005), but the explanatory power of the relationship is very low (slope = −0.00085, R2adjusted = 0.01) (see Supplementary Figure S2.3). The relationship between incubation duration and Q10 observed here for peat soils does not agree with prior observations for other soil types (Hamdi et al., 2013) of a convex quadratic relationship. But a notable limitation of this review is the scarcity of long incubation studies.
Q10 values may be useful when considering small temperature ranges (Hamdi et al., 2013), however it is a relative measure of temperature sensitivity and is itself temperature dependent, so may be a skewed metric if used over a larger range of temperatures (Davidson and Janssens, 2006; Hamdi et al., 2013; Sihi et al., 2016; Wu, Q. et al., 2021). Q10 values decrease with average incubation temperature across ecosystems until 20°C–25°C where it remains fairly constant (ca. 2) (Kirschbaum, 1995; Hamdi et al., 2013; Wang Q. et al., 2019). The results for peatland soils agree with those studies on global SOM decomposition as our peat incubation compilation suggests that there is a significant relationship between Q10 and the average incubation temperature (quadratic relationship for the natural logarithm of Q10 (df = 871, F = 10.94, p < 0.001)). However, this relationship is weak (R2adjusted = 0.022) (see Supplementary Figure S2.4; Supplementary Table S1).
Finally, peat decomposition response to temperature is dependent on the way temperature increases are applied (Updegraff et al., 1998; Gallego-Sala, 2008; Sihi et al., 2018). Many temperature sensitivity laboratory studies either compare two sub-samples exposed to two different temperatures (Updegraff et al., 1995; Thomas et al., 1996; Brady, 1997; Chapman and Thurlow, 1998; Waddington et al., 2001; Dioumaeva et al., 2002; Wang X. et al., 2010; Hardie et al., 2011; Inglett et al., 2012; Szafranek-Nakonieczna and Stêpniewska, 2014; Ye et al., 2016; Sjögersten et al., 2018; Girkin et al., 2020b) or modify the temperature of incubation stepwise on the same sample (McKenzie et al., 1998; Yavitt et al., 2000; Koch et al., 2007; Hilasvuori et al., 2013; Sihi et al., 2016; Byun et al., 2021; Li Q. et al., 2021). Unlike step increases or incubation comparisons of two different temperature (usually 5°C or 10°C apart), in-situ temperature changes in peatland soils are gradual. Gallego-Sala (2008) compared a 5°C step increase, a sinusoidal and ramp temperature increase paths. The sinusoidal temperature profile (mimicking the diurnal temperature fluctuations that we might expect in situ) yielded significantly lower Q10 values than the step profile. Sihi et al. (2018) compared step versus a ramped (0.1°C/day) increase of temperature from 15°C to 25°C. They observed a continuous increase in gas production in the ramp treatment (over the 120 days experiment length) but a stabilisation of production rates after ca. 3 weeks in the step treatment. In the slow warming treatment, microorganisms were able to buffer their functioning as evidenced by the fact that their functioning indices (carbon use efficiency, biomass carbon and stable carbon isotopes of CO2 and CH4) were close to those of the control treatment. Total CO2 production was higher in the ramp treatment and therefore resulted in higher Q10 values.
The Arrhenius function is an alternative approach for quantifying the temperature sensitivity of SHR-CO2. Unlike Q10, the Arrhenius function has a theoretical basis (Kirschbaum, 1995; Gudasz et al., 2021). Following Arrhenius’ equation, the soil respiration is a function of
It is also applicable to the whole range of temperatures where enzymes are functional. Unlike the Q10 approach, it is does not face the incubation duration and temperature path dependent response. Furthermore, it would be possible to apply the Arrhenius approach to the existing database if provided with the raw data. However, this is a metric that is not usually reported in incubation experiments.
The most common temperature type function used in the reviewed models is a Q10 type with each model using a slightly different variation of the basic Q10 exponential function. DigiBog uses a Q10 of 2.5 or 3 depending on the model version. Calibrated Q10 values in CoupModel range from 1.95 to 3.5. LPJ-GUESS, ORCHIDDEE and MWM all use a Q10 of 2 for temperatures above freezing. Below freezing, the temperature modifier decreases to zero at −1°C (LPJ-GUESS) or −4°C (ORCHIDEE and MWM). In PEATBOG, the labile SOC is given a lower Q10 than the recalcitrant SOC (2.3 and 3.3). Finally, the Q10 value is a function of the soil layer temperature in CLASS-CTEM, starting with a value just under 2 and very slowly decreasing. The other two temperature functions used in more than one of the reviewed models are an Arrhenius-type function (LPJ-WHyMe, LPX-Bern, PEATLAND-VU, ecosys) and the RothC temperature function (JULES-peat, ECOSSE). The RothC temperature function is an empirical function developed for temperate arable land in England (Jenkinson, 1990; Coleman and Jenkinson, 2014). For the Arrhenius function, PEATLAND-VU sets the reference temperature to 284 K and the activation energy between 96 and 126 kJ mol−1. LPX-Bern, LPJ-WHyMe and ecosys used a modified version of the Arrhenius equation parametrised for their specific sites.
Most models’ temperature functions are within the range of the empirical reaction rates calculated from the global Q10 data compilation (Figure 8). PEATLAND-VU uses the highest temperature sensitivity out of all the temperatures functions as it applies activation energy values that are much higher than, for instance, those in ecosys (one of the models with low temperature sensitivity), and also much higher than the activation energy estimates from our data compilation [n = 47, mean 37 ± 31 kJ mol−1 (Chapman and Thurlow, 1998; Yavitt et al., 2000; Gallego-Sala, 2008)]. DigiBog also reaches very high ratio of reaction rates for temperatures above 25°C because of the low base temperature they use (mostly from applications of the model to the boreal biome). Unlike some other models which are global, DigiBog has several regional versions, and the low base temperature is only used in regions with low temperatures. This highlights one of the limitations of using Q10, as the choice of the base temperature (at which the reaction rate ratio is 1) can lead to very different results. Conversely, both ORCHIDEE-Peat and Wetland DNDC show very low reaction rates even at high temperatures. The former rely on a high base temperature for Q10 (30°C), and the later uses a linear temperature function (fT = T/30) for temperatures below 30°C.
Figure 8. Variation of peat SHR-CO2 reaction rate ratio with temperature. (A) Global peat incubation data compilation, reaction rate ratio calculated based on the experiment base temperature (for equal-time Q10 method) or on the incubation mean temperature (for Arrhenius and model-fitting Q10 method) and only shown over the temperature range of the corresponding incubation. (B) Peat model temperature functions or range across versions or fitted parameter ranges. PEATLAND-VU goes up to 65. MILLENNIA and CLASS-CTEM are not represented as they require extra information on the site-specific temperature profile.
Isolating the temperature sensitivity of SHR-CO2 remains ambiguous as it is difficult to disentangle real variation in temperature responses from the noise due to methodological differences, characteristics of the peat samples (decomposability and substrate availability) and moisture conditions. Despite its limitations, Q10 is the most convenient metric to investigate temperature sensitivity of SHR-CO2 because of its widespread use in incubation and field experiments. The median of the global peatland Q10 measurements collated in this study was 2.04 (with 90% of observations between 1.16 and 3.62). The spread of those estimates over a large range of values could be explained by the various moisture and oxygen availability treatments across experiments as well as peat decomposability and methodological differences between studies such as incubation time or temperature range used to estimate the reported Q10 values.
This suggests that the frequent use of a constant Q10 of ca. 2 in peat models seems to be a reasonable simplification for lack of a better alternative at present.
Further empirical investigations maintaining all confounding factors (moisture, decomposability) constant would allow isolating the influence of temperature and would help to constrain a SHR-CO2 base rate. It would also be beneficial to adopt an approach based on underlying mechanisms of soil organic carbon decomposition such as an Arrhenius response. Existing incubation data could easily be used if provided with raw data to calculate and report an Arrhenius response without the need of further incubation experiments.
There is a variety of definitions of decomposability, not all linked to quality (Conant et al., 2011). SOM quality characterises how easily soil organic carbon can be mineralised, so a higher quality means more labile SOM. To link quality to thermodynamics, Bosatta and Ågren (1999) considered SOM quality as “the number of enzymatic steps required to release as carbon dioxide a carbon atom from an organic compound”. Therefore quality can be defined as the recalcitrance of SOM due to its molecular structure characteristics (Conant et al., 2011; Hoyos-Santillan et al., 2016). However, the decomposability of soil organic matter results from several independent processes. A more explicit description of soil organic matter resistance would include quality and physio-chemical protection (Conant et al., 2011).
There is not a single index to characterise a sample SOM quality (Bosatta and Ågren, 1999). B is one commonly used index of substrate quality which includes both availability and lability–a higher B index indicates a higher substrate quality (Fierer et al., 2005; Koch et al., 2007; Gallego-Sala, 2008; Inglett et al., 2012). B is a fit parameter from the equation describing an assumed exponential response of decomposition rates with temperature.
where
Depth and CO2 fluxes have been shown to be significantly related in some tropical peatlands (Brady, 1997; Hoyos-Santillan et al., 2016; Sihi et al., 2016) suggesting that decomposability plays a role in determining rates of decomposition in these ecosystems. From a litter decomposition modelling perspective, a “two carbon pools” (labile and recalcitrant) decay model also fitted litterbag data better than a single pool model (Chimner and Ewel, 2005). Wright et al. (2011) also highlighted a strong relationship between CO2 flux and quantity of labile carbon (expressed as abundance of carbohydrates and/or carbohydrates to aliphatic ratio). Some studies also suggest the quality–SHR-CO2 relationship in tropical peatlands is insignificant. Despite being related to organic matter quality, lignin and phenolic compounds abundance did not show a significant relationship with CO2 emissions in a peatland in Panama (Hoyos-Santillan et al., 2019). This is also corroborated by a study in Florida, where there was no significant correlation between CO2 flux and LCI or any fraction of SOM (lignin, cellulose, labile fraction) both under aerobic and anaerobic conditions (Inglett et al., 2012). Addition of labile organic matter can also enhance the mineralisation of more recalcitrant organic matter though a priming effect (Hamer and Marschner, 2002; Nottingham et al., 2012). Priming was observed in several tropical peatland incubation studies (Hamer and Marschner, 2002; Girkin et al., 2018a; 2018b; Akhtar et al., 2022). This suggests that labile carbon addition to peat via litter input and root exudates can stimulate decomposition (Girkin et al., 2018a; Girkin et al., 2018b; Girkin et al., 2020b; Akhtar et al., 2022). SHR-CO2 is therefore influenced by SOM decomposability itself and a priming effect due to labile carbon inputs. Altogether, those indexes such as LCI, depth, age, labile and recalcitrant fractions, might not be the adequate to quantify the link between decomposability and SHR-CO2 for peatlands. Those metrics have also been shown to be insufficient to describe patterns of litter decomposition as decomposition rate of litter is not simply a direct function of its decomposability but also controlled by interactions with microorganisms and local vegetation (Austin et al., 2014).
The microbial community structure is dependent on the rate and quality of litter accumulation (Andersen et al., 2013), and the reduction of readily utilisable substrate contributes to the observed decline in microbial biomass and CO2 production rates with depth (Jackson et al., 2009; Andersen et al., 2013; Li, Q. et al., 2021). In tropical peatlands, the microbial community has been shown to be significantly different between primary and secondary peat swamp forests (Dhandapani et al., 2019) or level of peatland disturbance (Dhandapani et al., 2020) but do not necessarily differ significantly between types of natural dominant vegetation (Girkin et al., 2020a).
Decomposition rate is also influenced by local vegetation. Faster decomposition of litter from the local vegetation at “home” compared to far from its environment of origin has been observed in some ecosystems (Austin et al., 2014). This phenomenon is referred to as the “home-field advantage” (HFA) and results from the specialisation over time of the decomposer community to degrading the specific local litter type. This can lead to fast decomposition of recalcitrant litter despite its recalcitrance or low nutrient availability in its home environment (Austin et al., 2014). Available studies report mixed results on the applicability of HFA for boreal (Haynes et al., 2015; Palozzi and Lindo, 2017), temperate (Bragazza et al., 2007) and tropical peatlands (Hoyos-Santillan et al., 2018; Lam et al., 2021). Hoyos-Santillan et al. (2018) highlighted a significant difference in leaf litter decomposition rates between the home and translocation sites but results presented by Lam et al. (2021) only weakly support the HFA.
All the reviewed models account for differences in decomposability via the base rate of SHR-CO2 of different carbon pools. Many models define some carbon pools with higher or lower recalcitrance, active vs. passive, litter vs. biomass or humus, and assign a base rate value to each pool. Other models consider the litter, the acrotelm and the catotelm as different carbon pools (LPJ-WHyMe, LPX-Bern). Others, set the value of the base rate based on the PFT and/or vegetation tissue (LPJ-GUESS, MWM, DigiBog, HPM). Less common is the use of base rate categories based on soil chemistry (MILLENA), or peat type and water table depth (CLASS-CTEM). Finally, LPJ-GUESS, MWM and HPM also define base rates to decline over time for all carbon pools.
The review of the literature highlights a lack of unified approach to characterise peat decomposability. Therefore, no general conclusion can be drawn from the existing studies on the impact of substrate decomposability on SHR-CO2 for tropical peatlands. Across indices and proxies used, most studies observe a lower SHR-CO2 with increasing recalcitrance but the lack of a widely used common index limits comparisons.
The lack of common decomposability proxy also limits our ability to identify clear patterns and the drawing of general conclusions that could be useful for models–other than the fact that having a two (or more) pools representation with different base rates is better than using a single pool model. Models often rely on vegetation type, vegetation tissue, peat age or peat depth to estimate the organic matter decomposability. For a local peat-specific model, this could be parametrised with corresponding local decomposition data. However, considering the currently available data, this would not be possible at the pantropical or global scale because the wide diversity of peatland ecosystems requires more experimental data on decomposition base rates across different vegetation types.
The noise resulting from diverse moisture and temperature conditions across studies also hinders our understanding of the contribution of carbon decomposability to SHR-CO2. This could be solved by experiments focusing on measuring decomposition base rates across vegetation types by using a single base temperature and maintaining optimum moisture conditions for their decomposition.
There is no consensus yet on how nutrient availability influences tropical peat decay. Hoyos-Santillan et al. (2018) reported that nutrient addition (nitrogen and/or phosphorus) did not have a significant impact on leaf, stem, and root litter decomposition in most of the treatments. Nitrogen addition only significantly increased the leaf decomposition at depth in waterlogged conditions. This could be explained by the nitrogen limitation in more degraded peat at depth or N-limitation because of the anaerobic conditions slowing down the release of nitrogen from peat. Conversely, phosphorous addition slightly decreased leaf decomposition rates in below-ground waterlogged conditions. This might be explained by lower enzyme activity due to higher phosphorous concentration slowing the decomposition of complex carbon (Hoyos-Santillan et al., 2018). Overall, nutrient limitation was not a strong control on general litter decomposition, while litter chemistry and O2 availability were stronger controls (Hoyos-Santillan et al., 2018). In a second nutrient addition experiment (nitrogen and/or phosphorus) on surface peat, CO2 fluxes were not significantly different between treatments (Hoyos-Santillan et al., 2019). The authors suggested that a strong effect of the water table variations cancelling out the effect of nutrient addition could explain this or insufficient labile organic matter availability limiting GHG fluxes. Conversely, Sihi et al. (2016) and Sihi et al. (2018) found a significant influence of nutrient levels on peat decomposition. However, both studies concluded that the nutrient level was not the strongest factor, but that decomposition was the most sensitive to depth and temperature (Sihi et al., 2016) or peat quality (Sihi et al., 2018).
Despite reaching opposite conclusions on the significance of nutrient levels on tropical peat decomposition, these four studies agree on the relative lower importance of nutrients on peat decay compared to moisture, temperature and quality. If there is any nutrient limitation to SHR-CO2 in tropical peatlands, which remains an open question, this limitation might also be complex simply because of differences amongst tropical peatland types and sites. The link between nutrients and peat/litter decomposition is further rendered more complex by interactions between litter type and soil organisms. Hoyos-Santillan et al. (2018) observed a clear HFA in Panama and suggests that decomposer communities are adapted and can overcome nutrient availability. While Lam et al. (2021) only observed a weak HFA but argue that this may be a result of unspecialised communities between their translocation sites resulting from of high plant diversity which is one of the known limiting factors to observing HFA (Austin et al., 2014; Lam et al., 2021). Further work and a change of approach from chemistry-centred to litter-decomposer interactions are needed to improve our understanding of the applicability of the HFA in peatlands and how decomposer communities can overcome nutrient availability.
Five of the reviewed models simulate, with various levels of complexity, nutrient cycles. LPX-Bern simply has two sets of SHR-CO2 base rates values to account or not for nitrogen limitation. JULES-Peat and ECOSSE assume nitrogen impacts SOM decomposition as it is controlled by the set CN ratio of the biomass and humus pools which must remain constant. PEATBOG includes a nitrogen multiplier depending on whether the CN ratio is above or below a critical threshold. Ecosys includes both nitrogen and phosphorus limitation based on the CN and CP ratios. And Wetland-DNDC ‘s nitrogen reduction factor varies from 1.1 to 0.2 for high N availability (expressed as a CN ration per day) to low N availability.
Only two of the reviewed models include the decomposers. Ecosys splits the soil carbon in organic matter and microbe complexes and the SHR-CO2 is a function of the active and its properties. In MWM, the decomposition rates are regulated by the microbial biomass, and it includes microbial dynamics.
There is no consensus in the literature yet about the impact of nitrogen and phosphorus on SHR-CO2 in tropical peatlands. However, existing studies do agree on the lesser importance of nutrients on SHR-CO2 compared to moisture, temperature, and peat decomposability. The two studies investigating the applicability of the HFA in tropical peatlands disagree on their results, while the few studies conducted for boreal and temperate peatlands also gave mixed results. Altogether, this highlights the need for more experiments to improve our understanding of both nutrients and litter-decomposers interactions in SHR-CO2 processes.
Those open questions limit the possible refinement of models at present. However, the available literature points toward the lesser importance of nutrients on organic matter decomposition in tropical peatlands compared to moisture, temperature, and carbon decomposability. Therefore, the priority for model improvement should be, in the first instance, further work on those three primary SHR-CO2 drivers.
There is no consensus on the influence of moisture on the temperature sensitivity of SHR-CO2 as some studies report an increase in Q10 under wetter and anoxic conditions (Inglett et al., 2012) while many others suggest the opposite, a Q10 increase with oxygen availability and lower water content (Hogg et al., 1992; Szafranek-Nakonieczna and Stêpniewska, 2014; Liu L. et al., Liu L. et al., 2016; Liu L. et al., 2022; Sjögersten et al., 2018). Some studies further suggest that the moisture-Q10 relationship is insignificant (Wang X. et al., 2010).
The influence of peat moisture on temperature sensitivity includes both the explicit influence of water and the implicit influence of oxygen availability. Teasing out the individual effects of water and oxygen availability offers a partial explanation for those contrasting conclusions. Sjögersten et al. (2018) compared the Q10 values of incubated flooded oxic and flooded anoxic samples, therefore isolating the impact of oxygen on temperature sensitivity, and concluded that oxygen limitation reduces the sensitivity of SHR-CO2 to temperature. On the other hand, two other studies examined the Q10 of samples from a range of moisture conditions under oxic (or mostly oxic conditions in the case of water saturation without flushing the O2 out of the sample and short incubation time). They both report an increase in temperature sensitivity with increasing moisture (Hardie et al., 2011; Byun et al., 2021).
From those conclusions on the individual role of water and oxygen on Q10 values, it might seem as though a simple relationship exists as Q10 increases with oxygen availability and with increasing water content. However, this does not translate into observed Q10 values when both moisture and oxygen are varied simultaneously as discussed previously, including some studies suggesting that oxygen availability is more important (i.e., oxic drier samples have a higher temperature sensitivity or Q10 than anoxic saturated samples) (Hogg et al., 1992; Szafranek-Nakonieczna and Stêpniewska, 2014; Sjögersten et al., 2018) while Inglett et al. (2012) suggests that the opposite is true. Finally, the non-significant results obtained by Wang X. et al. (2010) suggest that the relative importance of both parameters might not be straightforward.
The cross-studies of intact tropical peat incubation SHR-CO2 Q10 data compiled here suggest that anoxic conditions lead to significantly higher temperature sensitivity (ANOVA, F = 6.678, df = 2, p < 0.005) than oxic conditions (TukeyHSD p < 0.01) or saturated oxic conditions (TukeyHSD, p < 0.05). For the boreal and temperate peatlands groups, the anoxic Q10 values are only significantly different to the oxic Q10 values (boreal ANOVA, F = 4.696, df = 2, p < 0.001, TukeyHSD, p < 0.05, temperate ANOVA, F = 3.077, df = 2, p > 0.05, TukeyHSD, p < 0.05). At the global scale, there is no significant difference between the water-oxygen groups (ANOVA, F = 1.805, df = 2, p > 0.05) (Supplementary Figure S3.1). Under anoxic and flooded oxic conditions, the tropical group is significantly different to the boreal and temperate groups in terms of Q10 values (anoxic ANOVA, F = 7.146, df = 2, p < 0.001, TukeyHSD, p < 0.005, flooded oxic ANOVA, F = 39.68, df = 2, p < 0.001, TukeyHSD, p < 0.005) (Figure 9A). Under oxic incubations conditions, is only significantly different to the temperate group (ANOVA, F = 19.26, df = 2, p < 0.001, TukeyHSD, p < 0.005).
Figure 9. (A) Q10 estimates of peat SHR-CO2 from incubation for each biome and moisture and oxygen conditions (boreal anoxic, oxic and flooded oxic n = 163,286 and 14, temperate anoxic, oxic and flooded oxic n = 57, 263, 9 and tropical anoxic, oxic and flooded oxic n = 30, 40, 11). ANOVA across climate groups for the anoxic group (F = 7.146, df = 2, p < 0.001), oxic group (F = 19.26, df = 2, p < 0.001) and flooded oxic (F = 19.26, df = 2, p < 0.001). Tukey HSD letters indicate significant differences p < 0.05 for each moisture-oxygen group. (B) Resulting reaction rate ratio for the corresponding range of Q10 for 10°C and 20°C base temperatures. A lower base temperature results in a larger range of reaction rates at high temperatures.
The multivariate model of Q10 with latitude (degree decimal), moisture and oxygen condition and an interaction term is significant (p < 0.005) but the relationship is weak (R2adjusted = 0.01529) (Supplementary Figure S3.2). This model also does not significantly improve model fit compared to the simple linear model with only latitude (see Section 3.1) (p > 0.05).
The oxic group includes a wide range of moisture conditions. Therefore, the subset of data associated with a moisture content as %WFPS was analysed further. There is no significant linear or polynomial relationship between the water-filled-pore-space and Q10 (Figure 10) across oxygen-availability conditions (df = 246, F = 1.706, p > 0.05and df = 245, F = 0.856, p > 0.05) nor in the oxic subset.
Figure 10. Q10 estimates for global peatlands against moisture values, colours correspond to oxygen availability. Here we present a subset of observations with known water-filled pore space. The horizontal reference line is the median Q10 (2.04).
No clear significant relationship between Q10 and moisture was observed in our database which suggests that a constant Q10 of about 2 remains the most parsimonious option. If there is a relationship as suggested by some individual studies in the literature, it might be hidden in the noise of the database resulting from the lack of standardised incubation set-up and measurements and the unclear relative importance of the influence of moisture only vs. oxygen-availability.
According to kinetic theory, temperature sensitivity increases with organic matter recalcitrance as recalcitrant organic matter decomposition requires higher activation energies (Fierer et al., 2005; Wang Q. et al., 2019). This relationship between SOM decomposability and the sensitivity of its decomposition to temperature is also described as the carbon quality-temperature hypothesis (Wang Q. et al., 2019). Globally, decomposition of labile SOC has a significantly lower temperature sensitivity than decomposition of the recalcitrant fraction (Conant et al., 2011; Wang Q. et al., 2019). However, when substrate availability is limited, experiments show mixed results, with experiments that minimize the influence of substrate availability being consistent with kinetic theory (Conant et al., 2011). The temperature sensitivity of soil organic carbon decomposition is a multi-component problem including potentially counteracting interactions (Conant et al., 2011). Therefore, the debate needs to be centred on how temperature affects the various mechanisms controlling the resistance of SOM including both quality and physico-chemical protection (adsorption/desorption and occlusion in aggregates) (Conant et al., 2011). Further investigation on the processes controlling substrate availability and how they respond to temperature is needed. Quality also interacts with other variables and temperature sensitivity may have different controlling factors between labile pools (nitrogen) and recalcitrant pools (soil organic carbon) (Wang Q. et al., 2019).
Compared to mineral soils, substrate availability in carbon-rich peat soils is less likely to be important especially for shallower samples, although a large proportion of the carbon may be recalcitrant at all depths. However, substrate availability and quality are not commonly reported or investigated in peat SHR-CO2 incubation studies. Substrate availability due to adsorption and desorption should not have an important effect in peat except maybe if the peat is shallow and in contact with clay, or rich in iron or other metals. The importance of SOM protection via occlusion in aggregates in peat remains to be explored.
Some peatlands studies do not observe any interaction between decomposability (using quality or depth) and moisture on SHR-CO2 (Inglett et al., 2012; Szafranek-Nakonieczna and Stêpniewska, 2014). However, others report a significant interaction (based on sample depth) (Hogg et al., 1992; Wang, X. et al., 2010; Hoyos-Santillan et al., 2016; Liu L. et al., 2016; Li Q. et al., 2021; Liu L. et al., 2022). For instance, CO2 fluxes from peat samples from Panama significantly decreased with sample depth in oxic conditions but differences were insignificant in anoxic incubations (Hoyos-Santillan et al., 2016). Hogg et al. (1992), also observed that CO2 fluxes decrease with quality (sample depth) in all moisture treatments but the decrease relative to the surface sample was larger in drained conditions for some temperate peat samples.
While for temperature sensitivity in oxic incubations, lower quality (as evidenced by indexes such as B), larger recalcitrant pool size, deeper or older peat have all been suggested to be linked to higher temperature sensitivity in peatlands (Koch et al., 2007; Hardie et al., 2011; Inglett et al., 2012; Hilasvuori et al., 2013; Li Q. et al., 2021). However, Brady (1997) reports a higher temperature sensitivity in near-surface peat. Inglett’s et al. (2012) reported that Lignin Cellulose Index (LCI) was negatively correlated with Q10 values. Duval and Radu (2018) also observed a quadratic concave relationship between LCI and Q10, while other studies suggest that the interaction with decomposability (based on glucose addition and chemistry of the organic matter fraction) not being significant in controlling temperature sensitivity (Yavitt et al., 2000; Girkin et al., 2020b).
Under anoxic conditions, multiple studies have reported that the interaction between decomposability (estimated using LCI, depth, age, quality and addition of glucose) and Q10 is insignificant (Yavitt et al., 2000; Hardie et al., 2011; Inglett et al., 2012; Ye et al., 2016; Li Q. et al., 2021). However, Sihi et al. (2016), Sihi et al. (2018) do report a higher Q10 for older and lower quality (B) samples in anoxic conditions, while Wang, H. et al. (2023) similarly observed higher Q10 values in deeper layers. However, Q10 and B values from Gallego-Sala (2008) suggest the opposite and Duval and Radu (2018) also observed higher Q10 values for samples with a larger cellulose fraction.
Due to the lack of a single decomposability metric used across the dataset, the evaluation of the interaction of decomposability with the other drivers of SHR-CO2 is limited to depth as a surrogate. There is no significant linear relationship between sample depth and Q10 in our database under anoxic conditions (df = 248, F = 3.391, p > 0.05), saturated oxic conditions (df = 32, F = 0.135, p > 0.05) and for all moisture conditions grouped together (df = 871, F = 2.699, p > 0.05). However, there is a significant positive linear but very weak relationship under oxic conditions (df = 587, F = 24.94, p < 0.001, R2adjusted = 0.04) (see Supplementary Figure S3.3). This suggests kinetic theory could be applicable to peat under unsaturated oxic conditions, but it remains unclear under other moisture-oxygen treatments. Depth is also a useful indicator for decomposability for comparable sites but not a robust indicator when comparing diverse studies from across biomes and with methodological differences. Most of the samples in the database come from intact peatlands but some also come from disturbed peatlands. The difference between Q10 estimates for drained, forested or cultivated peatlands and intact peatland was insignificant, but Q10 estimates were much lower for the few samples of burnt peat (see Supplementary Figure S3.4).
The interaction between peat moisture and temperature sensitivity remains unclear, especially when we are not able to tease apart the individual role of moisture itself and related oxygen availability. However, the literature suggests that oxygen limitation is associated with lower temperature sensitivity and that increased moisture (while maintaining oxic conditions) is associated with higher sensitivity. The comparison between anoxic, oxic, oxic and water-saturated incubation groups when combining Q10 estimates from across studies does not support these conclusions and there is no significant relationship between Q10 values and moisture (%WFPS subset). This highlights the limitations of the dataset, and the difficulty in detecting trends where many factors are at play and where noise due to methodological differences is high.
The existing literature does not present enough evidence for a consensus on the interaction effect of moisture and temperature with decomposability on SHR-CO2. According to kinetic theory and global studies of SOM, recalcitrance is associated with higher temperature sensitivity when substrate is not limiting. Some studies on peat soil, and across oxic and anoxic conditions, agree with this hypothesis but some studies do suggest the opposite or that the interaction is insignificant. We observed a significant but weak positive linear relationship between peat depth and Q10 for unsaturated oxic incubations, but no evidence was found in the dataset to support the application of kinetic theory for peat soils under saturated or anoxic conditions. The lack of a widespread and comparable decomposability indicator among peat incubation studies and other methodological differences in the experiments also prevents us from reaching a conclusion for peatlands moisture-temperature-decomposability interactions at present.
Few of the reviewed models include interactions of the main controls on SHR-CO2. In ecosys, the moisture modifier is also a function of soil temperature, increasing the influence of moisture on SHR-CO2 under warmer temperatures. LPX-Bern uses a different SHR-CO2 moisture modifier between the acrotelm and the catotelm. Then PEATBOG differentiate the temperature response of labile and recalcitrant using a higher Q10 value for recalcitrant carbon. Finally, DigiBog has an anoxic and oxic Q10 parameters, therefore potentially taking into account moisture-temperature interaction, although both parameters were set to the same value in the versions reviewed here (Table 1).
Questions remain as few studies have explored the interaction between moisture and temperature for SHR-CO2 in tropical peatlands. Existing literature for peatlands globally gives contrasting results with the majority of studies pointing toward an increased temperature sensitivity with moisture and oxygen availability. However, considering the absence of a consensus, a constant Q10 across moisture and oxygen conditions remains a justifiable and parsimonious option for models, especially those at the global scale, as models applied to a single site may use available data for the site to capture interaction terms.
Similarly, the lack of comparable data to quantify a moisture-temperature-decomposability interaction prevents us from drawing a robust conclusion. Despite the relatively well supported kinetic theory explaining interaction terms for soil organic matter decomposition in non-peat soils, its applicability to peat decomposition is poorly quantified. Therefore, further work and a better understanding are required before adding more complexity to the models would be justified.
Representation of carbon cycling in tropical peatlands has been hindered by the lack of available data for model parametrisation. We therefore review the current state of knowledge of SHR-CO2 in tropical peat soils and compiled the related available data. We identify three main controls on SHR-CO2: moisture, temperature and quality of substrate. We also discuss some of the interactions between these drivers. Figure 11 summarises the outcomes of our review and organises the drivers into one of three categories: a) satisfactory understanding, b) some understanding but data insufficient to constrain models and c) lack of understanding and/or data insufficient to be represented in models.
Figure 11. Summary diagram of the outcomes of our review of SHR-CO2 for tropical peatlands. The moisture resting point and Q10 are both unitless ratios. Green: satisfactory understanding of the processes and data to constrain models, Orange: some understanding of the processes but not enough experimental data to constrain models, Red: lack of or limited understanding of the processes and data to represent or constraint models.
The effect of moisture is relatively well known for soils in general, but a knowledge gap remains for tropical peatlands, where more research is needed to better understand the shape of the relationship (Figure 11). There are two main critical points in the relationship between moisture and SHR-CO2 rates: the optimum moisture and the moisture resting point. First the optimum moisture was found to range between 25 and 95%WFPS. Secondly, the moisture resting point in anoxic conditions (median value: 0.105, range: 0.005–0.290) is significantly lower than moisture resting point estimates that consider only the effect of water-saturation without oxygen limitation. Most models use a similar function shape, but they differ in their optimum moisture (and sometimes consider a plateau of optimum moisture conditions) and their resting point. Overall, model moisture functions are mostly within the envelope of experimental data. However, further work is needed to narrow down the optimum moisture range and to clarify if there is a relationship with peat properties as observed for other types of soils. Remaining variability for the moisture resting point is likely due to interaction with temperature conditions and decomposability.
In terms of temperature dependency of decomposition processes, there are many studies in mineral soil ecosystems, but less have focussed on tropical peatlands. Q10 is a widely used metric to quantify and compare temperature sensitivity of SHR-CO2 in a variety of peat soil types, and mostly ranges between 0.85 and 3.10 (outliers excluded) for tropical peatlands. This range of values is not significantly different from estimates for boreal and temperate peatlands (global median Q10: 2.04, range: 0.24 - 12.10) (Figure 11). Correspondingly, the most widely used temperature function amongst the reviewed models is a Q10 formulation. In addition, the majority of the temperature functions fall within the envelope from experimental data. Remaining variability amongst the data is likely due differences in moisture and decomposability between samples and method differences across studies included in the dataset. The Q10 method has some limitations and is not based on a theoretical basis. Therefore, a move towards an Arrhenius type approach is advisable, but only possible if experimental studies report the necessary function parameters.
The lack of a widely applicable and unified decomposability metric limits general conclusions for tropical peatlands (Figure 11). The various methods used in models mirror the various indexes used amongst empirical studies. This prevents us from improving its modelling at the pan-tropical scale.
Nutrient-limitation studies in tropical peatlands are rare and do not reach a consensus on how they influence SHR-CO2. However, available studies do agree that nutrients seem to be of lesser importance compared to moisture, temperature and decomposability effects on SHR-CO2 (Figure 11). Few models attempt to simulate nutrient cycling, and yet fewer include the interaction with the decomposer community. More empirical investigation is needed to quantify the relationship between nutrients and SHR-CO2. The relationship of decomposition and nutrients is further complicated by the interaction with soil micro-organism community. Therefore, the nutrients- SHR-CO2 relationship should also be aimed to include the influence of the decomposer community.
Many unknowns remain about the key interactions between the drivers of SHR-CO2 (Figure 11). Therefore, simple assumptions such as a constant temperature sensitivity across moisture conditions, remain to date a justifiable and parsimonious approach. Future work should focus on improving our understanding of these processes and on providing more empirical data to investigate the applicability of kinetic theory under varying moisture-oxygen conditions before attempting to add more complexity into models.
Overall, we have been able to identify main knowledge gaps that we need to fill to improve modelling efforts. These include the optimum moisture range for tropical peatlands SHR-CO2, the applicability of kinetic theory to peat soils, the lack of a widely used and comparable decomposability metric, and overall, the lack of a standard methodological set up across peat incubation studies which complicates pan-tropical comparison.
ED: Conceptualization, Data curation, Formal Analysis, Investigation, Visualization, Writing–original draft, Writing–review and editing. EB: Conceptualization, Supervision, Writing–review and editing. SC: Supervision, Writing–review and editing. JK: Writing–review and editing. SS: Supervision, Writing–review and editing. NS: Conceptualization, Writing–review and editing. AG-S: Conceptualization, Supervision, Writing–review and editing.
The author(s) declare that financial support was received for the research and/or publication of this article. This project has received funding from the European Research Council (ERC) under the European Unionandapos;s Horizon 2020 research and innovation programme (grant agreement No 865403). A.V. Gallego-Sala and N.D. Smith gratefully acknowledge funding from the Natural Environment Research Council: NE/V018299/1 Improving Modelling approaches to assess climate change-related Thresholds and Ecological Range Shifts in the Earth’s Peatland ecosystems (MOTHERSHIP)).
We thank Eleanor Blythe for interesting discussions on the moisture resting point and its conceptualization.
The authors declare that the research was conducted in the absence of any commercial or financial relationships that could be construed as a potential conflict of interest.
All claims expressed in this article are solely those of the authors and do not necessarily represent those of their affiliated organizations, or those of the publisher, the editors and the reviewers. Any product that may be evaluated in this article, or claim that may be made by its manufacturer, is not guaranteed or endorsed by the publisher.
The Supplementary Material for this article can be found online at: https://www.frontiersin.org/articles/10.3389/fgeoc.2025.1492386/full#supplementary-material
Abdalla, M., Hastings, A., Bell, M. J., Smith, J. U., Richards, M., Nilsson, M. B., et al. (2014). Simulation of CO2 and attribution analysis at six European peatland sites using the ECOSSE model. Water, Air, and Soil Pollut. 225 (11), 2182. doi:10.1007/s11270-014-2182-8
Akhtar, H., Lupascu, M., and Sukri, R. S. (2022). Interactions between microtopography, root exudate analogues and temperature determine CO2 and CH4 production rates in fire-degraded tropical peat. Soil Biol. Biochem. 169, 108646. doi:10.1016/j.soilbio.2022.108646
Andersen, R., Chapman, S. J., and Artz, R. R. E. (2013). Microbial communities in natural and disturbed peatlands: a review. Soil Biol. Biochem. 57, 979–994. doi:10.1016/j.soilbio.2012.10.003
Apers, S., De Lannoy, G. J. M., Baird, A. J., Cobb, A. R., Dargie, G. C., del Aguila Pasquel, J., et al. (2022). Tropical peatland hydrology simulated with a global land surface model. J. Adv. Model. Earth Syst. 14 (3), e2021MS002784. doi:10.1029/2021MS002784
Arora, V. K., Katavouta, A., Williams, R. G., Jones, C. D., Brovkin, V., Friedlingstein, P., et al. (2020). Carbon–concentration and carbon–climate feedbacks in CMIP6 models and their comparison to CMIP5 models. Biogeosciences 17 (16), 4173–4222. doi:10.5194/bg-17-4173-2020
Austin, A. T., Vivanco, L., González-Arzac, A., and Pérez, L. I. (2014). There’s no place like home? An exploration of the mechanisms behind plant litter–decomposer affinity in terrestrial ecosystems. New Phytol. 204 (2), 307–314. doi:10.1111/nph.12959
Azima Busman, N., Maie, N., Sangok, F. E., Melling, L., and Watanabe, A. (2023). Impacts of agricultural drainage on the quantity and quality of tropical peat soil organic matter in different types of forests. Geoderma 439, 116670. doi:10.1016/j.geoderma.2023.116670
Baird, A. J., Low, R., Young, D., Swindles, G. T., Lopez, O. R., and Page, S. (2017). High permeability explains the vulnerability of the carbon store in drained tropical peatlands. Geophys. Res. Lett. 44 (3), 1333–1339. doi:10.1002/2016GL072245
Baird, A. J., Morris, P. J., and Belyea, L. R. (2012). The DigiBog peatland development model 1: rationale, conceptual model, and hydrological basis. Ecohydrology 5 (3), 242–255. doi:10.1002/eco.230
Best, M. J., Pryor, M., Clark, D. B., Rooney, G. G., Essery, R. L. H., Ménard, C. B., et al. (2011). The joint UK land environment simulator (JULES), model description – Part 1: energy and water fluxes. Geosci. Model Dev. 4 (3), 677–699. doi:10.5194/gmd-4-677-2011
Blake, G. R., Steinhardt, G. C., Pombal, X. P., Muñoz, J. C. N., and Cortizas, A. M. (2008). “Peat,” in Encyclopedia of soil science. Editor W. Chesworth (Dordrecht: Springer Netherlands). doi:10.1007/978-1-4020-3995-9_409
Booth, B. B. B., Harris, G. R., Murphy, J. M., House, J. I., Jones, C. D., Sexton, D., et al. (2017). Narrowing the range of future climate projections using historical observations of atmospheric CO2. J. Clim. 30, 3039–3053. doi:10.1175/JCLI-D-16-0178.1
Bosatta, E., and Ågren, G. I. (1999). Soil organic matter quality interpreted thermodynamically. Soil Biol. Biochem. 31 (13), 1889–1891. doi:10.1016/S0038-0717(99)00105-4
Brady, M. A. (1997). Organic matter dynamics of coastal peat deposits in Sumatra. Indonesia: [University of British Columbia. doi:10.14288/1.0075286
Bragazza, L., Siffi, C., Iacumin, P., and Gerdol, R. (2007). Mass loss and nutrient release during litter decay in peatland: the role of microbial adaptability to litter chemistry. Soil Biol. Biochem. 39 (1), 257–267. doi:10.1016/j.soilbio.2006.07.014
Byun, E., Rezanezhad, F., Fairbairn, L., Slowinski, S., Basiliko, N., Price, J. S., et al. (2021). Temperature, moisture and freeze–thaw controls on CO2 production in soil incubations from northern peatlands. Sci. Rep. 11 (1), 23219. doi:10.1038/s41598-021-02606-3
Carey, J. C., Tang, J., Templer, P. H., Kroeger, K. D., Crowther, T. W., Burton, A. J., et al. (2016). Temperature response of soil respiration largely unaltered with experimental warming. Proc. Natl. Acad. Sci. 113 (48), 13797–13802. doi:10.1073/pnas.1605365113
Chadburn, S. E., Burke, E. J., Gallego-Sala, A. V., Smith, N. D., Bret-Harte, M. S., Charman, D. J., et al. (2022). A new approach to simulate peat accumulation, degradation and stability in a global land surface scheme (JULES vn5.8_accumulate_soil) for northern and temperate peatlands. Geosci. Model Dev. 15 (4), 1633–1657. doi:10.5194/gmd-15-1633-2022
Chapman, S. J., and Thurlow, M. (1998). Peat respiration at low temperatures. Soil Biol. Biochem. 30 (8), 1013–1021. doi:10.1016/S0038-0717(98)00009-1
Charman, D. J., Beilman, D. W., Blaauw, M., Booth, R. K., Brewer, S., Chambers, F. M., et al. (2013). Climate-related changes in peatland carbon accumulation during the last millennium. Biogeosciences 10 (2), 929–944. doi:10.5194/bg-10-929-2013
Chaudhary, N., Miller, P. A., and Smith, B. (2017). Modelling Holocene peatland dynamics with an individual-based dynamic vegetation model. Biogeosciences 14 (10), 2571–2596. doi:10.5194/bg-14-2571-2017
Chimner, R. A., and Ewel, K. C. (2005). A tropical freshwater wetland: II. Production, decomposition, and peat formation. Wetl. Ecol. Manag. 13 (6), 671–684. doi:10.1007/s11273-005-0965-9
Clark, D. B., Mercado, L. M., Sitch, S., Jones, C. D., Gedney, N., Best, M. J., et al. (2011). The Joint UK Land Environment Simulator (JULES), model description – Part 2: carbon fluxes and vegetation dynamics. Geosci. Model Dev. 4 (3), 701–722. doi:10.5194/gmd-4-701-2011
Coleman, K., and Jenkinson, D. S. (2014). RothC- A Model for the turnover of carbon in soil - model description and users guide. Rothamsted Res. Harpenden. Available online at: https://www.abdn.ac.uk/staffpages/uploads/soi450/ECOSSE%20User%20manual%20310810.pdf.https://repository.rothamsted.ac.uk/item/98xv8/rothc-a-model-for-the-turnover-of-carbon-in-soil-model-description-and-users-guide-windows-version-updated-june-2014
Comeau, L.-P., Hergoualc’h, K., and Verchot, L. V. (2021). Dataset on soil carbon dioxide fluxes from an incubation with tropical peat from three different land-uses in Jambi Sumatra Indonesia. Data Brief 39, 107597. doi:10.1016/j.dib.2021.107597
Conant, R. T., Ryan, M. G., Ågren, G. I., Birge, H. E., Davidson, E. A., Eliasson, P. E., et al. (2011). Temperature and soil organic matter decomposition rates – synthesis of current knowledge and a way forward. Glob. Change Biol. 17 (11), 3392–3404. doi:10.1111/j.1365-2486.2011.02496.x
Cui, J., Li, C., and Trettin, C. (2005). Analyzing the ecosystem carbon and hydrologic characteristics of forested wetland using a biogeochemical process model. Glob. Change Biol. 11 (2), 278–289. doi:10.1111/j.1365-2486.2005.00900.x
Davidson, E. A., and Janssens, I. A. (2006). Temperature sensitivity of soil carbon decomposition and feedbacks to climate change. Nature 440 (7081), 165–173. doi:10.1038/nature04514
DeBusk, W. F., and Reddy, K. R. (1998). Turnover of detrital organic carbon in a nutrient-impacted everglades marsh. Soil Sci. Soc. Am. J. 62 (5), 1460–1468. doi:10.2136/sssaj1998.03615995006200050045x
Dhandapani, S., Ritz, K., Evers, S., Cooper, H., Tonks, A., and Sjögersten, S. (2020). Land-use changes associated with oil palm plantations impact PLFA microbial phenotypic community structure throughout the depth of tropical peats. Wetlands 40 (6), 2351–2366. doi:10.1007/s13157-020-01342-0
Dhandapani, S., Ritz, K., Evers, S., Yule, C. M., and Sjögersten, S. (2019). Are secondary forests second-rate? Comparing peatland greenhouse gas emissions, chemical and microbial community properties between primary and secondary forests in Peninsular Malaysia. Sci. Total Environ. 655, 220–231. doi:10.1016/j.scitotenv.2018.11.046
Dimitrov, D. D., Grant, R. F., Lafleur, P. M., and Humphreys, E. R. (2010). Modeling the effects of hydrology on ecosystem respiration at Mer Bleue bog. J. Geophys. Res. Biogeosciences 115 (G4). doi:10.1029/2010JG001312
Dioumaeva, I., Trumbore, S., Schuur, E. A. G., Goulden, M. L., Litvak, M., and Hirsch, A. I. (2002). Decomposition of peat from upland boreal forest: temperature dependence and sources of respired carbon. J. Geophys. Res. Atmos. 107 (D3). doi:10.1029/2001JD000848
Duval, T. P., and Radu, D. D. (2018). Effect of temperature and soil organic matter quality on greenhouse-gas production from temperate poor and rich fen soils. Ecol. Eng. 114, 66–75. doi:10.1016/j.ecoleng.2017.05.011
Fierer, N., Craine, J. M., McLauchlan, K., and Schimel, J. P. (2005). Litter quality and the temperature sensitivity of decomposition. Ecology 86 (2), 320–326. doi:10.1890/04-1254
Flanagan, N. E., Wang, H., Winton, S., and Richardson, C. J. (2020). Low-severity fire as a mechanism of organic matter protection in global peatlands: thermal alteration slows decomposition. Glob. Change Biol. 26 (7), 3930–3946. doi:10.1111/gcb.15102
Frolking, S., Roulet, N. T., Tuittila, E., Bubier, J. L., Quillet, A., Talbot, J., et al. (2010). A new model of Holocene peatland net primary production, decomposition, water balance, and peat accumulation. Earth Syst. Dyn. 1 (1), 1–21. doi:10.5194/esd-1-1-2010
Gallego-Sala, A. V. (2008). Temperature effects on trace gas production and uptake in aerobic and anaerobic soils [PhD thesis]. Bristol (UK): University of Bristol.
Gallego-Sala, A. V., Charman, D. J., Brewer, S., Page, S. E., Prentice, I. C., Friedlingstein, P., et al. (2018). Latitudinal limits to the predicted increase of the peatland carbon sink with warming. Nat. Clim. Change 8 (10), 907–913. doi:10.1038/s41558-018-0271-1
Girkin, N. T., Dhandapani, S., Evers, S., Ostle, N., Turner, B. L., and Sjögersten, S. (2020b). Interactions between labile carbon, temperature and land use regulate carbon dioxide and methane production in tropical peat. Biogeochemistry 147 (1), 87–97. doi:10.1007/s10533-019-00632-y
Girkin, N. T., Lopes dos Santos, R. A., Vane, C. H., Ostle, N., Turner, B. L., and Sjögersten, S. (2020a). Peat properties, dominant vegetation type and microbial community structure in a tropical peatland. Wetlands 40 (5), 1367–1377. doi:10.1007/s13157-020-01287-4
Girkin, N. T., Turner, B. L., Ostle, N., and Sjögersten, S. (2018a). Root-derived CO2 flux from a tropical peatland. Wetl. Ecol. Manag. 26 (5), 985–991. doi:10.1007/s11273-018-9617-8
Girkin, N. T., Turner, B. L., Ostle, N., and Sjögersten, S. (2018b). Composition and concentration of root exudate analogues regulate greenhouse gas fluxes from tropical peat. Soil Biol. Biochem. 127, 280–285. doi:10.1016/j.soilbio.2018.09.033
Grant, R. F. (2004). Modeling topographic effects on net ecosystem productivity of boreal black spruce forests. Tree Physiol. 24 (1), 1–18. doi:10.1093/treephys/24.1.1
Grant, R. F. (2015). Ecosystem CO2 and CH4 exchange in a mixed tundra and a fen within a hydrologically diverse Arctic landscape: 2. Modeled impacts of climate change. J. Geophys. Res. Biogeosciences 120 (7), 1388–1406. doi:10.1002/2014JG002889
Gudasz, C., Karlsson, J., and Bastviken, D. (2021). When does temperature matter for ecosystem respiration? Environ. Res. Commun. 3 (12), 121001. doi:10.1088/2515-7620/ac3b9f
Hamdi, S., Moyano, F. E., Sall, S., Bernoux, M., and Chevallier, T. (2013). Synthesis analysis of the temperature sensitivity of soil respiration from laboratory studies in relation to incubation methods and soil conditions. Soil Biol. Biochem. 58, 115–126. doi:10.1016/j.soilbio.2012.11.012
Hamer, U., and Marschner, B. (2002). Priming effects of sugars, amino acids, organic acids and catechol on the mineralization of lignin and peat. J. Plant Nutr. Soil Sci. 165 (3), 261–268. doi:10.1002/1522-2624(200206)165:3<261::AID-JPLN261>3.0.CO;2-I
Hardie, S. M. L., Garnett, M. H., Fallick, A. E., Rowland, A. P., Ostle, N. J., and Flowers, T. H. (2011). Abiotic drivers and their interactive effect on the flux and carbon isotope (14C and δ13C) composition of peat-respired CO2. Soil Biol. Biochem. 43 (12), 2432–2440. doi:10.1016/j.soilbio.2011.08.010
Haynes, K. M., Preston, M. D., McLaughlin, J. W., Webster, K., and Basiliko, N. (2015). Dissimilar bacterial and fungal decomposer communities across rich to poor fen peatlands exhibit functional redundancy. Can. J. Soil Sci. 95 (3), 219–230. doi:10.4141/cjss-2014-062
Heinemeyer, A., Croft, S., Garnett, M. H., Gloor, E., Holden, J., Lomas, M. R., et al. (2010). The MILLENNIA peat cohort model: predicting past, present and future soil carbon budgets and fluxes under changing climates in peatlands. Clim. Res. 45, 207–226. doi:10.3354/cr00928
Hilasvuori, E., Akujärvi, A., Fritze, H., Karhu, K., Laiho, R., Mäkiranta, P., et al. (2013). Temperature sensitivity of decomposition in a peat profile. Soil Biol. Biochem. 67, 47–54. doi:10.1016/j.soilbio.2013.08.009
Hirano, T., Jauhiainen, J., Inoue, T., and Takahashi, H. (2009). Controls on the carbon balance of tropical peatlands. Ecosystems 12 (6), 873–887. doi:10.1007/s10021-008-9209-1
Hirata, R., Adachi, M., and Ito, A. (2016). “Modeling of carbon and GHG budgets in tropical peatland,” in Tropical peatland ecosystems. Editors M. Osaki,, and N. Tsuji (Tokyo: Springer Japan). doi:10.1007/978-4-431-55681-7_32
Hodgkins, S. B., Richardson, C. J., Dommain, R., Wang, H., Glaser, P. H., Verbeke, B., et al. (2018). Tropical peatland carbon storage linked to global latitudinal trends in peat recalcitrance. Nat. Commun. 9 (1), 3640. doi:10.1038/s41467-018-06050-2
Hogg, E. H., Lieffers, V. J., and Wein, R. W. (1992). Potential carbon losses from peat profiles: effects of temperature, drought cycles, and fire. Ecol. Appl. 2 (3), 298–306. doi:10.2307/1941863
Hoyos-Santillan, J., Lomax, B. H., Large, D., Turner, B. L., Boom, A., Lopez, O. R., et al. (2015). Getting to the root of the problem: litter decomposition and peat formation in lowland Neotropical peatlands. Biogeochemistry 126 (1), 115–129. doi:10.1007/s10533-015-0147-7
Hoyos-Santillan, J., Lomax, B. H., Large, D., Turner, B. L., Boom, A., Lopez, O. R., et al. (2016). Quality not quantity: organic matter composition controls of CO2 and CH4 fluxes in neotropical peat profiles. Soil Biol. Biochem. 103, 86–96. doi:10.1016/j.soilbio.2016.08.017
Hoyos-Santillan, J., Lomax, B. H., Large, D., Turner, B. L., Lopez, O. R., Boom, A., et al. (2019). Evaluation of vegetation communities, water table, and peat composition as drivers of greenhouse gas emissions in lowland tropical peatlands. Sci. Total Environ. 688, 1193–1204. doi:10.1016/j.scitotenv.2019.06.366
Hoyos-Santillan, J., Lomax, B. H., Turner, B. L., and Sjögersten, S. (2018). Nutrient limitation or home field advantage: does microbial community adaptation overcome nutrient limitation of litter decomposition in a tropical peatland? J. Ecol. 106 (4), 1558–1569. doi:10.1111/1365-2745.12923
Husen, E., and Agus, F. (2011). Microbial activities as affected by peat dryness and ameliorant. Am. J. Environ. Sci. 7 (4), 348–353. doi:10.3844/ajessp.2011.348.353
Husen, E., Salma, S., and Agus, F. (2014). Peat emission control by groundwater management and soil amendments: evidence from laboratory experiments. Mitig. Adapt. Strategies Glob. Change 19 (6), 821–829. doi:10.1007/s11027-013-9526-3
Inglett, K. S., Inglett, P. W., Reddy, K. R., and Osborne, T. Z. (2012). Temperature sensitivity of greenhouse gas production in wetland soils of different vegetation. Biogeochemistry 108 (1), 77–90. doi:10.1007/s10533-011-9573-3
Intergovernmental Panel on Climate Change (IPCC) (2023). “Global carbon and other biogeochemical cycles and feedbacks,” in Climate change 2021 – the physical science basis: working group I contribution to the sixth assessment report of the intergovernmental Panel on climate change (Cambridge: Cambridge University Press). doi:10.1017/9781009157896.007
Jackson, C. R., Liew, K. C., and Yule, C. M. (2009). Structural and functional changes with depth in microbial communities in a tropical Malaysian peat swamp forest. Microb. Ecol. 57 (3), 402–412. doi:10.1007/s00248-008-9409-4
Jenkinson, D. S. (1990). The turnover of organic carbon and nitrogen in soil. Philosophical Trans. R. Soc. doi:10.1098/rstb.1990.0177
Kirschbaum, M. U. F. (1995). The temperature dependence of soil organic matter decomposition, and the effect of global warming on soil organic C storage. Soil Biol. Biochem. 27 (6), 753–760. doi:10.1016/0038-0717(94)00242-S
Koch, O., Tscherko, D., and Kandeler, E. (2007). Temperature sensitivity of microbial respiration, nitrogen mineralization, and potential soil enzyme activities in organic alpine soils. Glob. Biogeochem. Cycles 21 (4). doi:10.1029/2007GB002983
Kurnianto, S., Warren, M., Talbot, J., Kauffman, B., Murdiyarso, D., and Frolking, S. (2015). Carbon accumulation of tropical peatlands over millennia: a modeling approach. Glob. Change Biol. 21 (1), 431–444. doi:10.1111/gcb.12672
Kwon, M. J., Ballantyne, A., Ciais, P., Qiu, C., Salmon, E., Raoult, N., et al. (2022). Lowering water table reduces carbon sink strength and carbon stocks in northern peatlands. Glob. Change Biol. 28 (22), 6752–6770. doi:10.1111/gcb.16394
Lam, W. N., Lian, J. J., Chan, P. J., Ting, Y. Y., Chong, R., Rahman, N. E., et al. (2021). Leaf litter decomposition in tropical freshwater swamp forests is slower in swamp than non-swamp conditions. Biotropica 53 (3), 920–929. doi:10.1111/btp.12913
Laub, M., Ali, R. S., Demyan, M. S., Nkwain, Y. F., Poll, C., Högy, P., et al. (2021). Modeling temperature sensitivity of soil organic matter decomposition: splitting the pools. Soil Biol. Biochem. 153, 108108. doi:10.1016/j.soilbio.2020.108108
Lawson, I. T., Kelly, T. J., Aplin, P., Boom, A., Dargie, G., Draper, F. C. H., et al. (2015). Improving estimates of tropical peatland area, carbon storage, and greenhouse gas fluxes. Wetl. Ecol. Manag. 23 (3), 327–346. doi:10.1007/s11273-014-9402-2
Li, C., Cui, J., Sun, G., and Trettin, C. (2004). Modeling impacts of management on carbon sequestration and trace gas emissions in forested wetland ecosystems. Environ. Manag. 33 (1). doi:10.1007/s00267-003-9128-z
Li, C., Frolking, S., and Frolking, T. A. (1992). A model of nitrous oxide evolution from soil driven by rainfall events: 1. Model structure and sensitivity. J. Geophys. Res. Atmos. 97 (D9), 9759–9776. doi:10.1029/92JD00509
Li, Q., Leroy, F., Zocatelli, R., Gogo, S., Jacotot, A., Guimbaud, C., et al. (2021). Abiotic and biotic drivers of microbial respiration in peat and its sensitivity to temperature change. Soil Biol. Biochem. 153, 108077. doi:10.1016/j.soilbio.2020.108077
Liang, J., Li, D., Shi, Z., Tiedje, J. M., Zhou, J., Schuur, E. A. G., et al. (2015). Methods for estimating temperature sensitivity of soil organic matter based on incubation data: a comparative evaluation. Soil Biol. Biochem. 80, 127–135. doi:10.1016/j.soilbio.2014.10.005
Lippmann, T. J. R., van der Velde, Y., Heijmans, M. M. P. D., Dolman, H., Hendriks, D. M. D., and van Huissteden, K. (2023). Peatland-VU-NUCOM (PVN 1.0): using dynamic plant functional types to model peatland vegetation, CH4, and CO2 emissions. Geosci. Model Dev. 16 (22), 6773–6804. doi:10.5194/gmd-16-6773-2023
Liu, H., Rezanezhad, F., Zhao, Y., He, H., Van Cappellen, P., and Lennartz, B. (2024). The apparent temperature sensitivity (Q10) of peat soil respiration: a synthesis study. Geoderma 443, 116844. doi:10.1016/j.geoderma.2024.116844
Liu, L., Chen, H., and Tian, J. (2022). Varied response of carbon dioxide emissions to warming in oxic, anoxic and transitional soil layers in a drained peatland. Commun. Earth and Environ. 3 (1), 313. doi:10.1038/s43247-022-00651-y
Liu, L., Chen, H., Zhu, Q., Yang, G., Zhu, E., Hu, J., et al. (2016). Responses of peat carbon at different depths to simulated warming and oxidizing. Sci. Total Environ. 548-549, 429–440. doi:10.1016/j.scitotenv.2015.11.149
Lloyd, J., and Taylor, J. A. (1994). On the temperature dependence of soil respiration. Funct. Ecol. 8 (3), 315. doi:10.2307/2389824
Loisel, J., Gallego-Sala, A. V., Amesbury, M. J., Magnan, G., Anshari, G., Beilman, D. W., et al. (2021). Expert assessment of future vulnerability of the global peatland carbon sink. Nat. Clim. Change 11 (1), 70–77. doi:10.1038/s41558-020-00944-0
Maie, N., Maeda, M., Murouchi, A., Melling, L., Takamatsu, R., Sangok, F., et al. (2019). Influence of temperature and water conditions on the mineralization rate of tropical peat, 15. 15. Humic substances Research. Available online at: https://www.research.kobe-u.ac.jp/ans-soil/jhss/publishing.html
Mäkiranta, P., Laiho, R., Fritze, H., Hytönen, J., Laine, J., and Minkkinen, K. (2009). Indirect regulation of heterotrophic peat soil respiration by water level via microbial community structure and temperature sensitivity. Soil Biol. Biochem. 41 (4), 695–703. doi:10.1016/j.soilbio.2009.01.004
McKenzie, C., Schiff, S., Aravena, R., Kelly, C., and St. Louis, V. (1998). Effect of temperature on production of CH4 and CO2 from peat in a natural and flooded boreal forest wetland. Clim. Change 40 (2), 247–266. doi:10.1023/A:1005416903368
Melillo, J. M., Aber, J. D., Linkins, A. E., Ricca, A., Fry, B., and Nadelhoffer, K. J. (1989). Carbon and nitrogen dynamics along the decay continuum: plant litter to soil organic matter. Plant Soil 115 (2), 189–198. doi:10.1007/bf02202587
Melton, J. R., Sospedra-Alfonso, R., and McCusker, K. E. (2017). Tiling soil textures for terrestrial ecosystem modelling via clustering analysis: a case study with CLASS-CTEM (version 2.1). Geosci. Model Dev. 10 (7), 2761–2783. doi:10.5194/gmd-10-2761-2017
Metzger, C., Jansson, P.-E., Lohila, A., Aurela, M., Eickenscheidt, T., Belelli-Marchesini, L., et al. (2015). CO2 fluxes and ecosystem dynamics at five European treeless peatlands – merging data and process oriented modeling. Biogeosciences 12 (1), 125–146. doi:10.5194/bg-12-125-2015
Mezbahuddin, M., Grant, R. F., and Hirano, T. (2014). Modelling effects of seasonal variation in water table depth on net ecosystem CO2 exchange of a tropical peatland. Biogeosciences 11 (3), 577–599. doi:10.5194/bg-11-577-2014
Morris, P. J., Baird, A. J., and Belyea, L. R. (2012). The DigiBog peatland development model 2: ecohydrological simulations in 2D. Ecohydrology 5 (3), 256–268. doi:10.1002/eco.229
Morris, P. J., Baird, A. J., and Belyea, L. R. (2015). Bridging the gap between models and measurements of peat hydraulic conductivity. Water Resour. Res. 51 (7), 5353–5364. doi:10.1002/2015WR017264
Moyano, F. E., Manzoni, S., and Chenu, C. (2013). Responses of soil heterotrophic respiration to moisture availability: an exploration of processes and models. Soil Biol. Biochem. 59, 72–85. doi:10.1016/j.soilbio.2013.01.002
Moyano, F. E., Vasilyeva, N., Bouckaert, L., Cook, F., Craine, J., Curiel Yuste, J., et al. (2012). The moisture response of soil heterotrophic respiration: interaction with soil properties. Biogeosciences 9 (3), 1173–1182. doi:10.5194/bg-9-1173-2012
Mozafari, B., Bruen, M., Donohue, S., Renou-Wilson, F., and O’Loughlin, F. (2023). Peatland dynamics: a review of process-based models and approaches. Sci. Total Environ. 877, 162890. doi:10.1016/j.scitotenv.2023.162890
Nottingham, A. T., Turner, B. L., Chamberlain, P. M., Stott, A. W., and Tanner, E. V. J. (2012). Priming and microbial nutrient limitation in lowland tropical forest soils of contrasting fertility. Biogeochemistry 111 (1/3), 219–237. doi:10.1007/s10533-011-9637-4
Page, S. E., and Baird, A. J. (2016). Peatlands and global change: response and resilience. Annu. Rev. Environ. Resour. 41 (1), 35–57. doi:10.1146/annurev-environ-110615-085520
Palozzi, J. E., and Lindo, Z. (2017). Pure and mixed litters of Sphagnum and Carex exhibit a home-field advantage in Boreal peatlands. Soil Biol. Biochem. 115, 161–168. doi:10.1016/j.soilbio.2017.08.026
Parmesan, C., Morecroft, M. D., Trisurat, Y., Adrian, R., Anshari, G. Z., Arneth, A., et al. (2022). “Terrestrial and freshwater ecosystems and their services,” in Climate change 2022: impacts, adaptation and vulnerability. Contribution of working group II to the sixth assessment report of the intergovernmental Panel on climate change, H.-O. Pörtner, D. C. Roberts, M. M. B. Tignor, E. S. Poloczanska, K. Mintenbeck, and A. Alegría, (Cambridge University Press).
Petrescu, A. M. R., van Huissteden, J., de Vries, F., Bregman, E. P. H., and Scheper, A. (2009). Assessing CH4 and CO2 emissions from wetlands in the Drenthe Province, The Netherlands: a modelling approach. Neth. J. Geosciences 88 (2), 101–116. doi:10.1017/S0016774600000913
Qiu, C., Zhu, D., Ciais, P., Guenet, B., Krinner, G., Peng, S., et al. (2018). ORCHIDEE-PEAT (revision 4596), a model for northern peatland CO2, water, and energy fluxes on daily to annual scales. Geosci. Model Dev. 11 (2), 497–519. doi:10.5194/gmd-11-497-2018
Qiu, C., Zhu, D., Ciais, P., Guenet, B., Peng, S., Krinner, G., et al. (2019). Modelling northern peatland area and carbon dynamics since the Holocene with the ORCHIDEE-PEAT land surface model (SVN r5488). Geosci. Model Dev. 12 (7), 2961–2982. doi:10.5194/gmd-12-2961-2019
Ramirez, J. A., Peleg, N., Baird, A. J., Young, D. M., Morris, P. J., Larocque, M., et al. (2023). Modelling peatland development in high-boreal quebec, Canada, with DigiBog_Boreal. Ecol. Model. 478, 110298. doi:10.1016/j.ecolmodel.2023.110298
Ribeiro, K., Pacheco, F. S., Ferreira, J. W., de Sousa-Neto, E. R., Hastie, A., Krieger Filho, G. C., et al. (2021). Tropical peatlands and their contribution to the global carbon cycle and climate change. Glob. Change Biol. 27 (3), 489–505. doi:10.1111/gcb.15408
Sadaca, D., Hillis, D., Heller, H., and Berenbaum, M. (2012). Life the science of biology. 10th Edition. W. H. Freeman.
Shabbir, S., Qian, C., Faheem, M., Zhou, F., and Yu, Z.-G. (2024). New insights into the spatial variability of microbial diversity and density in peatlands exposed to various electron acceptors with an emphasis on methanogenesis and CO2 fluxes. Front. Microbiol. 15, 1468344. doi:10.3389/fmicb.2024.1468344
Shao, S., Wu, J., He, H., and Roulet, N. (2022). Integrating McGill Wetland Model (MWM) with peat cohort tracking and microbial controls. Sci. Total Environ. 806, 151223. doi:10.1016/j.scitotenv.2021.151223
Sihi, D., Inglett, P. W., Gerber, S., and Inglett, K. S. (2018). Rate of warming affects temperature sensitivity of anaerobic peat decomposition and greenhouse gas production. Glob. Change Biol. 24 (1), e259–e274. doi:10.1111/gcb.13839
Sihi, D., Inglett, P. W., and Inglett, K. S. (2016). Carbon quality and nutrient status drive the temperature sensitivity of organic matter decomposition in subtropical peat soils. Biogeochemistry 131 (1), 103–119. doi:10.1007/s10533-016-0267-8
Sitch, S., Smith, B., Prentice, I. C., Arneth, A., Bondeau, A., Cramer, W., et al. (2003). Evaluation of ecosystem dynamics, plant geography and terrestrial carbon cycling in the LPJ dynamic global vegetation model. Glob. Change Biol. 9 (2), 161–185. doi:10.1046/j.1365-2486.2003.00569.x
Sjögersten, S., Aplin, P., Gauci, V., Peacock, M., Siegenthaler, A., and Turner, B. L. (2018). Temperature response of ex-situ greenhouse gas emissions from tropical peatlands: interactions between forest type and peat moisture conditions. Geoderma 324, 47–55. doi:10.1016/j.geoderma.2018.02.029
Smith, J., Gottschalk, P., Bellarby, J., Richards, M., Nayak, D., Coleman, K., et al. (2010). Model to estimate carbon in organic soils – sequestration and emissions (ECOSSE). User-Manual. Available online at: https://www.abdn.ac.uk/staffpages/uploads/soi450/ECOSSE%20User%20manual%20310810.pdf.
Spahni, R., Joos, F., Stocker, B. D., Steinacher, M., and Yu, Z. C. (2013). Transient simulations of the carbon and nitrogen dynamics in northern peatlands: from the Last Glacial Maximum to the 21st century. Clim. Past 9 (3), 1287–1308. doi:10.5194/cp-9-1287-2013
St-Hilaire, F., Wu, J., Roulet, N. T., Frolking, S., Lafleur, P. M., Humphreys, E. R., et al. (2010). McGill wetland model: evaluation of a peatland carbon simulator developed for global assessments. Biogeosciences 7 (11), 3517–3530. doi:10.5194/bg-7-3517-2010
Stocker, B. D., Spahni, R., and Joos, F. (2014). DYPTOP: a cost-efficient TOPMODEL implementation to simulate sub-grid spatio-temporal dynamics of global wetlands and peatlands. Geosci. Model Dev. 7 (6), 3089–3110. doi:10.5194/gmd-7-3089-2014
Szafranek-Nakonieczna, A., and Stêpniewska, Z. (2014). Aerobic and anaerobic respiration in profiles of polesie lubelskie peatlands. Int. Agrophysics 28 (2), 219–229. doi:10.2478/intag-2014-0011
Thomas, K. L., Benstead, J., Davies, K. L., and Lloyd, D. (1996). Role of wetland plants in the diurnal control of CH4 and CO2 fluxes in peat. Soil Biol. Biochem. 28 (1), 17–23. doi:10.1016/0038-0717(95)00103-4
Uhelski, D. M., Kane, E. S., and Chimner, R. A. (2022). Plant functional types drive Peat Quality differences. Wetlands 42 (5), 51. doi:10.1007/s13157-022-01572-4
Updegraff, K., Bridgham, S. D., Pastor, J., and Weishampel, P. (1998). Hysteresis in the temperature response of carbon dioxide and methane production in peat soils. Biogeochemistry 43 (3), 253–272. doi:10.1023/A:1006097808262
Updegraff, K., Pastor, J., Bridgham, S. D., and Johnston, C. A. (1995). Environmental and substrate controls over carbon and nitrogen mineralization in northern wetlands. Ecol. Appl. 5 (1), 151–163. doi:10.2307/1942060
Upton, A., Vane, C. H., Girkin, N., Turner, B. L., and Sjögersten, S. (2018). Does litter input determine carbon storage and peat organic chemistry in tropical peatlands? Geoderma 326, 76–87. doi:10.1016/j.geoderma.2018.03.030
van Huissteden, J., van den Bos, R., and Marticorena Alvarez, I. (2006). Modelling the effect of water-table management on CO2 and CH4 fluxes from peat soils. Neth. J. Geosciences 85 (1), 3–18. doi:10.1017/S0016774600021399
Van Lent, J., Hergoualc’h, K., Verchot, L., Oenema, O., and van Groenigen, J. W. (2019). Greenhouse gas emissions along a peat swamp forest degradation gradient in the Peruvian Amazon: soil moisture and palm roots effects. Mitig. Adapt. Strategies Glob. Change 24 (4), 625–643. doi:10.1007/s11027-018-9796-x
Vitt, D. H. (2013). “Peatlands,” in Encyclopedia of ecology. Editor B. Fath Second Edition (Oxford: Elsevier). doi:10.1016/B978-0-12-409548-9.00741-7
Waddington, J. M., Rotenberg, P. A., and Warren, F. J. (2001). Peat CO2 production in a natural and cutover peatland: implications for restoration. Biogeochemistry 54 (2), 115–130. doi:10.1023/A:1010617207537
Wang, H., Xu, Y., Kumar, A., Knorr, K.-H., Zhao, X., Perez, J. P. H., et al. (2023). Temperature and organic carbon quality control the anaerobic carbon mineralization in peat profiles via modulating microbes: a case study of Changbai Mountain. Environ. Res. 237, 116904. doi:10.1016/j.envres.2023.116904
Wang, Q., Zhao, X., Chen, L., Yang, Q., Chen, S., and Zhang, W. (2019). Global synthesis of temperature sensitivity of soil organic carbon decomposition: latitudinal patterns and mechanisms. Funct. Ecol. 33 (3), 514–523. doi:10.1111/1365-2435.13256
Wang, X., Li, X., Hu, Y., Lv, J., Sun, J., Li, Z., et al. (2010). Effect of temperature and moisture on soil organic carbon mineralization of predominantly permafrost peatland in the Great Hing’an Mountains, Northeastern China. J. Environ. Sci. 22 (7), 1057–1066. doi:10.1016/S1001-0742(09)60217-5
Wania, R., Ross, I., and Prentice, I. C. (2009a). Integrating peatlands and permafrost into a dynamic global vegetation model: 1. Evaluation and sensitivity of physical land surface processes. Glob. Biogeochem. Cycles 23 (3). doi:10.1029/2008GB003412
Wania, R., Ross, I., and Prentice, I. C. (2009b). Integrating peatlands and permafrost into a dynamic global vegetation model: 2. Evaluation and sensitivity of vegetation and carbon cycle processes. Glob. Biogeochem. Cycles 23 (3). doi:10.1029/2008GB003413
Wania, R., Ross, I., and Prentice, I. C. (2010). Implementation and evaluation of a new methane model within a dynamic global vegetation model: LPJ-WHyMe v1.3.1. Geosci. Model Dev. 3 (2), 565–584. doi:10.5194/gmd-3-565-2010
Webster, K. L., McLaughlin, J. W., Kim, Y., Packalen, M. S., and Li, C. S. (2013). Modelling carbon dynamics and response to environmental change along a boreal fen nutrient gradient. Ecol. Model. 248, 148–164. doi:10.1016/j.ecolmodel.2012.10.004
Wiltshire, A. J., Burke, E. J., Chadburn, S. E., Jones, C. D., Cox, P. M., Davies-Barnard, T., et al. (2021). JULES-CN: a coupled terrestrial carbon–nitrogen scheme (JULES vn5.1). Geosci. Model Dev. 14 (4), 2161–2186. doi:10.5194/gmd-14-2161-2021
Wright, E. L., Black, C. R., Cheesman, A. W., Drage, T., Large, D., Turner, B. L., et al. (2011). Contribution of subsurface peat to CO2 and CH4 fluxes in a neotropical peatland. Glob. Change Biol. 17 (9), 2867–2881. doi:10.1111/j.1365-2486.2011.02448.x
Wu, Q., Ye, R., Bridgham, S. D., and Jin, Q. (2021). Limitations of the Q10 coefficient for quantifying temperature sensitivity of anaerobic organic matter decomposition: a modeling based assessment. J. Geophys. Res. Biogeosciences 126 (8). doi:10.1029/2021JG006264
Wu, Y., and Blodau, C. (2013). PEATBOG: a biogeochemical model for analyzing coupled carbon and nitrogen dynamics in northern peatlands. Geosci. Model Dev. 6 (4), 1173–1207. doi:10.5194/gmd-6-1173-2013
Wu, Y., Verseghy, D. L., and Melton, J. R. (2016). Integrating peatlands into the coupled Canadian land surface scheme (CLASS) v3.6 and the Canadian terrestrial ecosystem model (CTEM) v2.0. Geosci. Model Dev. 9 (8), 2639–2663. doi:10.5194/gmd-9-2639-2016
Yan, Z., Bond-Lamberty, B., Todd-Brown, K. E., Bailey, V. L., Li, S., Liu, C., et al. (2018). A moisture function of soil heterotrophic respiration that incorporates microscale processes. Nat. Commun. 9 (1), 2562. doi:10.1038/s41467-018-04971-6
Yavitt, J. B., Williams, C. J., and Wieder, R. K. (2000). Controls on microbial production of methane and carbon dioxide in three sphagnum-dominated peatland ecosystems as revealed by a reciprocal field peat transplant experiment. Geomicrobiol. J. 17 (1), 61–88. doi:10.1080/014904500270503
Ye, R., Keller, J. K., Jin, Q., Bohannan, B. J. M., and Bridgham, S. D. (2016). Peatland types influence the inhibitory effects of a humic substance analog on methane production. Geoderma 265, 131–140. doi:10.1016/j.geoderma.2015.11.026
Young, D. M., Baird, A. J., Morris, P. J., Dargie, G. C., Mampouya Wenina, Y. E., Mbemba, M., et al. (2023). Simulating carbon accumulation and loss in the central Congo peatlands. Glob. Change Biol. 29 (23), 6812–6827. doi:10.1111/gcb.16966
Keywords: tropical peat, soil heterotrophic respiration, carbon dioxide, temperature sensitivity, moisture effect, process-based models, peat decomposability
Citation: Dehaen EM, Burke EJ, Chadburn SE, Kaduk J, Sitch S, Smith ND and Gallego-Sala AV (2025) Drivers of soil heterotrophic respiration in tropical peatlands: a review to inform peat carbon accumulation modelling. Front. Geochem. 3:1492386. doi: 10.3389/fgeoc.2025.1492386
Received: 06 September 2024; Accepted: 17 March 2025;
Published: 04 April 2025.
Edited by:
Gabriela Bielefeld Nardoto, University of Brasilia, BrazilReviewed by:
Anderson da Rocha Gripp, Federal University of Rio de Janeiro, BrazilCopyright © 2025 Dehaen, Burke, Chadburn, Kaduk, Sitch, Smith and Gallego-Sala. This is an open-access article distributed under the terms of the Creative Commons Attribution License (CC BY). The use, distribution or reproduction in other forums is permitted, provided the original author(s) and the copyright owner(s) are credited and that the original publication in this journal is cited, in accordance with accepted academic practice. No use, distribution or reproduction is permitted which does not comply with these terms.
*Correspondence: Elise M. Dehaen, ed515@exeter.ac.uk
Disclaimer: All claims expressed in this article are solely those of the authors and do not necessarily represent those of their affiliated organizations, or those of the publisher, the editors and the reviewers. Any product that may be evaluated in this article or claim that may be made by its manufacturer is not guaranteed or endorsed by the publisher.
Research integrity at Frontiers
Learn more about the work of our research integrity team to safeguard the quality of each article we publish.