- 1British Antarctic Survey, Cambridge, United Kingdom
- 2Antarctic Climate and Ecosystems Cooperative Research Centre, University of Tasmania, Hobart, TAS, Australia
- 3Virginia Institute of Marine Science, College of William and Mary, Gloucester Point, VA, United States
- 4SKLEC-NIVA Centre for Marine and Coastal Research, East China Normal University, Shanghai, China
- 5Norwegian Institute for Water Research, Bergen, Norway
- 6Australian Antarctic Division, Australian Commonwealth Department of Environment and Energy, Kingston, TAS, Australia
- 7Department of Ecology and Evolutionary Biology, University of California, Santa Cruz, Santa Cruz, CA, United States
- 8Center for Coastal Physical Oceanography, Old Dominion University, Norfolk, VA, United States
- 9Alfred-Wegener-Institut Helmholtz Zentrum für Polar- und Meeresforschung, Bremerhaven, Germany
- 10Departamento das Ciências da Vida, Marine and Environmental Sciences Centre, Universidade de Coimbra, Coimbra, Portugal
Increasing concern about the impacts of climate change on ecosystems is prompting ecologists and ecosystem managers to seek reliable projections of physical drivers of change. The use of global climate models in ecology is growing, although drawing ecologically meaningful conclusions can be problematic. The expertise required to access and interpret output from climate and earth system models is hampering progress in utilizing them most effectively to determine the wider implications of climate change. To address this issue, we present a joint approach between climate scientists and ecologists that explores key challenges and opportunities for progress. As an exemplar, our focus is the Southern Ocean, notable for significant change with global implications, and on sea ice, given its crucial role in this dynamic ecosystem. We combined perspectives to evaluate the representation of sea ice in global climate models. With an emphasis on ecologically-relevant criteria (sea ice extent and seasonality) we selected a subset of eight models that reliably reproduce extant sea ice distributions. While the model subset shows a similar mean change to the full ensemble in sea ice extent (approximately 50% decline in winter and 30% decline in summer), there is a marked reduction in the range. This improved the precision of projected future sea ice distributions by approximately one third, and means they are more amenable to ecological interpretation. We conclude that careful multidisciplinary evaluation of climate models, in conjunction with ongoing modeling advances, should form an integral part of utilizing model output.
Introduction
Natural variability in the climate system and anthropogenic climate change result in a complex array of physical and biological responses. Marine ecosystems are inextricably connected to the climate system and significant changes to their structure and function are both observed and expected (Doney et al., 2012; Blois et al., 2013; Sydeman et al., 2015). Effects may be direct (e.g., temperature changes affecting physiological processes such as growth, reproduction, consumption and respiration), or indirect (e.g., those resulting from changes to primary productivity, which in turn can influence species abundance, distributions and interactions; Constable et al., 2014). Biological feedbacks to the climate system such as the role of biology in carbon sequestration are recognized (Hauck and Völker, 2015; Hickman, 2015) although difficult to quantify (Passow and Carlson, 2012).
Concern about the effects of climate change on marine ecosystems is growing (Hoegh-Goldberg and Bruno, 2010; Cheung et al., 2013; Halpern et al., 2015) and is a major focus of global environmental research programmes and targets such as Future Earth, the Scientific Committee on Oceanic Research (SCOR) and the United Nations Sustainable Development Goals. Ecologists are increasingly being asked to provide advice in this regard to support conservation and management decisions (Hollowed et al., 2013; Barange et al., 2014; García Molinos et al., 2015). Understanding and predicting these effects requires knowledge of the processes that determine the distribution and abundance of species, the structure and functioning of ecosystems within which they occur, and their past and present dynamics (Southward et al., 1995; Mieszkowska et al., 2006; Lima et al., 2007; Doney et al., 2012; Murphy et al., 2012). This is coupled with the need to understand the key physical drivers of change and for reliable projections of them. As such, the use of Intergovernmental Panel on Climate Change (IPCC)-class climate models (Box 1) is rapidly gaining momentum in ecological studies of change (Hunter et al., 2010; Bopp et al., 2013; Jenouvrier et al., 2014; Piñones and Fedorov, 2016).
BOX 1. IPCC-class climate models.
By “IPCC-class models” we refer to full complexity coupled atmosphere-ocean-sea ice- climate and earth system models. This type of model is a fundamental tool for quantifying how the environment may change in the future. They are increasingly able to take account of complex processes, including the “ozone hole,” carbon uptake in the ocean, and atmospheric aerosols. Because of the complexity, non-linearity and small horizontal scale of many meteorological and oceanographic processes, these models require intensive computer processing power and are therefore run at only a few dozen climate research centres. They can be run with “pre-industrial” concentrations of greenhouse gases (GHG) before anthropogenic forcing is introduced (from mid-nineteenth century) to quantify the impacts of the known increase in greenhouse gas concentrations and the development of the ozone hole. In addition, possible trajectories of twenty-first century climate change can be derived from climate model simulations run under different (GHG) emission scenarios and recovery of stratospheric ozone amounts.
Future GHG emissions will be the product of complex dynamic systems, determined by a combination of political decisions, demography, socio-economic development and technological change. Their evolution is highly uncertain and a range of plausible scenarios have been defined to provide a common set of future emission outcomes that can be used to help compare results across different climate models. The set of scenarios of future change in climate forcings that were used in the IPCC Fifth Assessment Report (AR5) are termed Representative Concentration Pathways (RCPs) (Meinshausen et al., 2011). There are four RCPs (RCP8.5 which corresponds to the pathway with the highest GHG emissions, followed by RCP6, RCP4.5, and RCP2.6), each one represents a different trajectory and cumulative emission concentration to 2100.
A major initiative that provided much of the climate model data for the most recent IPCC report is the Coupled Model Intercomparison Project Phase 5 (CMIP5, managed by the World Climate Research Programme) (Taylor et al., 2012). The CMIP5 dataset is a comprehensive set of outputs from approximately 60 of the world's most sophisticated climate models from major climate modeling centres (such collections are often referred to as climate model ensembles). The CMIP model intercomparisons have historically been synchronized with the preparation phases of IPCC reports, hence the models (and model setups) are often referred to as “IPCC-class” models. The most up-to-date climate models are currently being prepared for CMIP6 in advance of the IPCC Sixth Assessment Report (AR6), which is scheduled to be released around 2021-2022.
Although these models are designed to provide realistic representations of the climate system and projections of climate variables (e.g., atmospheric and ocean temperatures, sea ice extent and winds) that are known to influence ecological processes, applying them to ecological problems is challenging (Stock et al., 2011; Snover et al., 2013; Harris et al., 2014). No model is perfect and none reproduce all current and past climates, so projections need to be used with care. There are important caveats that users must consider and these issues are likely to be compounded in the ecosystem context where additional considerations, such as differences in spatial and temporal scale, may be significant. Most experiments using IPCC-class models are designed to provide projections on time scales of the order multiple decades (>30 years) and tend to be run with spatial grids of around 200 km. On such temporal scales, climate change signals should be clearly distinguishable from natural variability. However, from an ecological perspective, shorter (<30 years) time frames are key due to biological processes, including life cycles, generation lengths and phenology (Stock et al., 2011), and the time frames of human activities (such as fishing) and political decision-making. At these shorter temporal scales it is difficult or impossible to distinguish between natural variability and the background climate change signal (Macias et al., 2013). Furthermore, many key ecological processes occur at regional (i.e., tens to hundreds of kilometers in extent) or smaller scales, hence biological responses to change also vary at these scales (Helmuth et al., 2006; Clarke et al., 2009; Peck, 2011; Chave, 2013). Similarly, resource conservation and management is also often concerned with regional or smaller scales (e.g., subareas, divisions or subdivisions in the case of fishing areas) that may contain relatively discrete populations of certain species (Stock et al., 2011; Sydeman et al., 2015).
Here we focus on the Southern Ocean as an exemplar region for exploring the use of IPCC-class models in studies of ecological change. The region was the subject of much attention during IPCC Fifth Assessment Report (AR5), owing to its important role in the global climate system and the significant changes already observed (Le Quere et al., 2007; Böning et al., 2008; Hauck et al., 2013; Kennicutt and Chown, 2014; Larsen et al., 2014; Landschützer et al., 2015). One of the most notable changes in this region has been the increase in westerly wind speeds associated with stratospheric ozone depletion (Turner et al., 2009; Thompson et al., 2011; Meijers, 2014). There is strong evidence that this has had important effects on the Southern Ocean, in particular sea ice (Ferreira et al., 2015). However, major issues remain with regard to reproducing the observed physical state of the Southern Ocean in global climate models, and these have implications for the reliability of predicted responses to future climate forcing (Turner et al., 2013; Meijers, 2014).
Considerable progress has been made with qualitative assessments of the effects of physical changes on Southern Ocean ecosystems, revealing key drivers (including sea ice, wind, various water mass properties and mixed layer depth) and complex responses throughout the food web (e.g., Murphy et al., 2007; Massom and Stammerjohn, 2010; Gutt et al., 2012; Constable et al., 2014; Hunt et al., 2016; Xavier et al., 2016). Broadly, the effects are likely to include impacts on habitat, physiology, distribution, population densities, phenology, and community interactions (Trathan and Agnew, 2010). Quantitative assessments are more difficult. Relevant biological data are often patchy or imprecisely known, while effects are often indirect and multifaceted (Bednarek et al., 2011; Murphy et al., 2012; Melbourne-Thomas et al., 2013; Gutt et al., 2015). This makes the challenges of assessing future change even greater (Hill et al., 2013; Kawaguchi et al., 2013; Melbourne-Thomas et al., 2016).
Despite the challenges, quantitative assessments of the effects of change are urgently required, not least because the conservation and management of the Southern Ocean must account for the dual realities of climate-driven ecosystem change and growing demand for fishery resources (Murphy et al., 2008; CCAMLR, 2009; Trathan and Agnew, 2010; Hill et al., 2013). Input from the scientific community is critical in providing policy-relevant information on climate change impacts for use in decision making.
IPCC-class models are essential tools for quantitatively integrating knowledge of the climate system and making projections. There are general recommendations for ecologists on their use (e.g., Snover et al., 2013; Harris et al., 2014), but information specifically for climate scientists about the requirements of ecologists is lacking. Furthermore, while generic guidelines are useful, detail specific to particular regions is also important, and expert-agreed benchmarks would be valuable. Here, with our focus on the Southern Ocean, and on sea ice, given its crucial role in this dynamic system (Box 2), we ask how these models can be used most effectively to understand, and manage, the responses of species, communities and ecosystems to change. Our study represents a first step in bringing members of the Southern Ocean climate and ecological community together to jointly explore key challenges and consider opportunities for progress.
BOX 2. Southern Hemisphere sea ice.
Southern Hemisphere sea ice has a range of influences in the Earth System including on global heat and carbon cycles, and sea-level rise, and is a sensitive indicator of change in the Polar Regions; therefore, understanding its variability in the climate system is critical. It forms one of the largest, most dynamic marine ecosystems on Earth, with marine biota being highly adapted to its presence, seasonality and properties over evolutionary timescales (Clarke et al., 2007; Massom and Stammerjohn, 2010; Constable et al., 2014). Overall, sea ice extent around the Antarctic ranges from a late winter peak of ~19 million km2 to a minimum of ~3–4 million km2 in summer (Massom and Stammerjohn, 2010). Since the late 1970s the annual mean total Southern Hemisphere sea ice extent has increased by ~3%, albeit with considerable regional contrast and variability (Turner et al., 2013; Gagné et al., 2015; Simmonds, 2015). However, as the impacts of shorter term variability (associated with natural variability and ozone depletion) recede over longer time frames, this overall increasing trend is projected to reverse in direction (Bracegirdle et al., 2008; Turner et al., 2013).
Materials and Methods
Capabilities and Limitations of IPCC-class Climate Models
The first step in this study was the compilation of a set of key questions that Southern Ocean ecologists would ideally like to address through the use of climate models. To begin to consider the challenges of using IPCC-class models to address such questions, we focused in detail on one important driver, sea ice, owing to its crucial role in the Southern Ocean (Box 2) and identified properties of particular ecological significance.
Sea Ice in CMIP5 Models
We evaluated the representation of Southern Hemisphere sea ice in CMIP5 models with an emphasis on extent and seasonality due to the crucial ecological roles of these properties (see below). To assess which models most closely reproduced aspects of the Southern Ocean sea ice extent (SIE) cycle (e.g., late-summer minimum and amplitude of the annual cycle), we used selection criteria following the methodology of the Arctic sea ice analysis of Wang and Overland (2009), adjusted to reflect the timing of austral seasons. This specifies that simulated sea ice must fall within ±20% of satellite data for mean minimum SIE and mean seasonality (where seasonality is the annual difference between the maximum and minimum SIE).
Data
For the analysis presented here monthly-mean sea ice concentration data was used (CMIP5 variable name “sic”), which is the proportion of each model grid cell that is covered by sea ice. Output from two different types of model experiments were retrieved. The CMIP5 data can be downloaded from the Earth System Grid https://pcmdi.llnl.gov/projects/esgf-llnl/. Firstly “historical” simulations, which are run with observed concentrations of greenhouse gases and other known important climate drivers between the mid-nineteenth century to the present. Second, global warming simulations following a scenario of high emissions of greenhouse gases (RCP8.5). Future climate conditions are taken from the late twenty-first century in the RCP8.5 simulations (2079-2099) and these are compared against baseline (i.e., as a reference period from which to define twenty-first century change and over which to compare observations against climate model output) conditions taken from the period 1979-1999 in the historical simulations. After first eliminating the models for which both monthly sea ice concentration data and data for the RCP8.5 high emissions scenario were not available, a total of 35 different CMIP5 models were identified (see below). These baseline conditions were also used for comparing models and observations in the sub-setting procedure. The dataset used was the Bootstrap version 2 product described in Comiso (2000; updated 2015).
Sea Ice Diagnostics and CMIP5 Model Selection
The basic parameter used for evaluation was total Southern Hemisphere sea ice extent (SIE), which was calculated from monthly mean sea ice concentration data (both the CMIP5 and satellite Bootstrap 2 datasets). It was defined as the area enclosed by the 15% concentration contour of the sea ice concentration fields over the Southern Hemisphere.
Further details are provided in Supplementary Material Methods.
Results
Capabilities and Limitations of IPCC-class Climate Models
Considering the key questions identified by Southern Ocean ecologists alongside current capabilities and limitations of the models, revealed fundamental differences in perspectives and approaches between the two disciplines. These include methods; research interests and priorities; requirements of the models (Supplementary Table 1); and terminology (Supplementary Table 2). Issues around temporal and spatial scales were noted in particular, as was the need to resolve detailed features such as the Marginal Ice Zone (MIZ) (i.e., the transition area between open ocean and sea ice).
Sea Ice As an Exemplar Variable
Focusing on sea ice as an exemplar variable, information was compiled on properties of particular ecological relevance. Sea ice forms an essential surface habitat for resting, breeding and feeding, and sub-surface habitat for food and refugia, it also influences water column properties, and affects the reproductive cycles, recruitment and foraging behavior of a wide range of species. It plays a pivotal role in Southern Ocean biogeochemical cycles, and influences fisheries, not only through its impact on the distribution and potentially the abundance of target species, but by affecting the access of vessels to fishing grounds. It is clear that the seasonal advance and retreat of sea ice (timing and extent) is a major driver of the structure and functioning of the Southern Ocean pelagic ecosystem, and this formed the basis for our evaluation of the models (see Materials and Methods). Supplementary Table 3 summarizes the information collated on sea ice. Recognizing the range of multiple stressors involved, Supplementary Table 3 also includes information for other key drivers, detailing aspects of these that are particularly important ecologically, providing a baseline for future work.
Sea Ice in CMIP5 Models
The criteria we applied reduced the available 35 models (Supplementary Table 4) (referred to hereafter as the full ensemble) to a subset of eight models (referred to hereafter as the subset). The importance of this sub-setting is presented below.
Effect of Sub-Setting on Historical and Future CMIP5-Derived Sea Ice Distributions
Late twentieth century sea ice distributions from the full ensemble are shown both for austral summer (December-February) (Figure 1A) and winter (June-August) (Figure 2A). These highlight the large ranges in the summer and winter distributions in the full ensemble for the 1979-99 period. Large ranges are also seen in projected sea ice distributions in the late twenty-first century (Figures 1B, 2B).
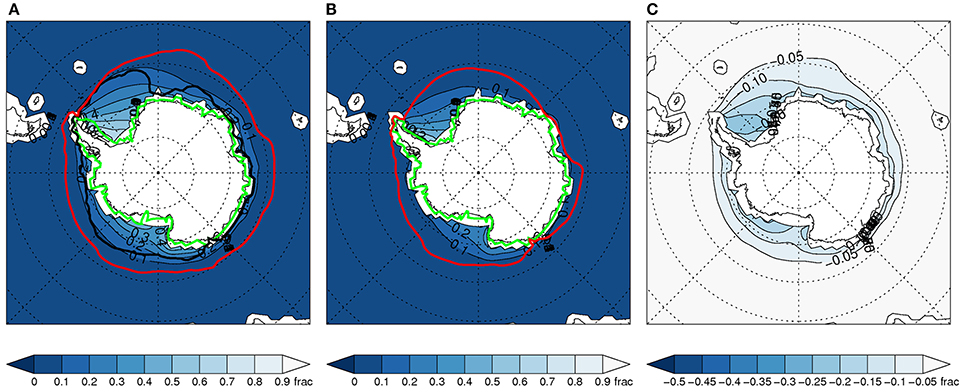
Figure 1. Austral summer (DJF) sea ice distribution for all available 35 models from historical simulations over the period 1979-1999 (A) and RCP8.5 (2079-2099) (B) and the RCP8.5-historical difference (C). On each plot the filled contours show the all-model ensemble mean sea ice concentration, the red lines show the ice edge for the model with most ice and the green lines show the ice edge for the model with least ice. The black lines on (A) show the observed extent (for 1979-1999 period) from the Comiso Bootstrap 2 dataset (see Materials and Methods).
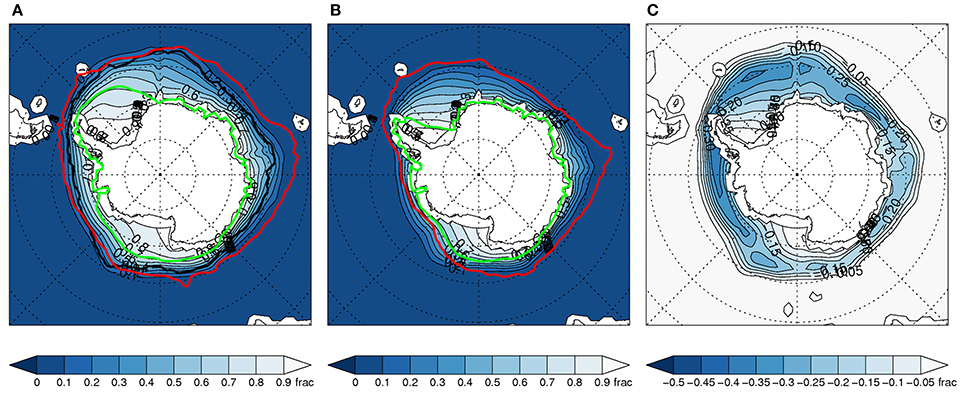
Figure 2. As Figure 1 above, but for winter (JJA).
The inter-model range of sea ice in the historical runs is much smaller for the subset (Figures 3A, 4A). This is expected since those with large deviations have been removed. However, Figures 3B, 4B illustrate that this narrower range is maintained in the projected sea ice spatial distribution in the late twenty-first century. This emphasizes that model-projected future sea ice distributions (and associated variables) are highly dependent on the match between simulated and observed climatological SIE conditions (Risbey et al., 2014; Bracegirdle et al., 2015).
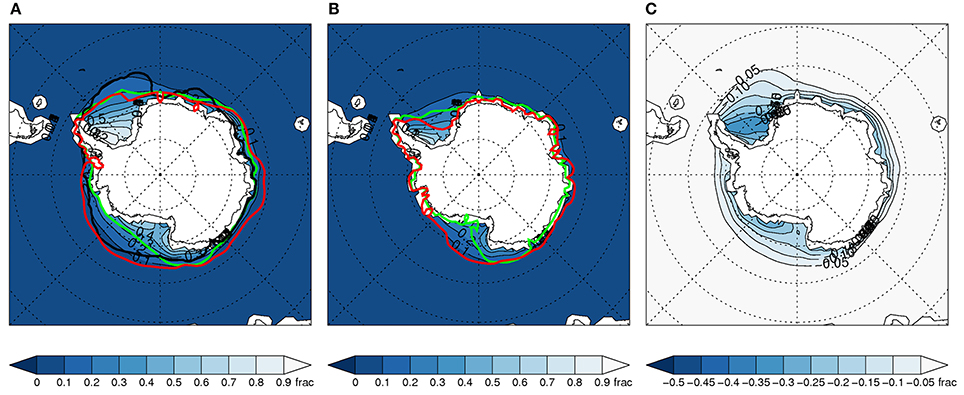
Figure 3. As Figure 1 above, but for the subset of 8 models.
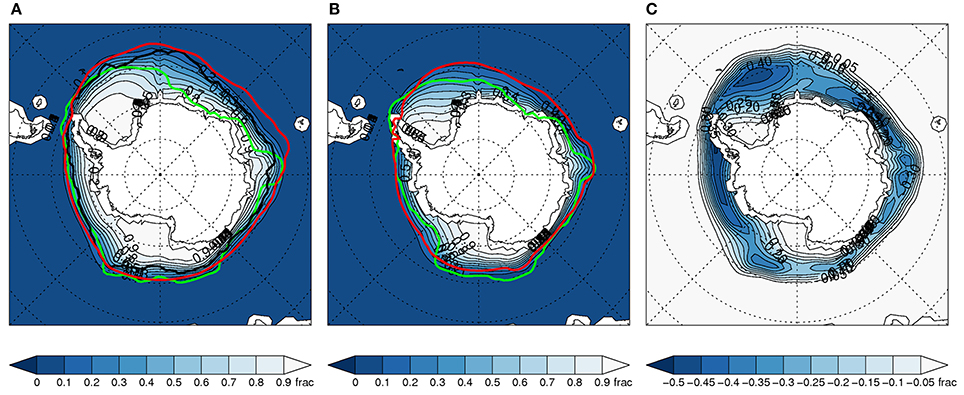
Figure 4. As Figure 2 above, but for the subset of 8 models.
In terms of ensemble mean change in sea ice concentration, it is evident that the subset gives more clearly defined regions of ice reduction with larger changes in many regions. This is particularly apparent in winter (Figures 2C, 4C). These regional differences between the full ensemble and subset would likely be more distinct in evaluations of sea ice at specific locations or within specific sectors.
The mean twenty-first century change in SIE is similar both in the full ensemble and the subset, with respective changes of 49 and 52% in summer and 31% for both in the winter. However, the range in projected CMIP5 SIE change is smaller across models that more closely reproduce the observed SIE climatology, with a standard deviation from 12 to 8% in winter and a reduction for the summer from 27 to 19% (Supplementary Table 5). It should be noted that proportional changes are not as strongly influenced by weighting, since, for example, models with too little sea ice produce smaller absolute changes that are a similar proportion of their own too-small SIE.
Discussion
Sea Ice in IPCC-class Models
The first step in the study highlighted the importance of understanding the nature and capabilities of models and the need for careful communication and interpretation of their outputs. Crucially, we have demonstrated the importance of model evaluation as a means to improve projections of change. For both communities, it is imperative that models reproduce the historical climate satisfactorily. In the case of sea ice this is a necessary (i.e., a biased baseline climatology will produce a biased projection) but, not sufficient (i.e., an accurate baseline climatology will not necessarily produce an accurate projection of future change) condition for producing reliable projections of future change. Despite the large differences between observed and simulated climatological sea ice extent in many climate models (Turner et al., 2013), previous projections for Southern Hemisphere sea ice have either treated all CMIP models equally (Collins et al., 2013) or used weightings based on other variables (Bracegirdle et al., 2008).
Our analysis shows that applying criteria, motivated by ecological considerations, to select a subset of the available CMIP5 models dramatically reduces the range in projected late-twenty-first century sea ice distribution and twenty-first minus twentieth century absolute sea ice extent change. By better representing Southern Hemisphere sea ice extent and seasonality, the subset provides more ecologically meaningful results (Figures 1–4). Box 3 captures some of the potential consequences for projecting ecosystem change based on poorly represented sea ice extent and seasonality.
BOX 3. Implications of using models that poorly represent Southern Hemisphere sea ice to project ecological responses to change.
Efforts to incorporate biology into physical models tend to include only components of the lower levels (e.g., phytoplankton) (Murphy et al., 2012). However, physical changes can affect all trophic levels. Sea ice has complex multi-directional effects throughout the food web that influence the structure, function and dynamics of Southern Ocean ecosystems (Figure 5, Supplementary Table 3). These can be direct effects (D) as with the provision of crucial habitat and its impact as a physical barrier. They can also be indirect (I) through effects on physical conditions such as upper ocean temperature, irradiance and vertical mixing that in turn influence key ecological processes, food type and availability, and species composition, distributions and abundance.
To capture some of the consequences of using models that poorly represent sea ice here we present a simplified view of some of its key effects in a Southern Ocean pelagic food web (for examples of detailed Southern Ocean food webs see, e.g., Ducklow et al., 2007; Murphy et al., 2007, 2012, 2013; Smith et al., 2007). Consider a model(s) that has sea ice melting a month early or a month late. The details of confounding factors and interactions aside, poorly-representing sea ice (in this case, melting too early or too late) will significantly impact phytoplankton and hence the overall productivity of the system:
Physical factors—One possible (greatly simplified) outcome is that ice melting too early exposes the water column to increased light, as well as increased air-sea interaction. The opposite would be the case for late melting.
Phytoplankton (a)—The early melting would provide increased irradiance for phytoplankton growth, altering bloom timing, composition and extent (D). Productivity would increase under these conditions. Conversely, productivity is likely to decrease if melting is late. There will also be indirect effects, e.g., on vertical flux (I).
Zooplankton (b and c)—Phytoplankton dynamics markedly influence zooplankton dynamics. If the ice melt is too early or too late, this will affect food availability and phytoplankton-zooplankton coupling (I). The effects of melt timing on sea ice as a physical habitat are also important, for example this may affect essential overwintering habitat for zooplankton larvae and disrupt life history patterns (D).
Fish and air-breathing predators (d and e)—Given the above effects, the timing of ice melt also influences the availability of prey (e.g., krill) for fish and higher predators (whales, seals and penguins) (I). Many Southern Ocean fish and higher predators are directly dependent on sea ice as a habitat for feeding, breeding and haul-out for which timing is crucial (D). Its presence or absence can also affect access to breeding and feeding grounds (D).
While there will always be associated uncertainty, by reducing the range and thereby better representing sea ice (Figures 1–4), the more confident we can be in the model output, and in this case, in generating ecologically-relevant results (Figure 6).
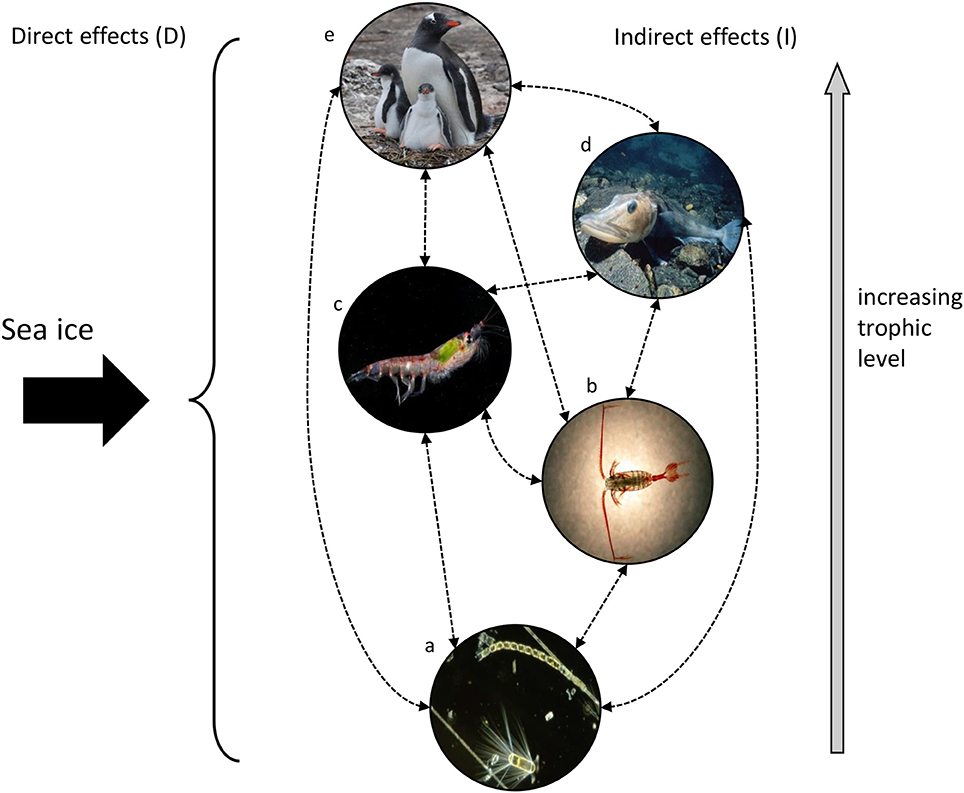
Figure 5. A simplified representation of a Southern Ocean pelagic food web, where the trophic levels are represented by phytoplankton (a), small zooplankton (copepods) (b), large zooplankton (krill) (c), fish (d), and air-breathing predators (seals, whales, penguins) (e). Sea ice exerts direct (D) (black arrow) and indirect (I) (dotted lines) effects at each trophic level.
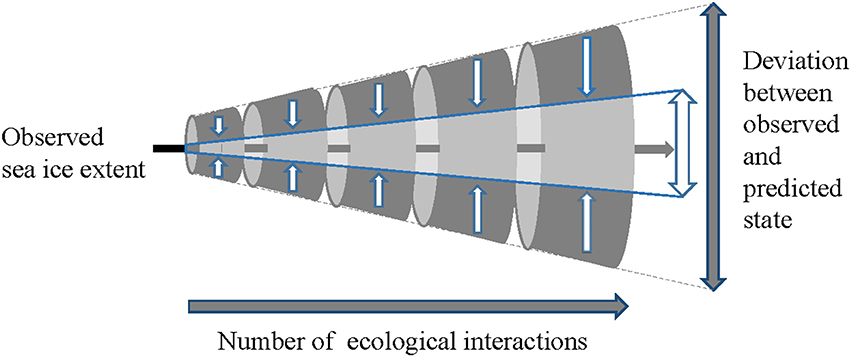
Figure 6. Schematic illustrating that the deviation between observed (actual) and predicted state (in this case sea ice extent) has the potential to amplify as ecological complexity increases. The pale central area and white arrows represent reductions in uncertainty by using the best available models (in this case the eight-model subset).
Key Challenges and Recommendations
Therefore, as a first order assessment of the utility of IPCC-class climate models in Southern Ocean ecosystem research, and in particular sea ice, we propose that ecologists use the eight-model subset and associated projected sea ice changes presented above. However, interpretation of these must be accompanied by an understanding of the associated uncertainty and caveats. Key issues include the subjectivity in defining thresholds for sub-setting (e.g., the 20% threshold used in this study), and the possibility that the “good” models share common biases and “get the right answer for the wrong reason.” Nevertheless, it is clear that a necessary condition for capturing realistic changes in ice-edge environments would be a satisfactory representation of past and current conditions (Bracegirdle et al., 2015). The sub-setting technique used here improved projections by taking this into account. The difficulty comes in determining the reliability of changes exhibited in sub-sets of “better” models. As the understanding of how to deal with these and other caveats (Supplementary Table 1) progresses within the climate modeling community, it will be important for those interested in climate change impacts on ecosystems to remain actively involved.
Challenges remain in Southern Ocean modeling, and many of these are common to areas other than the Antarctic (Murphy et al., 2012; IPCC, 2013; Giorgi and Gutowski, 2015). Some of these are particularly pressing for ecologists and those involved in conservation and management decision-making. Due to the timescale of many ecological processes, projections of change for the next two to three decades are required (Trathan and Agnew, 2010). Such timescales are also important for those studying sea ice and other aspects of the climatology. However, on such decadal time scales future change will be dominated by natural variability of the climate system which is challenging to predict with the current climate modeling tools (Meehl et al., 2009; O'Kane et al., 2013; Risbey et al., 2014). This results in a mismatch in temporal scale between what the models can deliver and the relevant time-window for ecological considerations (Massom and Stammerjohn, 2010; Macias et al., 2013; Supplementary Table 1).
Given the variability in sea ice around the continent, and the fact that many ecological processes, and conservation and management decisions, take place at relatively small spatial scales, improving the regional performance of climate models is another clear need (Supplementary Table 1). As a case in point, sea ice is highly variable in the Antarctic Peninsula and Scotia Sea region (Murphy et al., 2014; Turner et al., 2016) which is also the location of the main commercial fishery for Antarctic krill (CCAMLR, 2015). The timing of regional ice arrival, duration and retreat significantly influences habitat availability, food type and availability, species distributions and vessel access (Supplementary Table 3). Furthermore, the MIZ is an area of great ecological importance, yet this and other detailed features such as eddies are very difficult to capture in climate models due to their small spatial scale (Supplementary Table 1).
By beginning to collectively understand and address these needs (and others documented in Supplementary Table 1), information from climate models can be more usefully applied, and future research priorities can be jointly determined and addressed. In the meantime, if the sea ice projections are to be used, for example, to understand the changing dynamics of individual species at regional or even local spatial scales, then reconciling the information from global climate models requires careful interpretation. However, in some situations, particularly for more immediate (urgent) and high-level decision-making, a high degree of complexity may not always be required. For example, the projections could be used to help identify where particular research efforts should be concentrated, or in highlighting the main trends, at least in terms of direction and range of change. Large-scale projections can also help to focus more detailed studies. Smaller-scale regional studies and models are invaluable and continue to be developed for the Southern Ocean (e.g., Pinkerton et al., 2010; Smith et al., 2014; Graham et al., 2016), and IPCC model projections may be considered as boundary forcing for downscaled, regional or local fields. Regional climate downscaling is a growing field of research, with CORDEX providing an example (Dosio et al., 2014; Katragkou et al., 2015), although this needs to be carefully applied with an appreciation of its strengths and weaknesses (Grose et al., 2012; Corney et al., 2013).
In essence, a combination of large-scale and regional information is key for the conservation and management of Antarctic marine resources. The circumpolar nature and high connectivity of the Southern Ocean, including to the global ocean, means that a large-scale view is crucial in understanding change (Murphy et al., 2008, 2012). Both regional and large-scale information is required for ecosystem-based management such as that of the Antarctic krill fishery in the Scotia Sea. Regional information is needed to provide an understanding of how harvested populations, dependent species, and access to fishing areas may change over time (CCAMLR, 2016). However, because conditions further afield are known to influence the population dynamics of krill and their predators (see Supplementary Table 3 also Nicol et al., 2000; Thorpe et al., 2007; Atkinson et al., 2008; Loeb et al., 2008; Murphy et al., 2013; Melbourne-Thomas et al., 2016), large-scale information is also valuable. Similarly, regional information is important in the design and implementation of marine protected areas (MPAs)—this might include ensuring appropriate protection for vulnerable areas or those identified as likely to be most resilient to change - with large-scale information needed to ensure that regions are not considered in isolation.
Future Work
This work initiates an iterative process, alongside climate model development for IPCC AR6, to provide model outputs (projections) that can serve as a common resource for use in ecological studies. This evaluation was concerned with processes at the ocean surface whereas different life stages of species can often be associated with deeper waters, thus our approach may not be optimal for whole system studies. In building a more comprehensive understanding of Southern Ocean change it must also be recognized that physical and biogeochemical changes do not act in isolation (Gruber, 2011; Bopp et al., 2013), although understanding and modeling the interactive and cumulative effects of multiple stressors presents a significant challenge (Murphy et al., 2012; Boyd et al., 2014; Gutt et al., 2015).
Progress can now be made in extending this work to evaluate model outputs for other key physical variables (e.g., winds, various water mass properties, mixed layer depth, temperature, and biogeochemical changes, see Supplementary Table 3) from an ecological perspective. Comparison of model results and performance with existing and emerging observations, data products and technology (e.g., new GLODAPv2 (Global Ocean Data Analysis Project Version 2), satellite products for chlorophyll, primary production, data from the Southern Ocean Continuous Plankton Recorder and Southern Ocean Observing System, FISH-MIP (Fisheries and Marine Ecosystem Model Intercomparison Project) and others (Rintoul et al., 2012; Constable et al., 2016; Olsen et al., 2016) will be an essential aspect of future work. In addition, more research is needed on the mechanisms that link the physical variables to ecological processes. Beyond that there is a need to consider ecological scenarios, including the effects of the recovery of over-exploited baleen whale populations (Branch et al., 2004; Noad et al., 2011; Tulloch et al., 2017) toward fully integrated system understanding. Future fishery scenarios are also required to explore how demand may change over time.
We advocate the need for synergy between the climate modeling community and a range of disciplines, including but certainly not limited to, marine ecologists, as state-of-the-art models continue to advance. We stress there is no prescriptive method, rather a combination of approaches is required. By refining large scale climate model output and carefully using large-scale projections, together with specific regional downscaling, the likely impacts of change on biota can be predicted and appropriate conservation and management decisions can be made.
Author Contributions
RC, EM, JT, TB, and NJ conceived the study; CK and TB analysed the data; TB, RC, EM, and JT interpreted the results; RC led the writing of the paper together with EM, JT, and TB; CK, SC, WS, and CW contributed to the writing of the paper; all other authors reviewed the manuscript; all authors approved the final version.
Conflict of Interest Statement
The authors declare that the research was conducted in the absence of any commercial or financial relationships that could be construed as a potential conflict of interest.
The handling Editor declared a shared affiliation, though no other collaboration, with one of the authors DW.
Acknowledgments
This paper builds on discussions that took place at a multidisciplinary workshop convened by the Integrating Climate and Ecosystem Dynamics in the Southern Ocean programme (ICED) and hosted by the British Antarctic Survey (BAS). We thank all the workshop participants. The study (and specifically RC, EM, NJ, JT, CK) was supported by ICED under a Natural Environment Research Council (NERC) International Opportunities Fund Grant NE/I029943/1, together with NERC core funding to BAS. Additional workshop funding was provided by Integrated Marine Biosphere Research (IMBeR). SC and AC were supported by the Australian Government's Cooperative Research Centres Programme through the Antarctic Climate and Ecosystems Cooperative Research Centre. JX was supported by the Investigator FCT program (IF/00616/2013) and this study benefited from the strategic program of MARE, financed by FCT (MARE- UID/MAR/04292/2013). We acknowledge Tony Philips (BAS) for downloading and managing local copies of the required CMIP5 data. We acknowledge the World Climate Research Programme's Working Group on Coupled Modelling (responsible for CMIP), and we thank the climate modelling groups (Supplementary Table 4) for producing and making their model output available. For CMIP the U.S. Department of Energy's Program for Climate Model Diagnosis and Intercomparison provides coordinating support and led development of software infrastructure in partnership with the Global Organization for Earth System Science Portals. All images belong to the British Antarctic Survey.
Supplementary Material
The Supplementary Material for this article can be found online at: http://journal.frontiersin.org/article/10.3389/fmars.2017.00308/full#supplementary-material
References
Atkinson, A., Siegel, V., Pakhomov, E. A., Rothery, P., Loeb, V., Ross, R. M., et al. (2008). Oceanic circumpolar habitats of Antarctic krill. Mar. Ecol. Prog. Ser. 362, 1–23. doi: 10.3354/meps07498
Barange, M., Merino, G., Blanchard, J. L., Scholtens, J., Harle, J., Allison, E. H., et al. (2014). Impacts of climate change on marine ecosystem production in societies dependent on fisheries. Nat. Clim. Chang. 4, 211–216. doi: 10.1038/nclimate2119
Bednarek, A. T., Cooper, A. B., Cresswell, K. A., Mangel, M., Satterthwaite, W. H., Simpfendorfer, C. A., et al. (2011). The certainty of uncertainty in marine conservation and what to do about it. Bull. Mar. Sci. 87, 177–195. doi: 10.5343/bms.2010.1060
Blois, J. L., Zarnetske, P. L., Fitzpatrick, M. C., and Finnegan, S. (2013). Climate change and the past, present, and future of biotic interactions. Science 341, 499–504. doi: 10.1126/science.1237184
Böning, C. W., Dispert, A., Visbeck, M., Rintoul, S. R., and Schwarzkopf, F. U. (2008). The response of the Antarctic Circumpolar Current to recent climate change. Nat. Geosci. 1, 864–869. doi: 10.1038/ngeo362
Bopp, L., Resplandy, L., Orr, J. C., Doney, S. C., Dunne, J. P., Gehlen, M., et al. (2013). Multiple stressors of ocean ecosystems in the 21st century: projections with CMIP5 models. Biogeosciences 10, 6225–6245. doi: 10.5194/bg-10-6225-2013
Boyd, P. W., Lennartz, S. T., Glover, D. M., and Doney, S. C. (2014). Biological ramifications of climate-change-mediated oceanic multi-stressors. Nat. Clim. Chang. 5, 71–79. doi: 10.1038/nclimate2441
Bracegirdle, T. J., Connolley, W. M., and Turner, J. (2008). Antarctic climate change over the twenty first century. J. Geophys. Res. 113:D03103. doi: 10.1029/2007JD008933
Bracegirdle, T. J., Stephenson, D. B., Turner, J., and Phillips, T. (2015). The importance of sea ice area biases in 21st century multimodel projections of Antarctic temperature and precipitation. Geophys. Res. Lett. 42, 10832–10839. doi: 10.1002/2015GL067055
Branch, T. A., Matsuoka, K., and Miyashita, T. (2004). Evidence for increases in Antarctic Blue Whales based on Bayesian modelling. Mar. Mamm. Sci. 20, 726–754. doi: 10.1111/j.1748-7692.2004.tb01190.x
CCAMLR (2016). CCAMLR, 2016. Report of the Thirty-third meeting of the Scientific Committee (SC-CAMLR-XXXIII) CCAMLR, Hobart, Australia.
Chave, J. (2013). The problem of pattern and scale in ecology: what have we learned in 20 years? Ecol. Lett. 16 (Suppl 1), 4–16. doi: 10.1111/ele.12048
Cheung, W. W., Watson, R., and Pauly, D. (2013). Signature of ocean warming in global fisheries catch. Nature 497, 365–368. doi: 10.1038/nature12156
Clarke, A., Griffiths, H. J., Barnes, D. K. A., Meredith, M. P., and Grant, S. M. (2009). Spatial variation in seabed temperatures in the Southern Ocean: implications for benthic ecology and biogeography. J. Geophys. Res. 114:G03003. doi: 10.1029/2008JG000886
Clarke, A., Johnston, N. M., Murphy, E. J., and Rogers, A. D. (2007). Introduction. Antarctic ecology from genes to ecosystems: the impact of climate change and the importance of scale. Philos. Trans. R. Soc. Lond. B. Biol. Sci. 362, 5–9. doi: 10.1098/rstb.2006.1943
Collins, M., Knutti, R., Arblaster, J., Dufresne, J. L., Fichefet, T., Friedlingstein, P., et al. (2013). “Long-term climate change: projections, commitments and irreversibility,” in Climate Change 2013: The Physical Science Basis. Contribution of Working Group I to the Fifth Assessment Report of the Intergovernmental. Panel on Climate, eds T. F. Stocker, D. Qin, G.-K. Plattner, M. Tignor, S. K. Allen, J. Boschung, A. Nauels, Y. Xia, V. Bex, and P. M. Midgley (Cambridge; New York, NY: Cambridge University Press), 1029–1136.
Comiso, J. C. (2000). Bootstrap Sea Ice Concentrations from Nimbus-7 SMMR and DMSP SSM/I-SSMIS. Version 2. Updated 2015. Boulder, CO: NASA DAAC at the National Snow and Ice Data Center.
Constable, A. J., Costa, D. P., Schofield, O., Newman, L., Urban, R. D., Fulton, E. A., et al. (2016). Developing priority variables (“ecosystem Essential Ocean Variables” — eEOVs). for observing dynamics and change in Southern Ocean ecosystems. J. Mar. Syst. 161, 26–41. doi: 10.1016/j.jmarsys.2016.05.003
Constable, A. J., Melbourne-Thomas, J., Corney, S. P., Arrigo, K. R., Barbraud, C., Barnes, D. K., et al. (2014). Climate change and Southern Ocean ecosystems I: how changes in physical habitats directly affect marine biota. Glob. Chang. Biol. 20, 3004–3025. doi: 10.1111/gcb.12623
Corney, S., Grose, M., Bennett, J. C., White, C., Katzfey, J., McGregor, J., et al. (2013). Performance of downscaled regional climate simulations using a variable-resolution regional climate model: Tasmania as a test case. J. Geophys. Res. Atmos. 118, 11936–11950. doi: 10.1002/2013JD020087
Doney, S. C., Ruckelshaus, M., Duffy, J. E., Barry, J. P., Chan, F., English, C. A., et al. (2012). Climate change impacts on marine ecosystems. Ann. Rev. Mar. Sci. 4, 11–37. doi: 10.1146/annurev-marine-041911-111611
Dosio, A., Panitz, H.-J., Schubert-Frisius, M., and Lüthi, D. (2014). Dynamical downscaling of CMIP5 global circulation models over CORDEX-Africa with COSMO-CLM: evaluation over the present climate and analysis of the added value. Clim. Dyn. 44, 2637–2661. doi: 10.1007/s00382-014-2262-x
Ducklow, H. W., Baker, K., Martinson, D. G., Quetin, L. B., Ross, R. M., Smith, R. C., et al. (2007). Marine pelagic ecosystems: the West Antarctic Peninsula. Philos. Trans. R. Soc. Lond. B. Biol. Sci. 362, 67–94. doi: 10.1098/rstb.2006.1955
Ferreira, D., Marshall, J., Bitz, C. M., Solomon, S., and Plumb, A. (2015). Antarctic ocean and sea ice response to ozone depletion: a two-time-scale problem. J. Clim. 28, 1206–1226. doi: 10.1175/JCLI-D-14-00313.1
Gagné, M. È., Gillett, N. P., and Fyfe, J. C. (2015). Observed and simulated changes in Antarctic sea ice extent over the past 50 years. Geophys. Res. Lett. 42, 90–95. doi: 10.1002/2014GL062231
García Molinos, J., Halpern, B., Schoeman, D., Brown, C. J., Kiessling, W., Moore, P. J., et al. (2015). Climate velocity and the future global redistribution of marine biodiversity. Nat. Clim. Chang. 6, 83–88. doi: 10.1038/nclimate2769
Giorgi, F., and Gutowski, W. J. (2015). Regional dynamical downscaling and the CORDEX initiative. Annu. Rev. Environ. Resour. 40, 467–490. doi: 10.1146/annurev-environ-102014-021217
Graham, J. A., Dinniman, M. S., and Klinck, J. M. (2016). Impact of model resolution for on-shelf heat transport along the West Antarctic Peninsula. J. Geophys. Res. Oceans 121, 7880–7897. doi: 10.1002/2016JC011875
Grose, M. R., Corney, S. P., Katzfey, J. J., Bennett, J. C., Holz, G. K., White, C. J., et al. (2012). A regional response in mean westerly circulation and rainfall to projected climate warming over Tasmania, Australia. Clim. Dyn. 40, 2035–2048. doi: 10.1007/s00382-012-1405-1
Gruber, N. (2011). Warming up, turning sour, losing breath: ocean biogeochemistry under global change. Philos. Trans. A. Math. Phys. Eng. Sci. 369, 1980–1996. doi: 10.1098/rsta.2011.0003
Gutt, J., Bertler, N., Bracegirdle, T. J., Buschmann, A., Comiso, J., Hosie, G., et al. (2015). The Southern Ocean ecosystem under multiple climate change stresses–an integrated circumpolar assessment. Glob. Chang. Biol. 21, 1434–1453. doi: 10.1111/gcb.12794
Gutt, J., Zurell, D., Bracegridle, T., Cheung, W., Clark, M. S., Convey, P., et al. (2012). Correlative and dynamic species distribution modelling for ecological predictions in the Antarctic: a cross-disciplinary concept. Polar Res. 31:11091. doi: 10.3402/polar.v31i0.11091
Halpern, B. S., Frazier, M., Potapenko, J., Casey, K. S., Koenig, K., Longo, C., et al. (2015). Spatial and temporal changes in cumulative human impacts on the world's ocean. Nat. Commun. 6:7615. doi: 10.1038/ncomms8615
Harris, R. M. B., Grose, M. R., Lee, G., Bindoff, N. L., Porfirio, L. L., and Fox-Hughes, P. (2014). Climate projections for ecologists. Wiley Interdiscipl. Rev. Clim. Change 5, 621–637. doi: 10.1002/wcc.291
Hauck, J., and Völker, C. (2015). Rising atmospheric CO2 leads to large impact of biology on Southern Ocean CO2 uptake via changes of the Revelle factor. Geophys. Res. Lett. 42, 1459–1464. doi: 10.1002/2015GL063070
Hauck, J., Völker, C., Wang, T., Hoppema, M., Losch, M., and Wolf-Gladrow, D. A. (2013). Seasonally different carbon flux changes in the Southern Ocean in response to the southern annular mode. Glob. Biogeochem. Cycles 27, 1236–1245. doi: 10.1002/2013GB004600
Helmuth, B., Mieszkowska, N., Moore, P., and Hawkins, S. J. (2006). Living on the edge of two changing worlds: forecasting the responses of rocky intertidal ecosystems to climate change. Annu. Rev. Ecol. Evol. Syst. 37, 373–404. doi: 10.1146/annurev.ecolsys.37.091305.110149
Hickman, J. E. (2015). Carbon sequestration: biology's growing role. Nat. Geosci. 8, 173–173. doi: 10.1038/ngeo2386
Hill, S. L., Phillips, T., and Atkinson, A. (2013). Potential climate change effects on the habitat of antarctic krill in the weddell quadrant of the southern ocean. PLoS ONE 8:e72246. doi: 10.1371/journal.pone.0072246
Hoegh-Goldberg, O., and Bruno, J. F. (2010). The impact of climate change on the world's marine ecosystems. Science 328, 1523–1528. doi: 10.1126/science.1189930
Hollowed, A. B., Barange, M., Beamish, R. J., Brander, K., Cochrane, K., Drinkwater, K., et al. (2013). Projected impacts of climate change on marine fish and fisheries. ICES J. Mar. Sci. 70, 1023–1037. doi: 10.1093/icesjms/fst081
Hunt, G. L., Drinkwater, K. F., Arrigo, K., Berge, J., Daly, K. L., Danielson, S., et al. (2016). Advection in polar and sub-polar environments: impacts on high latitude marine ecosystems. Prog. Oceanogr. 149, 40–81. doi: 10.1016/j.pocean.2016.10.004
Hunter, C. M., Caswell, H., Runge, M. C., Regehr, E. V., Amstrup, S. C., and Stirling, I. (2010). Climate change threatens polar bear populations: a stochastic demographic analysis. Ecology 91, 2883–2897. doi: 10.1890/09-1641.1
IPCC (2013). “Summary for policymakers,” in Climate Change 2013: The Physical Science Basis. Contribution of Working Group I to the Fifth Assessment Report of the Intergovernmental Panel on Climate Change, eds T. F. Stocker, D. Qin, G.-K. Plattner, M. Tignor, S. K. Allen, J. Boschung, et al. (Cambridge, UK; New York, NY: Cambridge University Press), 3–29.
Jenouvrier, S., Holland, M., Stroeve, J., Serreze, M., Barbraud, C., Weimerskirch, H., et al. (2014). Projected continent-wide declines of the emperor penguin under climate change. Nat. Clim. Chang. 4, 715–718. doi: 10.1038/nclimate2280
Katragkou, E., García-Díez, M., Vautard, R., Sobolowski, S., Zanis, P., Alexandri, G., et al. (2015). Regional climate hindcast simulations within EURO-CORDEX: evaluation of a WRF multi-physics ensemble. Geoscientific Model Dev. 8, 603–618. doi: 10.5194/gmd-8-603-2015
Kawaguchi, S., Ishida, A., King, R., Raymond, B., Waller, N., Constable, A., et al. (2013). Risk maps for Antarctic krill under projected Southern Ocean acidification. Nat. Clim. Chang. 3, 843–847. doi: 10.1038/nclimate1937
Kennicutt, M. C., and Chown, S. L. (2014). Six priorities for Antarctic science. Nature 512, 23–25. doi: 10.1038/512023a
Landschützer, P. N., Gruber, N., Haumann, F. A., Rodenbeck, C., Bakker, D. C. E., van Heuven, S., et al. (2015). The reinvigoration of the Southern Ocean carbon sink. Science 349, 1221–1224. doi: 10.1126/science.aab2620
Larsen, J. N., Anisimov, O. A., Constable, A., Hollowed, A. B., Maynard, N., Prestrud, P., et al. (2014). “Part B: Regional Aspects. Contribution of Working Group II to the Fifth Assessment Report of the Intergovernmental Panel on Climate Change,” in Climate Change 2014: Impacts, Adaptation, and Vulnerability, eds V. R. Barros, C. B. Field, D. J. Dokken, M. D. Mastrandrea, K. J. Mach, T. E. Bilir, M. Chatterjee, K. L. Ebi, Y. O. Estrada, R. C. Genova, B. Girma, E. S. Kissel, A. N. Levy, S. MacCracken, P. R. Mastrandrea, and L. L. White (Cambridge; New York, NY: Cambridge University Press), 1567–1612.
Le Quere, C., Rodenbeck, C., Buitenhuis, E. T., Conway, T. J., Langenfelds, R., Gomez, A., et al. (2007). Saturation of the southern ocean CO2 sink due to recent climate change. Science 316, 1735–1738. doi: 10.1126/science.1136188
Lima, F. P., Ribeiro, P. A., Queiroz, N., Hawkins, S. J., and Santos, A. M. (2007). Do distributional shifts of northern and southern species of algae match the warming pattern? Glob. Chang. Biol. 13, 2592–2604. doi: 10.1111/j.1365-2486.2007.01451.x
Loeb, V. J., Hofmann, E. E., Klinck, J. M., Holm-Hansen, O., and White, W. B. (2008). ENSO and variability of the Antarctic Peninsula pelagic marine ecosystem. Antarct. Sci. 21, 135. doi: 10.1017/S0954102008001636
Macias, D., Garcia-Gorriz, E., and Stips, A. (2013). Understanding the causes of recent warming of mediterranean waters. How much could be attributed to climate change? PLoS ONE 8:e81591. doi: 10.1371/journal.pone.0081591
Massom, R. A., and Stammerjohn, S. E. (2010). Antarctic sea ice change and variability – Physical and ecological implications. Polar Sci. 4, 149–186. doi: 10.1016/j.polar.2010.05.001
Meehl, G. A., Goddard, L., Murphy, J., Stouffer, R. J., Boer, G., Danabasoglu, G., et al. (2009). Decadal prediction. Bull. Ame. Meteorol. Soc. 90, 1467–1485. doi: 10.1175/2009BAMS2778.1
Meijers, A. J. (2014). The Southern ocean in the coupled model intercomparison project phase 5. Philos. Trans. A Math. Phys. Eng. Sci. 372:20130296. doi: 10.1098/rsta.2013.0296
Meinshausen, M., Smith, S. J., Calvin, K., Daniel, J. S., Kainuma, M. L. T., Lamarque, J. F., et al. (2011). The RCP greenhouse gas concentrations and their extensions from 1765 to 2300. Clim. Change 109, 213–241. doi: 10.1007/s10584-011-0156-z
Melbourne-Thomas, J., Constable, A., Wotherspoon, S., and Raymond, B. (2013). Testing paradigms of ecosystem change under climate warming in Antarctica. PLoS ONE 8:e55093. doi: 10.1371/journal.pone.0055093
Melbourne-Thomas, J., Corney, S. P., Trebilco, R., Meiners, K. M., Stevens, R. P., Kawaguchi, S., et al. (2016). Under ice habitats for Antarctic krill larvae: could less mean more under climate warming? Geophys. Res. Lett. 43, 10322–10327. doi: 10.1002/2016GL070846
Mieszkowska, N., Kendall, M. A., Hawkins, S. J., Leaper, R., Williamson, P., Hardman-Mountford, N. J., et al. (2006). Changes in the range of some common rocky shore species in Britain – a response to climate change? Hydrobiologia 555, 241–251. doi: 10.1007/s10750-005-1120-6
Murphy, E. J., Cavanagh, R. D., Hofmann, E. E., Hill, S. L., Constable, A. J., Costa, D. P., et al. (2012). Developing integrated models of Southern Ocean food webs: including ecological complexity, accounting for uncertainty and the importance of scale. Prog. Oceanogr. 102, 74–92. doi: 10.1016/j.pocean.2012.03.006
Murphy, E. J., Cavanagh, R. D., Johnston, N. M., Reid, K., and Hofmann, E. E. (2008). Integrating Climate and Ecosystem Dynamics in the Southern Ocean (ICED) Science Plan and Implementation Strategy. Plymouth; Bergen: GLOBEC; IMBER.
Murphy, E. J., Clarke, A., Abram, N. J., and Turner, J. (2014). Variability of sea-ice in the northern Weddell Sea during the 20th century. J. Geophys. Res. Oceans 119, 4549–4572. doi: 10.1002/2013JC009511
Murphy, E. J., Hofmann, E. E., Watkins, J. L., Johnston, N. M., Pinones, A., Ballerini, T., et al. (2013). Comparison of the structure and function of Southern Ocean regional ecosystems: the Antarctic Peninsula and South Georgia. J. Mar. Syst. 109–110, 22–42. doi: 10.1016/j.jmarsys.2012.03.011
Murphy, E. J., Watkins, J. L., Trathan, P. N., Reid, K., Meredith, M. P., Thorpe, S. E., et al. (2007). Spatial and temporal operation of the Scotia Sea ecosystem: a review of large-scale links in a krill centred food web. Philos. Trans. R. Soc. B. Lond. Biol. Sci. 362, 113–148. doi: 10.1098/rstb.2006.1957
Nicol, S., Pauly, T., Bindoff, N., Wright, S., Thiele, D., Hosie, G. W., et al. (2000). Ocean circulation off east Antarctica affects ecosystem structure and sea-ice extent. Nature 406, 504–507. doi: 10.1038/35020053
Noad, M., Dunlop, R., Paton, D., and Kniest, H. (2011). “Abundance estimates of the east Australian humpback whale population: 2010 survey,” in Final Report for the Australian Marine Mammal Centre (Australian Antarctic Division).
O'Kane, T. J., Matear, R. J., Chamberlain, M. A., Risbey, J. S., Sloyan, B. M., and Horenko, I. (2013). Decadal variability in an OGCM Southern Ocean: intrinsic modes, forced modes and metastable states. Ocean Model. 69, 1–21. doi: 10.1016/j.ocemod.2013.04.009
Olsen, A., Key, R. M., Van Heuven, S., Lauvset, S. K., Velo, A., Lin, X., et al. (2016). The Global Ocean Data Analysis Project version 2 (GLODAPv2) – an internally consistent data product for the world ocean. Earth Syst. Sci. Data 8, 297–323. doi: 10.5194/essd-8-297-2016
Passow, U., and Carlson, C. A. (2012). The biological pump in a high CO2 world. Mar. Ecol. Prog. Ser. 470, 249–271. doi: 10.3354/meps09985
Peck, L. S. (2011). Organisms and responses to environmental change. Mar. Genomics 4, 237–243. doi: 10.1016/j.margen.2011.07.001
Pinkerton, M. H., Bradford-Grieve, J. M., and Hanchet, S. M. (2010). A balanced model of the food web of the Ross Sea, Antarctica. CCAMLR Sci. 17, 1–31.
Piñones, A., and Fedorov, A. V. (2016). Projected changes of Antarctic krill habitat by the end of the 21st century. Geophys. Res. Lett. 43, 8580–8589. doi: 10.1002/2016GL069656
Rintoul, S. R., Sparrow, M., Meredith, M. P., Wadley, V., Speer, K., Hofmann, E., et al. (2012). The Southern Ocean Observing System: Initial Science and Implementation Strategy. Cambridge, UK: SCAR.
Risbey, J. S., Lewandowsky, S., Langlais, C., Monselesan, D. P., O'Kane, T. J., and Oreskes, N. (2014). Well-estimated global surface warming in climate projections selected for ENSO phase. Nat. Clim. Chang 4, 835–840. doi: 10.1038/nclimate2310
Simmonds, I. (2015). Comparing and contrasting the behaviour of Arctic and Antarctic sea ice over the 35 year period 1979–2013. Ann. Glaciol. 56, 18–28. doi: 10.3189/2015AoG69A909
Smith, W. O., Ainley, D. G., and Cattaneo-Vietti, R. (2007). Trophic interactions within the Ross Sea continental shelf ecosystem. Philos. Trans. R. Soc. B. Lond. Biolol. Sci. 362, 95–111. doi: 10.1098/rstb.2006.1956
Smith, W. O., Dinniman, M. S., Hofmann, E. E., and Klinck, J. M. (2014). The effects of changing winds and temperatures on the oceanography of the Ross Sea in the 21st century. Geophys. Res. Lett. 41, 1624–1631. doi: 10.1002/2014GL059311
Snover, A. K., Mantua, N. J., Littell, J. S., Alexander, M. A., McClure, M. M., and Nye, J. (2013). Choosing and using climate-change scenarios for ecological-impact assessments and conservation decisions. Conserv. Biol. 27, 1147–1157. doi: 10.1111/cobi.12163
Southward, A. J., Hawkins, S. J., and Burrows, M. T. (1995). Seventy years' observations of changes in disrtibution and abundance of zooplankton and intertidal organisms in the western English Channel in relation to rising sea temperatures. J. Therm. Biol. 20, 127–155. doi: 10.1016/0306-4565(94)00043-I
Stock, C. A., Alexander, M. A., Bond, N. A., Brander, K. M., Cheung, W., Curchitser, E. N., et al. (2011). On the use of IPCC-class models to assess the impact of climate on Living Marine Resources. Prog. Oceanogr. 88, 1–27. doi: 10.1016/j.pocean.2010.09.001
Sydeman, W. J., Poloczanska, E., Reed, T. E., and Thompson, S. A. (2015). Climate change and marine vertebrates. Science 350, 772–777. doi: 10.1126/science.aac9874
Taylor, K. E., Stouffer, R. J., and Meehl, G. A. (2012). An overview of CMIP5 and the experiment design. Bull. Ame. Meteorol. Soc. 93, 485–498. doi: 10.1175/BAMS-D-11-00094.1
Thompson, D. W. J., Solomon, S., Kushner, P. J., England, M. H., Grise, K. M., and Karoly, D. J. (2011). Signatures of the Antarctic ozone hole in Southern Hemisphere surface climate change. Nat. Geosci. 4, 741–749. doi: 10.1038/ngeo1296
Thorpe, S. E., Murphy, E. J., and Watkins, J. L. (2007). Circumpolar connections between Antarctic krill (Euphausia superba Dana) populations: investigating the roles of ocean and sea ice transport. Deep Sea Res. Part I Oceanogr. Res. Pap. 54, 792–810. doi: 10.1016/j.dsr.2007.01.008
Trathan, P. N., and Agnew, D. (2010). Climate change and the Antarctic marine ecosystem: an essay on management implications. Antarct. Sci. 22, 387–398. doi: 10.1017/S0954102010000222
Tulloch, V. J. D., Plagányi, É. E., Matear, R., Brown, C. J., and Richardson, A. J. (2017). Ecosystem modelling to quantify the impact of historical whaling on Southern Hemisphere baleen whales. Fish Fish. doi: 10.1111/faf.12241. [Epub ahead of print].
Turner, J., Bracegirdle, T. J., Phillips, T., Marshall, G. J., and Hosking, J. S. (2013). An initial assessment of Antarctic Sea ice extent in the CMIP5 models. J. Clim. 26, 1473–1484. doi: 10.1175/JCLI-D-12-00068.1
Turner, J., Comiso, J. C., Marshall, G. J., Lachlan-Cope, T. A., Bracegirdle, T., Maksym, T., et al. (2009). Non-annular atmospheric circulation change induced by stratospheric ozone depletion and its role in the recent increase of Antarctic sea ice extent. Geophys. Res. Lett. 36:L08502. doi: 10.1029/2009GL037524
Turner, J., Lu, H., White, I., King, J. C., Phillips, T., Hosking, J. S., et al. (2016). Absence of 21st century warming on Antarctic Peninsula consistent with natural variability. Nature 535, 411–415. doi: 10.1038/nature18645
Wang, M., and Overland, J. E. (2009). A sea ice free summer Arctic within 30 years? Geophys. Res. Lett. 36:L07502. doi: 10.1029/2009GL037820
Keywords: IPCC, CMIP5, climate models, Southern Ocean, marine ecosystems, climate change, sea ice
Citation: Cavanagh RD, Murphy EJ, Bracegirdle TJ, Turner J, Knowland CA, Corney SP, Smith WO Jr, Waluda CM, Johnston NM, Bellerby RGJ, Constable AJ, Costa DP, Hofmann EE, Jackson JA, Staniland IJ, Wolf-Gladrow D and Xavier JC (2017) A Synergistic Approach for Evaluating Climate Model Output for Ecological Applications. Front. Mar. Sci. 4:308. doi: 10.3389/fmars.2017.00308
Received: 13 June 2017; Accepted: 08 September 2017;
Published: 26 September 2017.
Edited by:
Elvira S. Poloczanska, Alfred-Wegener-Institut für Polar- und Meeresforschung, GermanyReviewed by:
Diego M. Macias, European Commission. Joint Research Center, ItalyNova Mieszkowska, Marine Biological Association of the United Kingdom, United Kingdom
Copyright © 2017 Cavanagh, Murphy, Bracegirdle, Turner, Knowland, Corney, Smith, Waluda, Johnston, Bellerby, Constable, Costa, Hofmann, Jackson, Staniland, Wolf-Gladrow, Xavier. This is an open-access article distributed under the terms of the Creative Commons Attribution License (CC BY). The use, distribution or reproduction in other forums is permitted, provided the original author(s) or licensor are credited and that the original publication in this journal is cited, in accordance with accepted academic practice. No use, distribution or reproduction is permitted which does not comply with these terms.
*Correspondence: Rachel D. Cavanagh, rcav@bas.ac.uk
†Present Address: Cheryl A. Knowland, King's College London, Graduate School, London, United Kingdom